- 1Key Laboratory of Radiation Oncology of Taizhou, Radiation Oncology Institute of Enze Medical Health Academy, Department of Radiation Oncology, Taizhou Hospital Affiliated to Wenzhou Medical University, Taizhou, China
- 2Department of Radiology, The First Affiliated Hospital of Wenzhou Medical University, Wenzhou, China
- 3Department of Radiology, Taizhou Hospital of Zhejiang Province Affiliated to Wenzhou Medical University, Taizhou, China
- 4Department of Radiation Oncology, University of Arizona, Tucson, AZ, United States
- 5Department of Radiation Oncology, Indiana University School of Medicine, Indianapolis, IU, United States
Objective: We aimed to investigate whether enhanced CT-based radiomics can predict micropapillary pattern (MPP) of lung invasive adenocarcinoma (IAC) in the pre-op phase and to develop an individual diagnostic predictive model for MPP in IAC.
Methods: 170 patients who underwent complete resection for pathologically confirmed lung IAC were included in our study. Of these 121 were used as a training cohort and the other 49 as a test cohort. Clinical features and enhanced CT images were collected and assessed. Quantitative CT analysis was performed based on feature types including first order, shape, gray-level co-occurrence matrix-based, gray-level size zone matrix-based, gray-level run length matrix-based, gray-level dependence matrix-based, neighboring gray tone difference matrix-based features and transform types including Log, wavelet and local binary pattern. Receiver operating characteristic (ROC) and area under the curve (AUC) were used to value the ability to identify the lung IAC with MPP using these characteristics.
Results: Using quantitative CT analysis, one thousand three hundred and seventeen radiomics features were deciphered from R (https://www.r-project.org/). Then these radiomic features were decreased to 14 features after dimension reduction using the least absolute shrinkage and selection operator (LASSO) method in R. After correlation analysis, 5 key features were obtained and used as signatures for predicting MPP within IAC. The individualized prediction model which included age, smoking, family tumor history and radiomics signature had better identification (AUC=0.739) in comparison with the model consisting only of radiomics features (AUC=0.722). DeLong test showed that the difference in AUC between the two models was statistically significant (P<0.01). Compared with the simple radiomics model, the more comprehensive individual prediction model has better prediction performance.
Conclusion: The use of radiomics approach is of great value in the diagnosis of tumors by non-invasive means. The individualized prediction model in the study, when incorporated with age, smoking and radiomics signature, had effective predictive performance of lung IAC with MPP lesions. The combination of imaging features and clinical features can provide additional diagnostic value to identify the micropapillary pattern in IAC and can affect clinical diagnosis and treatment.
Introduction
Lung cancer is the most common cause of cancer-related death worldwide. Non-small cell lung cancer (NSCLC) accounts for approximately 85% of all cases of lung cancer, and with the most common histological subtype of NSCLC being adenocarcinoma (1). Currently, surgical resection is the main treatment for lung adenocarcinoma (2).
According to the 2015 World Health Organization Classification of Lung Cancer (3), based on prognosis, lung adenocarcinoma is classified into five histopathological subtypes. Micropapillary type is a high-risk subtype which has the potential for rapid metastasis and a poor prognosis (4, 5). Micropapillary pattern (MPP) refers to the free central cluster of cells lacking fibrous vessels (6). It has been reported in published literature that the micropapillary pattern of lung adenocarcinoma also has a negative effect on prognosis and survival after postoperative radiotherapy and chemotherapy (7, 8). According to Lee G et al., even a small proportion of MPP specifically <5% of the entire tumor volume has a significant negative prognostic impact on the overall survival (OS). Thus, the negative prognostic impact of MPP plays an important role in the clinical treatment plan and the early diagnosis of MPP in the lung IAC become very important (9).
Currently, the common preoperative diagnostic methods of lung cancer are histological puncture biopsy and CT imaging methods. However, histological biopsies are invasive and conventional CT imaging diagnostic methods are subjective and qualitative (10, 11). Moreover, most lung adenocarcinomas do not have high-grade components, and so radiographic features may not be obvious in identifying these cases (12–14).
Radiomics is a non-invasive and quantitative methods for describing the biological characteristics and heterogeneity of the tumor and we aimed to use this for the early diagnosis of pulmonary micropapillary lesions. Radiomics has become one of the research hotspots due to its high patient-specific and non-invasive comprehensive advantages. Possibly, radiomics has potential application for the precise treatment of NSCLC. Radiomics is expected to be used to judge biological characteristics of tumors such as benign or malignant pulmonary nodular, lymph node metastasis and tumor gene phenotype, to reflect tumor response to treatment, and to predict prognosis and radiation-induced lung injury and provide an objective basis for individualized treatment (11, 15, 16). This study is based on the features from CT imaging data over a given region of interest (ROI).
Previously, we investigated the diagnosis of micropapillary type of lung adenocarcinoma to some extent by radiomic methods (17) and the purpose of the current study is to further study the use of CT-based radiomics to preoperationally predict MPP in lung invasive adenocarcinoma.
Materials and Methods
Patients
A total of 170 patients between July 2014 and July 2020 were included in our study. 121 cases of single lung adenocarcinoma diagnosed by pathology were included as the training cohort, including 46 cases with MPP and 75 cases without MPP, aged 45-87 (67.7 ± 8.8) years old. Inclusion criteria: (1) no treatment before enhanced CT examination; (2) complete surgical resection with pathological confirmation; (3) complete DICOM data. Exclusion criteria: (1) clinical data were incomplete or could not be statistically analyzed; (2) preoperative chemotherapy or radiotherapy; (3) other malignant tumor or a history of malignant tumor; (4) radiomics analysis of CT images was not possible. In addition, forty-nine cases of single lung adenocarcinoma were collected and used as an independent testing cohort, including 22 cases with MPP and 27 cases without MPP, aged 26-85 (63.2 ± 12.1) years old. Inclusion criteria and exclusion criteria were the same as the training cohort. The flow chart is shown in Figure 1. The study is approved by the ethics committee of the author’s medical institution and informed consent was obtained.
Image Acquisition and Segmentation
Helical CT images were gained by a 16-row CT scanner (GE Ultra) after deep inhalations. The examination parameters were as follows: voltage, 120KV; tube current, 150mA; layer thick, 1.25mm; and matrix, 512×512mm. A power syringe was used to inject contrast agent (60-85ml iodioxitol or iodipamil) at a rate of 2.5-3.0ml/s, and rapid scanning was performed after a delay of 30 seconds to obtain arterial CT images for the study. Two radiologists read the images and sketched the ROI layer independently and blindly with each other using ITK-SNAP (www.itksnap.org/). Then radiomic features were extracted from those volumes for further analysis.
Clinical Data Collection
Clinical data were collected via electronic medical records. Clinical features were assessed at the time of diagnosis. Gender, age, smoking status, drinking status, family tumor history, UICC stage, type of surgery, and treatment history were recorded. Clinic characteristics are shown in Table 1.
Extraction of Radiomics Characteristics
Artificial Intelligent Kit (A.K., version V3.2.0. R) was used for image processing and feature extraction. The original DICOM images and the ROI files were separately resampled by the Image Preprocessing module of A.K., and the resampling voxel size was set as 1.000 × 1.000 × 1.000. Then the two types of enhanced CT images were matched to extract radiomics features which included features types and transform types by A.K. platform. The former types included first order, shape, gray-level co-occurrence matrix (GLCM), gray-level size zone matrix (GLSZM), gray-level run length matrix (GLRLM), gray-level dependence matrix (GLDM) and neighboring gray tone difference matrix (NGTDM), and the latter types included log, wavelet and local binary pattern (LBP).
Selection of Relevant Features and Establishment of Individualized Prediction Model
The least absolute shrinkage and selection operator (LASSO) was applied for dimensionality reduction screening to produce reproducible and stable features that would be used to screen for the predictive performance studies. And the “corrplot” package was used for correlation analysis (Supplementary Material). The cor-function of R software was used to further analyze the potential correlation between radiomics features and clinical features in the differentiation of MPP within lung adenocarcinoma. Finally, the most relevant features were selected and used to develop prediction models for MPP of lung IAC.
Statistical Method
R software (version 4.0.0, http://www.Rproject.org) was used for statistical analysis. The “glmnet” and “pROC” packages were used for LASSO reduction and ROC curve plotting. The LASSO method was used for features dimensionality reduction and the selection of the discriminative features. The cor-function was used to detect whether the differences of variables or indicators among different classifications and different sets were statistically significant. Intra-class correlation coefficient (ICC) values were compared to estimate the reproducibility and stability of tumor segmentation and feature extraction (ICC > 0.75 indicating good consistency). The receiver operating characteristic (ROC) curve and area under the curve (AUC) are used to evaluate the predictive effectiveness of models, and DeLong test was used to compare whether the efficiency differences between the models were statistically significant. Calibration curves were used to evaluate the correction effect of the nomograms.
Results
Extraction and Selection of Radiomics Features and the Establishment of Radiomics Signature
Data preprocessing was performed by R software. After deleting meaningless and empty data, 1317 radiomics features remained. Then, LASSO was used for dimension reduction of these features and the resulting lambda.1se=0.074 is obtained (Figures 2A, B). According to the results, 14 relevant radiomic features were selected. Then correlation analysis was conducted for the 14 features by the cor-function of R software and 5 key features were selected (Figure 3). They are referred to in this article as “Features 1-5”, as shown in Table 2.
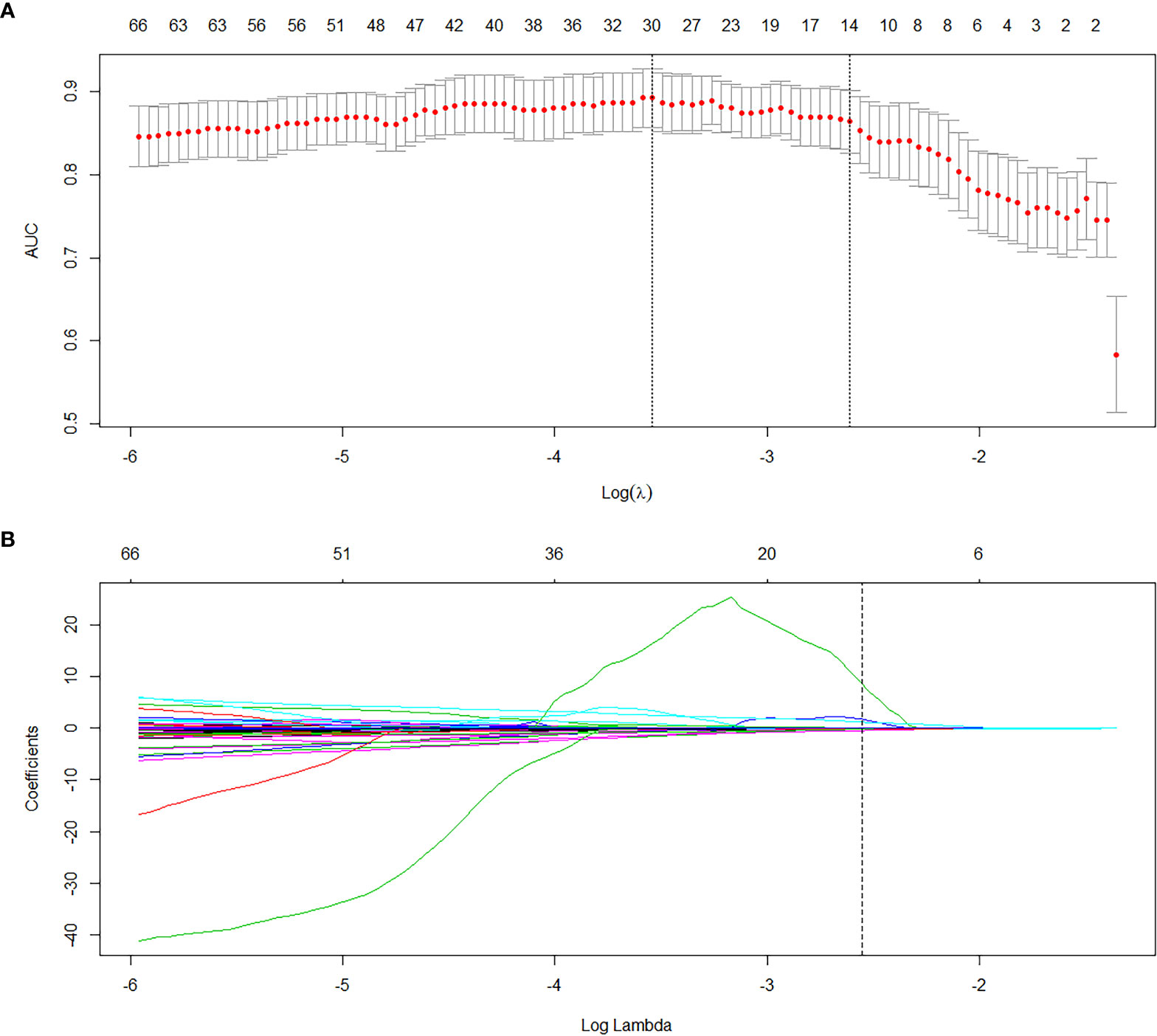
Figure 2 Dimension reduction to find 14 relevant radiomics features by LASSO. (A) The cross validation was performed by LASSO regression and the parameter λ was adjusted to find the best set of functions. The vertical dotted line on the left indicates that the logarithm (λ) corresponds to the optimal λ. The selection criterion is the minimum deviation value. (B) The texture parameter coefficients varied with λ. The vertical line represents the 14 features selected when the LASSO cross validation coefficients is non-zero. (λ=0.074).
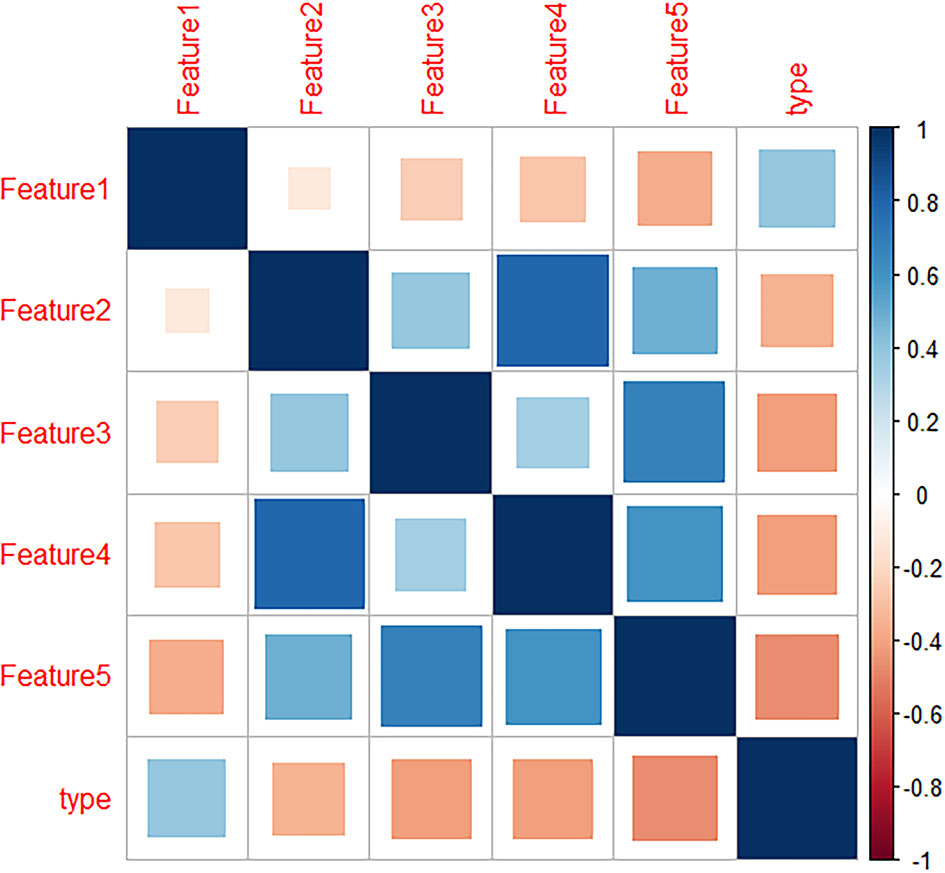
Figure 3 Heatmap of radiomics features which showed the correlation between the 5 radiomics features and lung invasive adenocarcinoma (IAC) with micropapillary pattern (MPP).
Predictive Efficacy of Radiomics Signature
The mean of each feature of the radiomics signature is shown in Table 2. Correlation test showed that the median difference of radiomics signatures between the lung IAC with MPP and lung IAC with non-MPP was statistically significant (P<0.05). As shown in Figure 5A, the efficacy of radiomics signature for differential diagnosis was good in the training cohort (AUC = 0.889, 95% CI: 0.843 – 0.944) and in the test cohort (AUC = 0.722, 95% CI: 0.574 – 0.870).
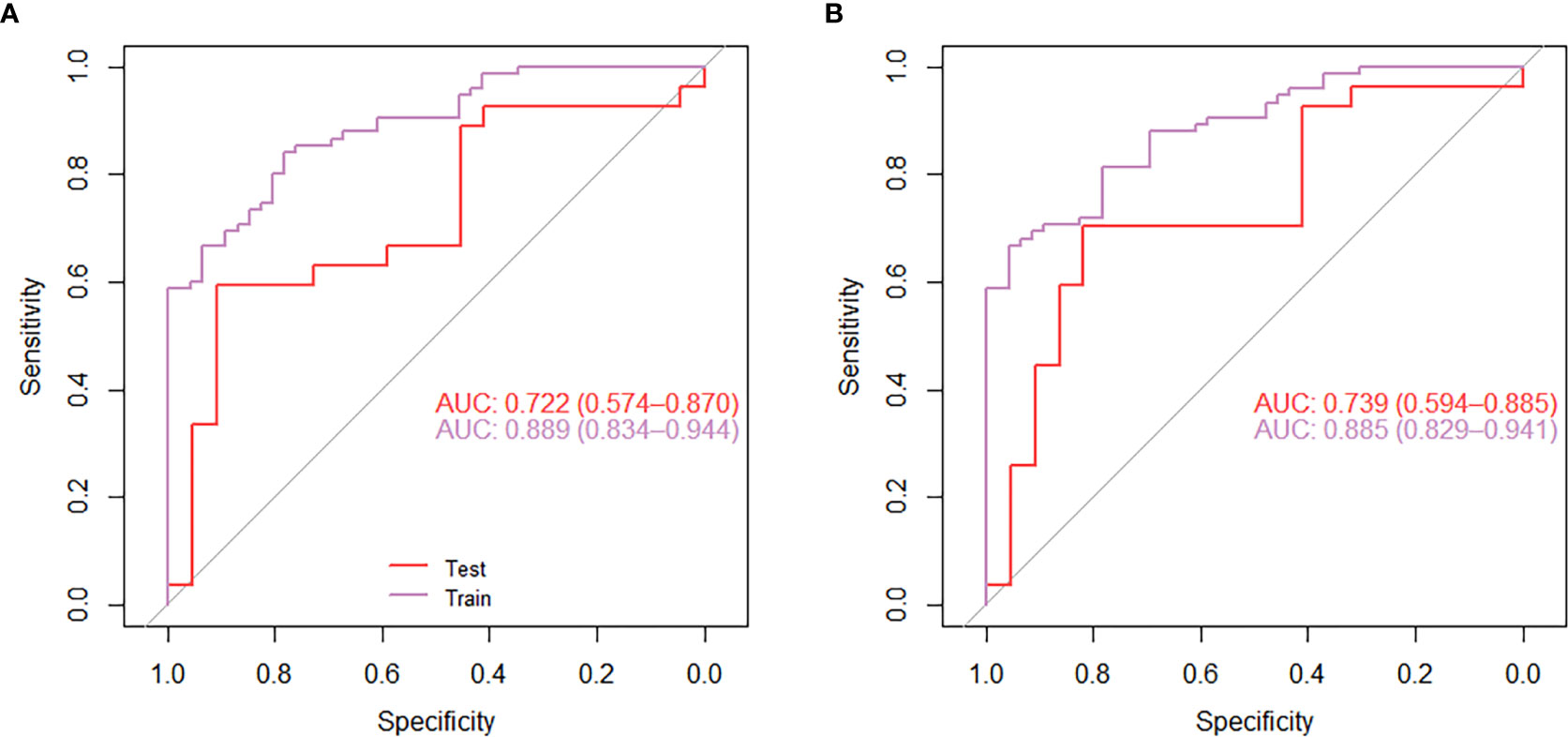
Figure 5 The receiver operating characteristic curve (ROC) analysis of the effect of the radiomics model nomogram (A) and the individual diagnostic prediction model nomogram (B) to predict micropapillary pattern within lung invasive adenocarcinoma.
Establishment of Individualized Diagnostic Prediction Model
Correlation analysis was conducted for clinical features including age, gender, smoking status, drinking status and family tumor history, which was the same as the radiomic features selection above. There was no statistically significant difference in gender and drinking between the lung IAC with MPP and lung IAC with non-MPP in the training cohort and the test cohort. These clinically significant features were combined with the previously selected radiomic features to establish a personalized diagnostic prediction model for lung IAC with MPP, which is shown by a nomogram Figure 4.
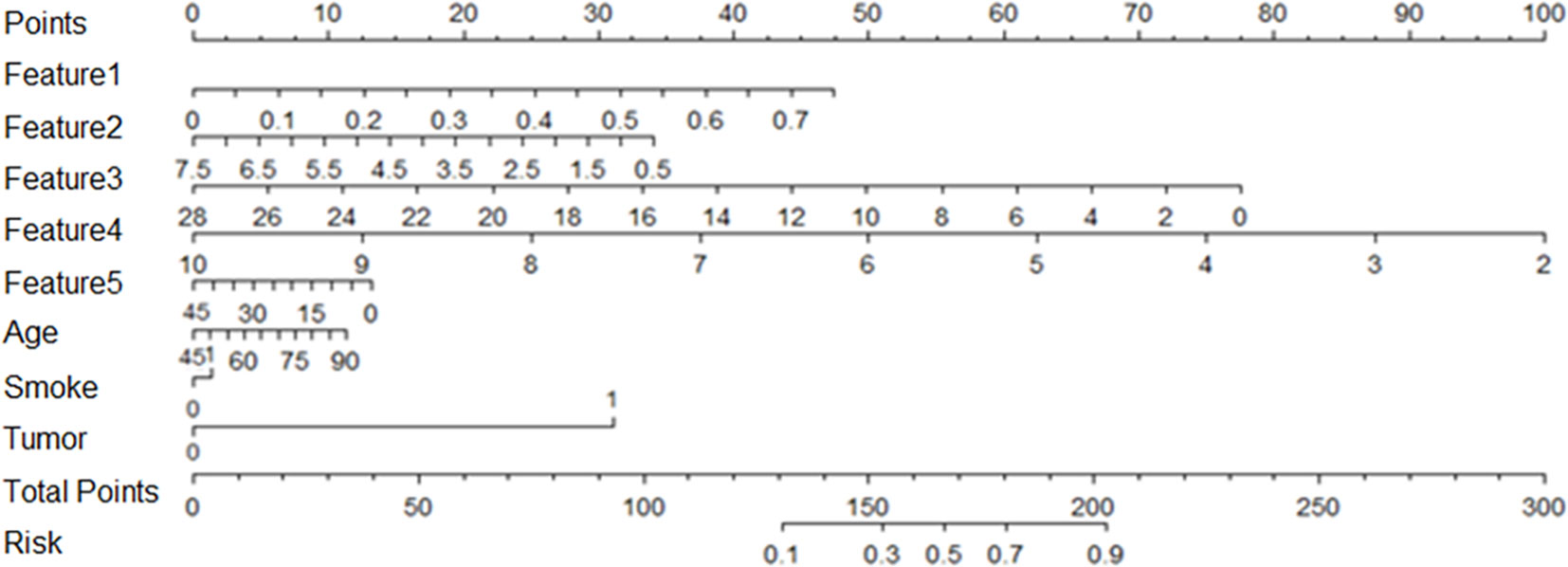
Figure 4 Based on the training cohort data, the nomogram of radiomics was established, and the statistical analysis showed that the nomogram consisted of radiomics signature, age, smoking and family tumor history, which could predict the risk of micropapillary pattern within lung invasive adenocarcinoma.
Comparison of Predictive Performance Between Radiomics Model and Individualized Diagnostic Model
For the individualized diagnostic model, the AUC in the training cohort was 0.885 (95% CI: 0.829 – 0.941), and in the test cohort, the AUC was 0.739 (95% CI: 0.594 – 0.885) (Figure 5B). In the test cohort, the diagnostic prediction effect of the individualized diagnostic model was relatively better than that of the radiomics model alone.
Discussion
Recently, there has been some reports that lung IACs with MPP are associated with poor prognosis, such as the possible propensity for recurrence and metastasis (11, 19). MPP is considered to be associated with a high rate of lymphatic invasion, visceral pleural invasion and lymph node metastasis as well (20–23). Previous studies have shown that patients with neoplasms with an MPP component of 5% or more have a higher recurrence rate after limited resection than similar patients with lobectomies (24), suggesting that MPP may influence the choice of surgical approach. Therefore, preoperatively diagnosing lung IAC with MPP is very important for the clinic.
CT is the most widely applied method to diagnose lung cancer, but it is subjective for doctors to judge the nature of lesions and the sensitivity and specificity of detecting lymph node metastasis is also low (25, 26). Radiomics provides an more objective method for diagnosis. Recent developments in radiomics have made it possible to extract high-throughput imaging features, making it easier to obtain more accurate information about individual patients and their treatment options. Many studies have developed models of different clinical features for prediction based on radiomics, including prognosis and the conditions of lymph node metastasis. In addition, some researchers believe that radiomics analysis is valuable in predicting the clinical characteristics and molecular background of brain tumors (27–32). Our study also shows that radiomic features can be applied to determinate lung adenocarcinoma with MPP from other lung IAC and it is helpful not only for follow-up therapy but also suggests that radiomic analyses could be used to guide the selection of therapy for specific tumors (27). Radiomics may have a bigger role in the future.
We have studied the diagnosis of micropapillary type of lung IAC by means of radiomics before (17), and this study is a supplement and extension on the basis of the previous research. In this study, we investigated radiomics features to differentiate lung IAC with MPP from lung IAC with non-MPP based on enhanced CT images. In our previous study, only radiomics signature based on CT images were obtained, and this study added a comprehensive model combining clinical features and a comparison between the two models. The results showed that the radiomics features of CT images may be used to identify micropapillary pattern of lung invasive adenocarcinoma, and that the predictive efficiency of the personalized diagnostic model combined with clinical features was better than that of the omics label alone.
The clinical sample selection of our study is closely linked to postoperative pathology, which shows the reliability of the research results. CT images were used validate the radiomics nomogram. Reliable imaging characteristics are necessary for radiomics analysis (28). Previous studies have selected certain 3D imaging features and put them into application (33, 34). They showed the visual representation of tumors (27, 28). To construct the radiomic features, the correlation of prediction results was detected by LASSO, and the large numbers of radiomic features were reduced to 14 potential predictors. This method is not only better than the method of selecting predictors based on the univariate association strength of predictors and results, but also can combine the selected feature panels for performing predictive function (30).
There are some limitations in our study. First, this was a single-center study with a lack of external validation. Secondly, the sample size needs to be expanded for more reliable results. Third, texture features were extracted from manually segmented data, which made it difficult to exclude small vessels and bronchus inside the lesions, and may affect the accuracy of some features, and the development of a more reliable and repeatable automated segmentation method seems necessary. Moreover, the current study did not include genomics studies. It has been reported that radiogenomics can offer a practical way to leverage limited and incomplete data to generate knowledge that may lead to improved decision making, and as a result, improve patient outcomes (35). Other studies have shown that EMT-related molecules, EGFR, KRAS and Ki67 have some relationship with MPP (36–38). In the future, we will combine the genetic characteristics for further research and exploration.
In conclusion, radiomics has an important role to play in the diagnosis of tumors. The individualized predictive model, which consists of clinical features and radiomic signatures, expressed in the form of a histogram, can provide a non-invasive, rapid, inexpensive, and reproducible method for the individualized preoperative prediction of micropapillary pattern within lung invasive adenocarcinoma to determine the presence of micropapillary components and facilitate clinical management.
Data Availability Statement
The original contributions presented in the study are included in the article/Supplementary Material. Further inquiries can be directed to the corresponding authors.
Ethics Statement
The studies involving human participants were reviewed and approved by the Ethics Committee of Taizhou Enze Medical Center (Group) Taizhou Enze Medical Center (Group). The patients/participants provided their written informed consent to participate in this study.
Author Contributions
YX, HY, and MW contributed to conception and design of the study. WJ, CZ, and WW organized the database. LH, SL, and YM performed the statistical analysis. YX wrote the first draft of the manuscript. YS and XC wrote sections of the manuscript. All authors contributed to the article and approved the submitted version.
Funding
This work was supported in part by National Natural Science Foundation of China (CN) (NSFC 81874221).
Conflict of Interest
The authors declare that the research was conducted in the absence of any commercial or financial relationships that could be construed as a potential conflict of interest.
Publisher’s Note
All claims expressed in this article are solely those of the authors and do not necessarily represent those of their affiliated organizations, or those of the publisher, the editors and the reviewers. Any product that may be evaluated in this article, or claim that may be made by its manufacturer, is not guaranteed or endorsed by the publisher.
Supplementary Material
The Supplementary Material for this article can be found online at: https://www.frontiersin.org/articles/10.3389/fonc.2021.704994/full#supplementary-material
References
1. Li YZ, Ren YJ, Chen C. Recent Advances on Invasive Mechanism of Pulmonary Adenocarcinoma With Micropapillary Pattern. Chin J Pathol (2018) 47(4):317–20. doi: 10.3760/cma.j.issn.0529-5807.2018.04.021
2. Ding H, Xia W, Zhang L, Mao Q, Cao B, Zhao Y, et al. CT-Based Deep Learning Model for Invasiveness Classification and Micropapillary Pattern Prediction Within Lung Adenocarcinoma. Front Oncol (2020) 10:1186. doi: 10.3389/fonc.2020.01186
3. Marx A, Chan JK, Coindre JM, Detterbeck F, Girard N, Harris NL. The 2015 World Health Organization Classification of Tumors of the Thymus: Continuity and Changes. J Thorac Oncol (2015) 10:1383–95. doi: 10.1097/JTO.0000000000000654
4. Zhou J, Liu B, Li Z, Li Y, Chen X, Ma Y, et al. Proteomic Analyses Identify Differentially Expressed Proteins and Pathways Between Low-Risk and High-Risk Subtypes of Early-Stage Lung Adenocarcinoma and Their Prognostic Impacts. Mol Cell Proteomics (2021) 20:100015. doi: 10.1074/MCP.RA120.002384
5. Yuan Y, Ma G, Zhang Y, Chen H. Presence of Micropapillary and Solid Patterns are Associated With Nodal Upstaging and Unfavorable Prognosis Among Patient With Ct1n0m0 Lung Adenocarcinoma: A Large-Scale Analysis. J Cancer Res Clin Oncol (2018) 144:743–9. doi: 10.1007/s00432-017-2571-7
6. Shi XY, Pang QS, Zhao G, Zhao L, Wang P. Advances of Pulmonary Adenocarcinoma With Micropapillary Pattern. Chin J Lung Cancer (2015) 18:701–5. doi: 10.3779/j.issn.1009-3419.2015.11.08
7. Zeng J, Cui X, Cheng L, Chen Y, Du X, Sheng L. Micropapillary Pattern of Stage IIIA-N Lung Adenocarcinoma Is a Prognostic Factor After Adjuvant Chemoradiotherapy. Future Oncol (2020) 16:3075–84. doi: 10.2217/fon-2020-0597
8. Qian F, Yang W, Wang R, Xu J, Wang S, Zhang Y, et al. Prognostic Significance and Adjuvant Chemotherapy Survival Benefits of a Solid or Micropapillary Pattern in Patients With Resected Stage IB Lung Adenocarcinoma. J Thorac Cardiovasc Surg (2018) 155:1227–35.e2. doi: 10.1016/j.jtcvs.2017.09.143
9. Lee G, Lee HY, Jeong JY, Han J, Cha MJ, Lee KS, et al. Clinical Impact of Minimal Micropapillary Pattern in Invasive Lung Adenocarcinoma: Prognostic Significance and Survival Outcomes. Am J Surg Pathol (2015) 39:660–6. doi: 10.1097/PAS.0000000000000399
10. Song Z, Liu Y. Progress of Liquid Biopsy in Early Diagnosis of Lung Cancer. Chin J Lung Cancer (2018) 21:620–7. doi: 10.3779/j.issn.1009-3419.2018.08.08
11. Song SH, Park H, Lee G, Lee HY, Sohn I, Kim HS, et al. Imaging Phenotyping Using Radiomics to Predict Micropapillary Pattern Within Lung Adenocarcinoma. J Thoracic Oncol (2017) 12(4):624–32. doi: 10.1016/j.jtho.2016.11.2230
12. Chen LW, Yang SM, Wang HJ, Chen YC, Lin MW, Hsieh HMS, et al. Prediction of Micropapillary and Solid Pattern in Lung Adenocarcinoma Using Radiomic Values Extracted From Near-Pure Histopathological Subtypes. Eur Radiol (2021) 31:5127–38. doi: 10.1007/s00330-020-07570-6
13. Lederlin M, Puderbach M, Muley T, Schnabel PA, Stenzinger A, Kauczor HU, et al. Correlation of Radio- and Histomorphological Pattern of Pulmonary Adenocarcinoma. Eur Respir J (2013) 41:943–51. doi: 10.1183/09031936.00056612
14. Lee H-J, Kim YT, Kang CH, Zhao B, Tan Y, Schwartz LH, et al. Epidermal Growth Factor Receptor Mutation in Lung Adenocarcinomas: Relationship With CT Characteristics and Histologic Subtypes. Radiology (2013) 268:254–64. doi: 10.1148/radiol.13112553
15. Fave X, Zhang L, Yang J, Mackin D, Balter P, Gomez D, et al. Delta-Radiomics Features for the Prediction of Patient Outcomes in Non–Small Cell Lung Cancer. Sci Rep (2017) 7:588. doi: 10.1038/s41598-017-00665-z
16. Jia TY, Yu W, Fu XL. Progress on Application of Radiomics in Precise Treatment of Non-Small Cell Lung Cancer. Chin J Radiol Med Prot (2016) 36:947–50. doi: 10.3760/cma.j.issn.0254-5098.2016.12.015
17. Xu Y, Ji W, Hou L, Zhou C, Wang W, Zhou S, et al. Discrimination of Lung Invasive Adenocarcinoma With Micropapillary Pattern Based on CT Radiomics. J Thorac Oncol (2019) 14(10):S222. doi: 10.1016/j.jtho.2019.08.440
18. Goldstraw P, Chansky K, Crowley J, Rami-Porta R, Asamura H, Eberhardt WE. The IASLC Lung Cancer Staging Project: Proposals for Revision of the TNM Stage Groupings in the Forthcoming (Eighth) Edition of the TNM Classification for Lung Cancer. J Thorac Oncol (2016) 11(1):39–51. doi: 10.1016/j.jtho.2015.09.009
19. Cha MJ, Lee HY, Lee KS, Jeong JY, Han J, Shim YM, et al. Micropapillary and Solid Subtypes of Invasive Lung Adenocarcinoma: Clinical Predictors of Histopathology and Outcome. J Thorac Cardiovasc Surg (2014) 147:921–8.e2. doi: 10.1016/j.jtcvs.2013.09.045
20. Zhang J, Liang Z, Gao J, Luo Y, Liu T. Pulmonary Adenocarcinoma With a Micropapillary Pattern: A Clinicopathological, Immunophenotypic and Molecular Analysis. Histopathology (2011) 59:1204–14. doi: 10.1111/j.1365-2559.2011.04050.x
21. Amin MB, Tamboli P, Merchant SH, Ordóñez NG, Ro J, Ayala AG, et al. Micropapillary Component in Lung Adenocarcinoma: A Distinctive Histologic Feature With Possible Prognostic Significance. Am J Surg Pathol (2002) 26:358–64. doi: 10.1097/00000478-200203000-00010
22. Hung JJ, Yeh YC, Jeng WJ, Wu KJ, Huang BS, Wu YC, et al. Predictive Value of the International Association for the Study of Lung Cancer/American Thoracic Society/European Respiratory Society Classification of Lung Adenocarcinoma in Tumor Recurrence and Patient Survival. J Clin Oncol (2014) 32:2357–64. doi: 10.1200/JCO.2013.50.1049
23. Miyoshi T, Satoh Y, Okumura S, Nakagawa K, Shirakusa T, Tsuchiya E, et al. Early-Stage Lung Adenocarcinomas With a Micropapillary Pattern, a Distinct Pathologic Marker for a Significantly Poor Prognosis. Am J Surg Pathol (2003) 27:101–9. doi: 10.1097/00000478-200301000-00011
24. Nitadori J, Bograd AJ, Kadota K, Sima CS, Rizk NP, Morales EA, et al. Impact of Micropapillary Histologic Subtype in Selecting Limited Resection vs Lobectomy for Lung Adenocarcinoma of 2 Cm or Smaller. J Natl Cancer Inst (2013) 105:1212–20. doi: 10.1093/jnci/djt166
25. Liam CK, Andarini S, Lee P, Ho CM, Chau NQ, Tscheikuna J. Lung Cancer Staging Nowand in the Future. Respirology (2015) 20:526–34. doi: 10.1111/resp.12489
26. Tsim S, O’Dowd CA, Milroy R, Davidson S. Staging of Non-Small Celllung Cancer (NSCLC): A Review. Respir Med (2010) 104:1767–74. doi: 10.1016/j.rmed.2010.08.005
27. Gillies RJ, Kinahan PE, Hricak H. Radiomics: Images are More Than Pictures, They are Data. Radiology (2016) 278:563–77. doi: 10.1148/radiol.2015151169
28. Liu Z, Wang Y, Liu X, Du Y, Tang Z, Wang K, et al. Radiomics Analysis Allows for Precise Prediction of Epilepsy in Patients With Low-Grade Gliomas. NeuroImage Clin (2018) 19:271–8. doi: 10.1016/j.nicl.2018.04.024
29. Lambin P, van Stiphout RG, Starmans MH, Rios-Velazquez E, Nalbantov G, Aerts HJ, et al. Predicting Outcomes in Radiation Oncology– Multifactorial Decision Support Systems. Nat Rev Clin Oncol (2013) 10:27–40. doi: 10.1038/nrclinonc.2012.196
30. Huang YQ, Liang CH, He L, Tian J, Liang CS, Chen X, et al. Development and Validation of a Radiomics Nomogram for Preoperative Prediction Oflymph Node Metastasis in Colorectal Cancer. J Clin Oncol (2016) 34:2157–64. doi: 10.1200/JCO.2015.65.9128
31. Nie K, Shi L, Chen Q, Hu X, Jabbour S, Yue N, et al. Rectal Cancer: Assessment of Neoadjuvant Chemoradiation Outcome Based on Radiomics of Multiparametric MRI. Clin Cancer Res (2016) 22:5256–64. doi: 10.1158/1078-0432.CCR-15-2997
32. Gevaert O, Mitchell LA, Achrol AS, Xu J, Echegaray S, Steinberg GK, et al. Glioblastoma Multiforme: Exploratory Radiogenomic Analysis by Using Quantitative Image Features. Radiology (2015) 276:313. doi: 10.1148/radiol.2015154019
33. Itakura H, Achrol AS, Mitchell LA, Loya JJ, Liu T, Westbroek EM, et al. Magnetic Resonance Image Features Identify Glioblastoma Phenotypic Subtypes With Distinct Molecular Pathway Activities. Sci Transl Med (2015) 7:303ra138. doi: 10.1126/scitranslmed.aaa7582
34. Aerts HJ, Velazquez ER, Leijenaar RT, Parmar C, Grossmann P, Carvalho S, et al. Decoding Tumour Phenotype by Noninvasive Imaging Using a Quantitative Radiomics Approach. Nat Commun (2014) 5:4006. doi: 10.1038/ncomms5006
35. Mazurowski MA. Radiogenomics: What It Is and Why It Is Important. J Am Coll Radiol (2015) 12:862–6. doi: 10.1016/j.jacr.2015.04.019
36. Zhou JH, Xu LY, Lu CC, Huang YY, Wang XC, Le HB. Features of CT Reconstruction Images and Expressions of Epithelia-Mesenchymal Transition Related Genes in Lung Adenocarcinoma With Micropapillary Pattern. Acta Anatomica Sin (2019) 1:63–71. doi: 10.16098/j.issn.0529-1356.2019.01.011
37. Wang J, Sun LN, Zhan ZL. Correlation of Pulmonary Adenocarcinoma With Micropapillary Pattern With EGFR and KRAS Mutation and Their Clinicopathological Features. Chin J Clin Oncol (2013) 40:89–92. doi: 10.3969/j.issn.1000-8179.2013.02.007
Keywords: lung adenocarcinoma, micropapillary pattern, radiomics, early diagnosis of cancer, computer tomography
Citation: Xu Y, Ji W, Hou L, Lin S, Shi Y, Zhou C, Meng Y, Wang W, Chen X, Wang M and Yang H (2021) Enhanced CT-Based Radiomics to Predict Micropapillary Pattern Within Lung Invasive Adenocarcinoma. Front. Oncol. 11:704994. doi: 10.3389/fonc.2021.704994
Received: 04 May 2021; Accepted: 09 August 2021;
Published: 27 August 2021.
Edited by:
Long Jiang Zhang, Nanjing General Hospital of Nanjing Military Command, ChinaReviewed by:
Nesrin Dogan, University of Miami, United StatesZhongxiang Ding, Zhejiang University, China
Copyright © 2021 Xu, Ji, Hou, Lin, Shi, Zhou, Meng, Wang, Chen, Wang and Yang. This is an open-access article distributed under the terms of the Creative Commons Attribution License (CC BY). The use, distribution or reproduction in other forums is permitted, provided the original author(s) and the copyright owner(s) are credited and that the original publication in this journal is cited, in accordance with accepted academic practice. No use, distribution or reproduction is permitted which does not comply with these terms.
*Correspondence: Haihua Yang, yhh93181@hotmail.com; Meihao Wang, wzwmh@wmu.edu.cn
†These authors have contributed equally to this work