- 1Department of Urology, Peking University First Hospital, Beijing, China
- 2Institute of Urology, Peking University, Beijing, China
- 3National Urological Cancer Center, Beijing, China
- 4Beijing Key Laboratory of Urogenital Diseases (Male) Molecular Diagnosis and Treatment Center, Beijing, China
- 5Guangdong and Shenzhen Key Laboratory of Male Reproductive Medicine and Genetics, Institute of Urology, Peking University Shenzhen Hospital, Shenzhen-Peking University-The Hong Kong University of Science and Technology Medical Center, Shenzhen, China
N6-Methyladenosine (m6A) is the most widespread internal RNA modification in several species. In spite of latest advances in researching the biological roles of m6A, its function in the development and progression of bladder cancer remains unclear. In this study, we used MeRIPty -55-seq and RNA-seq methods to obtain a comprehensive transcriptome-wide m6A profiling and gene expression pattern in bladder cancer and paired normal adjacent tissues. Our findings showed that there were 2,331 hypomethylated and 3,819 hypermethylated mRNAs, 32 hypomethylated and 105 hypermethylated lncRNAs, and 15 hypomethylated and 238 hypermethylated circRNAs in bladder cancer tissues compared to adjacent normal tissues. Furthermore, m6A is most often harbored in the coding sequence (CDS), with some near the start and stop codons between two groups. Functional enrichment analysis revealed that differentially methylated mRNAs, lncRNAs, and circRNAs were mostly enriched in transcriptional misregulation in cancer and TNF signaling pathway. We also found that different m6A methylation levels of gene might regulate its expression. In summary, our results for the first time provide an m6A landscape of human bladder cancer, which expand the understanding of m6A modifications and uncover the regulation of mRNAs, lncRNAs, and circRNAs through m6A modification in bladder cancer.
Introduction
Bladder transitional cell carcinoma (TCC) is the most common urothelial tumor in urology departments in China. The vast majority originated from epithelial tissue, and TCC accounts for more than 90% (1–3). At present, the diagnosis of bladder transitional cell carcinoma mainly relies on invasive cystoscopy and pathological biopsy. The biggest difficulty in the treatment of bladder cancer is its easy recurrence. Early detection of bladder cancer can improve the chances of bladder preservation and overall survival. After bladder-sparing tumor resection, even with regular infusion of chemotherapy into the bladder, there is still a 10% to 40% recurrence rate, and some of them also show grade, stage progression, or metastasis (4, 5). Recent studies have shown that the occurrence and development of urothelial carcinoma of the bladder are closely related to changes in DNA methylation levels (6). A great deal of research has been done on the pathogenesis of bladder cancer, and numerous pathways and mechanisms involved in the progression of bladder cancer have been discovered, such as proto-oncogene activation, tumor-suppressor gene inactivation (point mutation, rearrangement, deletion), and chromosomal abnormalities (7, 8). However, many molecular mechanisms involved in the development and progression of bladder cancer remain unclear. Therefore, clarifying the molecular mechanism of the occurrence and progression of bladder cancer provides an experimental basis for the discovery of new molecular biological markers of bladder cancer and has important significance and application value for improving the survival rate of patients with bladder cancer.
More than 100 types of RNA modifications have been confirmed in mammalian cells, among which N6-methyladenosine methylation modification is the most common in mRNA and non-coding RNA (9). In recent years, the application of transcriptomic MeRIP-seq technology and the confirmation of m6A demethyltransferase and methyltransferase complex have provided a new sight for the study of the biological function of m6A, as well as the diversity of biological functions regulated by them. It is proved that m6A is a dynamic and reversible RNA modification mode (10–12). In the nucleus of cells, the m6A modification of mRNA is dynamically catalyzed by the methyltransferases METTL3 and METTLl4, as well as the demethyltransferase FTO and ALKBH5 (13). MeRIP-seq revealed that m6A methylation modification was widely distributed in the transcription region, and there was about one m6A modification site in every 2,000 base pairs. There are about 12,000 m6A loci in more than 7,000 human genes, with an average of one to three loci in each transcript, which exist in the conserved sequence RRACH (R=A, G; H=A, C or U), and mostly located near the stop codon, 3′-UTR, and long exon of transcript (14, 15).
Transcriptome refers to the collection of all RNA that is transcribed in a specific tissue or cell at a certain developmental stage or functional state, including protein-coding mRNA and non-coding RNA (16, 17). A large number of studies have shown that m6A methylation modification is involved in the regulation of RNA processing, growth and development of the body, the occurrence of diseases, and other physiological and pathological processes. In addition, it also plays an important role in the occurrence and development of leukemia, malignant glioma, lung cancer, liver cancer, breast cancer, and other malignant tumors (18–21). These studies have shown that abnormal mRNA and non-coding RNA epigenetic modification leads to abnormal oncogene expression, and there may be an internal relationship between m6A methylation and malignant transformation of cells. However, the exact mechanism and its role in tumorigenesis have not been clarified. In this study, we used MeRIP-seq and RNA-seq to research the difference of mRNA, lncRNA, and circRNA expression levels and m6A methylation levels between bladder cancer tissues and normal adjacent tissues. This proved that abnormal m6A methylation modifications in bladder cancer might directly modulate gene expression. Finally, we hope this study will facilitate further investigations of potential roles of m6A modification in bladder cancer pathogenesis.
Results
General Features of m6A Methylation Modification in Bladder Cancer Tissues and Tumor-Adjacent Normal Tissues
Human bladder cancer tissues and tumor-adjacent normal tissues from five patients were used for MeRIP-seq analysis. In tumor tissues, we detected a total of 10,601 m6A peaks within mRNAs (Figure 1A), 576 m6A peaks within lncRNAs (Figure 1B), and 3,116 m6A peaks within circRNAs (Figure 1C). While in adjacent normal tissues, there were a total of 9,198 m6A peaks within mRNAs (Figure 1A), 334 m6A peaks within lncRNAs (Figure 1B), and 1460 m6A peaks within circRNAs (Figure 1C). Among them, 8,460 m6A peaks within mRNAs (Figure 1A), 292 m6A peaks within lncRNAs (Figure 1B), and 1,004 m6A peaks within circRNAs (Figure 1C) were overlapped between adjacent normal and tumor tissues and shown by a Venn diagram. Compared with normal tissues, 4,537 new peaks appeared in tumor tissues, and 1,236 peaks disappeared, indicating that the global m6A modification patterns were significantly different between two groups (Figures 1A–C). We then examined the distribution of m6A methylation modifications in the human transcriptome. We found that most of methylated sequences within mRNA, lncRNA, and circRNA in adjacent normal and tumor tissues contained less than five m6A peaks, while few of them contained five or more sites (Figures 1D–F). The top 10 hypermethylated and hypomethylated m6A-modified peaks for bladder cancer tissues are listed in Tables 1, 2.
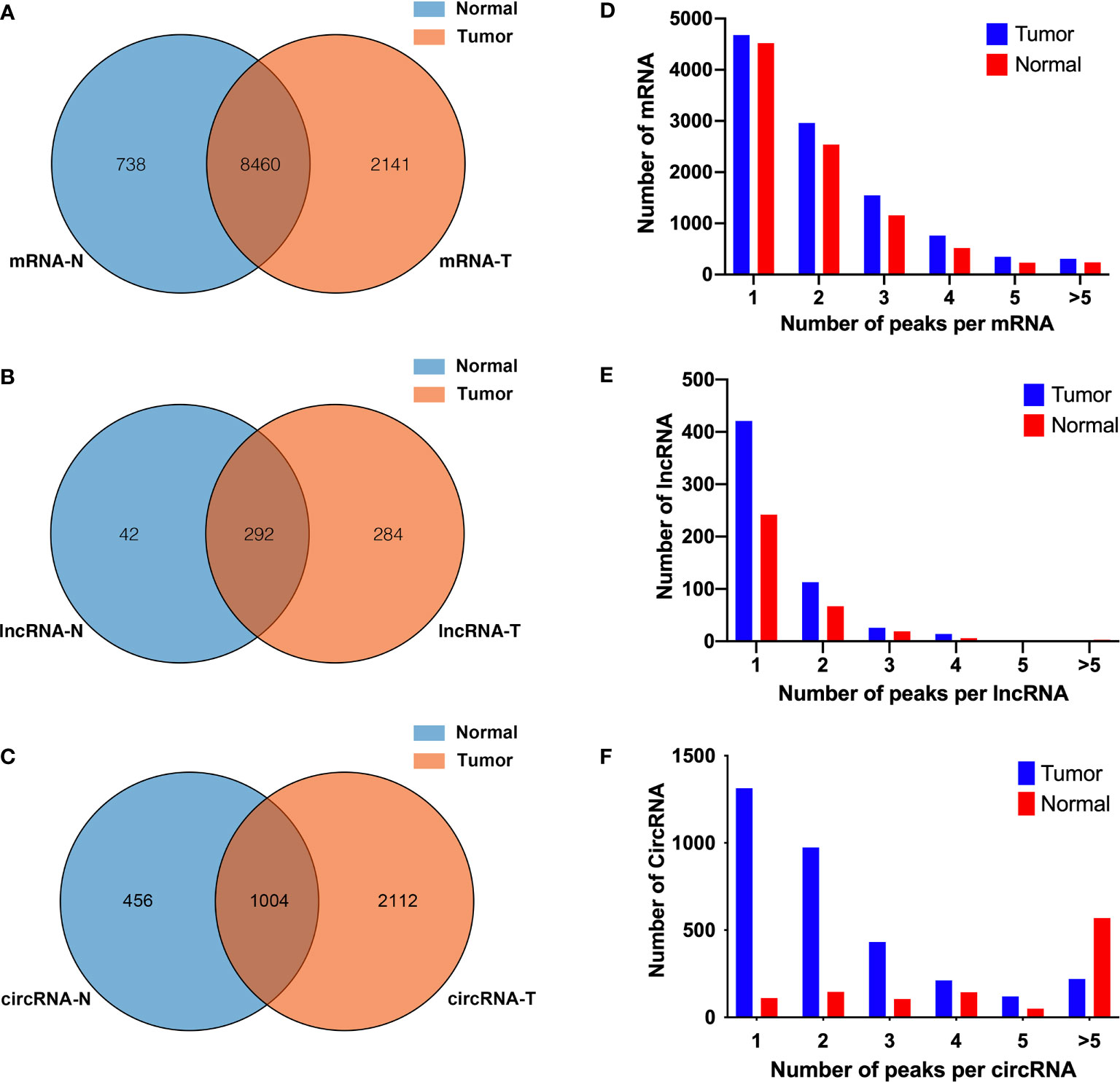
Figure 1 Overview of N6-methyladenosine methylation within mRNAs, lncRNAs, and circRNAs in bladder cancer tissues and adjacent normal tissues. (A–C) Venn diagram showing the overlapped m6A peaks within mRNAs (A), lncRNAs (B), and circRNAs (C) between the two groups. (D–F) Proportion of mRNAs (D), lncRNAs (E), and circRNAs (F) harboring different numbers of m6A peaks in two groups.
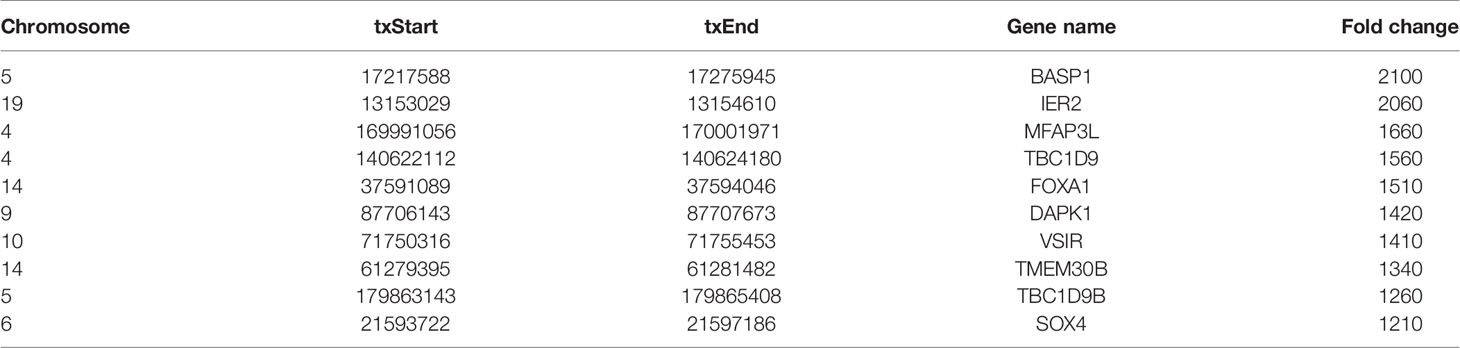
Table 1 The top 10 hypermethylated m6A-modified peaks for bladder cancer tissues compared to normal tissues.
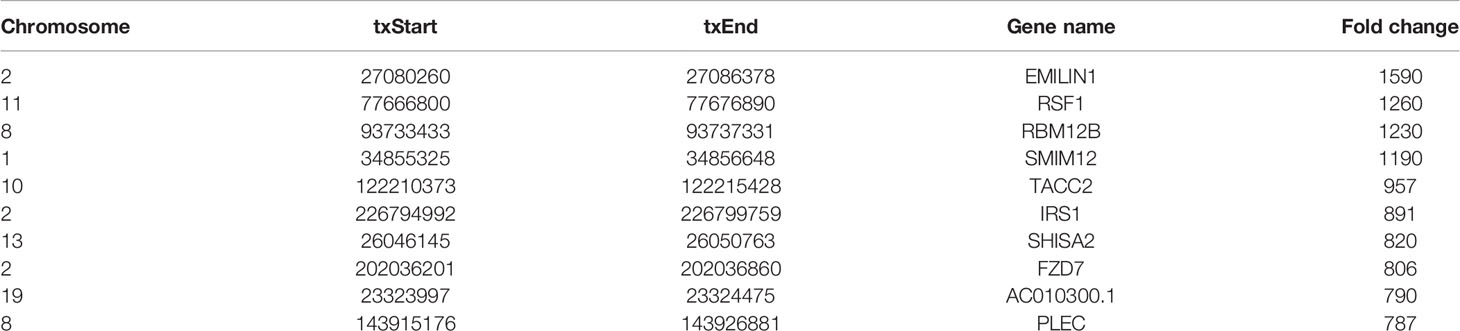
Table 2 The top 10 hypomethylated m6A-modified peaks for bladder cancer tissues compared to normal tissues.
Distribution of m6A Modification in Bladder Cancer Tissues and Tumor-Adjacent Normal Tissues
To study whether the m6A peaks recognized by us had conserved the RRACH motif, we performed the HOMER motif software to analyze the m6A peaks that we identified from the MeRIP-seq data. In the normal and tumor groups, the motif sequence was GGACU and GGACC, respectively (Figure 2A). This showed that there was a difference of m6A motif in tumor and adjacent normal tissues, but their motif sequences were similar to those previously identified. To make clear the priority position of m6A in the whole transcriptome of bladder cancer tissues and adjacent normal tissues, we then studied the metagene profiles of transcript peaks in the two groups. We observed that the m6A peaks were mostly located at the end of the 5′UTRs and start of the 3′UTRs in tumor tissues and adjacent normal tissues (Figure 2B). In addition, we found that the proportion of m6A peaks located at CDS was the highest and the proportion of m6A peaks located at TSS was the least in both tissues (Figures 2C, D).
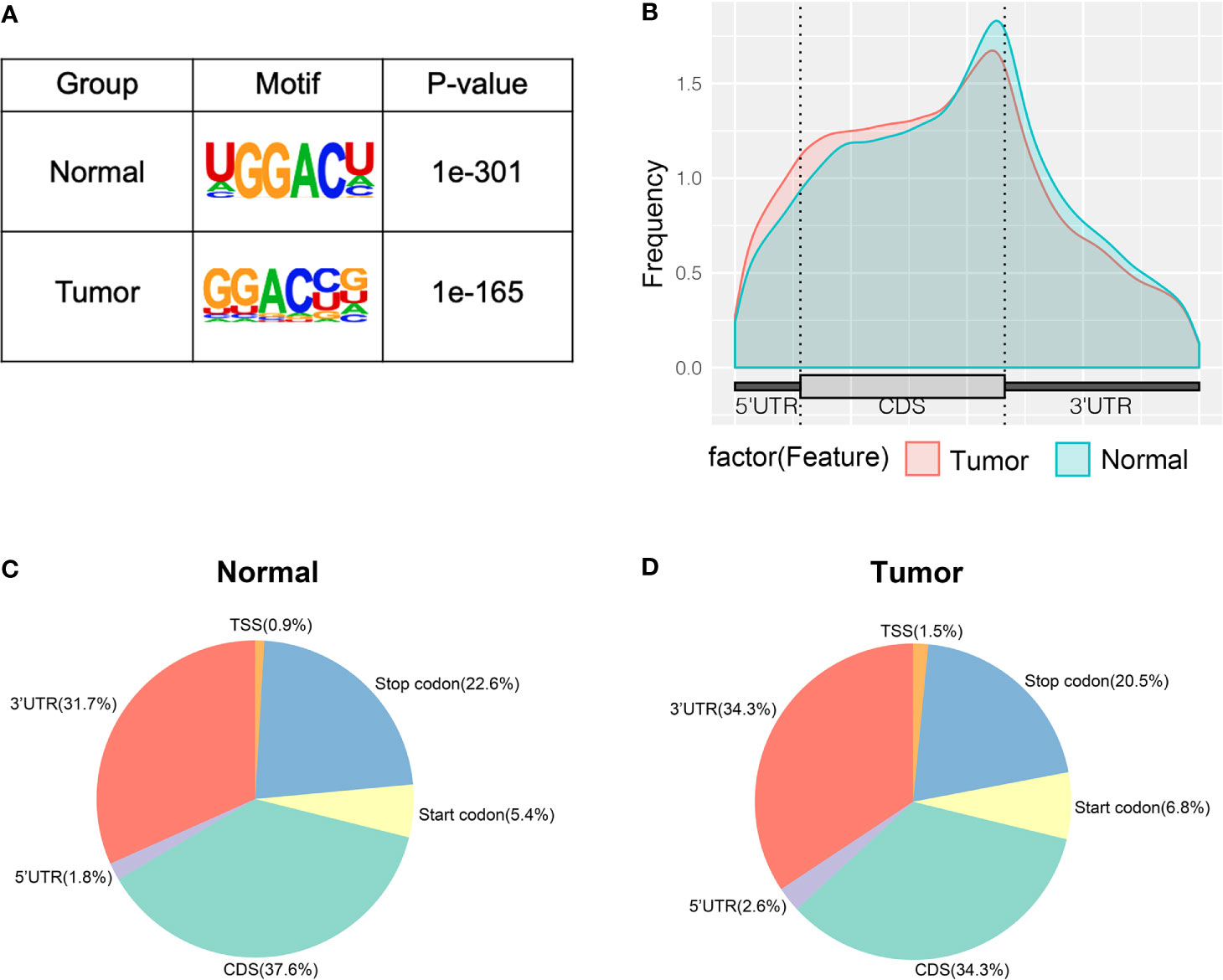
Figure 2 Characteristics of m6A peaks within mRNAs, lncRNAs, and circRNAs in bladder cancer tissues and adjacent normal tissues. (A) Sequence motif of m6A-containing peak regions in tumor and adjacent normal tissues respectively. (B) The metagene profiles of transcripts peaks in tumor and adjacent normal tissues. (C, D) The proportion of m6A peaks in the whole transcriptome of tumor and adjacent normal tissues.
To obtain the distribution profiles of all differentially m6A methylated mRNAs, lncRNAs, and circRNAs across chromosomes, the containment of differentially methylated m6A sites harbored by chromosomes was classified by respective chromosome. This result showed that hypermethylated and hypomethylated m6A sites within mRNAs were primarily located on chromosomes 1, 2, and 19 (Figure 3A). Hypermethylated and hypomethylated m6A sites within lncRNAs were primarily located on chromosomes 11, 12, and X (Figure 3B). Moreover, hypermethylated and hypomethylated m6A sites within circRNAs were primarily located on chromosomes 1, 2, and 3 (Figure 3C). Totally, the top three chromosomes containing the differentially methylated m6A sites were chromosomes 1, 2, and 19. Then, these hypermethylated and hypomethylated m6A sites within mRNAs, lncRNAs, and circRNAs were classified by five regions. For both hypermethylated and hypomethylated mRNAs, lncRNAs, and circRNAs, the fold change of the start codon region was the highest (Figures 3D–F). These results of the distribution of m6A modifications were similar to those of previous studies.
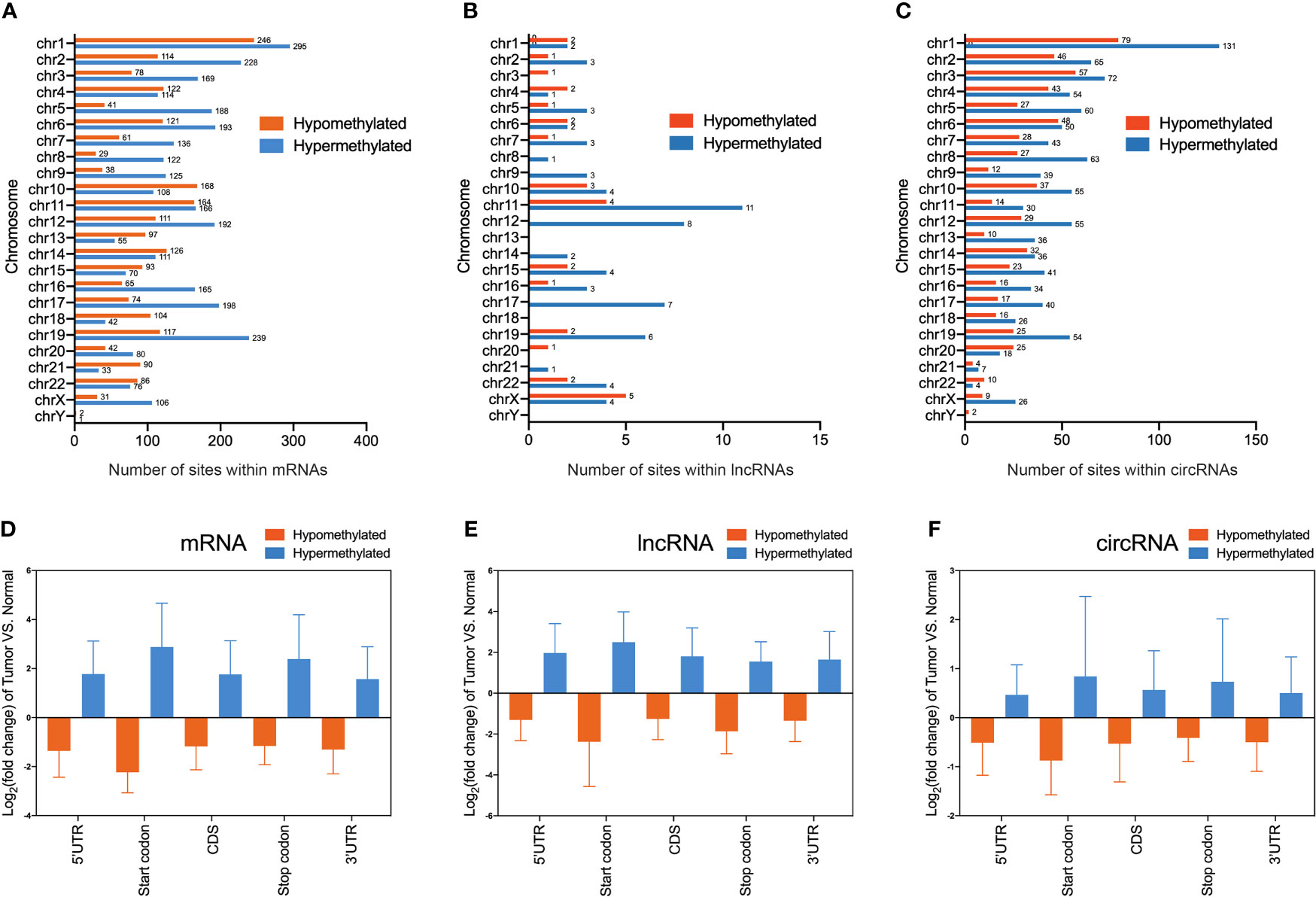
Figure 3 Distribution of differentially methylated N6-methyladenosine sites between bladder cancer tissues and adjacent normal tissues. (A–C) Chromosomal distribution of all differentially methylated N6-methyladenosine sites within mRNAs (A), lncRNAs (B), and circRNAs (C). (D–F) Statistics of fold change of differentially methylated N6-methyladenosine peaks within mRNAs (D), lncRNAs (E), and circRNAs (F) in five segments.
Functional Analysis of Differentially m6A Methylated mRNAs, lncRNAs, and circRNAs Between Two Groups
Differentially m6A methylated mRNAs, lncRNAs, and circRNAs were identified between bladder cancer tissues and adjacent normal tissues based on |log2FC| > 1 and p-value < 0.05. Totally, volcano plots showed 2,331 hypomethylated and 3,819 hypermethylated mRNAs (Figure 4A), 32 hypomethylated and 105 hypermethylated lncRNAs (Figure 4B), and 15 hypomethylated and 238 hypermethylated circRNAs (Figure 4C) in bladder cancer tissues compared to adjacent normal tissues. To uncover the functions of m6A methylation modification in bladder cancer, differentially methylated mRNAs, lncRNAs, and circRNAs between tissues were selected for Gene Ontology enrichment analysis and Kyoto Encyclopedia of Genes and Genomes pathway analysis. The results of GO analysis showed that differentially m6A methylated mRNAs were mostly enriched in regulation of transcription and RNA splicing (Figure 4D), differentially m6A methylated lncRNAs were mostly enriched in protein binding and cell cycle (Figure 4E), and differentially m6A methylated circRNAs were mostly enriched in the transcription process and nucleic acid binding (Figure 4F). Furthermore, KEGG pathway analysis showed that differentially m6A methylated mRNAs were mostly involved in TNF signaling pathway and transcriptional misregulation in cancer (Figure 4G), differentially m6A methylated lncRNAs were mostly enriched in pathways in cancer and endocytosis (Figure 4H), and differentially m6A methylated circRNAs were mostly enriched in spliceosome and mRNA surveillance pathway (Figure 4I). In summary, we found that differentially m6A methylated genes identified from bladder cancer tissues were involved in important biological processes and pathways.
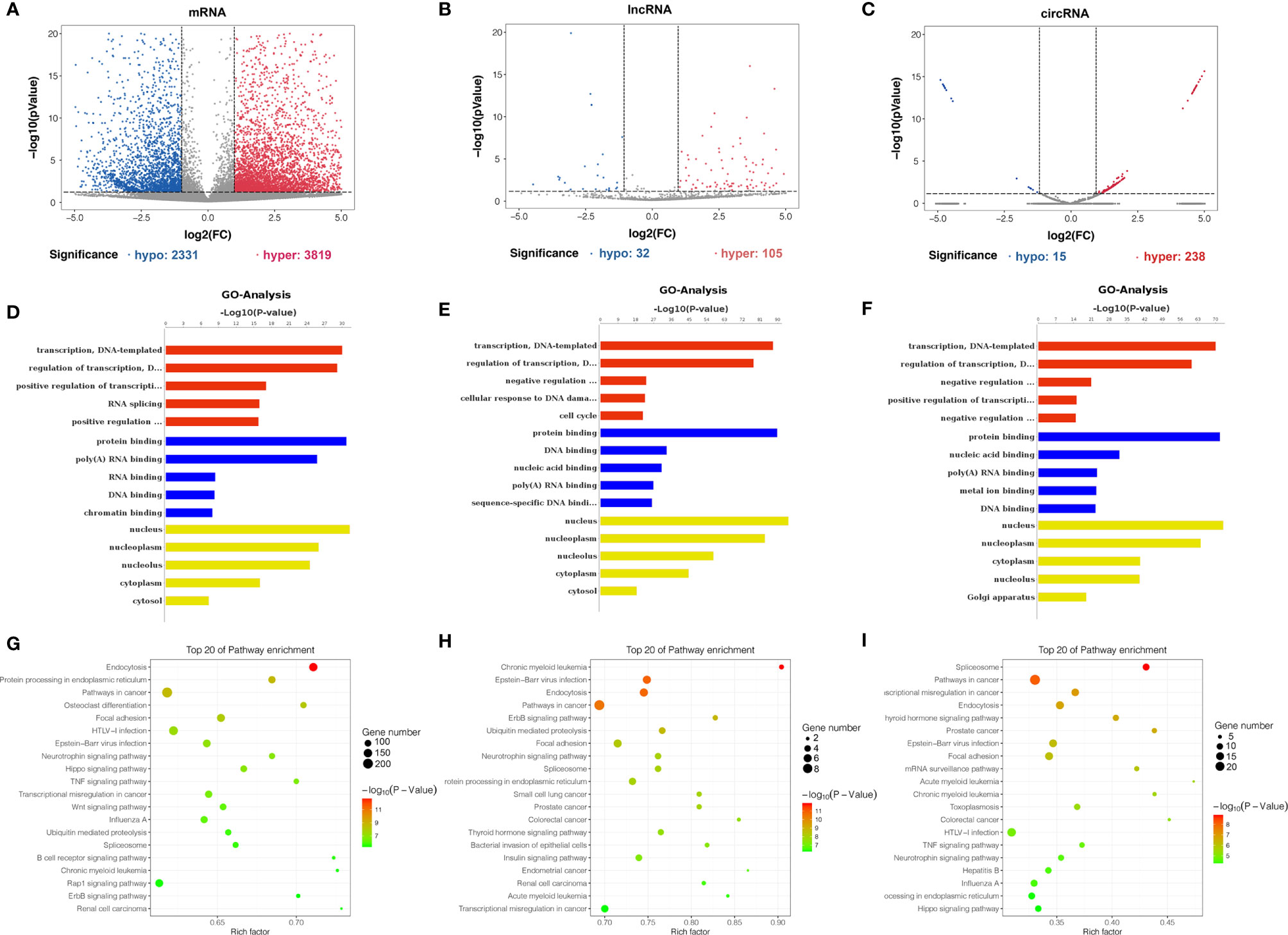
Figure 4 Gene ontology and KEGG pathway analyses of genes harboring differentially methylated N6-methyladenosine sites. (A–C) Volcano plots showing differentially m6A-modified mRNAs (A), lncRNAs (B), and circRNAs (C) based on |log2FC| > 1 and p-value < 0.05. In volcano plots, red blots represent hypermethylation and blue blots represent hypomethylation. (D–F) Gene ontology functional enrichment analysis results of differentially methylated m6A sites-contained mRNAs (D), lncRNAs (E) and circRNAs-associated (F) genes. (G–I) KEGG pathway analysis results of differentially methylated m6A sites-contained mRNAs (G), lncRNAs (H), and circRNAs-associated genes (I).
Conjoint Analysis of MeRIP-seq and RNA-seq Results Between Two Groups
By conjoint analysis of the results from MeRIP-seq and RNA-seq between tissues, we found that there were 34 hypermethylated and upregulated (hyper-up) genes, 15 hypomethylated and downregulated (hypo-down) genes, 76 hypermethylated and downregulated (hyper-down) genes, and 51 hypomethylated and upregulated (hypo-up) genes in bladder cancer tissues compared to adjacent normal tissues (Figure 5A). To further analyze whether m6A methylation affects gene expression, we divided all expressed transcripts into m6A transcripts and non-m6A transcripts, calculated the log two-fold change (log2FC) values of these transcripts, and generated a cumulative curve. The result revealed that the proportion of transcripts modified by m6A was larger than that of transcripts not modified by m6A, especially in terms of the log2FC of the transcript FPKM value between 0 and 20 (Figure 5B). This result promoted us to investigate the general locations of differentially methylated m6A sites within bladder cancer- or other tumor-related genes in bladder cancer tissues compared to adjacent normal tissues. For instance, sphingomyelin phosphodiesterase 4 (SMPD4) was overexpressed in the late stage of clear cell renal cancer and acted as a biomarker for discriminating the early and late stages of ccRCC (22). We found that the m6A peak was enriched around the 5′UTR of SMPD4 in the tumor group of bladder cancer not in adjacent normal tissues (Figure 5C). Moreover, interferon-induced transmembrane protein 2 (IFITM2) promotes gastric cancer growth and metastasis (23), within which m6A was hypomethylated (bladder cancer tissues vs. normal adjacent tissues) and enriched in coding sequence (CDS) (Figure 5D). Within lncRNA PCAT1, a significantly hypermethylated m6A peak enriched in exon 2 was shown in tumor tissues (Figure 5E) and has been reported to suppress castration-resistant prostate cancer progression by activating AKT and NF-κB signaling (24). Circular RNA circ-HIPK3 is downregulated and suppresses cell proliferation, migration, and invasion in osteosarcoma (25) and shows a significantly hypomethylated m6A peak in the tumor group (Figure 5F).
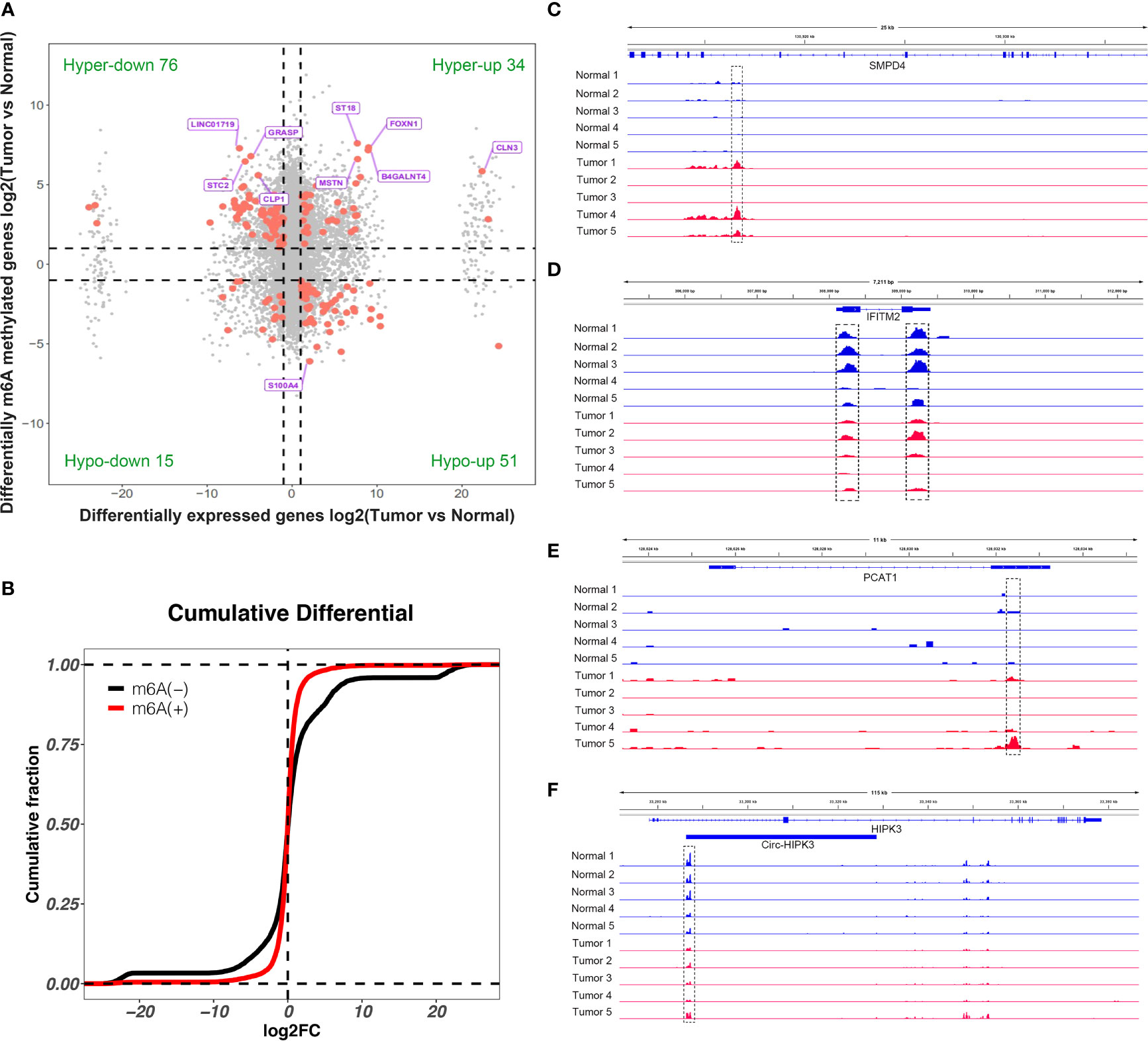
Figure 5 Conjoint analysis of differentially methylated genes and differentially expressed genes. (A) Four-quadrant diagram showed the differentially methylated genes and differentially expressed genes in tumor and adjacent normal tissues. (B) Cumulative distribution of gene expression between tumor and adjacent normal tissues for m6A transcripts (red) and non-m6A transcripts (black). (C–F) Integrative Genome Viewer (IGV) software showed representative differentially methylated mRNAs (SMPD4 and IFITM2), lncRNA (PCAT1), and circRNA (circ-HIPK3) in tumor and adjacent normal tissues.
Expression of Candidate Genes Correlates With Worse Overall Survival in Bladder Cancer Patients
To further confirm the results of our m6A-seq data, we conducted gene-specific m6A-IP qPCR assays for 10 hypermethylated (ST18, FOXN1, SMPD4, MSTN, LINC00482, LINC01719, GRASP, STC2, CLP1, and SGK2) and 10 hypomethylated genes (S100A4, MZB1, SFTPB, GALNT5, CACYBP, WNT5A, PRR16, NR4A2, GLIPR1, and KIAA1551) which might participate in tumor progression in bladder cancer. We observed the almost same m6A-level changes in these genes, confirming the validity of our MeRIP-seq results (Figure 6A). Sequentially, transcript levels of the abovementioned genes (ST18, FOXN1, SMPD4, MSTN, LINC00482, S100A4, MZB1, SFTPB, GALNT5, and CACYBP were upregulated genes, LINC01719, GRASP, STC2, CLP1, SGK2, WNT5A, PRR16, NR4A2, GLIPR1, and KIAA1551 were downregulated genes) were also measured in five pairs of bladder cancer and adjacent normal tissues by RT-qPCR (Figure 6B). Results showed a similar tendency of transcript levels with RNA-seq data in two groups, which validated our RNA-seq results. To confirm the clinical significance of the candidate genes discovered in this study, Kaplan–Meier analysis extracted from the TCGA database was explored. We found that a low expression of METTL14 (a m6A methyltransferase), SMPD4, and SGK2, but a high expression of ALKBH5 (a m6A de methyltransferase), LINC00482, and HIPK3, showed a tendency to associate with worse overall survival in bladder cancer patients (Figures 6C–H).
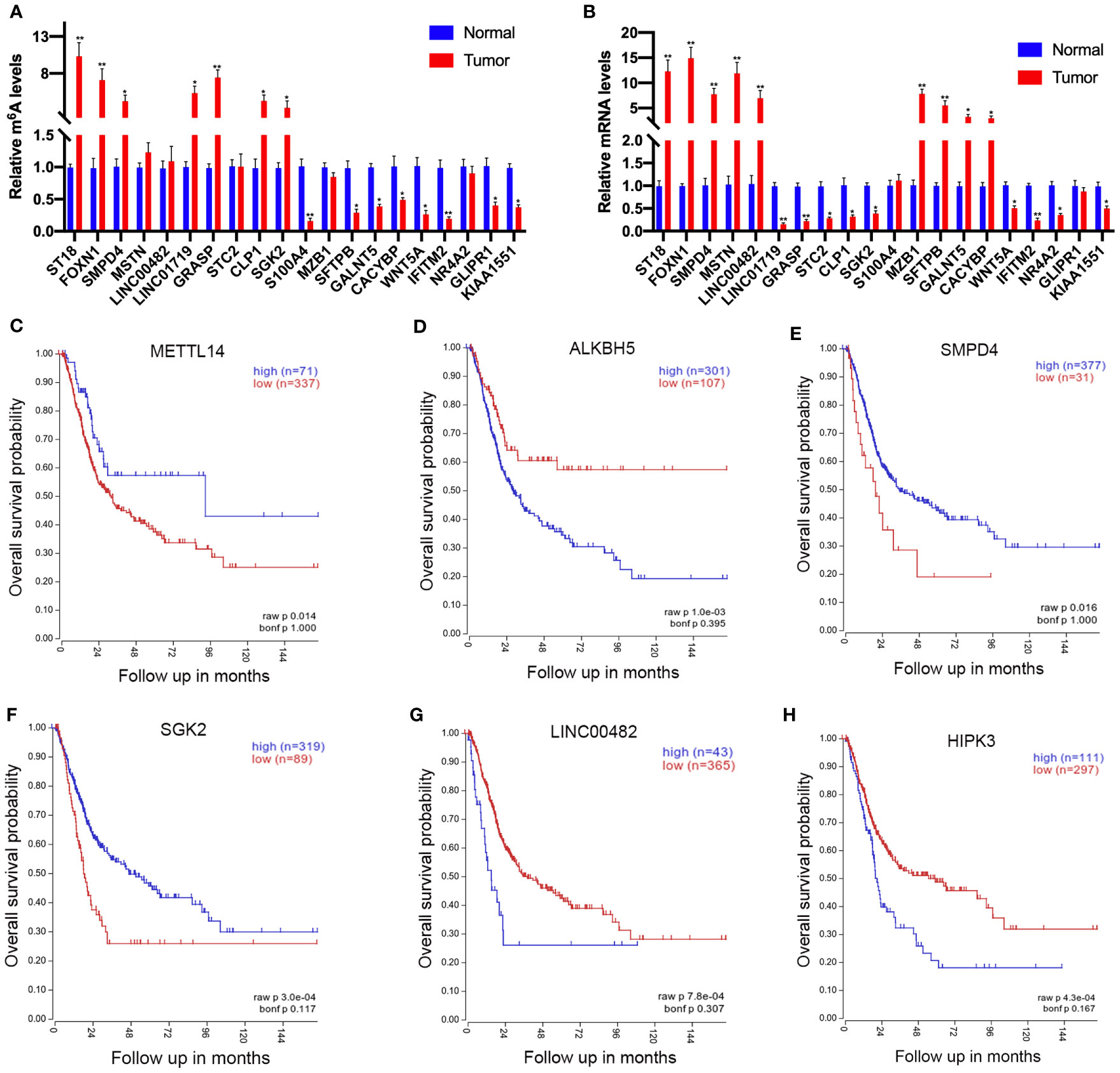
Figure 6 Prognostic value of the survival-associated gene signature in bladder cancer patients. (A) Validations of the m6A enrichments of 10 hypermethylated genes and 10 hypomethylated genes by m6A-immunoprecipitation (IP)-qPCR. (B) Validations of the mRNA expression levels of 10 upregulated genes and 10 downregulated genes by RT-qPCR. (C–H) The low expressions of METTL14 (C), SMPD4 (E), and SGK2 (F) in mRNA level correlate with worse overall survival in bladder cancer patients. The high expression of ALKBH5 (D), LINC00482 (G), and HIPK (H) expression levels showed a tendency to correlate with worse overall survival in bladder cancer patients. *p < 0.05, **p < 0.01.
Discussion
N6-methyladenosine (m6A) is the most common mRNA modification in eukaryotic cells of all higher animals (26). It is involved in various physiological and pathological processes by regulating mRNA transcription, processing, and other metabolic processes (27–32). At present, MeRIP-seq was used to study the distribution sites and expression levels of m6A on transcripts in mammalian cells, and it was found that m6A was distributed in the entire transcriptome including mRNA and non-coding RNA, mainly concentrated in the 3′-UTR and near the transcriptional stop codon (14). Studies have shown that m6A is dynamically regulated by methyltransferase and demethyltransferase, but the biological function of m6A in cancer is not yet fully understood (33). In this study, bladder cancer tissues and normal adjacent tissues were created to assess the m6A state, which revealed big differences between the tumor and adjacent normal groups, supporting the dynamic characteristic of m6A modification.
In the current study, we figured out that m6A modification in tumor and adjacent normal tissues mainly occurs in the motif, GGACC and GGACU, respectively, which is similar to the previous data. Moreover, transcript methylated peaks are mainly located at CDS. Almost 85% of methylated genes have one to five m6A methylated sites, and others contain over five m6A methylated sites in mRNAs, lncRNAs, and circRNAs of tumor and adjacent normal tissues. In addition, differentially methylated genes between tumor and adjacent normal tissues were detected and shown to be involved in many important biological pathways such as pathways in cancer, transcriptional misregulation in cancer, TNF signaling pathway, and hippo signaling pathway. Studies have reported that TNF-alpha induced MMP-9 expression in bladder cancer cells by activating the transcription factor NF-kappaB, which is involved in the p38 MAP kinase-mediated control of MMP-9 regulation (34). Another study reported that the Hippo signaling pathway is a conserved pathway that plays a crucial role in cellular proliferation, differentiation, and apoptosis in bladder cancer (35). A combined analysis of our MeRIP-seq and mRNA-seq data revealed 34 hyper-up genes, 15 hypo-down genes, 76 hyper-down genes, and 51 hypo-up genes in the tumor group compared with the adjacent normal group; these genes may play critical roles in the development of bladder cancer. Moreover, some of these genes were reported to facilitate tumor growth and metastasis in different kinds of cancers. For example, SMPD4 was overexpressed in the late stage of clear cell renal cancer and acted as a biomarker for discriminating early and late stages of ccRCC (22), but its function in bladder cancer is unclear. In this study, we found that SMPD4 was hypermethylated and upregulated in bladder cancer tissues in comparison to normal adjacent tissues, and a low expression of SMPD4 showed a tendency to associate with worse overall survival in bladder cancer patients. Our data indicate that m6A methylation could participate in tumor progression through the modification of tumor-related genes. However, further experiments should be required to confirm these results.
m6A modification is involved in almost every step in mRNA metabolism. Furthermore, it also affects the processing of lncRNAs and circRNAs. Our findings provide the first m6A modification landscape in bladder cancer. Differentially expressed mRNAs with hyper-methylated or hypo-methylated m6A modifications are identified, which may help observe the mechanisms of m6A-mediated gene expression regulation. In further studies, we will evaluate the biological relevance and clinical value of m6A in bladder cancer.
Materials and Methods
Patients and Samples
Five pairs of bladder cancer tissues and adjacent non-malignant tissues with patients’ informed consent were obtained from the Urology Department of Peking University First Hospital (PKUFH), Beijing, China. This study followed the Helsinki declaration and was approved by the Institutional Ethical Review Board of PKUFH. Samples were collected immediately in the operating room after surgical removal and were stored in liquid nitrogen after rapid freezing in liquid nitrogen for the following RNA isolation.
MeRIP-seq and RNA-seq of the Whole Transcriptome
MeRIP-seq and RNA-seq were performed at CloudSeq Biotech, Inc. (Shanghai, China) (36–38) and as described previously. Briefly, total RNAs were isolated from five pairs of bladder cancer tissues and normal adjacent tissues using TRIzol (Invitrogen). Then, total RNA was fragmented into almost 100 nt and were incubated with anti-m6A antibody (Manga) for 2 h at 4°C. Then, the beads were prepared and incubated with the total RNA for 2 h at 4°C. Finally, the mixture was washed and the m6A-bound RNA was purified with TE buffer. After being purified, the samples were used to construct the library by Prep Kit (Illumina) on HiSeq 3000.
MeRIP-seq and Data Analysis
Total RNA was extracted from the two groups of cells by using TRIzol Reagent (Life Technologies). The quality and quantity of total RNA were assessed by using NanoDrop ND 2000 (Thermo Fisher Scientific, MA, USA). The RNA integrity was measured using denaturing agarose. Seq-Star T M poly(A) mRNA Isolation Kit (Arraystar, MD, USA) was used to isolate mRNA from total RNA. The GenSeq™ m6A RNA IP Kit (GenSeq Inc., China) was used to perform m6A RNA immunoprecipitation by following the manufacturer’s instructions. The input samples without immunoprecipitation and the m6A IP samples were both used for library construction with NEBNext Ultra II Directional RNA Library Prep Kit (New England Biolabs, Inc., MA, USA). The library quality was evaluated with the Bioanalyzer 2100 system (Agilent Technologies Inc., CA, USA). Library sequencing was performed on an Illumina HiSeq instrument with 150-bp paired-end reads.
Paired-end reads were harvested using the Illumina HiSeq 4000 sequencer and were quality controlled by Q30. After 3′ adaptor-trimming, low-quality reads were removed by Cutadapt software (v1.9.3). First, clean reads of all libraries were aligned to the reference genome (HG19) by Hisat2 software (v2.0.4). Methylated sites on RNAs (peaks) were identified by MACS software (39). Identified m6A peaks were subjected to motif enrichment analysis by HOMER (40), and metagene m6A distribution was characterized by R package MetaPlotR (41). Differentially methylated sites (fold change ≥2 and p < 0.05) were identified by diffReps (42). These peaks identified by software overlapping with exons of mRNA were figured out and chosen by homemade scripts. Genes of interest were visualized in the IGV (Integrative Genomics Viewer) software (v2.3.68) (43). The gene ontology (GO) analysis and pathway enrichment analysis were performed on the differentially methylated protein-coding genes by using the GO (www.geneontology.org) and Kyoto Encyclopedia of Genes and Genomes (KEGG) databases (www.genome.jp/kegg). Clinical survival data (including expression level and survival time) were downloaded from the TCGA database (https://cancergenome.nih.gov/).
RNA-seq and Data Analysis
Total RNA was extracted from biological samples using TRIzol Reagent (Life Technologies) according to the manufacturer’s protocol. Denatured agarose gel electrophoresis was applied to evaluate the integrity of total RNA. Seq-Star TM poly(A) mRNA Isolation Kit (Arraystar, MD, USA) was used to purify mRNA from total RNA after confirming its quantity and quality by NanoDrop ND-2000. Then, fragmented mRNA was subjected to 50-bp single-end sequencing with a BGISEQ-500 platform. Adapter and low-quality reads were trimmed by SOAPnuke (44), and trimmed reads were aligned to reference genome by bowtie2 (45). RSEM (46) was used to calculate expression levels, and DEGs were identified by DEGseq (47).
M6A-IP-qPCR and RT-qPCR
Twenty genes with differentially methylated sites according to MeRIP-seq were tested by reverse transcription (RT)-qPCR. A small number of fragmented RNA was used as the input control. The rested RNA was incubated with anti-m6A antibody-coupled beads. The m6A-containing RNAs were then immunoprecipitated and eluted from the beads. Both input control and m6A-IP samples were subjected to RT-qPCR with gene-specific primers.
Statistical Analysis
Experiments were performed at least three times, and representative results are shown. All statistical analyses were performed and visualized using RStudio (Version1.2.1335, Boston, MA, USA), GSEA (Version4.0, UC San Diego and Broad Institute, USA) 23, MedCalc (Version16.8, Ostend, Belgium), and GraphPad Prism (Version 8.0, GraphPad, Inc., La Jolla, CA, USA). Differences between individual groups were analyzed using the chi-squared test and Student’s t-test (two-tailed and unpaired) with triplicate or quadruplicate sets. A two-tailed p < 0.05 was considered statistically significant.
Data Availability Statement
All RNA sequencing data were deposited in the NCBI SRA (Sequence Read Achieve) database with the accession number of PRJNA733602.
Ethics Statement
The studies involving human participants were reviewed and approved by the Institutional Ethical Review Board of PKUFH. The patients/participants provided their written informed consent to participate in this study. Written informed consent was obtained from the individual(s) for the publication of any potentially identifiable images or data included in this article.
Author Contributions
AL, YG, and CC performed the experiments and data analysis. CC and QuZ prepared the diagrams and wrote the manuscript. AL and YG designed the project. QiZ and LY supervised the project and provided financial support. All authors contributed to the article and approved the submitted version.
Funding
This work was supported by grants from the National Natural Science Foundation of China to QZ (Nos. 82072826 and 81872088) and a grant from Peking University Medicine Fund of Fostering Young Scholars’ Scientific & Technological Innovation to YG (No. BMU2020PYB028).
Conflict of Interest
The authors declare that the research was conducted in the absence of any commercial or financial relationships that could be construed as a potential conflict of interest.
Publisher’s Note
All claims expressed in this article are solely those of the authors and do not necessarily represent those of their affiliated organizations, or those of the publisher, the editors and the reviewers. Any product that may be evaluated in this article, or claim that may be made by its manufacturer, is not guaranteed or endorsed by the publisher.
Supplementary Material
The Supplementary Material for this article can be found online at: https://www.frontiersin.org/articles/10.3389/fonc.2021.717622/full#supplementary-material
References
1. Siegel RL, Miller KD, Jemal A. Cancer Statistics, 2018. CA Cancer J Clin (2018) 68(1):7–30. doi: 10.3322/caac.21442
2. Antoni S, Ferlay J, Soerjomataram I, Znaor A, Jemal A, Bray F. Bladder Cancer Incidence and Mortality: A Global Overview and Recent Trends. Eur Urol (2017) 71(1):96–108. doi: 10.1016/j.eururo.2016.06.010
3. Katsila T, Liontos M, Patrinos GP, Bamias A, Kardamakis D. The New Age of -Omics in Urothelial Cancer - Re-Wording Its Diagnosis and Treatment. EBioMedicine (2018) 28:43–50. doi: 10.1016/j.ebiom.2018.01.044
4. Felsenstein KM, Theodorescu D. Precision Medicine for Urothelial Bladder Cancer: Update on Tumour Genomics and Immunotherapy. Nat Rev Urol (2018) 15(2):92–111. doi: 10.1038/nrurol.2017.179
5. Kaufman DS, Shipley WU, Feldman AS. Bladder Cancer. Lancet (2009) 374(9685):239–49. doi: 10.1016/S0140-6736(09)60491-8
6. Shimizu T, Suzuki H, Nojima M, Kitamura H, Yamamoto E, Maruyama R, et al. Methylation of a Panel of microRNA Genes is a Novel Biomarker for Detection of Bladder Cancer. Eur Urol (2013) 63(6):1091–100. doi: 10.1016/j.eururo.2012.11.030
7. Dueñas M, Martínez-Fernández M, García-Escudero R, Villacampa F, Marqués M, Saiz-Ladera C, et al. PIK3CA Gene Alterations in Bladder Cancer are Frequent and Associate With Reduced Recurrence in non-Muscle Invasive Tumors. Mol Carcinog (2015) 54(7):566–76. doi: 10.1002/mc.22125
8. Yap KL, Kiyotani K, Tamura K, Antic T, Jang M, Montoya M, et al. Whole-Exome Sequencing of Muscle-Invasive Bladder Cancer Identifies Recurrent Mutations of UNC5C and Prognostic Importance of DNA Repair Gene Mutations on Survival. Clin Cancer Res (2014) 20(24):6605–17. doi: 10.1158/1078-0432.CCR-14-0257
9. Pan T. N6-Methyl-Adenosine Modification in Messenger and Long non-Coding RNA. Trends Biochem Sci (2013) 38(4):204–9. doi: 10.1016/j.tibs.2012.12.006
10. Sibbritt T, Patel HR, Preiss T. Mapping and Significance of the mRNA Methylome. Wiley Interdiscip Rev RNA (2013) 4(4):397–422. doi: 10.1002/wrna.1166
11. Jia G, Fu Y, Zhao X, Dai Q, Zheng G, Yang Y, et al. N6-Methyladenosine in Nuclear RNA is a Major Substrate of the Obesity-Associated FTO. Nat Chem Biol (2011) 7(12):885–7. doi: 10.1038/nchembio.687
12. Zheng G, Dahl JA, Niu Y, Fedorcsak P, Huang CM, Li CJ, et al. ALKBH5 is a Mammalian RNA Demethylase That Impacts RNA Metabolism and Mouse Fertility. Mol Cell (2013) 49(1):18–29. doi: 10.1016/j.molcel.2012.10.015
13. Lin S, Gregory RI. Methyltransferases Modulate RNA Stability in Embryonic Stem Cells. Nat Cell Biol (2014) 16(2):129–31. doi: 10.1038/ncb2914
14. Meyer KD, Saletore Y, Zumbo P, Elemento O, Mason CE, Jaffrey SR. Comprehensive Analysis of mRNA Methylation Reveals Enrichment in 3' UTRs and Near Stop Codons. Cell (2012) 149(7):1635–46. doi: 10.1016/j.cell.2012.05.003
15. Dominissini D, Moshitch-Moshkovitz S, Schwartz S, Salmon-Divon M, Ungar L, Osenberg S, et al. Topology of the Human and Mouse M6a RNA Methylomes Revealed by M6a-Seq. Nature (2012) 485(7397):201–6. doi: 10.1038/nature11112
16. Vdovikova S, Gilfillan S, Wang S, Dongre M, Wai SN, Hurtado A. Modulation of Gene Transcription and Epigenetics of Colon Carcinoma Cells by Bacterial Membrane Vesicles. Sci Rep (2018) 8(1):7434. doi: 10.1038/s41598-018-25308-9
17. Yue Y, Liu J, He C. RNA N6-Methyladenosine Methylation in Post-Transcriptional Gene Expression Regulation. Genes Dev (2015) 29(13):1343–55. doi: 10.1101/gad.262766.115
18. Deng X, Su R, Feng X, Wei M, Chen J. Role of N(6)-Methyladenosine Modification in Cancer. Curr Opin Genet Dev (2018) 48:1–7. doi: 10.1016/j.gde.2017.10.005
19. Huisman B, Manske G, Carney S, Kalantry S. Functional Dissection of the M6a RNA Modification. Trends Biochem Sci (2017) 42(2):85–6. doi: 10.1016/j.tibs.2016.12.004
20. Cao G, Li HB, Yin Z, Flavell RA. Recent Advances in Dynamic M6a RNA Modification. Open Biol (2016) 6(4):160003. doi: 10.1098/rsob.160003
21. Nishizawa Y, Konno M, Asai A, Koseki J, Kawamoto K, Miyoshi N, et al. Oncogene C-Myc Promotes Epitranscriptome M(6)A Reader YTHDF1 Expression in Colorectal Cancer. Oncotarget (2018) 9(7):7476–86. doi: 10.18632/oncotarget.23554
22. Bhalla S, Chaudhary K, Kumar R, Sehgal M, Kaur H, Sharma S, et al. Gene Expression-Based Biomarkers for Discriminating Early and Late Stage of Clear Cell Renal Cancer. Sci Rep (2017) 7:44997. doi: 10.1038/srep44997
23. Xu L, Zhou R, Yuan L, Wang S, Li X, Ma H, et al. IGF1/IGF1R/STAT3 Signaling-Inducible IFITM2 Promotes Gastric Cancer Growth and Metastasis. Cancer Lett (2017) 393:76–85. doi: 10.1016/j.canlet.2017.02.014
24. Shang Z, Yu J, Sun L, Tian J, Zhu S, Zhang B, et al. LncRNA PCAT1 Activates AKT and NF-κb Signaling in Castration-Resistant Prostate Cancer by Regulating the PHLPP/Fkbp51/Ikkα Complex. Nucleic Acids Res (2019) 47(8):4211–25. doi: 10.1093/nar/gkz108
25. Xiao-Long M, Kun-Peng Z, Chun-Lin Z. Circular RNA Circ_HIPK3 is Down-Regulated and Suppresses Cell Proliferation, Migration and Invasion in Osteosarcoma. J Cancer (2018) 9(10):1856–62. doi: 10.7150/jca.24619
26. Zhu W, Wang JZ, Xu Z, Cao M, Hu Q, Pan C, et al. Detection of N6−methyladenosine Modification Residues (Review). Int J Mol Med (2019) 43(6):2267–78. doi: 10.3892/ijmm.2019.4169
27. Lin S, Choe J, Du P, Triboulet R, Gregory RI. The M(6)A Methyltransferase METTL3 Promotes Translation in Human Cancer Cells. Mol Cell (2016) 62(3):335–45. doi: 10.1016/j.molcel.2016.03.021
28. Batista PJ, Molinie B, Wang J, Qu K, Zhang J, Li L, et al. M(6)A RNA Modification Controls Cell Fate Transition in Mammalian Embryonic Stem Cells. Cell Stem Cell (2014) 15(6):707–19. doi: 10.1016/j.stem.2014.09.019
29. Fustin JM, Doi M, Yamaguchi Y, Hida H, Nishimura S, Yoshida M, et al. RNA-Methylation-Dependent RNA Processing Controls the Speed of the Circadian Clock. Cell (2013) 155(4):793–806. doi: 10.1016/j.cell.2013.10.026
30. Tao L, Mu X, Chen H, Jin D, Zhang R, Zhao Y, et al. FTO Modifies the M6a Level of MALAT and Promotes Bladder Cancer Progression. Clin Transl Med (2021) 11(2):e310. doi: 10.1002/ctm2.310
31. Cheng M, Sheng L, Gao Q, Xiong Q, Zhang H, Wu M, et al. The M(6)A Methyltransferase METTL3 Promotes Bladder Cancer Progression via AFF4/NF-κb/MYC Signaling Network. Oncogene (2019) 38(19):3667–80. doi: 10.1038/s41388-019-0683-z
32. Jin H, Ying X, Que B, Wang X, Chao Y, Zhang H, et al. N(6)-Methyladenosine Modification of ITGA6 mRNA Promotes the Development and Progression of Bladder Cancer. EBioMedicine (2019) 47:195–207. doi: 10.1016/j.ebiom.2019.07.068
33. Zhang C, Samanta D, Lu H, Bullen JW, Zhang H, Chen I, et al. Hypoxia Induces the Breast Cancer Stem Cell Phenotype by HIF-Dependent and ALKBH5-Mediated M⁶A-Demethylation of NANOG mRNA. Proc Natl Acad Sci USA (2016) 113(14):E2047–56. doi: 10.1073/pnas.1602883113
34. Lee SJ, Park SS, Lee US, Kim WJ, Moon SK. Signaling Pathway for TNF-Alpha-Induced MMP-9 Expression: Mediation Through P38 MAP Kinase, and Inhibition by Anti-Cancer Molecule Magnolol in Human Urinary Bladder Cancer 5637 Cells. Int Immunopharmacol (2008) 8(13-14):1821–6. doi: 10.1016/j.intimp.2008.08.018
35. Xia J, Zeng M, Zhu H, Chen X, Weng Z, Li S. Emerging Role of Hippo Signalling Pathway in Bladder Cancer. J Cell Mol Med (2018) 22(1):4–15. doi: 10.1111/jcmm.13293
36. Li P, Yu H, Zhang G, Kang L, Qin B, Cao Y, et al. Identification and Characterization of N6-Methyladenosine CircRNAs and Methyltransferases in the Lens Epithelium Cells From Age-Related Cataract. Invest Ophthalmol Vis Sci (2020) 61(10):13. doi: 10.1167/iovs.61.10.13
37. Niu X, Xu J, Liu J, Chen L, Qiao X, Zhong M. Landscape of N(6)-Methyladenosine Modification Patterns in Human Ameloblastoma. Front Oncol (2020) 10:556497. doi: 10.3389/fonc.2020.556497
38. Wang YN, Jin HZ. Transcriptome-Wide M(6)A Methylation in Skin Lesions From Patients With Psoriasis Vulgaris. Front Cell Dev Biol (2020) 8:591629. doi: 10.3389/fcell.2020.591629
39. Zhang Y, Liu T, Meyer CA, Eeckhoute J, Johnson DS, Bernstein BE, et al. Model-Based Analysis of ChIP-Seq (MACS). Genome Biol (2008) 9(9):R137. doi: 10.1186/gb-2008-9-9-r137
40. Heinz S, Benner C, Spann N, Bertolino E, Lin YC, Laslo P, et al. Simple Combinations of Lineage-Determining Transcription Factors Prime Cis-Regulatory Elements Required for Macrophage and B Cell Identities. Mol Cell (2010) 38(4):576–89. doi: 10.1016/j.molcel.2010.05.004
41. Olarerin-George AO, Jaffrey SR. MetaPlotR: A Perl/R Pipeline for Plotting Metagenes of Nucleotide Modifications and Other Transcriptomic Sites. Bioinformatics (2017) 33(10):1563–4. doi: 10.1093/bioinformatics/btx002
42. Shen L, Shao NY, Liu X, Maze I, Feng J, Nestler EJ. Diffreps: Detecting Differential Chromatin Modification Sites From ChIP-Seq Data With Biological Replicates. PloS One (2013) 8(6):e65598. doi: 10.1371/journal.pone.0065598
43. Thorvaldsdóttir H, Robinson JT, Mesirov JP. Integrative Genomics Viewer (IGV): High-Performance Genomics Data Visualization and Exploration. Brief Bioinform (2013) 14(2):178–92. doi: 10.1093/bib/bbs017
44. Chen Y, Chen Y, Shi C, Huang Z, Zhang Y, Li S, et al. SOAPnuke: A MapReduce Acceleration-Supported Software for Integrated Quality Control and Preprocessing of High-Throughput Sequencing Data. Gigascience (2018) 7(1):1–6. doi: 10.1093/gigascience/gix120
45. Langmead B, Salzberg SL. Fast Gapped-Read Alignment With Bowtie 2. Nat Methods (2012) 9(4):357–9. doi: 10.1038/nmeth.1923
46. Li B, Dewey CN. RSEM: Accurate Transcript Quantification From RNA-Seq Data With or Without a Reference Genome. BMC Bioinformatics (2011) 12:323. doi: 10.1186/1471-2105-12-323
Keywords: m6A (N6-methyladenosine), MeRIP-seq, bladder cancer, mRNA, lncRNA, circRNA
Citation: Li A, Gan Y, Cao C, Ma B, Zhang Q, Zhang Q and Yao L (2021) Transcriptome-Wide Map of N6-Methyladenosine Methylome Profiling in Human Bladder Cancer. Front. Oncol. 11:717622. doi: 10.3389/fonc.2021.717622
Received: 31 May 2021; Accepted: 18 October 2021;
Published: 15 November 2021.
Edited by:
Daniela Terracciano, University of Naples Federico II, ItalyReviewed by:
Valerio Costa, Institute of Genetics and Biophysics (CNR), ItalyCarlos Eduardo Fonseca-Alves, Paulista University, Brazil
Copyright © 2021 Li, Gan, Cao, Ma, Zhang, Zhang and Yao. This is an open-access article distributed under the terms of the Creative Commons Attribution License (CC BY). The use, distribution or reproduction in other forums is permitted, provided the original author(s) and the copyright owner(s) are credited and that the original publication in this journal is cited, in accordance with accepted academic practice. No use, distribution or reproduction is permitted which does not comply with these terms.
*Correspondence: Qian Zhang, emhhbmdxaWFuYmptdUAxMjYuY29t; Lin Yao, cG9wYXJpZXNAMTYzLmNvbQ==
†These authors have contributed equally to this work