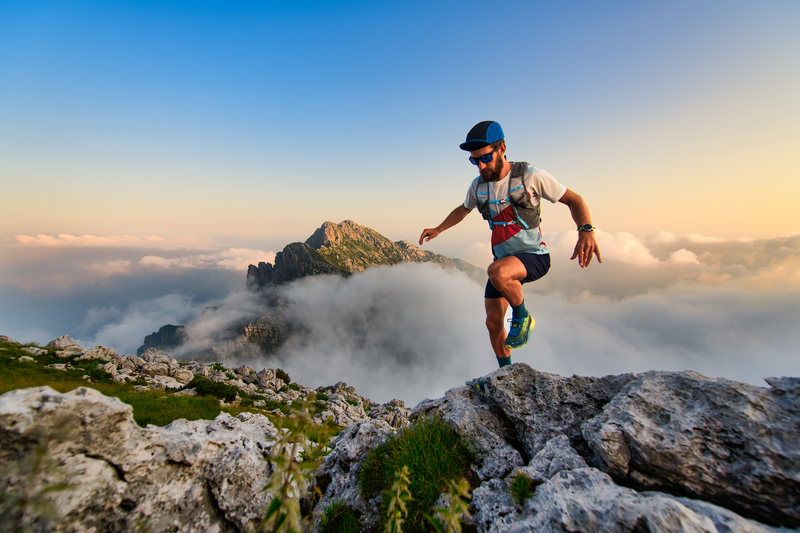
95% of researchers rate our articles as excellent or good
Learn more about the work of our research integrity team to safeguard the quality of each article we publish.
Find out more
REVIEW article
Front. Oncol. , 31 August 2021
Sec. Cancer Imaging and Image-directed Interventions
Volume 11 - 2021 | https://doi.org/10.3389/fonc.2021.722703
This article is part of the Research Topic Imaging Elasticity as a Tangible Prognostic Factor in Cancers View all 6 articles
The change in tissue stiffness caused by pathological changes in the tissue’s structure could be detected earlier, prior to the manifestation of their clinical features. Magnetic resonance elastography (MRE) is a noninvasive imaging technique that uses low-frequency vibrations to quantitatively measure the elasticity or stiffness of tissues. In tumor tissue, stiffness is directly related to tumor development, invasion, metastasis, and chemoradiotherapy resistance. It also dictates the choice of surgical method. At present, MRE is widely used in assessing different human organs, such as the liver, brain, breast, prostate, uterus, gallbladder, and colon stiffness. In the field of oncology, MRE’s value lies in tumor diagnosis (especially early diagnosis), selection of treatment method, and prognosis evaluation. This article summarizes the principle of MRE and its research and application progress in tumor diagnosis and treatment.
Stiffness is an important mechanical parameter and one of the physical properties of human tissues, closely related to biological characteristics (1, 2). Different tissues or organs have different degrees of stiffness (see Figure 1A). It was found that the elasticity of tumor cells (0.05-3.0 kPa) was lower than that of normal human cells (0.75-90 kPa), while the stiffness of tumor tissue is higher than that of normal tissue (3, 4). This is due to the rapid proliferation of tumor cells and increased cell density after normal tissues have become cancerous; at the same time, a large amount of microvascular reconstruction and reduction of normal gland structure has resulted in tumor tissues becoming harder and tougher than the surrounding normal tissues (5). The infiltration of tumor tissue into the surrounding tissue also leads to increased collagen deposition (6, 7). In addition, microvascular pressure, abnormal blood flow, lymphatic and vascular leakage, and increased osmotic pressure in the interstitial space are also factors that increase the stiffness of tumor tissues. In sum, the abnormal structure and composition increased the stiffness of the tumor tissue. Therefore, studying the stiffness of tumor tissue leads to better understanding of its features and behavior, especially in terms of local invasion, distant metastasis, and chemoradiotherapy resistance. More importantly, it will influence the choice of surgical method to be used as part of the treatment (8–10).
Elastography, also known as “palpation by imaging,” was proposed by Ophir et al. in 1991 (11). It was first used in ultrasound, but its accuracy in assessing tissue elasticity was poor due to factors such as increased subjectivity and lack of uniform operating specifications. MRE is a noninvasive imaging technique that quantifies the elastic characteristics of tissues. It has been used in clinical research and application that involve different kinds of tumors. It is considered an ideal diagnostic tool because it is safe to use, noninvasive, highly repeatable, produces reliable results, less operator-dependent, and provides a clear, highly detailed images of tumors (12).
MRE is based on magnetic resonance technology. First, it applies continuous and dynamic mechanical shear waves to the target tissue through an exciter. Then, the motion-sensitive gradient of magnetic resonance (MR) is used to obtain the phase distribution information of the mass points in the tissue. By doing this, the phase and wave patterns of the dynamic shear wave propagation are obtained. Finally, both the full amplitude and full quantitative color-coded elastic graph are generated by the inversion algorithm of the waveform map through the elastic imaging software (see Figure 1B) (8, 13). The MRE imaging process can be summarized as follows: 1) Shear waves are generated in the tissue; 2) MR images reflecting the propagation of the shear waves are created; 3) After processing the shear wave image, the elastogram quantifying the stiffness of the tissue is obtained (9). By selecting any region of interest (ROI) in the graph, the elasticity value (in kiloPascal or kPa) in the region can be obtained to quantitatively evaluate the elasticity attribute. MRE can combine different magnetic resonance imaging (MRI) sequences to acquire signals, such as gradient echo (GRE), spin-echo (SE), spin-echo echo-planar imaging (SE-EPI), and balanced steady-state free precession (bSSFP) (13–16). At present, the most commonly used clinically is the traditional 60 Hz 2D gradient echo (GRE) sequence. Compared with the GRE sequence, SE-EPI has the advantage of short scanning time, high image quality and success rate of tumor detection. This makes it highly favorable to researchers (17, 18). MRE that combines SE-EPI sequence and 3D increases spatial coverage and reduces the errors caused by oblique wave propagation and edge artifacts, so the measured values obtained are more stable and accurate (19, 20). In addition, the SE-EPI sequence is less sensitive to liver iron overload and requires less patient cooperation, both of which make obtaining data faster (21). 3D-MRE’s current scanning time is long, which makes it less ideal to use (19). However, at the rate that technological advances are happening right now, it would not be long before 3D-MRE becomes the main application of MRE.
Changes in the elasticity and stiffness of human tissues reflect the pathological development process to a certain extent (22). For example, 80–90% of patients with liver cirrhosis will develop liver cancer, with tumor stiffness directly related to tumor grade (23–25). In addition, high tumor stiffness hinders the effective delivery of anti-cancer drugs in the body (26–28). Accurately identifying and reducing elastic stiffness between tissues contribute to the efficient delivery of drugs in patients undergoing treatment (29, 30). MRE technology provides a new strategy for the precise diagnosis and treatment of tumors.
Currently, MRE is extensively applied in the diagnosis of liver diseases. It is used in accurately assessing normal liver parenchyma, liver fibrosis, focal nodular hyperplasia, and liver cancer (22). MRE effectively identifies the stages of liver fibrosis and detects early liver cirrhosis (see Table 1). The sensitivity of MRE in distinguishing between severe and mild liver fibrosis is 98%. The increase in liver stiffness in patients with liver cirrhosis is an important risk factor for developing hepatocellular carcinoma (HCC) (24).
Richard et al. evaluated 29 patients with 44 liver tumors using MRE with improved gradient echo sequence. The results showed that the average stiffness of malignant liver tumor (10.1 kPa) was higher than that of benign liver tumor (2.7 kPa), liver fibrosis (5.9 kPa), and normal liver parenchyma (2.3 kPa) (45). There was no significant difference in shear stiffness between benign liver tumors and normal liver parenchyma. They initially determined that the stiffness value of the liver was 5 kPa, which was the critical value that distinguishes malignant liver tumor from benign liver tumor or normal liver parenchyma (see Figures 2A, B).
Figure 2 (A) MRE of liver tumors. The stiffness value of benign tumors (focal nodular hyperplasia, a-c; liver adenoma, d-f) is equivalent to that of normal liver parenchyma (generally less than 5 kPa), while the stiffness of malignant tumors (hepatocellular carcinoma-cholangiocarcinoma, g-i; colorectal cancer metastasis, j-l) is increased Reproduced with permission from ref. (46), copyright 2013, John Wiley & Sons Inc. (B) Shear stiffness of different tissues. Reproduced with permission from ref. (45), copyright 2008, American Roentgen Ray Society. (C) Schematic diagram of HCC tumor grade corresponding to tumor stiffness (kPa). Reproduced with permission from ref. 23, copyright 2017, Elsevier.
Garteiser et al. found that the loss modulus of hepatocellular carcinomas was significantly higher than that of benign hepatocellular tumors (47). In addition, MRE can also be used to initially assess the level of hepatocellular carcinoma (HCC). For example, compared with poorly differentiated liver cancer (4.9 ± 1.2 kPa; N = 8), well-differentiated/moderately differentiated HCC (6.5 ± 1.2 kPa; N = 13) tumor stiffness increased significantly (see Figure 2C). However, there is no exact correlation between the stiffness of liver tumors and tumor size. MRE is a promising diagnostic technique for evaluating solid liver tumors.
Liver stiffness measured by MRE can be used to predict the early recurrence of liver tumors after treatment. The stiffness of liver tumors is an independent factor in the early recurrence of HCC (48). For every 1 kPa increase in tumor stiffness, the risk of tumor recurrence increased by 16.3%. The relapsed HCC has higher tumor stiffness (49). Liver stiffness measured by MRE can also be used as a prognostic indicator for HCC patients undergoing hepatectomy. Liver stiffness (≥ 4.02 kPa) was the only important factor for poor overall survival (OS) (50). Meanwhile, the value of liver stiffness is negatively correlated with the regenerating ability of the residual liver after hepatectomy (51). Therefore, liver stiffness measured by MRE can be used to predict liver regeneration in patients with liver cirrhosis and liver cancer. Moreover, MRE data of patients with colorectal liver metastases treated by transcatheter arterial chemoembolization (TACE) showed that the stiffness of the metastases was higher (P< 0.001) (52). MRE provides a reference value for the treatment of patients with liver metastasis.
MRE imaging of the breast requires low-frequency emission (generally, 40–100 Hz). Ehman et al. detected MRE with 100 Hz shear wave in healthy volunteers and breast cancer patients. The results showed that the average elastic values of normal adipose tissue, fibroglandular tissue, and tumor tissue were 3.3 kPa, 7.5 kPa, and 33 kPa, respectively (see Figure 3A) (53). Between these elastic values, the stiffness of breast cancer tissue is four times that of normal fibroglandular tissue. Meanwhile, Lorenzen et al. found that the median elasticity of breast adipose tissue, breast parenchyma, benign tumor tissue, and malignant tumor tissue were 1.7 kPa, 2.5 kPa, 7.0 kPa, and 15.9 kPa, respectively (see Figure 3B) (54). The elasticity value of breast cancer tissue was higher than that of normal tissue around the tumor, benign tumor, and normal breast tissue (55).
Figure 3 (A) Shear stiffness estimates of different types of breast tissue (mean value). Reproduced with permission from ref. (53), copyright 2002, American Roentgen Ray Society; (B) MRE–based elasticity values for malignant, benign, parenchyma, fatty, and stiff lesions (median). Reproduced with permission from ref. (54), copyright 2002, Georg Thieme Verlag KG.
Balleyguier et al. studied 43 breast tumor patients with high scores in the breast imaging report and data system (BI-RADS), and found that the sensitivity, specificity, positive predictive value, and negative predictive value of MRE for breast cancer diagnosis were 79%, 90%, 96%, and 56%, respectively. MRE with an AUC (Area under the curve) value of 0.92 as compared with 0.84 for MRI alone (56). Sinkus et al. observed an increase in specificity from 40 to 60% at 100% sensitivity, and Siegmann et al. also improved the specificity from 75% to 90% while maintaining a high sensitivity of 90% (57, 58). In these studies, MRE improved the accuracy of MRI in diagnosing breast cancer. This is because the elasticity of benign and malignant tumors overlaps. MRI alone cannot provide an accurate diagnosis; it needs to be combined with viscoelastic parameters (elasticity, viscosity, etc.) in order to provide a more comprehensive evaluation. It is also necessary to reduce scanning time and improve spatial resolution in the future to promote the clinical application of MRE in the diagnosis of breast cancer (59).
MRE possesses significant clinical value as a tool in the diagnosis and treatment of brain tumors. The nondestructive conduction of shear waves into the skull is the key to this (60). Wuerfel et al. first discovered the correlation between brain stiffness and pathological process sensitivity in multiple sclerosis (MS). The average shear modulus of the white matter and gray matter of a normal human brain, independent of age, are 14.8 and 5.22 kPa, respectively (61).
One of the most important factors determining the difficulty of brain tumor resection is the consistency of the tumors. Several studies have shown that the shear stiffness of meningiomas and pituitary adenomas measured by MRE is closely related to the subjective assessment of tumor consistency by surgeons during surgery (62) (see Figure 4A). The sensitivity, specificity, positive predictive value, and negative predictive value of MRE for judging meningioma heterogeneity are 75%, 100%, 100%, and 87%, respectively; while the sensitivity, specificity, positive predictive value, and negative predictive value of MRE for judging tumor stiffness are 60%, 100%, 100%, and 56%, respectively (7). Another important factor affecting brain tumor resection is tumor adhesion. The slip interface imaging (SII) technology that was developed based on MRE is a noninvasive method of assessing the degree of adhesion of meningioma to adjacent brain tissues (63–65). SII’s ability to strongly predict tumor compliance indicates that it is a promising technique for surgical planning to help predict the duration and risk of surgery (see Figure 4B) (63).
Figure 4 (A) Brain imaging of stiff meningioma subjects (top row) and soft meningioma subjects (bottom row). The area encircled by the green dots represents the location of the tumor. Normal brain parenchyma, dural meningioma, and soft meningioma have different mechanical properties, indicating the great potential of MRE in brain imaging. Reproduced with permission from ref. (62), copyright 2013, American Association of Neurological Surgeons. (B) The image of a sliding interface in the case of adherent (top) and non-adherent (bottom) meningiomas. In non-adherent cases, the tumor was surrounded by bright rings. Left: T2-weighted fluid-attenuated inversion recovery (FLAIR) images; Right: shear strain maps. Reproduced with permission from ref. (63).
Shear wave excitation devices for prostate MRE have been reported, such as transurethral excitation device, transrectal excitation device, and transperineal excitation device. These devices can observe uniform shear waves in animal and human experiments and have high rate of repeatability (66–69). The results of prostate MRE through perineal stimulation showed that the average elastic value of prostate cancer tissues was different from that of normal tissues, and their diagnostic sensitivity and specificity were 63% and 68%, respectively (5). Initial data showed that the average elasticity values of prostate cancer tissue, prostatitis tissue, and normal prostate tissue were 6.55 kPa, 1.99 kPa, and 2.26 kPa, respectively (70). Salcudean et al. developed a novel active shielding electromagnetic sensor and adopted a fast pulse sequence, which shortened the MRE data acquisition time to 8–10 minutes, making it more acceptable to patients (71).
Recently, Klatt et al. found that MRE can overcome some limitations of MRI in evaluating prostate cancer, such as interobserver variability and low specificity (72). In a 9.4T preclinical scanner, 14 patients with prostate cancer were examined using MRE at 500 Hz immediately after prostatectomy. MRE data showed that the average stiffness of tumor tissue and healthy tissue were 10.84 ± 4.65 and 5.44 ± 4.40 kPa (p ≤ 0.001), respectively, indicating that MRE is a highly promising imaging technique for diagnosing prostate cancer. The Youden index showed that the sensitivity and specificity of diagnosis were 69% and 79%, respectively. In addition, Wang et al. found that the detection of MRE-based prostate cancer (PCa) stiffness may help noninvasively predict the degree of lymph node metastasis prior to surgery, with sensitivity and specificity as high as 100% and 86.5%, respectively (73).
Among the main problems encountered regarding the early diagnosis of pancreatic cancer are early clinical symptoms that are not obvious and the unsuitableness of biopsy or laparotomy. Pancreatic cancer tumor cells infiltrate and grow into surrounding tissues, so they produce a large amount of collagen, which leads to increased stiffness. Generally, the stiffness of pancreatic cancer tissue is 6.06 ± 0.49 kPa, which is higher than that of normal pancreatic tissue (2.47 ± 0.11 kPa, P<0.0001) (74). Guo et al. found that the sensitivity, accuracy, and specificity of MRE in evaluating pancreatic cancer and pancreatitis were higher than 0.9, showing better diagnostic performance than carbohydrate antigen 19-9 (CA199) (75). Low frequency 40 Hz MRE combined with MRI can improve the specificity of diagnosis of pancreatic cancer (96.9% versus 62.1%, P = 0.002) (76). Hence, MRE has important research and clinical application value for the diagnosis and treatment of pancreatic cancer.
MRE has also been used in the diagnosis of myoma, colorectal cancer, and thyroid tumors (77–81). Overall research shows that MRE can accurately distinguish between benign and malignant tumors, which helps improve the specificity and sensitivity of tumor diagnosis.
MRE is a noninvasive technology that can improve the diagnosis and treatment of malignant tumors. It has gradually become a new research method in the field of oncology. MRE has the advantage of being highly accurate, producing clearer results, being highly repeatable, and having a high success rate. It can better assess the characteristics of malignant tumors, so that the best treatment and surgical methods are identified and applied. However, MRE technology still has many questions to be answered. Biological tissues filter the spectrum out of control, how does this frequency filter affect the measurement result? What is the role and relationship between stiffness, elasticity, and viscosity? The urgent challenge is to standardize the technology and to standardize the MRE examination and the “units” used. So far, elastography still without strict measurement conditions, MRE provides stiffness in kPa by calculating the shear modulus, while transient elastography provides Young’s modulus, which is nearly three times the shear modulus. Not to mention the limitations of the clinical application of tumor MRE: 1) vulnerability to respiratory factors and artifacts when diagnosing liver cancer and breast cancer; 2) limited ability to track calcified lesions; 3) low spatial resolution; 4) high iron content affecting data acquisition of liver MRE; 5) estimation error caused by different measurement parameters, such as field strength, scanning sequence, and shear wave frequency; 6) human error when processing and analyzing the image. Given these current limitations, it is necessary to further improve the MRE’s in vitro excitation device, optimize the acquisition parameters and stimulation frequency, and combine multiple parameters for comprehensive diagnosis. When these technical issues are addressed, with the application of artificial intelligence and machine learning in medical image processing, the MRE’s wider clinical application, especially in the field of oncology, will be fully realized.
J-YY: project idea and writing of the manuscript. J-YY and B-SQ: project idea, project planning, and review of the manuscript. All authors contributed to the article and approved the submitted version.
The authors declare that the research was conducted in the absence of any commercial or financial relationships that could be construed as a potential conflict of interest.
All claims expressed in this article are solely those of the authors and do not necessarily represent those of their affiliated organizations, or those of the publisher, the editors and the reviewers. Any product that may be evaluated in this article, or claim that may be made by its manufacturer, is not guaranteed or endorsed by the publisher.
1. Handorf AM, Zhou Y, Halanski MA, Li W-J. Tissue Stiffness Dictates Development, Homeostasis, and Disease Progression. Organogenesis (2015) 11(1):1–15. doi: 10.1080/15476278.2015.1019687
2. d’Angelo M, Benedetti E, Tupone MG, Catanesi M, Castelli V, Antonosante A, et al. The Role of Stiffness in Cell Reprogramming: A Potential Role for Biomaterials in Inducing Tissue Regeneration. Cells (2019) 8(9):1036. doi: 10.3390/cells8091036
3. Li QS, Lee GY, Ong CN, Lim CT. AFM Indentation Study of Breast Cancer Cells. Biochem Biophys Res Commun (2008) 374(4):609–13. doi: 10.1016/j.bbrc.2008.07.078
4. Suresh S. Biomechanics and Biophysics of Cancer Cells. Acta Biomater (2007) 3(4):413–38. doi: 10.1016/j.actbio.2007.04.002
5. Sahebjavaher RS, Nir G, Honarvar M, Gagnon LO, Ischia J, Jones EC, et al. MR Elastography of Prostate Cancer: Quantitative Comparison With Histopathology and Repeatability of Methods. NMR Biomed (2015) 28(1):124–39. doi: 10.1002/nbm.3218
6. Xu S, Xu H, Wang W, Li S, Li H, Li T, et al. The Role of Collagen in Cancer: From Bench to Bedside. J Transl Med (2019) 17(1):309. doi: 10.1186/s12967-019-2058-1
7. Hughes JD, Fattahi N, Van Gompel J, Arani A, Meyer F, Lanzino G, et al. Higher-Resolution Magnetic Resonance Elastography in Meningiomas to Determine Intratumoral Consistency. Neurosurgery (2015) 77(4):653–9. doi: 10.1227/NEU.0000000000000892
8. Huang J, Zhang L, Wan D, Zhou L, Zheng S, Lin S, et al. Extracellular Matrix and Its Therapeutic Potential for Cancer Treatment. Signal Transduct Target Ther (2021) 6(1):153. doi: 10.1038/s41392-021-00544-0
9. Reid SE, Kay EJ, Neilson LJ, Henze A-T, Serneels J, McGhee EJ, et al. Tumor Matrix Stiffness Promotes Metastatic Cancer Cell Interaction With the Endothelium. EMBO J (2017) 36(16):2373–89. doi: 10.15252/embj.201694912
10. Joyce MH, Lu C, James ER, Hegab R, Allen SC, Suggs LJ, et al. Phenotypic Basis for Matrix Stiffness-Dependent Chemoresistance of Breast Cancer Cells to Doxorubicin. Front Oncol (2018) 8:337. doi: 10.3389/fonc.2018.00337
11. Ophir J, Céspedes I, Ponnekanti H, Yazdi Y, Li X. Elastography: A Quantitative Method for Imaging the Elasticity of Biological Tissues. Ultrason Imaging. (1991) 13(2):111–34. doi: 10.1177/016173469101300201
12. Lee Y, Lee JM, Lee JE, Lee KB, Lee ES, Yoon JH, et al. MR Elastography for Noninvasive Assessment of Hepatic Fibrosis: Reproducibility of the Examination and Reproducibility and Repeatability of the Liver Stiffness Value Measurement. J Magn Reson Imaging (2014) 39(2):326–31. doi: 10.1002/jmri.24147
13. Morisaka H, Motosugi U, Glaser KJ, Ichikawa S, Ehman RL, Sano K, et al. Comparison of Diagnostic Accuracies of Two- and Three-Dimensional MR Elastography of the Liver. J Magn Reson Imaging (2017) 45(4):1163–70. doi: 10.1002/jmri.25425
14. Wagner M, Besa C, Bou Ayache J, Yasar TK, Bane O, Fung M, et al. Magnetic Resonance Elastography of the Liver: Qualitative and Quantitative Comparison of Gradient Echo and Spin Echo Echoplanar Imaging Sequences. Invest Radiol (2016) 51(9):575–81. doi: 10.1097/rli.0000000000000269
15. Mariappan YK, Dzyubak B, Glaser KJ, Venkatesh SK, Sirlin CB, Hooker J, et al. Application of Modified Spin-Echo-Based Sequences for Hepatic MR Elastography: Evaluation, Comparison With the Conventional Gradient-Echo Sequence, and Preliminary Clinical Experience. Radiology (2017) 282(2):390–8. doi: 10.1148/radiol.2016160153
16. Shi Y, Xia F, Li QJ, Li JH, Yu B, Li Y, et al. Magnetic Resonance Elastography for the Evaluation of Liver Fibrosis in Chronic Hepatitis B and C by Using Both Gradient-Recalled Echo and Spin-Echo Echo Planar Imaging: A Prospective Study. Am J Gastroenterol (2016) 111(6):823–33. doi: 10.1038/ajg.2016.56
17. Hoodeshenas S, Yin M, Venkatesh SK. Magnetic Resonance Elastography of Liver: Current Update. Top Magn Reson Imaging (2018) 27(5):319–33. doi: 10.1097/rmr.0000000000000177
18. Mathew RP, Venkatesh SK. Imaging of Hepatic Fibrosis. Curr Gastroenterol Rep (2018) 20(10):45. doi: 10.1007/s11894-018-0652-7
19. Castera L, Friedrich-Rust M, Loomba R. Noninvasive Assessment of Liver Disease in Patients With Nonalcoholic Fatty Liver Disease. Gastroenterology (2019) 156(5):1264–81.e4. doi: 10.1053/j.gastro.2018.12.036
20. Tang A, Cloutier G, Szeverenyi NM, Sirlin CB. Ultrasound Elastography and MR Elastography for Assessing Liver Fibrosis: Part 1, Principles and Techniques. Am J Roentgenol (2015) 205(1):22–32. doi: 10.2214/ajr.15.14552
21. Calle-Toro JS, Serai SD, Hartung EA, Goldberg DJ, Bolster BD Jr, Darge K, et al. Magnetic Resonance Elastography SE-EPI vs GRE Sequences at 3T in a Pediatric Population With Liver Disease. Abdom Radiol (NY) (2019) 44(3):894–902. doi: 10.1007/s00261-018-1884-6
22. Herron TJ. Stiffness Triggers Non-Coding RNA Pathology. Nat BioMed Eng (2019) 3(2):85–6. doi: 10.1038/s41551-019-0352-0
23. Thompson SM, Wang J, Chandan VS, Glaser KJ, Roberts LR, Ehman RL, et al. MR Elastography of Hepatocellular Carcinoma: Correlation of Tumor Stiffness With Histopathology Features-Preliminary Findings. Magn Reson Imaging (2017) 37:41–5. doi: 10.1016/j.mri.2016.11.005
24. Yoo J, Seo BK, Park EK, Kwon M, Jeong H, Cho KR, et al. Tumor Stiffness Measured by Shear Wave Elastography Correlates With Tumor Hypoxia as Well as Histologic Biomarkers in Breast Cancer. Cancer Imaging (2020) 20(1):85. doi: 10.1186/s40644-020-00362-7
25. Huo TI, Liu PH, Hsu CY. Predicting Post-Resection Recurrence of Hepatocellular Carcinoma: Spleen Stiffness vs. ALBI Grade. J Hepatol (2019) 70(4):808. doi: 10.1016/j.jhep.2018.11.020
26. Chen WL, Simmons CA. Lessons From (Patho)Physiological Tissue Stiffness and Their Implications for Drug Screening, Drug Delivery and Regenerative Medicine. Adv Drug Deliv Rev (2011) 63(4-5):269–76. doi: 10.1016/j.addr.2011.01.004
27. Bhaw-Luximon A, Jhurry D. New Avenues for Improving Pancreatic Ductal Adenocarcinoma (PDAC) Treatment: Selective Stroma Depletion Combined With Nano Drug Delivery. Cancer Lett (2015) 369(2):266–73. doi: 10.1016/j.canlet.2015.09.007
28. Nicolas-Boluda A, Silva AKA, Fournel S, Gazeau F. Physical Oncology: New Targets for Nanomedicine. Biomaterials (2018) 150:87–99. doi: 10.1016/j.biomaterials.2017.10.014
29. Nia HT, Munn LL, Jain RK. Mapping Physical Tumor Microenvironment and Drug Delivery. Clin Cancer Res (2019) 25(7):2024–6. doi: 10.1158/1078-0432.Ccr-18-3724
30. Qin X, Lv X, Li P, Yang R, Xia Q, Chen Y, et al. Matrix Stiffness Modulates ILK-Mediated YAP Activation to Control the Drug Resistance of Breast Cancer Cells. Biochim Biophys Acta Mol Basis Dis (2020) 1866(3):165625. doi: 10.1016/j.bbadis.2019.165625
31. Park CC, Nguyen P, Hernandez C, Bettencourt R, Ramirez K, Fortney L, et al. Magnetic Resonance Elastography vs Transient Elastography in Detection of Fibrosis and Noninvasive Measurement of Steatosis in Patients With Biopsy-Proven Nonalcoholic Fatty Liver Disease. Gastroenterology (2017) 152(3):598–607(e2). doi: 10.1053/j.gastro.2016.10.026
32. Cui J, Ang B, Haufe W, Hernandez C, Verna E, Sirlin C, et al. Comparative Diagnostic Accuracy of Magnetic Resonance Elastography vs. Eight Clinical Prediction Rules for Non-Invasive Diagnosis of Advanced Fibrosis in Biopsy-Proven Non-Alcoholic Fatty Liver Disease: A Prospective Study. Aliment Pharmacol Ther (2015) 41(12):1271–80. doi: 10.1111/apt.13196
33. Costa-Silva L, Ferolla SM, Lima AS, Vidigal PVT, Ferrari TCDA. MR Elastography Is Effective for the Non-Invasive Evaluation of Fibrosis and Necroinflammatory Activity in Patients With Nonalcoholic Fatty Liver Disease. Eur J Radiol (2018) 98:82–9. doi: 10.1016/j.ejrad.2017.11.003
34. Kim D, Kim WR, Talwalkar JA, Kim HJ, Ehman RL. Advanced Fibrosis in Nonalcoholic Fatty Liver Disease: Noninvasive Assessment With MR Elastography(Article). Radiology (2013) 268(2):411–9. doi: 10.1148/radiol.13121193
35. Jayakumar S, Middleton MS, Lawitz EJ, Mantry PS, Caldwell SH, Arnold H, et al. Longitudinal Correlations Between MRE, MRI-PDFF, and Liver Histology in Patients With Non-Alcoholic Steatohepatitis: Analysis of Data From a Phase II Trial of Selonsertib. J Hepatol (2019) 70(1):133–41. doi: 10.1016/j.jhep.2018.09.024
36. Han MAT, Vipani A, Noureddin N, Ramirez K, Gornbein J, Saouaf R, et al. MR Elastography-Based Liver Fibrosis Correlates With Liver Events in Nonalcoholic Fatty Liver Patients: A Multicenter Study. Liver Int (2020) 40(9):2242–51. doi: 10.1111/liv.14593
37. Singh S, SKV CA, Wang Z, Miller FH, Motosugi U, Low RN, et al. Diagnostic Performance of Magnetic Resonance Elastography in Staging Liver Fibrosis: A Systematic Review and Meta-Analysis of Individual Participant Data. Clin Gastroenterol Hepatol (2015) 13(3):440–51(e6). doi: 10.1016/j.cgh.2014.09.046
38. Ichikawa SA, Motosugi UA, Ichikawa TA, Sano KA, Morisaka HA, Enomoto NB, et al. Magnetic Resonance Elastography for Staging Liver Fibrosis in Chronic Hepatitis C(Article). Magn Reson Med Sci (2012) 11(4):291–7. doi: 10.2463/mrms.11.291
39. Venkatesh SK, Wang G, Lim SG, Wee A. Magnetic Resonance Elastography for the Detection and Staging of Liver Fibrosis in Chronic Hepatitis B. Eur Radiol (2014) 24(1):70–8. doi: 10.1007/s00330-013-2978-8
40. Wang J, Malik N, Yin M, Smyrk T, Czaja A, Ehman R, et al. Magnetic Resonance Elastography Is Accurate in Detecting Advanced Fibrosis in Autoimmune Hepatitis. World J Gastroenterol (2017) 23(5):859–68. doi: 10.3748/wjg.v23.i5.859
41. Jhaveri KS, Hosseini-Nik H, Sadoughi N, Janssen H, Feld JJ, Fischer S, et al. The Development and Validation of Magnetic Resonance Elastography for Fibrosis Staging in Primary Sclerosing Cholangitis. Eur Radiol (2019) 29(2):1039–47. doi: 10.1007/s00330-018-5619-4
42. Bensamoun SF, Leclerc GE, Debernard L, Cheng X, Robert L, Charleux F, et al. Cutoff Values for Alcoholic Liver Fibrosis Using Magnetic Resonance Elastography Technique. Alcohol Clin Exp Res (2013) 37(5):811–7. doi: 10.1111/acer.12025
43. Nguyen D, Talwalkar JA, Yin M, Lindor KD, Ehman RL. Assessment of Hepatic Fibrosis by Magnetic Resonance Elastography in Patients With Sclerosing Cholangitis. Gastroenterology (2011) 140(5Suppl1):S–919. doi: 10.1016/s0016-5085(11)63811-0
44. Wagner M, Hectors S, Bane O, Gordic S, Kennedy P, Besa C, et al. Noninvasive Prediction of Portal Pressure With MR Elastography and DCE-MRI of the Liver and Spleen: Preliminary Results. J Magn Reson Imaging (2018) 48(4):1091–103. doi: 10.1002/jmri.26026
45. Venkatesh SK, Yin M, Glockner JF, Takahashi N, Araoz PA, Talwalkar JA, et al. MR Elastography of Liver Tumors: Preliminary Results. Am J Roentgenol. (2008) 190(6):1534–40. doi: 10.2214/ajr.07.3123
46. Venkatesh SK, Yin M, Ehman RL. Magnetic Resonance Elastography of Liver: Technique, Analysis, and Clinical Applications. J Magn Reson Imaging (2013) 37(3):544–55. doi: 10.1002/jmri.23731
47. Garteiser P, Doblas S, Daire JL, Wagner M, Leitao H, Vilgrain V, et al. MR Elastography of Liver Tumours: Value of Viscoelastic Properties for Tumour Characterisation. Eur Radiol (2012) 22(10):2169–77. doi: 10.1007/s00330-012-2474-6
48. Cho HJ, Kim B, Kim HJ, Huh J, Kim JK, Lee JH, et al. Liver Stiffness Measured by MR Elastography Is a Predictor of Early HCC Recurrence After Treatment. Eur Radiol (2020) 30(8):4182–92. doi: 10.1007/s00330-020-06792-y
49. Wang J, Shan Q, Liu Y, Yang H, Kuang S, He B, et al. 3d MR Elastography of Hepatocellular Carcinomas as a Potential Biomarker for Predicting Tumor Recurrence. J Magn Reson Imaging (2019) 49(3):719–30. doi: 10.1002/jmri.26250
50. Lee DH, Lee JM, Yi NJ, Lee KW, Suh KS, Lee JH, et al. Hepatic Stiffness Measurement by Using MR Elastography: Prognostic Values After Hepatic Resection for Hepatocellular Carcinoma. Eur Radiol (2017) 27(4):1713–21. doi: 10.1007/s00330-016-4499-8
51. Jang S, Lee JM, Lee DH, Joo I, Yoon JH, Chang W, et al. Value of MR Elastography for the Preoperative Estimation of Liver Regeneration Capacity in Patients With Hepatocellular Carcinoma. J Magn Reson Imaging (2017) 45(6):1627–36. doi: 10.1002/jmri.25517
52. Vogl TJ, Martin SS, Johnson AA, Haas Y. Evaluation of MR Elastography as a Response Parameter for Transarterial Chemoembolization of Colorectal Liver Metastases. Eur Radiol (2020) 30(7):3900–7. doi: 10.1007/s00330-020-06706-y
53. McKnight AL, Kugel JL, Rossman PJ, Manduca A, Hartmann LC, Ehman RL. MR Elastography of Breast Cancer: Preliminary Results. Am J Roentgenol. (2002) 178(6):1411–7. doi: 10.2214/ajr.178.6.1781411
54. Lorenzen J, Sinkus R, Lorenzen M, Dargatz M, Leussler C, Röschmann P, et al. MR Elastography of the Breast: Preliminary Clinical Results. Rofo-Fortschr Gebiet Rontgenstrahlen Bildgeb Verfahr (2002) 174(7):830–4. doi: 10.1055/s-2002-32690
55. Xydeas T, Siegmann K, Sinkus R, Krainick-Strobel U, Miller S, Claussen CD. Magnetic Resonance Elastography of the Breast: Correlation of Signal Intensity Data With Viscoelastic Properties. Invest Radiol (2005) 40(7):412–20. doi: 10.1097/01.rli.0000166940.72971.4a
56. Balleyguier C, Lakhdar AB, Dunant A, Mathieu MC, Delaloge S, Sinkus R. Value of Whole Breast Magnetic Resonance Elastography Added to MRI for Lesion Characterization. NMR Biomed (2018) 31(1):e3795. doi: 10.1002/nbm.3795
57. Sinkus R, Siegmann K, Xydeas T, Tanter M, Claussen C, Fink M. MR Elastography of Breast Lesions: Understanding the Solid/Liquid Duality Can Improve the Specificity of Contrast-Enhanced MR Mammography. Magn Reson Med (2007) 58(6):1135–44. doi: 10.1002/mrm.21404
58. Siegmann KC, Xydeas T, Sinkus R, Kraemer B, Vogel U, Claussen CD. Diagnostic Value of MR Elastography in Addition to Contrast-Enhanced MR Imaging of the Breast-Initial Clinical Results. Eur Radiol (2010) 20(2):318–25. doi: 10.1007/s00330-009-1566-4
59. Patel BK, Samreen N, Zhou Y, Chen J, Brandt K, Ehman R, et al. MR Elastography of the Breast: Evolution of Technique, Case Examples, and Future Directions. Clin Breast Cancer (2021) 21(1):e102–11. doi: 10.1016/j.clbc.2020.08.005
60. Pepin KM, Ehman RL, McGee KP. Magnetic Resonance Elastography (MRE) in Cancer: Technique, Analysis, and Applications. Prog Nucl Magn Reson Spectrosc. (2015) 90–91:32–48. doi: 10.1016/j.pnmrs.2015.06.001
61. Kruse SA, Rose GH, Glaser KJ, Manduca A, Felmlee JP, Jack CR Jr., et al. Magnetic Resonance Elastography of the Brain. Neuroimage (2008) 39(1):231–7. doi: 10.1016/j.neuroimage.2007.08.030
62. Murphy MC, Huston J 3rd, Glaser KJ, Manduca A, Meyer FB, Lanzino G, et al. Preoperative Assessment of Meningioma Stiffness Using Magnetic Resonance Elastography. J Neurosurg (2013) 118(3):643–8. doi: 10.3171/2012.9.Jns12519
63. Murphy MC, Huston J 3rd, Ehman RL. MR Elastography of the Brain and Its Application in Neurological Diseases. Neuroimage (2019) 187:176–83. doi: 10.1016/j.neuroimage.2017.10.008
64. Bunevicius A, Schregel K, Sinkus R, Golby A, Patz S. REVIEW: MR Elastography of Brain Tumors. NeuroImage Clin (2019) 187:176–83. doi: 10.1016/j.neuroimage.2017.10.008
65. Yin Z, Hughes JD, Trzasko JD, Glaser KJ, Manduca A, Van Gompel J, et al. Slip Interface Imaging Based on MR-Elastography Preoperatively Predicts Meningioma-Brain Adhesion. J Magn Reson Imaging (2017) 46(4):1007–16. doi: 10.1002/jmri.25623
66. Chopra R, Arani A, Huang Y, Musquera M, Wachsmuth J, Bronskill M, et al. In Vivo MR Elastography of the Prostate Gland Using a Transurethral Actuator. Magn Reson Med (2009) 62(3):665–71. doi: 10.1002/mrm.22038
67. Arani A, Plewes D, Chopra R. Transurethral Prostate Magnetic Resonance Elastography: Prospective Imaging Requirements. Magn Reson Med (2011) 65(2):340–9. doi: 10.1002/mrm.22633
68. Sahebjavaher RS, Baghani A, Honarvar M, Sinkus R, Salcudean SE. Transperineal Prostate MR Elastography: Initial In Vivo Results. Magn Reson Med (2013) 69(2):411–20. doi: 10.1002/mrm.24268
69. Dittmann F, Reiter R, Guo J, Haas M, Asbach P, Fischer T, et al. Tomoelastography of the Prostate Using Multifrequency MR Elastography and Externally Placed Pressurized-Air Drivers. Magn Reson Med (2018) 79(3):1325–33. doi: 10.1002/mrm.26769
70. Li S, Chen M, Wang W, Zhao W, Wang J, Zhao X, et al. A Feasibility Study of MR Elastography in the Diagnosis of Prostate Cancer at 3.0T. Acta Radiol (2011) 52(3):354–8. doi: 10.1258/ar.2010.100276
71. Sahebjavaher RS, Frew S, Bylinskii A, ter Beek L, Garteiser P, Honarvar M, et al. Prostate MR Elastography With Transperineal Electromagnetic Actuation and a Fast Fractionally Encoded Steady-State Gradient Echo Sequence. NMR Biomed (2014) 27(7):784–94. doi: 10.1002/nbm.3118
72. Reiter R, Majumdar S, Kearney S, Kajdacsy-Balla A, Macias V, Crivellaro S, et al. Prostate Cancer Assessment Using MR Elastography of Fresh Prostatectomy Specimens at 9.4 T. Magn Reson Med (2020) 84(1):396–404. doi: 10.1002/mrm.28127
73. Hu B, Deng Y, Chen J, Kuang S, Tang W, He B, et al. Evaluation of MR Elastography for Prediction of Lymph Node Metastasis in Prostate Cancer. Abdom Radiol (NY) (2021) 46(7):3387–400. doi: 10.1007/s00261-021-02982-4
74. Itoh Y, Takehara Y, Kawase T, Terashima K, Ohkawa Y, Hirose Y, et al. Feasibility of Magnetic Resonance Elastography for the Pancreas at 3T. J Magn Reson Imaging (2016) 43(2):384–90. doi: 10.1002/jmri.24995
75. Shi Y, Gao F, Li Y, Tao S, Yu B, Liu Z, et al. Differentiation of Benign and Malignant Solid Pancreatic Masses Using Magnetic Resonance Elastography With Spin-Echo Echo Planar Imaging and Three-Dimensional Inversion Reconstruction: A Prospective Study. Eur Radiol (2018) 28(3):936–45. doi: 10.1007/s00330-017-5062-y
76. Liu Y, Wang M, Ji R, Cang L, Gao F, Shi Y. Differentiation of Pancreatic Ductal Adenocarcinoma From Inflammatory Mass: Added Value of Magnetic Resonance Elastography. Clin Radiol (2018) 73(10):865–72. doi: 10.1016/j.crad.2018.05.016
77. Jondal DE, Wang J, Chen J, Gorny KR, Felmlee J, Hesly G, et al. Uterine Fibroids: Correlations Between MRI Appearance and Stiffness via Magnetic Resonance Elastography. Abdom Radiol (NY) (2018) 43(6):1456–63. doi: 10.1007/s00261-017-1314-1
78. Ichikawa S, Motosugi U, Omori M, Sano K, Omiya Y, Hirata S, et al. MR-Guided Focused Ultrasound for Uterine Fibroids: A Preliminary Study of Relationship Between the Treatment Outcomes and Factors of MR Images Including Elastography. Magn Reson Med Sci (2019) 18(1):82–7. doi: 10.2463/mrms.tn.2017-0103
79. Gregory A, Bayat M, Kumar V, Denis M, Kim BH, Webb J, et al. Differentiation of Benign and Malignant Thyroid Nodules by Using Comb-Push Ultrasound Shear Elastography: A Preliminary Two-Plane View Study. Acad Radiol (2018) 25(11):1388–97. doi: 10.1016/j.acra.2018.02.025
80. Jugé L, Doan BT, Seguin J, Albuquerque M, Larrat B, Mignet N, et al. Colon Tumor Growth and Antivascular Treatment in Mice: Complementary Assessment With MR Elastography and Diffusion-Weighted MR Imaging. Radiology (2012) 264(2):436–44. doi: 10.1148/radiol.12111548
Keywords: noninvasive, hardness, stiffness, tumor diagnosis, MRE
Citation: Yang J-Y and Qiu B-S (2021) The Advance of Magnetic Resonance Elastography in Tumor Diagnosis. Front. Oncol. 11:722703. doi: 10.3389/fonc.2021.722703
Received: 09 June 2021; Accepted: 04 August 2021;
Published: 31 August 2021.
Edited by:
Haibin Shi, Soochow University, ChinaReviewed by:
Bin Song, Sichuan University, ChinaCopyright © 2021 Yang and Qiu. This is an open-access article distributed under the terms of the Creative Commons Attribution License (CC BY). The use, distribution or reproduction in other forums is permitted, provided the original author(s) and the copyright owner(s) are credited and that the original publication in this journal is cited, in accordance with accepted academic practice. No use, distribution or reproduction is permitted which does not comply with these terms.
*Correspondence: Ben-Sheng Qiu, YnFpdUB1c3RjLmVkdS5jbg==
Disclaimer: All claims expressed in this article are solely those of the authors and do not necessarily represent those of their affiliated organizations, or those of the publisher, the editors and the reviewers. Any product that may be evaluated in this article or claim that may be made by its manufacturer is not guaranteed or endorsed by the publisher.
Research integrity at Frontiers
Learn more about the work of our research integrity team to safeguard the quality of each article we publish.