- 1Experimental Therapeutics Unit, Medical Oncology Department, Hospital Clínico Universitario San Carlos (HCSC), Instituto de Investigación Sanitaria (IdISSC), Madrid, Spain
- 2Translational Oncology Laboratory, Centro Regional de Investigaciones Biomedicas, Castilla‐La Mancha University (CRIB‐UCLM), Albacete, Spain
- 3Instituto de Biología Molecular y Celular del Cáncer and Centro de Investigación Biomédica en Red de Cáncer (CIBERONC), Centro Superior de Investigaciones Científicas (CSIC), Salamanca, Spain
- 4Department of Bioinformatics, Faculty of Medicine, Semmelweis University, Budapest, Hungary
- 52nd Department of Pediatrics, Faculty of Medicine, Semmelweis University, Budapest, Hungary
- 6Institute of Enzymology, Research Centre of Nature Sciences, Budapest, Hungary
- 7Ottawa University Hospital, University of Ottawa, Ottawa, ON, Canada
Targeting the immune system has emerged as an effective therapeutic strategy for the treatment of various tumor types, including Head and Neck Squamous Cell Carcinoma (HNSCC) and Non-small-Cell Lung Cancer (NSCLC), and checkpoint inhibitors have shown to improve patient survival in these tumor types. Unfortunately, not all cancers respond to these agents, making it necessary to identify responsive tumors. Several biomarkers of response have been described and clinically tested. As of yet what seems to be clear is that a pre-activation state of the immune system is necessary for these agents to be efficient. In this study, using established transcriptomic signatures, we identified a group of gene combination associated with favorable outcome in HNSCC linked to a higher presence of immune effector cells. CD2, CD3D, CD3E, and CXCR6 combined gene expression is associated with improved outcome of HNSCC patients and an increase of infiltrating immune effector cells. This new signature also identifies a subset of cervical squamous cell carcinoma (CSCC) patients with favorable prognosis, who show an increased presence of immune effector cells in the tumor, which outcome shows similarities with the HP-positive HNSCC cohort of patients. In addition, CD2, CD3D, CD3E, and CXCR6 signature is able to predict the best favorable prognosis in terms of overall survival of CSSC patients. Of note, these findings were not reproduced in other squamous cell carcinomas like esophageal SCC or lung SCC. Prospective confirmatory studies should be employed to validate these findings.
Introduction
Squamous Cell Carcinoma (SCC) includes a wide range of tumors originated from diverse anatomical locations that share common molecular and genetic features (1). SCCs arise from squamous and non-squamous epithelial tissues, and they are classified according to their location as head and neck, esophagus, lung, and cervix, among others (2). SCCs are in many occasions incurable diseases particularly in their advanced stages (1). This is the case for Head and Neck Squamous Cell Carcinoma (HNSCC) and Cervical Squamous Cell Carcinoma (CSCC), since the therapeutic options for both tumors, when diagnosed in the metastatic setting, are limited and outcome is severely compromised (3, 4). In both tumors, human papilloma virus (HPV) infection plays a central oncogenic role in a substantial proportion of cases and associates with aggressiveness and clinical outcome particularly in HNSCC (5). The classical treatment for HNSCC includes chemotherapies based on platinum agents and taxanes combined with anti-EGFR antibodies, which has demonstrated to improve survival (3). Recently, immunomodulators, particularly immune checkpoint inhibitors like pembrolizumab or nivolumab, have shown to improve relapse-free survival (RFS) and overall survival (OS) (5–8). Although this has dramatically changed the expected survival of HNSCC patients, the metastatic setting, nevertheless, remained an incurable condition (9). In a similar manner, immunotherapy has shown efficacy in CSCC patients with recurrent or metastatic cancers with disease progression or after chemotherapy when tumors express PD-L1 (Combined Positive Score, CPS ≥1) (10). In other tumor types like non-small-cell lung (NSCLC) or bladder cancer, checkpoint inhibitors have also demonstrated to provide clinical efficacy (6–8, 11). However, blocking of immune inhibitory signals with antibodies against PD1 or PD-L1 does not always result in clinical response (12, 13). Activation of the immune system, including the presence of effector T cells in the tumor, is a main requisite for these therapies to be effective (14). In addition, expression of PD1 or PD-L1 or the presence of Tumor Infiltrating Lymphocytes (TIL) is associated with favorable survival, independently of the therapy administered, confirming the relevant role of the immune system in the antitumoral action (15, 16).
Identification of genomic correlations of immune activation is an approach that could permit the selection of tumors susceptible to respond to immunotherapies. In this context, the mutational burden or altered mismatch repair mechanisms have been described as predictors of response to immunotherapies in several types of tumors (17–19). Likewise, some molecular alterations have been described as linked to the lack of activity of immunotherapeutic agents including JAK2 or B2M mutations (20). Regardless, recognition of immune pre-activated tumors, usually associated with favorable prognosis, is a requisite for most immune therapies to be efficient.
In this article we explored gene sets that predict favorable prognosis in HNSCC, with the aim to identify pre-activated immune tumors. We identified a transcriptomic signature associated with favorable outcome and linked with the infiltration of effector immune cells in this tumor. Similar findings were observed in Cervical SCC (CSCC), confirming its relevance.
Material and Methods
Immune Gene Signatures
Previously described immune signatures, i.e., expanded immune gene signature (CD3D, IL2RG, CXCL10, IDO1, NKG7, CIITA, HLA-E, HLA-DRA, CD3E, CXCR6, STAT1, CCL5, LAG3, GZMK, TAGAP, CD2, STAT1, CXCL13, GZMB), IFN gamma signature (IDO1, CXCL10, CXCL9, HLA-DRA, IRF9, IFNG, STAT1), cytotoxic T lymphocyte (CTL) signature (CD8A, CD8B, GZMA, GZMB, and PRF1), and HLA genes (HLA-A, HLA-B) were used to study the prognostic capacity of the genes composing each signature (21–23).
Clinical Outcome Analysis of Individual Genes and Signatures
The KM Plotter Online Tool (http://www.kmplot.com) (24, 25) was used to explore the relationship between the expression of described gene signatures (expanded immune gene signature, IFN gamma signature, CTL signature, and HLA genes) and the newly identified signature (CD2, CD3D, CD3E, and CXCR6) with patient clinical outcomes. We evaluated the prognostic values of mRNA expression of previously described gene signatures, for overall survival (OS) in a cohort of HNSCC patients (n=527) in all stages from the Cancer Genome Atlas (TCGA) database.
Briefly, publicly available RNA-seq HTSeq count files obtained from Illumina HiSeq 2000 RNA Sequencing Version 2 platform were analyzed for quantification of mRNA expression. Negative binomial distribution method was used through DESeq package to normalize the raw count data, and Bioconductor AnnotationDbi package (http://bioconductor.org/packages/AnnotationDbi/) was employed to annotate Ensembl transcript IDs with gene symbols (n = 25,228). After that, second scaling normalization was performed to calculate the mean expression of all genes in each patient sample to 1,000 to reduce batch effects.
In order to determine the correlation between gene expression and OS, Cox proportional hazards regression analysis was performed by using the Survival R package v2.38 (http://CRAN.R-project.org/package=survival/). Log-rank P values, hazard ratios (HR), and 95% confidence intervals (CI) were calculated. In terms of statistical analysis, false discovery rate (FDR) was computed to correct for multiple hypothesis testing, and the result was only accepted as significant in the case of FDR < 10%. Each possible cutoff was evaluated between the highest and lowest quartile of expression, and the best performing threshold with the lowest p value was used in the final analysis when drawing the Kaplan–Meier plot. In addition, multivariate survival analysis was performed for the gene expression and clinical features to assess independence from known epidemiological and clinical variables, including race, sex, age, tumor stage, and tumor grade when available. Finally, only genes associated with good outcome (HR<0.65, p<0.05, and FDR≤ 5%) were selected after screening through KM Plotter.
According to the results, the gene expression of the individual genes and the newly identified signature (CD2, CD3D, CD3E, and CXCR6) were assessed for OS in the different cohorts including HNSCC (n= 527), Esophageal SCC (n=81), Lung SCC (n=501), and Cervical SCC (n=254). In case we identified an association with multiple genes, the mean expression of the selected genes was used. Patients were divided according to the best cutoff values of the gene expression [lowest p-value (p)] into high vs low expression.
For graphical representation, a heatmap plot was performed using GraphPad Prism 8.0 tool. Survival HR parameter was represented as labels overlaid on the graph. The scale color meaning was represented as follows: blue, favorable outcome; red, detrimental outcome. Detailed information about the patients and clinical variables that were included in this study are resumed in Table 1.
Analysis of Tumor Mutational Burden and HPV
Clinicopathological characteristics of patients, including stage, grade, sex, race, including tumor mutational burden (TMB), were available and allowed to restrict the analysis in the cited KM Plotter Online Tool (http://www.kmplot.com).
The TMB was determined from whole-exome sequencing data from TCGA datasets used as the number of genes with a mutation. A gene was assigned “mutated” in case it had, at least, one mutation. Then, the median number of mutations across all samples within each tumor type was determined and was used as a cutoff: samples that had more “mutated” genes were determined as “high TMB,” and samples that had fewer “mutated” genes were assigned to the “low TMB” cohort.
In terms of HPV status detection, the Cancer Genomic Atlas (TCGA) dataset used the HPV16 DNA genotyping and mRNA expression to detect HPV oncoprotein transcripts.
Association Between Tumor Immune Infiltrates and Gene Expression
The correlation between gene expression and the presence of tumor immune infiltrates (CD8+T cells, NK cells, macrophages, and dendritic cells) in HNSCC and CSCC was analyzed using the Tumor Immune Estimation Resource (TIMER 2.0) platform (http://cistrome.org/TIMER/) (26, 27), a dataset that contains 10,897 samples from diverse cancer types available in the TCGA database. To analyze the relationship between tumor gene expression and immune infiltration, the available “Gene Module” from TIMER 2.0 was used. TIMER 2.0 uses an R package that integrates six computational algorithms to associate the tumor immune infiltrate populations with genomic and transcriptomic changes in the tumors (based on microarrays or RNAsequencing data), providing an estimation of immune infiltration levels for TCGA database or user-provided tumor profiles. To make the estimations of the immune cell populations, the cited algorithms are based on gene signature-based approaches utilizing a list of cell-type-specific gene sets and using the expression values of these signature gene sets in tissue samples. Specific tissue types, distinct cancer-cell intrinsic gene expression, and different immune cell types are considered to establish Spearman’s correlations between the expression of the input gene and the abundance of the immune cell type as well as its subtypes across cancer types under study. These algorithms were applied to the expression profiles of the Cancer Genome Atlas (TCGA) tumors, allowing to explore various associations between immune infiltrates and genetic features in the TCGA cohorts. The association between the immune infiltrates and the clinical features, such as HPV infection condition, was possible sorting patient cohorts in case of HNSCC. The results are displayed as a functional heatmap, and by clicking on each box of the heatmap, subsequently it generates a scatter plot showing the association of the gene expression with the infiltrated immune cell type. “Purity adjusted” option was selected (the correlation of the given gene expression with tumor purity as proportion of cancer cells in a sample), and the most immune cell types are negatively correlated with tumor purity (data not shown). Partial Spearman’s correlation was used to perform this association analysis, and statistical significance was expressed (p<0.05). Correlation value was displayed by “Rho” parameter. Positive correlation is associated with Rho>0 values, and negative correlation is associated with Rho<0 values.
Results
Evaluation of Immune Activated Signatures and Clinical Outcome in HNSCC
With the main goal to identify immunologic correlates associated with prognosis in HNSCC, we took advantage of previous published immune transcriptomic signatures (21–23). We first explored the association of the genes included in each signature with favorable prognosis, and latter their correlation with immune populations as described in Materials and Methods (Figure 1A). The correlation analysis between each immune signature and the 32 individual genes with OS is displayed as a heatmap in Figure 1B and Table 2. Favorable survival was observed for the expanded immune genes signature (HR=0.72; 95% confidence intervals CI=0.55–0.94; log rank p=0.016), the IFN gamma signature (HR=0.66; 95% CI=0.50–0.87; log rank p=0.0028), and the CTL level signature (HR=0.68; 95% CI=0.52–0.89; log rank p=0.0053) (Figure 1B). Only the HLA signature predicted unfavorable survival (HR=1.25; 95% CI=0.92–1.70; log rank p=0.150) (Figure 1B). For the whole population of tumors (all stages, n=527), most individual genes were associated with favorable outcome (Table 2). The different gene signatures did not predict for better OS in comparison with some individual transcripts, particularly with CD2 (HR=0.59; 95% CI=0.45–0.77; log rank p= 7.5e−05), CD3D (HR=0.59; 95% CI=0.45–0.77; log rank p=0.0001), CD3E (HR=0.60; 95% CI=0.46–0.78; log rank p=0.00013), and CXCR6 (HR=0.59; 95% CI=0.45–0.78; log rank p=0.00023) (Table 2). In addition, these individual genes presented a smaller FDR (FDR<10%) compared with the other analyzed genes and signatures (Figure 1C).
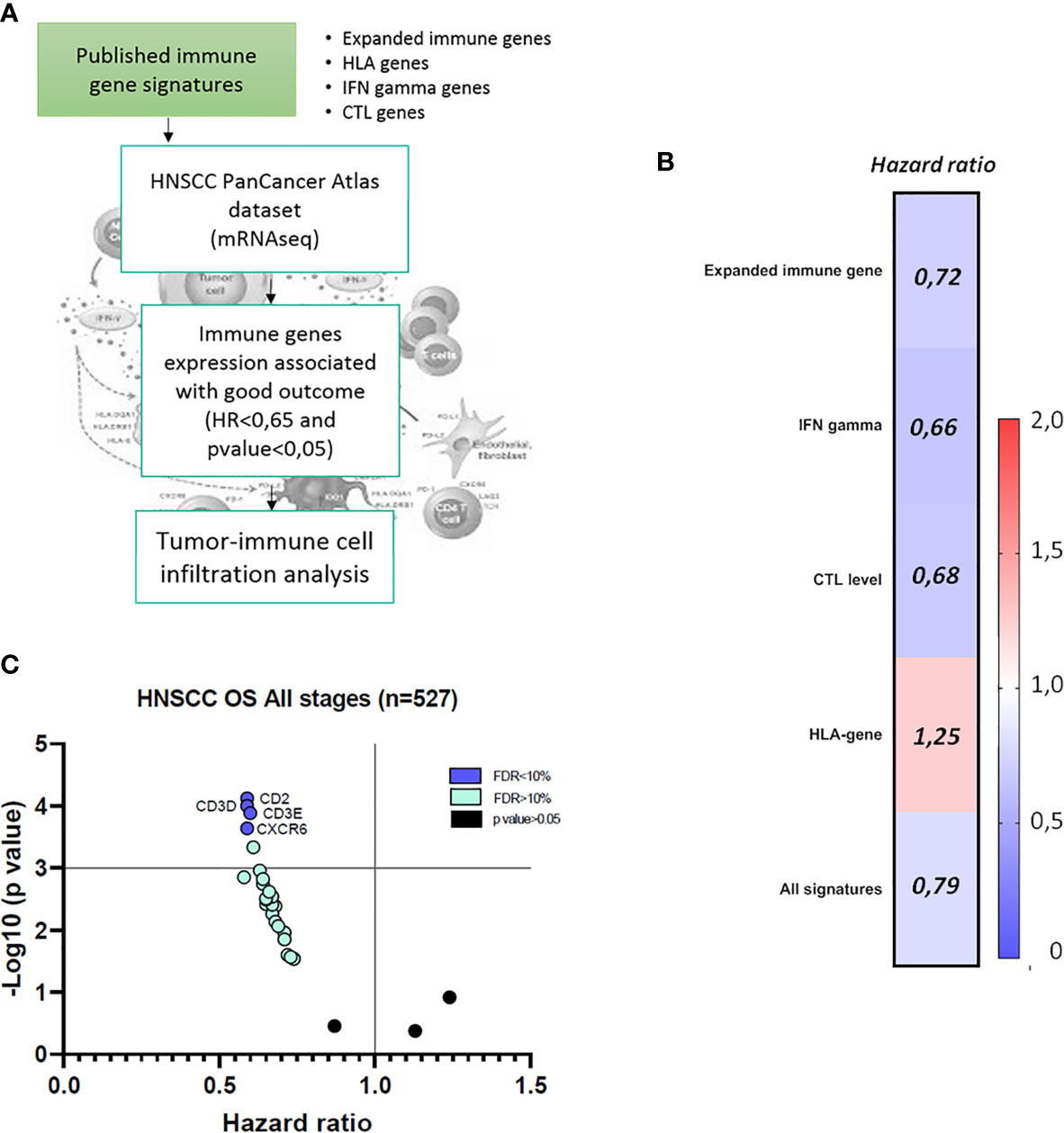
Figure 1 Evaluation of the impact of immune gene signatures (Expanded immune genes, IFN gamma genes, CTL level, and HLA genes) on clinical outcome in Head and Neck Squamous Cell Carcinoma (HNSCC) patients. (A) Flow chart of the analysis of the four published immune gene signatures and the transcriptomic expression effect in HNSCC patients’ clinical outcome and the correlation with tumor immune cell infiltrate composition. (B) Hazard ratio (HR) heat map for risk of death of each published immune gene signatures (Expanded immune genes, IFN gamma genes, CTL levels, and HLA genes) and combined, in association with overall survival (OS) in HNSCC patients at all stages (n = 527), using data from TCGA database as described in Materials and Methods. HR < 1 discriminates a risk reduction. Blue color represents favorable prognosis, and red color represents detrimental prognosis, with 95% confidence interval (CI) and p value < 0.05. (C) Graph representation of immune genes with the most favorable outcome in HNSCC (HR < 1, p value < 0.05 and FDR < 10%), in blue spots. Green spots represent immune genes with good prognosis and FDR > 10% and p < 0.05. Black spots represent immune genes without statistical significance (p value > 0.05).
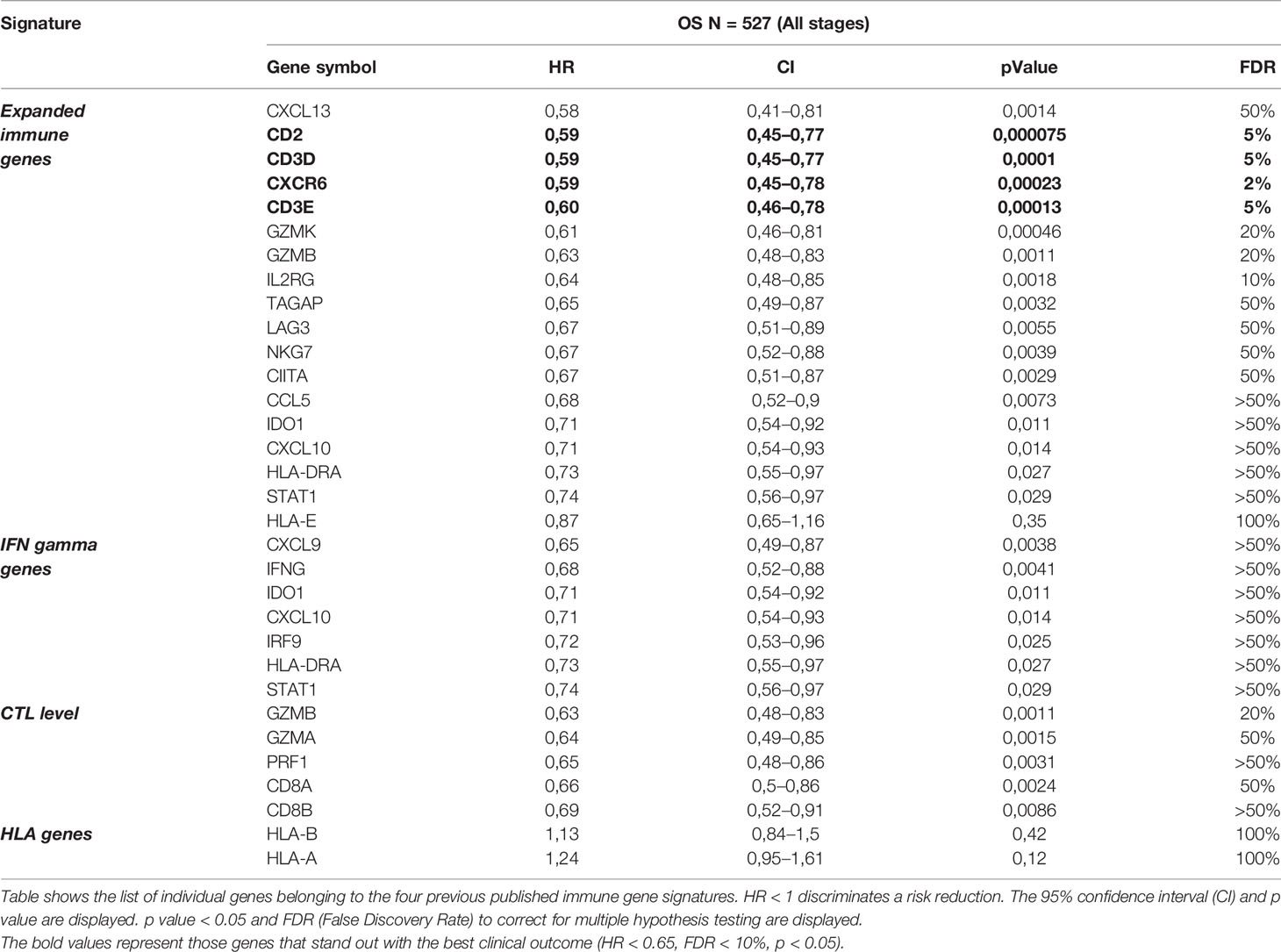
Table 2 Clinical outcome of individual immune genes from the four immune gene signatures in terms of overall survival (OS) of HNSCC patients.
Considering these results, we decided to analyze the gene set combination of CD2, CD3D, CD3E, and CXRC6.
The expression of PDL1 or PD1 is associated with favorable clinical outcome and improved response to immunotherapy including HNSCC tumors (5–8). We identified a positive correlation between the new immune gene signature (CD2, CD3D, CD3E, CXCR6) and CD274 (PD-L1) expression in HNSCC patients (Spearman rank correlation coefficient = 0.56; P=2.4E-43), which could support the outcome prediction in this group.
The Combined Gene Signature CD2, CD3D, CD3E, and CXCR6 Predicted Favorable Prognosis in Different HNSCC Clinical Stages
Next, we tested whether a new signature composed by CD2, CD3D, CD3E, and CXRC6 could improve the potential prediction capacity in HNSCC patients. The combined immune signature demonstrated a higher prediction in the stage II and III patient subgroups, even with a small number of patients: for stage II (n=69), HR=0.39; 95% CI=0.15–0.99; log rank p=0.041; and stage III subgroup: (n=78), HR=0.31; 95% CI=0.15–0.66; log rank p=0.0012). For all stages the combined signature also predicted favorable survival: (n=527), HR=0.58; 95% CI=0.44–0.76; log rank p=8e-05). Results in stage IV were also significant but with less magnitude of benefit compared with the other subgroups: (n=259), HR=0.62; 95% CI=0.43–0.89; log rank p=0.0089) (Figure 2).
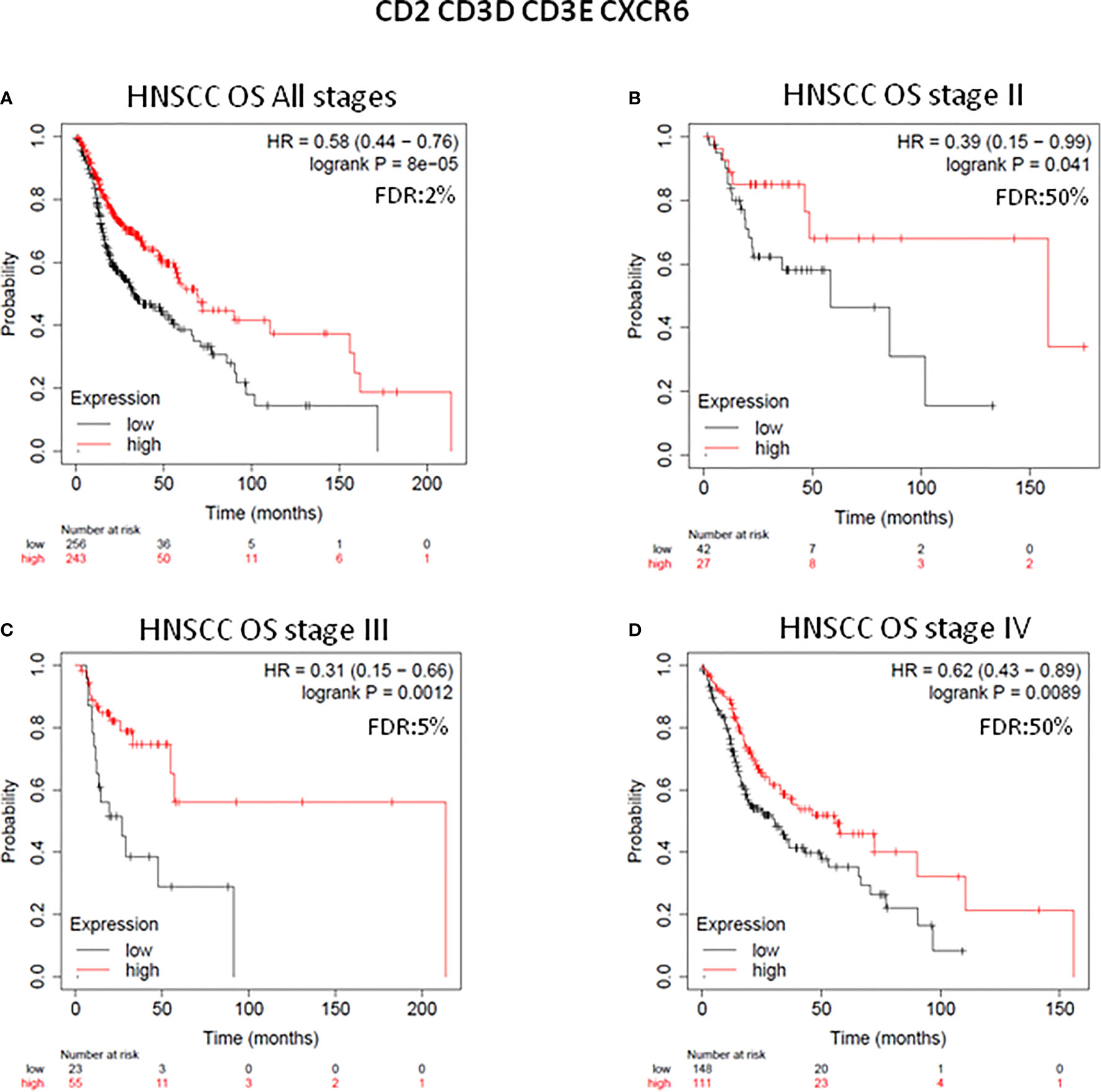
Figure 2 Clinical outcome analysis of CD2, CD3D, CD3E, CXCR6 immune gene-set combination in each stage of HNSCC patients. Survival plots of the combination of four immune genes with the most favorable prognosis (HR < 0.65, p value < 0.05, and FDR < 10%) at all stages of HNSC patients (n = 527) (A), at stage II (n = 69) (B), at stage III (n = 78) (C), and at stage IV (n = 259) (D) are displayed. Patients whose tumors harbor high gene expression levels predicted better survival (red line), and those with low gene expression levels predicted worse survival (black line). Number of patients at risk at every time (months), with high (in red) and low gene expression (in black) are displayed. HR for risk of death and OS are displayed. HR < 0.65 discriminates a risk reduction. FDR is also displayed. The gene combination is displayed at the top of the figure.
CD2, CD3D, CD3E, and CXCR6 Expression Is Associated With Infiltration of Memory CD8+ T, Activated NK, Dendritic Cells, and M1 Macrophages in HPV-Positive HNSCC
We next explored the association of the expression at an individual transcriptomic level of CD2, CD3D, CD3E, and CXRC6 with the presence of tumor-infiltrating immune cell populations. All genes had a negative correlation with tumor purity, demonstrating the high presence of immune populations.
In HPV-positive HNSCC tumors (n=98), we observed the strongest positive correlation with central memory CD8+ T cell subpopulation (CD2: Rho=0.904, CD3D: Rho=0.933, CD3E: Rho=0.938 and CXCR6: Rho=0.886) and also a positive correlation with effector memory CD8+ T cells (CD2: Rho=0.612, CD3D: Rho=0.693, CD3E: Rho=0.623 and CXCR6: Rho=0.606) (Figure 3). In the case of NK cells, the stronger association was found for activated NK cells (CD2: Rho=0.573, CD3D: Rho=0.644, CD3E: Rho=0.576 and CXCR6: Rho=0.536), compared with a negative association with resting NK cells (CD2: Rho=−0.336, CD3D: Rho=−0.425, CD3E: Rho=−0.370 and CXCR6: Rho=−0.289). Regarding the macrophage population, HPV-positive tumors showed a positive correlation with both subtypes (M1 and M2): M1 (CD2: Rho=0.632, CD3D: Rho=0.631, CD3E: Rho=0.683, and CXCR6: Rho=0.664), and less significant for the M2 subtype (CD2: Rho=0.334, CD3D: Rho=0.203, CD3E: Rho=0.289, and CXCR6: Rho=0.358) (Figure 3). For the activated dendritic cell population, a strong correlation was observed (CD2: Rho=0.797, CD3D: Rho=0.814, CD3E: Rho=0.814, and CXCR6: Rho=0.771) (Figure 3).
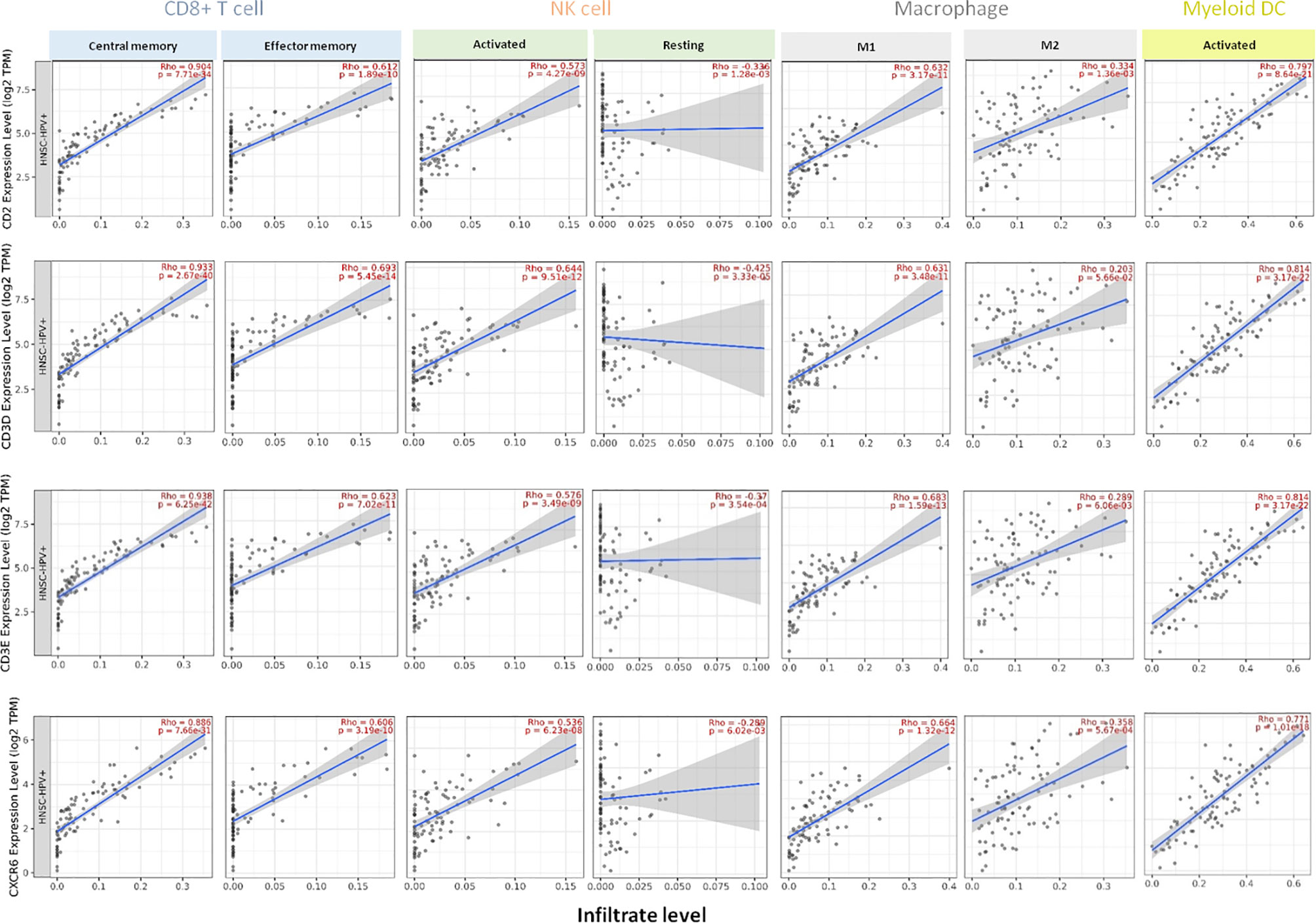
Figure 3 Association of the expression of CD2, CD3D, CD3E, CXCR6 with specific tumor-infiltrating immune cell populations in HPV-positive HNSCC patients. Expression of each gene and association with the presence of CD8+ T cells (central memory and effector memory subsets), NK cells (activated and resting), macrophages (M1 and M2 types), and activated myeloid dendritic cells in HPV-positive HNSCC (n = 98). Each panel presents the relationship between infiltrates estimation value with the purity-adjusted spearman’s correlation parameter Rho and gene expression join p value < 0.001. Rho value > 0 represents a positive correlation, and Rho < 0 represents a negative correlation. Association with immune cell populations was provided by TIMER 2.0 software and was correlated with transcriptome expression level of each immune gene, as described in Material and Methods.
In case of HPV-negative HNSCC tumors (n=422), we found a positive correlation between the gene expression level of CD2, CD3D, CD3E, and CXRC6, and CD8+ T cells subpopulations, finding the higher correlation with CD8+ central memory cells (CD2: Rho=0.817, CD3D: Rho=0.800, CD3E: Rho=0.826, and CXCR6: Rho=0.797). A less significant association was observed for the CD8+ effector memory cells (CD2: Rho=0.570, CD3D: Rho=0.592, CD3E: Rho=0.550, and CXCR6: Rho=0.570) (Figure 4). In addition, we found a positive association between the gene expression level of CD2, CD3D, CD3E, and CXRC6 and the activated fraction of NK cells present in the tumor (CD2: Rho=0.442, CD3D: Rho=0.460, CD3E: Rho=0.423, and CXCR6: Rho=0.401). A negative association with resting NK cells (CD2: Rho=−0.059, CD3D: Rho=−0.111, CD3E: Rho=−0.039, and CXCR6: Rho=0.02) was observed. Similar findings were observed for M1 macrophage infiltrates (CD2: Rho=0.760, CD3D: Rho=0.706, CD3E: Rho=0.747, and CXCR6: Rho=0.744) and M2 macrophages (CD2: Rho=0.753, CD3D: Rho=0.667, CD3E: Rho=0.748, and CXCR6: Rho=0.715), being the association with M2 macrophages higher than those observed for HPV-positive HNSCC tumors. Finally, we analyzed the activation state of the myeloid dendritic cell subpopulation, observing a high correlation with activated dendritic cells (CD2: Rho=0.829, CD3D: Rho=0.796, CD3E: Rho=0.796, and CXCR6: Rho=0.760) (Figure 4).
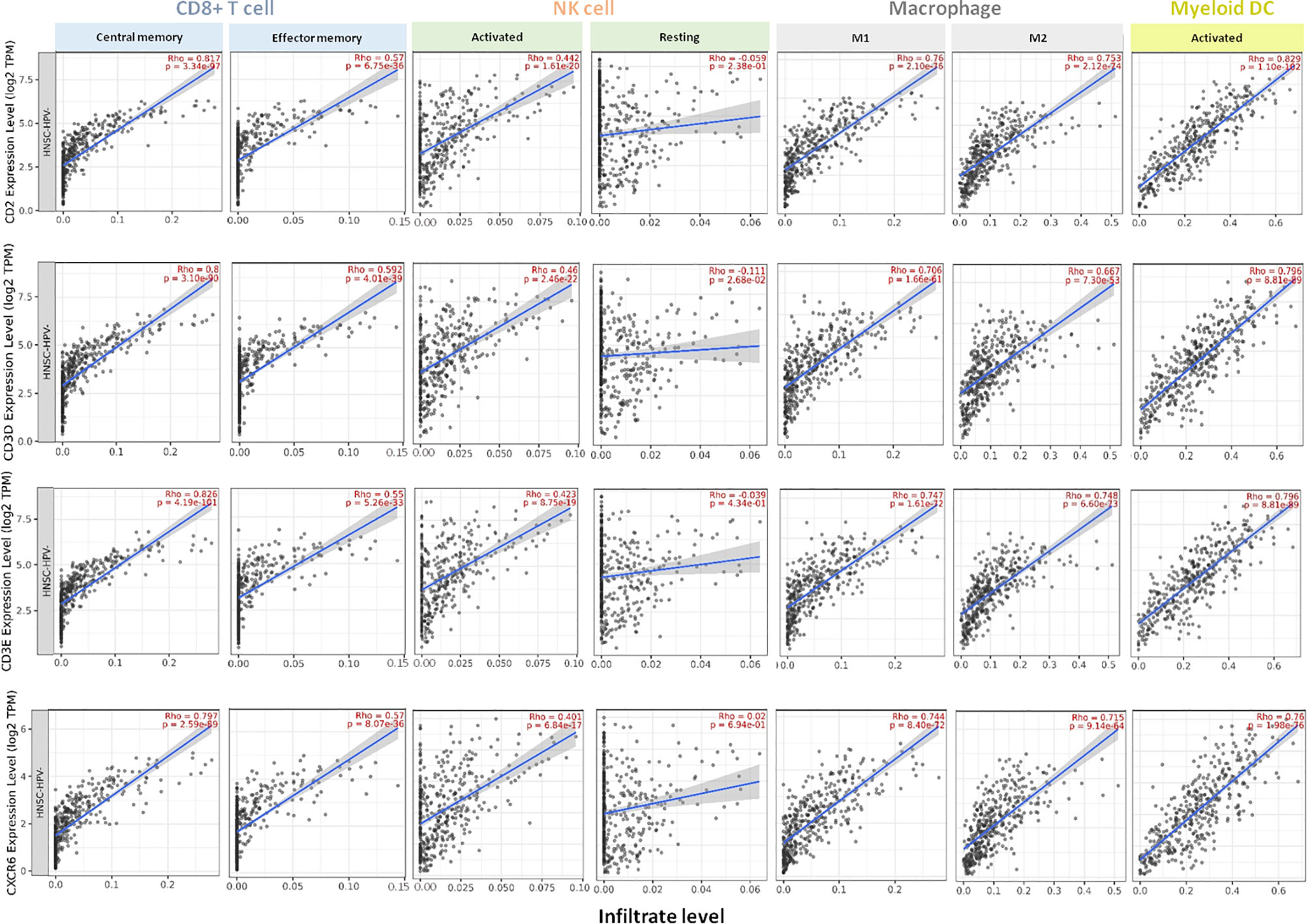
Figure 4 Association of the expression of CD2, CD3D, CD3E, CXCR6 with specific tumor-infiltrating immune cell populations in HPV-negative HNSCC patients. Expression of each gene and association with the presence of CD8+ T cells (central memory and effector memory subsets), NK cells (activated and resting), macrophages (M1 and M2 types), and activated myeloid dendritic cells in HPV-negative HNSCC (n = 422). Each panel presents the relationship between infiltrates estimation value with the purity-adjusted spearman’s correlation parameter Rho and gene expression join p value < 0.001. Rho value > 0 represents a positive correlation, and Rho < 0 represents a negative correlation. Association with immune cell populations was provided by TIMER 2.0 software and was correlated with transcriptome expression level of each immune gene.
Evaluation of Immune Activated Genes in Other Squamous Cell Tumors
SCCs arise in a different locations and share molecular and genetic alterations (1). In this context, we decided to explore if the expression of four previous published immune gene signatures (Expanded immune genes, IFN gamma genes, CTL genes, and HLA) and the new identified CD2, CD3D, CD3E, and CXCR6 gene combination were able to classify patients with different outcome in lung, esophageal, and cervical SCC. Unexpectedly, no significant effect of four previous published immune gene signatures was observed in lung SCC (data not shown), neither in the case of the evaluated 32 individual genes (Table 3) or in case of the new CD2, CD3D, CD3E, and CXCR6 signature: (n=504), HR=0.85, 95% CI=0.64−1.13; log rank p=0.27 (Supplementary Figure 1). However, a detrimental outcome was observed for both, the 32 individual genes (Table 4), and the new signature in esophageal SCC (n=81; HR=2.92; 95% CI=1.19−7.18; log rank p=0.015) (Supplementary Figure 1).
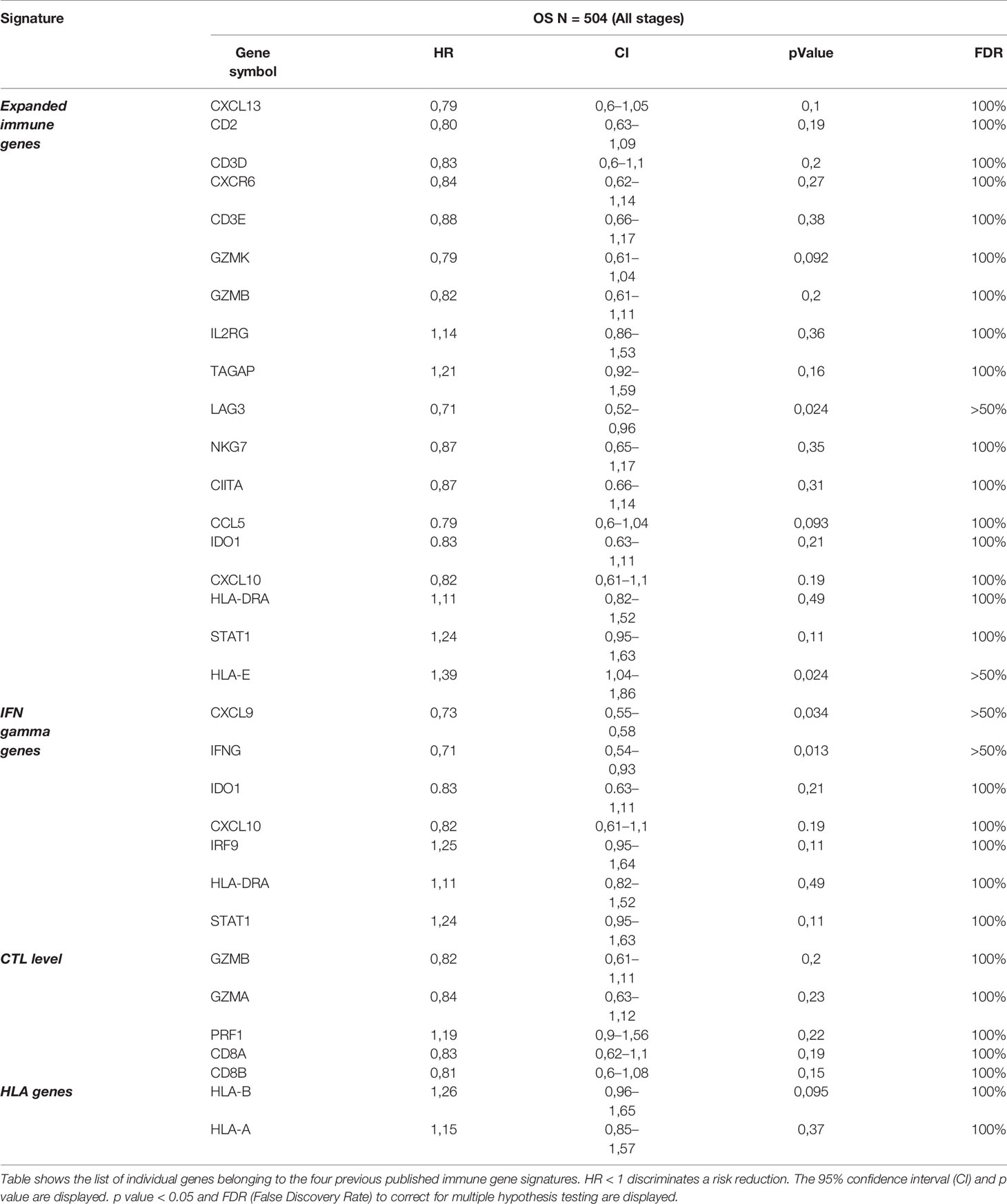
Table 3 Clinical outcome of individual immune genes from the four immune gene signatures in terms of overall survival (OS) of LSCC patients.
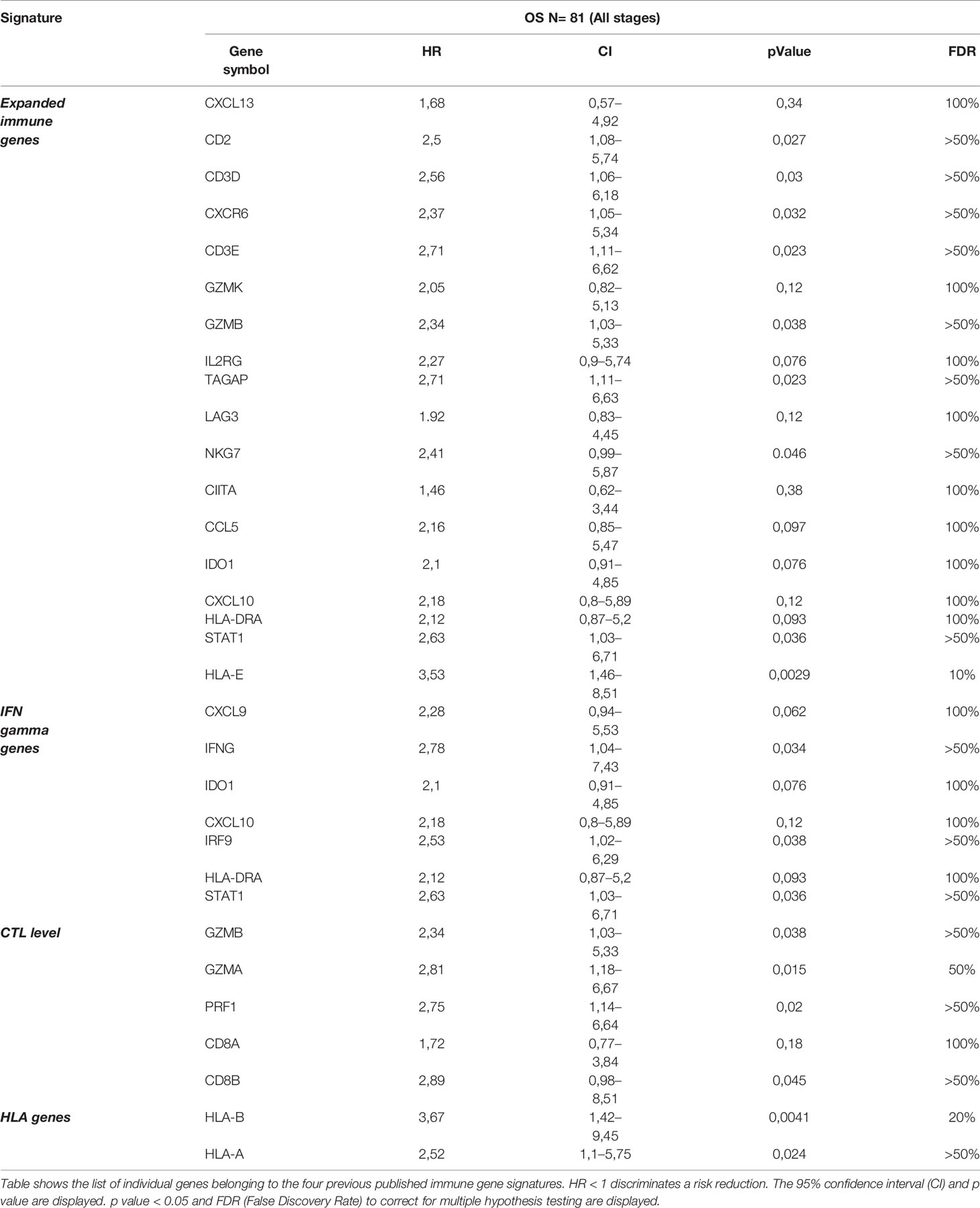
Table 4 Clinical outcome of individual immune genes from the four immune gene signatures in terms of overall survival (OS) of ESCC patients.
In CSCC we found that the expression of the analyzed immune signatures (Expanded immune genes, IFN gamma genes, CTL genes, and HLA genes) were associated with favorable OS in all stages (HR=0.6; 95% CI=0.35–1.04; log rank p=0.068) (Figure 5A and Table 5), showing similar results with that in the of case of HNSCC. Most individual genes were associated with favorable outcome at all stages (n: 254; Table 5), and the previously selected genes showed the most favorable prediction capacity: CD2 (HR=0.41; 95% CI=0.26−0.66; log rank p= 0,0001), CD3D (HR=0.39; 95% CI=0.24–0.64; log rank p= 8,5e−05), CD3E (HR=0.42; 95% CI=0.26–0.67; log rank p=0.0002), and CXCR6 (HR=0.44; 95% CI=0.27–0.70; log rank p=0.0004). Of note, in the CSCC cohort, we identified that expression of LAG3 was associated with very favorable outcome (HR=0.3; 95% CI=0.15−0.61; log rank p= 0,0004). We next evaluated the combined effect of the identified immune gene signature (combining CD2, CD3D, CD3E, and CXCR6), demonstrating a favorable prognosis (HR=0.43; 95% CI=0.27–0.69; log rank p=0.00031) with a low FDR (<5%) (Figure 5B).
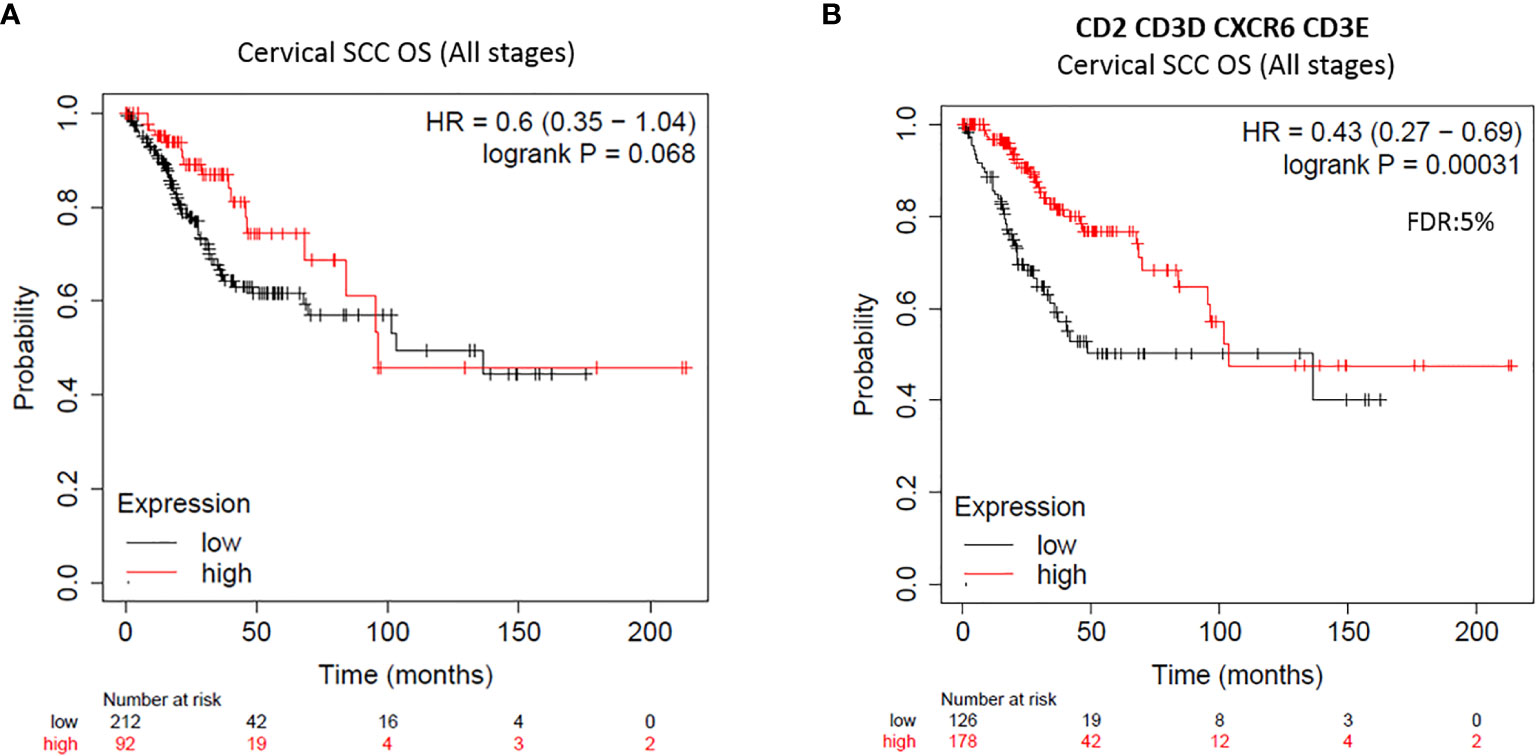
Figure 5 Expression of CD2, CD3D, CD3E, CXCR6 immune gene combination shows better clinical outcome of CSSC patients. Clinical outcome of survival plots in CSCC at all stages (n = 254) with transcriptomic expression of all four previous published immune signatures (A) and with the new immune gene-set combination CD2, CD3D, CD3E, CXCR6 (HR < 0.65 and FDR = 5%) (B). HR for risk of death and OS are displayed. HR < 0.65 discriminates a risk reduction. FDR is also displayed.
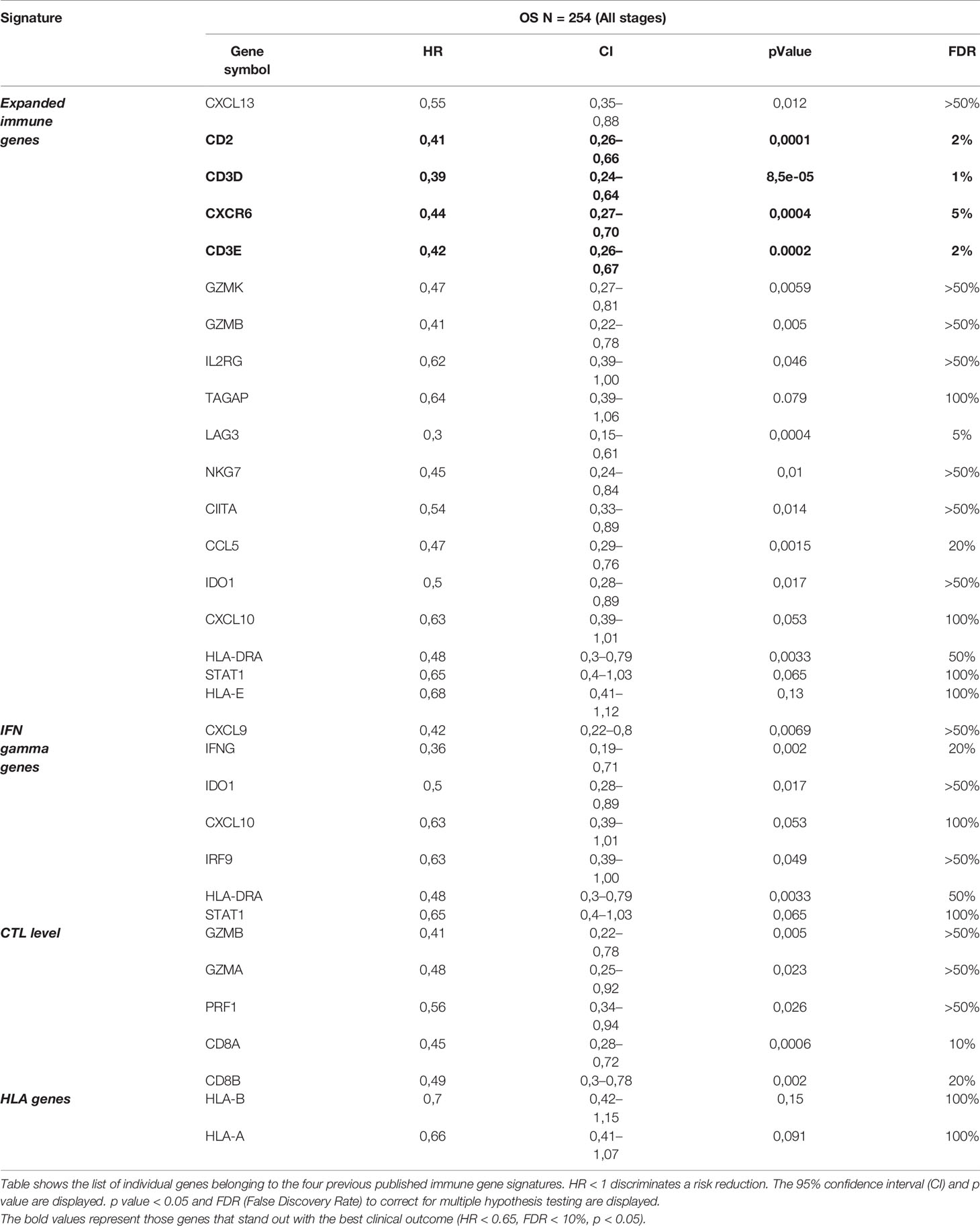
Table 5 Clinical outcome of individual immune genes from the four immune gene signatures in terms of overall survival (OS) of CSCC patients.
The assessment of the new immune gene signature (CD2, CD3D, CD3E, and CXCRC6) in the CSCC cohort per tumor stage was not possible because sample size was too low for a meaningful analysis when we filtered data by stage.
Expression of CD2, CD3D, CD3E, and CXCR6 Is Linked to a High Expression of Memory CD8+ T Cells, Activated NK Cells, and M1 Macrophages in CSCC
Since CD2, CD3D, CD3E, and CXCR6 predict very favorable prognosis in CSCC, we evaluated the association of this new signature at transcriptomic level with the presence of tumor-infiltrating immune cell populations in CSCC. All genes showed a negative correlation with tumor purity. As in the case of HNSCC patients, we found a positive correlation between the gene expression of CD2, CD3D, CD3E, and CXCR6 and CD8+ T cells (central and effector memory subpopulations), and the strongest correlation was observed for the central memory CD8+ T cells (CD2: Rho=0.881, CD3D: Rho=0.883, CD3E: Rho=0.896, and CXCR6: Rho=0.850) (Figure 6). A positive association was also observed for the CD8+ effector memory subpopulation (CD2: Rho=0.665, CD3D: Rho=0.700, CD3E: Rho=0.631, and CXCR6: Rho=0.636) (Figure 6).
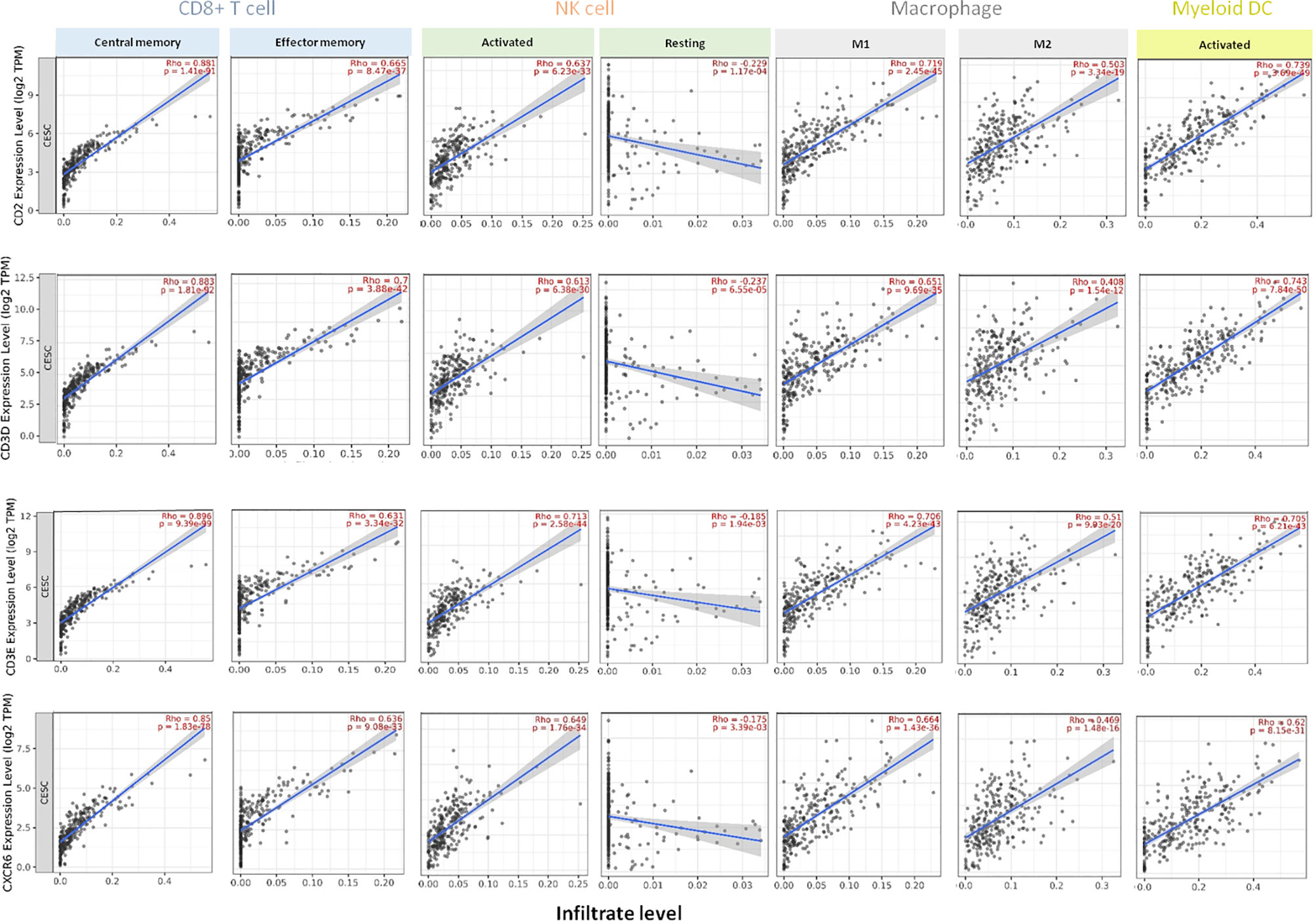
Figure 6 Association of CD2, CD3D, CD3E, CXCR6 transcriptomic expression with specific tumor-infiltrating immune cell populations in CSCC patients. Expression of each gene in association with the presence of CD8+ T cells (central memory and effector memory subsets), NK cells (activated or resting), macrophages (M1 and M2 types), and activated myeloid dendritic infiltrates in CSSC patients (n = 254). Each panel presents the relationship between infiltrates estimation value with the purity-adjusted spearman’s correlation parameter Rho and gene expression, join p value < 0.001. Rho value > 0 represents a positive correlation, and Rho < 0 represents a negative correlation. Association with immune cell populations was provided by TIMER 2.0 software and was correlated with transcriptome expression level of each immune gene.
In case of the NK cell population, the strongest correlation was found with the activated fraction (CD2: Rho=0.637, CD3D: Rho=0.613, CD3E: Rho=0.713, and CXCR6: Rho=0.649). In the macrophage population, the highest association was identified for the M1 group (CD2: Rho=0.719, CD3D: Rho=0.651, CD3E: Rho=0.706, and CXCR6: Rho=0.664) compared with the M2 macrophage population (CD2: Rho=0.503, CD3D: Rho=0.408, CD3E: Rho=0.510, and CXCR6: Rho=0.469). For activated dendritic cells, the results were as follow: CD2: Rho=0.739, CD3D: Rho=0.743, CD3E: Rho=0.705, and CXCR6: Rho=0.620) (Figure 6).
Clinical Outcome in HNSCC and CSSC With Expression of CD2, CD3D, CD3E, CXCR6 and High Mutation Burden
As the mutational burden has been associated with response to immune-modulatory drugs (18, 19), we explored if the expression of the identified immune signature CD2, CD3D, CD3E, CXCR6 was able to predict a better outcome in HNSCC and in CSCC with high mutational burden. In HNSCC with high mutational burden, the presence of the immune signature was able to predict favorable OS (n=251; HR=0.53; 95% CI=0.37–0.76; log rank p=0.00051; FDR=5%) (Figure 7A). Similarly, in CSCC tumors with high mutational burden, the presence of the immune signature predicted strongly favorable survival (n=143; HR=0.19; 95% CI=0.09–0.39; log rank p=4.5e-07, FDR=1%) (Figure 7B). This result suggests that outcome prediction of the aforementioned immune signature is much more effective in HSCC and CSCC tumors with high mutational burden.
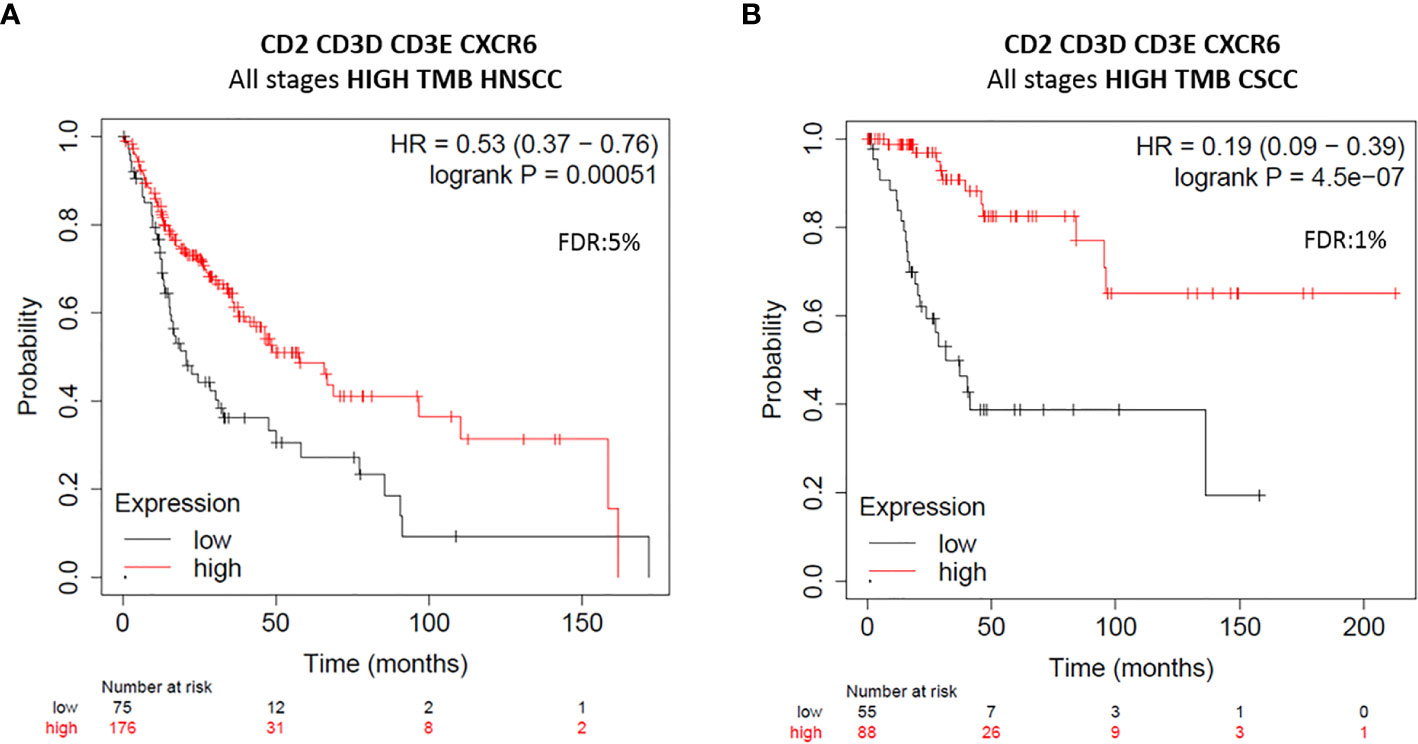
Figure 7 Clinical outcome analysis of CD2, CD3D, CD3E, CXCR6 immune gene signature expression in HNSCC and CSCC tumors with high mutational burden. Clinical outcomes of survival plots of new immune gene signature with improved favorable prognosis at all stages of HNSCC tumors (n = 251) (A) and CSCC tumors (n = 143) (B) with high mutational burden (TMB) are displayed. Red line represents survival of patients whose tumors harbor high gene expression levels, and those with low gene expression levels show in black line. Number of patients at risk at every time (months), with high (in red) and low gene expression (in black), is displayed. HR for risk of death and OS are displayed. HR < 0.65 discriminates a risk reduction. FDR is also displayed. The gene combination is displayed at the top of each figure.
Discussion
In the present article we describe immune genomic signatures associated with favorable outcome in HNSCC and CSCC. Identification of genomic immune correlates that predict outcome as an indirect measure of immune activation is a key task in oncology.
SCCs comprise a large family of tumors from epithelial tissues that arise from different locations but that share some common biological characteristics like genomic instability, dysfunction of DNA repair mechanisms, or relative sensibility to therapeutic agents that induce DNA damage or affect DNA repair mechanisms (1). In addition, some of them have shown to be more sensitive to immunologic agents probably due to the retained viral antigens produced by the presence of HPV infection (4, 5).
Using previously described transcriptomic signatures associated with immune activation, we aimed to identify genes that were linked with favorable outcome in HNSCC patients. We found a correlation with outcome of most of the signatures except for the HLA one. The fact that some genes correlated better than the whole signature let us explore a combination of transcripts that could increase the prediction capacity, and this was the case for a signature that included only four genes: CD2, CD3D, CD3E, and CXCR6. Of note, these findings were reproduced in CSCC and with minor significance in esophagus tumors. The association between gene expression profile and clinical outcome in patients selecting by HPV condition was not available with KM Plotter Online Tool in the case of the HNSCC cohort of patients.
A remarkable finding is the fact that the identified results were not reproduced in other squamous cell lung cancers. These results, although surprising, confirm the heterogeneity of tumors at a location and histology level (28, 29). Probably, the results have been conditioned by the presence of HPV in these two indications: HNSCC and CSCC (most CSCC tumors are HPV positive), a situation that is not observed in other squamous cell tumors like esophagus or lung. These tumors lack the presence of HPV infection, so they do not exhibit the viral neoantigens or molecules likely recognized by the identified immune cell populations in HNSCC and CSCC.
The immune gene signature comprised several genes. CD3D and CD3E are part of the TCR-CD3 complex present on T-lymphocyte cell surface (30, 31). CD3 chains contain immunoreceptor tyrosine-based activation motifs (ITAMs) in their cytoplasmic domain, and after T-cell receptor engagement, they transmit the activation signaling by phosphorylation of SRC (30). In this context, the presence of these two genes is an indirect measure of the existence of activated T cells. It is not surprising to see that their presence has also been described as linked with prognosis (32). CD2 is a cell adhesion protein expressed on the T cell and NK cell surface and has been used as a specific marker for these two populations (33). Finally, CXCR6 has been described as a chemokine associated with the activation of IFN gamma effector cells, therefore can constitute an adequate marker to select active T cells (34).
The presence of the selected genes correlated with low tumor purity in HNSCC and CSCC. Moreover, we observed a very clear and strong association with the presence of populations of cells involved in adaptive immune cell response including activated T cells and dendritic cells. In addition, we observed an increase in M1 macrophages and in activated NK cells, demonstrating that the innate immunity was also present and therefore can have a role in the antitumoral action. This finding is relevant as a single signature of four genes can identify an immunologic state that is linked with a favorable prognosis compromising an adaptive and innate activated immune response. Unfortunately, no evaluation of immune populations in esophageal SCC was performed, since data are not publicly available.
When exploring differences between HNSCC HPV-positive and HPV-negative tumors, we did not observe major discrepancies beyond the fact that HPV-positive tumors had a stronger presence of the described immune cells (35). In fact, almost all CSCC tumors are HPV positive. As mentioned before, tumors in which a viral infection is a key oncogenic event can have a wider presence of neoantigens and therefore an increase presence of immune activated cells, what suggests that could be more sensitive to agents that modulate the immune system. In fact, we observed that the expression of the new immune signature correlated with the same composition of tumoral immune cell infiltrates in HNSCC and CSCC (see and compare Figure 3 and Figure 6), maybe since both types of tumors shared the same pattern of neoantigens.
A relevant finding of our study is the discrimination in outcome between those tumors with a high tumor mutational burden (TMB). In this context, not all tumors with TMB respond to immune modulators, which suggests that the identification of biomarkers within this population will also be of help.
We acknowledge that our study has limitations. This is an in silico analysis using datasets from different sources. However, all the datasets included in this study are publicly available and have been incorporated in several studies that support their consideration as representative of the general population. Of note, the compilation and integration of molecular biology and clinical data into the available datasets is sometimes scarce. For instance, we could not explore if in terms of expression our new signature had different prediction in the HNSCC cohort per HPV status. This was not the case in CSCC where all patients are HPV positive.
In conclusion, we describe a set of genes that are able to identify immune activated tumors involving adaptive and innate immune response, associated with favorable prognosis in HNSCC and CSCC. Prospective studies should be performed to confirm these results.
Data Availability Statement
Publicly available datasets were analyzed in this study. This data can be found here: https://kmplot.com/analysis/, http://cistrome.org/TIMER/download.html.
Author Contributions
AO, CS-L, MB-P, and AM have contributed to conception and design of the study. AO has performed the main design of the work. CS-L and MB-P have carried out the acquisition, analysis, or interpretation of data for the work. All authors contributed to the article and approved the submitted version.
Funding
This work has been supported by Instituto de Salud Carlos III (PI16/01121 and PI19/00808), ACEPAIN; Diputación de Albacete, CIBERONC and CRIS Cancer Foundation (to AO). Ministry of Economy and Competitiveness of Spain (BFU2015-71371-R), the Instituto de Salud Carlos III through the Spanish Cancer Centers Network Program (RD12/0036/0003) and CIBERONC, the scientific foundation of the AECC and the CRIS Foundation (to AP). The work carried out in our laboratories receive support from the European Community through the regional development funding program (FEDER).
Conflict of Interest
The authors declare that the research was conducted in the absence of any commercial or financial relationships that could be construed as a potential conflict of interest.
Publisher’s Note
All claims expressed in this article are solely those of the authors and do not necessarily represent those of their affiliated organizations, or those of the publisher, the editors and the reviewers. Any product that may be evaluated in this article, or claim that may be made by its manufacturer, is not guaranteed or endorsed by the publisher.
Supplementary Material
The Supplementary Material for this article can be found online at: https://www.frontiersin.org/articles/10.3389/fonc.2021.714550/full#supplementary-material
Supplementary Figure 1 | Clinical outcome analysis of CD2, CD3D, CD3E, CXCR6 immune gene signature expression in lung SCC and esophageal SCC tumors. Clinical outcomes of survival plots of new immune gene signature with no prediction at all stages of lung SCC tumors (n = 501) (A) and poor prognosis at all stages in esophageal SCC tumors (n = 81) (B) are displayed. Red line represents survival of patients whose tumors harbor high gene expression levels, and black line represents those with low gene expression levels. Number of patients at risk at every time (months) is displayed. HR for risk of death and overall survival (OS) are displayed. HR < 0.65 discriminates a risk reduction. FDR is also displayed. The gene combination is displayed at the top of each figure.
References
2. Sánchez-Danés A, Blanpain C. Deciphering the Cells of Origin of Squamous Cell Carcinomas. Nat Rev Cancer (2018) 18(9):549–61. doi: 10.1038/s41568-018-0024-5
3. Cramer JD, Burtness B, Le QT, Ferris RL. The Changing Therapeutic Landscape of Head and Neck Cancer. Nat Rev Clin Oncol (2019) 16(11):669–83. doi: 10.1038/s41571-019-0227-z
4. Wendel Naumann R, Leath CA 3rd. Advances in Immunotherapy for Cervical Cancer. Curr Opin Oncol (2020) 32(5):481–7. doi: 10.1097/CCO.0000000000000663
5. Cohen EEW, Soulières D, Le Tourneau C, Dinis J, Licitra L, Ahn M-J, et al. Pembrolizumab Versus Methotrexate, Docetaxel, or Cetuximab for Recurrent or Metastatic Head-and-Neck Squamous Cell Carcinoma (KEYNOTE-040): A Randomised, Open-Label, Phase 3 Study. Lancet (London England) (2019) 393(10167):156–67. doi: 10.1016/S0140-6736(18)31999-8
6. Chow LQM, Haddad R, Gupta S, Mahipal A, Mehra R, Tahara M, et al. Antitumor Activity of Pembrolizumab in Biomarker-Unselected Patients With Recurrent and/or Metastatic Head and Neck Squamous Cell Carcinoma: Results From the Phase Ib KEYNOTE-012 Expansion Cohort. J Clin Oncol Off J Am Soc Clin Oncol (2016) 34(32):3838–45. doi: 10.1200/JCO.2016.68.1478
7. Ferris RL, Blumenschein GJ, Fayette J, Guigay J, Colevas AD, Licitra L, et al. Nivolumab for Recurrent Squamous-Cell Carcinoma of the Head and Neck. N Engl J Med (2016) 375(19):1856–67. doi: 10.1056/NEJMoa1602252
8. Seiwert TY, Burtness B, Mehra R, Weiss J, Berger R, Eder JP, et al. Safety and Clinical Activity of Pembrolizumab for Treatment of Recurrent or Metastatic Squamous Cell Carcinoma of the Head and Neck (KEYNOTE-012): An Open-Label, Multicentre, Phase 1b Trial. Lancet Oncol (2016) 17(7):956–65. doi: 10.1016/S1470-2045(16)30066-3
9. Miyauchi S, Kim SS, Pang J, Gold KA, Gutkind JS, Califano JA, et al. Immune Modulation of Head and Neck Squamous Cell Carcinoma and the Tumor Microenvironment by Conventional Therapeutics. Clin Cancer Res an Off J Am Assoc Cancer Res (2019) 25(14):4211–23. doi: 10.1158/1078-0432.CCR-18-0871
10. Chung HC, Ros W, Delord J-P, Perets R, Italiano A, Shapira-Frommer R, et al. Efficacy and Safety of Pembrolizumab in Previously Treated Advanced Cervical Cancer: Results From the Phase II KEYNOTE-158 Study. J Clin Oncol Off J Am Soc Clin Oncol (2019) 37(17):1470–8. doi: 10.1200/JCO.18.01265
11. Mirza AH, Thomas G, Ottensmeier CH, King EV. Importance of the Immune System in Head and Neck Cancer. Head Neck (2019) 41(8):2789–800. doi: 10.1002/hed.25716
12. Mahoney KM, Rennert PD, Freeman GJ. Combination Cancer Immunotherapy and New Immunomodulatory Targets. Nat Rev Drug Discov (2015) 14(8):561–84. doi: 10.1038/nrd4591
13. Ribas A, Wolchok JD. Cancer Immunotherapy Using Checkpoint Blockade. Science (2018) 359(6382):1350–5. doi: 10.1126/science.aar4060
14. Topalian SL, Taube JM, Anders RA, Pardoll DM. Mechanism-Driven Biomarkers to Guide Immune Checkpoint Blockade in Cancer Therapy. Nat Rev Cancer (2016) 16(5):275–87. doi: 10.1038/nrc.2016.36
15. Adams S, Gray RJ, Demaria S, Goldstein L, Perez EA, Shulman LN, et al. Prognostic Value of Tumor-Infiltrating Lymphocytes in Triple-Negative Breast Cancers From Two Phase III Randomized Adjuvant Breast Cancer Trials: ECOG 2197 and ECOG 1199. J Clin Oncol Off J Am Soc Clin Oncol (2014) 32(27):2959–66. doi: 10.1200/JCO.2013.55.0491
16. Loi S, Michiels S, Salgado R, Sirtaine N, Jose V, Fumagalli D, et al. Tumor Infiltrating Lymphocytes are Prognostic in Triple Negative Breast Cancer and Predictive for Trastuzumab Benefit in Early Breast Cancer: Results From the FinHER Trial. Ann Oncol Off J Eur Soc Med Oncol (2014) 25(8):1544–50. doi: 10.1093/annonc/mdu112
17. Goodman AM, Kato S, Bazhenova L, Patel SP, Frampton GM, Miller V, et al. Tumor Mutational Burden as an Independent Predictor of Response to Immunotherapy in Diverse Cancers. Mol Cancer Ther (2017) 16(11):2598–608. doi: 10.1158/1535-7163.MCT-17-0386
18. Le DT, Durham JN, Smith KN, Wang H, Bartlett BR, Aulakh LK, et al. Mismatch Repair Deficiency Predicts Response of Solid Tumors to PD-1 Blockade. Science (2017) 357(6349):409–13. doi: 10.1126/science.aan6733
19. Mandal R, Samstein RM, Lee K-W, Havel JJ, Wang H, Krishna C, et al. Genetic Diversity of Tumors With Mismatch Repair Deficiency Influences Anti-PD-1 Immunotherapy Response. Science (2019) 364(6439):485–91. doi: 10.1126/science.aau0447
20. Havel JJ, Chowell D, Chan TA. The Evolving Landscape of Biomarkers for Checkpoint Inhibitor Immunotherapy. Nat Rev Cancer (2019) 19(3):133–50. doi: 10.1038/s41568-019-0116-x
21. Rooney MS, Shukla SA, Wu CJ, Getz G, Hacohen N. Molecular and Genetic Properties of Tumors Associated With Local Immune Cytolytic Activity. Cell (2015) 160(1–2):48–61. doi: 10.1016/j.cell.2014.12.033
22. Ayers M, Lunceford J, Nebozhyn M, Murphy E, Loboda A, Kaufman DR, et al. IFN-γ-Related mRNA Profile Predicts Clinical Response to PD-1 Blockade. J Clin Invest (2017) 127(8):2930–40. doi: 10.1172/JCI91190
23. Jiang P, Gu S, Pan D, Fu J, Sahu A, Hu X, et al. Signatures of T Cell Dysfunction and Exclusion Predict Cancer Immunotherapy Response. Nat Med (2018) 24(10):1550–8. doi: 10.1038/s41591-018-0136-1
24. Györffy B, Lanczky A, Eklund AC, Denkert C, Budczies J, Li Q, et al. An Online Survival Analysis Tool to Rapidly Assess the Effect of 22,277 Genes on Breast Cancer Prognosis Using Microarray Data of 1,809 Patients. Breast Cancer Res Treat (2010) 123(3):725–31. doi: 10.1007/s10549-009-0674-9
25. Nagy Á, Lánczky A, Menyhárt O, Győrffy B. Validation of miRNA Prognostic Power in Hepatocellular Carcinoma Using Expression Data of Independent Datasets. Sci Rep (2018) 8(1):9227. doi: 10.1038/s41598-018-27521-y
26. Li B, Severson E, Pignon J-C, Zhao H, Li T, Novak J, et al. Comprehensive Analyses of Tumor Immunity: Implications for Cancer Immunotherapy. Genome Biol (2016) 17(1):174. doi: 10.1186/s13059-016-1028-7
27. Li T, Fan J, Wang B, Traugh N, Chen Q, Liu JS, et al. TIMER: A Web Server for Comprehensive Analysis of Tumor-Infiltrating Immune Cells. Cancer Res (2017) 77(21):e108–10. doi: 10.1158/0008-5472.CAN-17-0307
28. Lu J, Wang W, Xu M, Li Y, Chen C, Wang X. A Global View of Regulatory Networks in Lung Cancer: An Approach to Understand Homogeneity and Heterogeneity. Semin Cancer Biol (2017) 42:31–8. doi: 10.1016/j.semcancer.2016.11.004
29. Lin L, Lin D-C. Biological Significance of Tumor Heterogeneity in Esophageal Squamous Cell Carcinoma. Cancers (Basel) (2019) 11(8):1156. doi: 10.3390/cancers11081156
30. Delgado P, Fernández E, Dave V, Kappes D, Alarcón B. CD3delta Couples T-Cell Receptor Signalling to ERK Activation and Thymocyte Positive Selection. Nature (2000) 406(6794):426–30. doi: 10.1038/35019102
31. Pan Q, Gollapudi AS, Dave VP. Biochemical Evidence for the Presence of a Single CD3delta and CD3gamma Chain in the Surface T Cell Receptor/CD3 Complex. J Biol Chem (2004) 279(49):51068–74. doi: 10.1074/jbc.M406145200
32. Zuo Z, Xiong J, Zeng C, Jiang Y, Xiong K, Tao H, et al. Exploration of a Robust and Prognostic Immune Related Gene Signature for Cervical Squamous Cell Carcinoma. Front Mol Biosci (2021) 8:625470. doi: 10.3389/fmolb.2021.625470
33. Sanchez-Madrid F, Krensky AM, Ware CF, Robbins E, Strominger JL, Burakoff SJ, et al. Three Distinct Antigens Associated With Human T-Lymphocyte-Mediated Cytolysis: LFA-1, LFA-2, and LFA-3. Proc Natl Acad Sci USA (1982) 79(23):7489–93. doi: 10.1073/pnas.79.23.7489
34. Calabresi PA, Yun SH, Allie R, Whartenby KA. Chemokine Receptor Expression on MBP-Reactive T Cells: CXCR6 Is a Marker of IFNgamma-Producing Effector Cells. J Neuroimmunol (2002) 127(1–2):96–105. doi: 10.1016/S0165-5728(02)00106-6
Keywords: head and neck squamous cell carcinoma (HNSCC), human papillomavirus, transcriptome signature, immune gene signatures, cervical squamous cell carcinoma (CSCC)
Citation: Saiz-Ladera C, Baliu-Piqué M, Cimas FJ, Manzano A, García-Barberán V, Camarero SC, Hinojal GF, Pandiella A, Győrffy B, Stewart D, Cruz-Hernández JJ, Pérez-Segura P and Ocana A (2021) Transcriptomic Correlates of Immunologic Activation in Head and Neck and Cervical Cancer. Front. Oncol. 11:714550. doi: 10.3389/fonc.2021.714550
Received: 25 May 2021; Accepted: 13 September 2021;
Published: 06 October 2021.
Edited by:
Hui Wang, Hunan Cancer Hospital, ChinaCopyright © 2021 Saiz-Ladera, Baliu-Piqué, Cimas, Manzano, García-Barberán, Camarero, Hinojal, Pandiella, Győrffy, Stewart, Cruz-Hernández, Pérez-Segura and Ocana. This is an open-access article distributed under the terms of the Creative Commons Attribution License (CC BY). The use, distribution or reproduction in other forums is permitted, provided the original author(s) and the copyright owner(s) are credited and that the original publication in this journal is cited, in accordance with accepted academic practice. No use, distribution or reproduction is permitted which does not comply with these terms.
*Correspondence: Alberto Ocana, YWxiZXJ0by5vY2FuYUBzYWx1ZC5tYWRyaWQub3Jn
†These authors have contributed equally to this work and share first authorship