- 1Pensylvania Cancer and Regenerative Medicine Research Center, Baruch S. Blumberg Institute, Wynnewood, PA, United States
- 2Xavier University School of Medicine at Aruba, Oranjestad, Aruba
- 3Program in Cardiovascular Medicine, Lankenau Institute for Medical Research, Wynnewood, PA, United States
- 4Medical School, Faculty of Health and Medical Sciences, The University of Western Australia, Crawley, WA, Australia
- 5Cancer Center, Wistar Institute, Philadelphia, PA, United States
Reprogramming of metabolic priorities promotes tumor progression. Our understanding of the Warburg effect, based on studies of cultured cancer cells, has evolved to a more complex understanding of tumor metabolism within an ecosystem that provides and catabolizes diverse nutrients provided by the local tumor microenvironment. Recent studies have illustrated that heterogeneous metabolic changes occur at the level of tumor type, tumor subtype, within the tumor itself, and within the tumor microenvironment. Thus, altered metabolism occurs in cancer cells and in the tumor microenvironment (fibroblasts, immune cells and fat cells). Herein we describe how these growth advantages are obtained through either “convergent” genetic changes, in which common metabolic properties are induced as a final common pathway induced by diverse oncogene factors, or “divergent” genetic changes, in which distinct factors lead to subtype-selective phenotypes and thereby tumor heterogeneity. Metabolic heterogeneity allows subtyping of cancers and further metabolic heterogeneity occurs within the same tumor mass thought of as “microenvironmental metabolic nesting”. Furthermore, recent findings show that mutations of metabolic genes arise in the majority of tumors providing an opportunity for the development of more robust metabolic models of an individual patient’s tumor. The focus of this review is on the mechanisms governing this metabolic heterogeneity in breast cancer.
Introduction
Breast Cancer. Breast cancer is the most common non-dermatological malignancy in women representing approximately one third of all malignancies diagnosed in US women (1, 2). In approximately 10% of cases breast cancers are associated with gene mutations inherited from one relative. Almost 50% of breast cancer cases occur in less developed countries with incidence rates ranging from 19 per 100,000 women in Eastern Africa to 90 per 100,000 women in Western Europe. Efforts to provide more precise therapies to patients with breast cancer has led to subclassification using the coding genome, the non-coding genome or more recently, metabolic subtypes.
Precision medicine approaches have identified genetic subtypes of BCa based initially on the coding genome (3). At least five distinct coding genome molecular subtypes are recognized including luminal A, luminal B, human epidermal growth factor receptor 2 (HER2)-enriched, basal-like, and claudin-low and normal-like (4, 5). Triple negative breast cancer (TNBC), which lacks estrogen receptor-α (ERα), progesterone receptor (PR) and HER2, characteristically includes mutations of DNA damage repair (6), altered PD-L1 expression (7) and increased expression of the G protein coupled receptor CCR5 (8, 9). Breast cancer has also been characterized based on the non-coding genome (10, 11). Altered expression of miRNA was observed in breast cancer (12). In subsequent studies hierarchical clustering of human breast cancers defined four distinct miRNA clusters (G1-G4) associated with distinguishable relapse-free survival by Kaplan-Meier analysis (10). These studies defined a cyclin D1-regulated miRNA signature which included several oncomirs, that was conserved in multiple breast cancer cell lines, and was associated with the G2 tumor miRNA cluster, ERα+ status, better outcome and activation of the Wnt pathway (10). Of interest these studies showed that the coding and non-coding genome for any given tumor were discordant within breast cancer subtypes. In recent studies metabolic subtyping of breast cancer has revealed distinguishable characteristics. Triple negative breast cancer (TNBC), for example, expresses low levels of glutamine synthetase (GLUL, glutamate-ammonia ligase). Withdrawing glutamine suppresses growth of the basal and claudin low triple negative tumors BCa tumor subtype (13). In contrast, luminal tumor cells express GLUL and are resistant to glutamine deprivation (14). The glutaminase isozyme GLS2, is upregulated and essential in luminal-subtype breast tumors (15).
Currently, therapies for breast cancer rely on surgical, radiotherapeutic, chemotherapeutic and biological therapeutic approaches (16). Despite these advances in medicine, 30% of patients relapse and develop a metastatic cancer (17). Patients with triple negative breast cancer (ERα Negative, HER2 Negative, PR Negative) have a poor outcome and require additional therapy. Historically targeting of the coding genome has improved mortality rates in breast cancer patients, and in vitro pre-clinical studies have shown promise in targeting the non-coding genome (18–20). The identification of metabolic dependencies specific to the cancer vs. normal cells therefore represents an important new opportunity for therapeutic intervention. In this regard the xCT antiporter, which is expressed on 1/3 of triple negative tumors in vivo, is essential to support environmental cystine acquisition (13). Inhibition of the xCT antiporter with the anti-inflammatory Sulfasalazine decreased tumor growth (13). Targeting GLS1/GLS2 with the small-molecule inhibitor 968 reduced tumor growth in luminal breast cancer (15).
Tumor metabolism. The abnormalities associated with tumor metabolism have been recently reviewed (21–23). In contrast to normal differentiated cells, which rely primarily on mitochondrial oxidative phosphorylation to generate the energy needed for cellular processes, most cancer cells instead rely substantially on cytosolic aerobic glycolysis, a phenomenon termed “the Warburg effect” (Figure 1) (24, 25). In Warburg’s view, so central were metabolic changes to the cancer phenotype that he opined, “From this point of view, mutation and carcinogenic agent are not alternatives, but empty words, unless metabolically specified” (24).
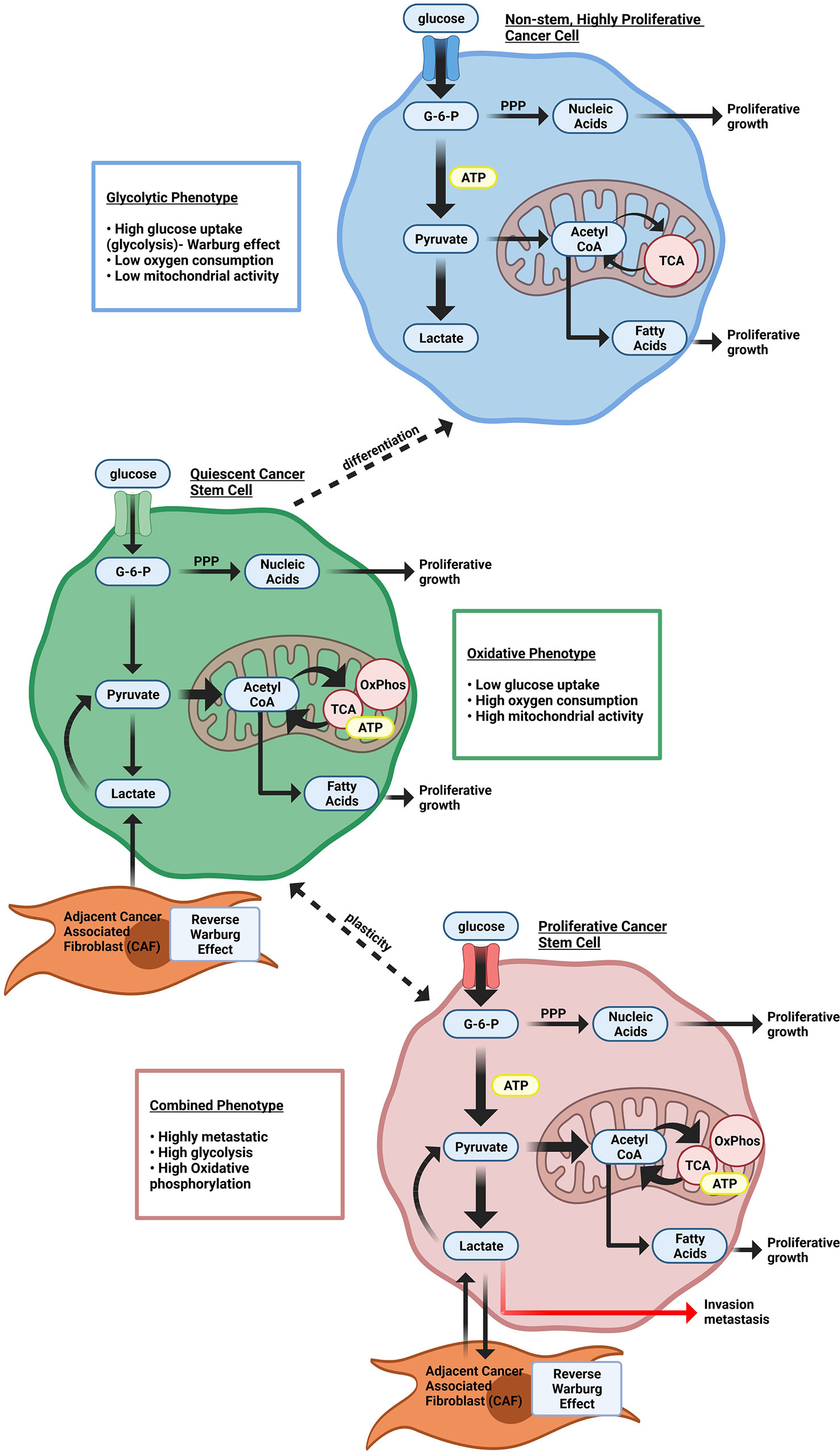
Figure 1 Heterogeneous cellular energy metabolism within breast cancer cells. Highly proliferating breast cancer cells rely on glycolysis to provide cellular energy. Quiescent breast cancer stem cells use more oxidative phosphorylation (OxPhos) instead of glycolysis to generate ATP. Proliferating cancer stem cells rely on both glycolysis and Oxphos. Both quiescent and proliferating cancer stem cells can use catabolites from cancer-associated stromal cells through the Reverse Warburg effect.
As the analytical tools used to interrogate the characteristics of tumor metabolism have evolved, it has become increasingly clear that metabolic adaptations of tumors are highly heterogeneous (26–30). Historically the understanding of cancer metabolism was drawn from principles of “convergent” metabolic phenotypes, properties that are governed by diverse factors shared among diverse tumor types. As the resolution of investigative tools has evolved, evidence for “divergent” metabolic pathways has provided compelling evidence for breast cancer metabolic heterogeneity.
Convergent properties include the principal that cancer cells have evolved multiple distinct mechanisms in order to provide metabolic substrates for proliferation within the tumor microenvironment (25). Like all living cells cancer cells need ATP, together with carbon intermediates for the synthesis of DNA, proteins and lipids. Cancer cells augment the procurement of nutrients, scavenge nutrients from alternative sources (alternative substrates), reprogram metabolic process needed for growth, and upregulate the apparatus for processing the nutrients into energy and structural intermediates for replication, growth, and invasion. Cancer-associated metabolic changes have been usefully categorized as: (i) deregulated uptake of glucose and amino acids, (ii) opportunistic nutrient acquisition from both intracellular and environmental sources, (iii) use of glycolysis/TCA cycle intermediates for biosynthesis and NADPH production, (iv) increased demand for nitrogen and means to satisfy it, (v) alterations in metabolite-driven gene regulation, and (vi) metabolic interactions with the tumor microenvironment. Tumors vary in the degree to which they deploy these individual changes (31). Thus, in addition to changes in glucose uptake, increased levels of methionine, glutamine, cystine, tryptophan, tyrosine, and other amino acids have been noted in breast cancer (32–36). Cancer cells with upregulation of amino acid metabolism stimulate increased transport of amino acids into the cell. The increased consumption of amino acids and overexpression of amino acid transporters (L-type amino acid transporter 1) during breast cancer progression, has led to an interest in radiolabeled amino acids imaging agents (37).
“Divergent” properties derive from distinct genetic or epigenetic alterations with a tumor which govern distinct molecular subsets of genes that in turn alter cellular metabolism thereby contributing to metabolic heterogeneity. Distinct oncogenotypes have been characterized in a variety of cancers. IDH1 and IDH2 mutations give rise to accumulation of (R)-2HG (38, 39). In lung cancer distinct somatic mutations (TP53, KRAS, BRAF, NF1, EGFR, KEAP1) appear to be each sufficient to regulate tumor metabolism (26–28). Although less well characterized in breast cancer, genetic alterations that occur in breast cancer (cyclin D1 overexpression (40, 41) and epigenetic changes [FBP1 (42), the Jumonji-domain histone demethylase (JHDM)3C (43)], are sufficient to induces metabolic changes reflected by the Warburg effect.
“Convergent” Genetic Properties Driving The Warburg Effect
Warburg observed that cancer cells primarily supply energy from glucose through avid glycolysis, even in aerobic conditions where more efficient mitochondrial oxidative phosphorylation (OXPHOS) was potentially available. Per molecule of glucose, glycolysis followed by OXPHOS generates up to 18 times more adenosine 5´-triphosphate (ATP) than glycolysis alone (44). The Warburg effect is pervasive among cancer cells of many but not all cancer types, For example the Warburg effect is not prominent in early prostate cancer (45, 46) and is found in only one of the metabolic subtypes of glioblastoma multiforme (21). Furthermore, the Warburg effects occurs in a heterogeneous manner within tumors, generating an intratumoral “nesting phenomenon”. Aerobic cells proliferate best when they are clustered with some glycolytic cells (44, 47, 48).
Several convergent genetic processes further drive the Warburg effect in tumors. Oncogenic mutations, tumor suppressor deletions and overexpression of collaborative oncogenes contributes to the tumor metabolic shift as these genes govern expression of glycolytic enzymes. In this regard c-myc, k-Ras, mutant p53, cyclin D1 and the β-catenin/TCF signaling pathway augment the Warburg effect (41, 49–52). Hypoxia-inducible factor-1α (HIF1α), which normally contributes to regulation of glycolysis in hypoxia, is also more protected from degradation in some cancer types.
A transition towards aerobic glycolysis is available to normal cells during proliferation (53). In tumor cells that activate the Warburg effect, several metabolic consequences occur. Cancer cells accelerate aerobic glycolysis partly through regulatory processes that are general to proliferating cells (54). Normally, glycolysis proceeds at a rate that reflects negative feedback control of intracellular ATP and NAD+/NADH homeostasis. There may be an advantage for an energetically active cancer cell in the speed of ATP production in glycolysis. ATP can be rapidly synthesized by glycolysis, up to 100 times faster than OXPHOs (55). Cancer cells, with diminished OXPHOS-generated ATP and efficient export of NADH reducing equivalents as lactate, maintain a permissive intracellular environment for dysregulated glycolysis. The diversion of pyruvate away from acetyl CoA production to lactate, simultaneously regenerates NAD+ and deprives the mitochondrial electron transport chain of NADH for ATP synthesis.
Aerobic glycolysis also confers an advantage to cancer cells by generation of macromolecules to increase the cellular biomass (53). Rapid proliferation of cancer cells needs to be sustained by increased macromolecular biosynthesis. As well as its role in providing substrates for mitochondrial OXPHOS, glycolysis intermediates supply the pentose phosphate pathway, support NADPH generation, contribute one-carbon species into the one-carbon cycle and make acetyl CoA available for lipid synthesis (53). Glucose-6-phosphate dehydrogenase action on the first product of glycolysis, glucose-6-phosphate, initiates the pentose phosphate pathway. Products of the pentose phosphate pathway include ribose-5-phosphate for nucleotide synthesis and NADPH. NADPH, an essential intracellular reductant that is consumed in numerous lipid, amino acid and nucleotide anabolic pathways, is created in both the pentose phosphate pathway and in one-carbon cycle reactions (56).
Later steps in glycolysis yield fructose-6-phosphate, which may proceed to hexosamine synthesis and dihydroacetone phosphate, which is a substrate for glycerol-3-phosphate, and thence lipid, synthesis. Enhanced expression of 3-phosphoglycerate dehydrogenase has been described in breast cancer cells (57). In glycolysis, 3-phosphoglycerate is diverted out of the pathway under the action of 3-phosphoglycerate dehydrogenase. This is a quantitatively important source of substrates for the one-carbon cycle, enabling production of glycine, serine and thence S-adenosylmethionine and one-carbon derivatives of tetrahydrofolic acid (58). These are essential for the synthesis of purine and pyrimidine bases for nucleotides and many other biosynthetic processes. The glycolysis product, pyruvate, is available for acetyl CoA generation and thereby lipogenesis (53, 59).
Increased lactate production from aerobic glycolysis leading to cellular acidification and results in lactate efflux from cells to maintain cellular pH. Lactate efflux results in an acidic extracellular tumor microenvironment, which promotes angiogenesis (60) increasing HIF1α stabilization, promoting VEGF production from cancer associated macrophages (61), enhancing hyaluronic acid production from fibroblasts (62), inducing extracellular matrix degrading cathepsins and matrix metaloproteases (63, 64), and augmenting endothelial cell PI3K and NFκb signaling (65) thereby promoting vasculogenesis.
Epigenetic Changes in Tumors that Promote the Warburg Effect
Epigenetic modifications in breast cancer cells can alter metabolism in a particular tumor, thereby contributing to divergent heterogeneous metabolic changes. Epigenetic changes, which are inheritable, reversible changes in selective gene expression that occur without any alterations to the DNA sequence itself, include DNA methylation, histone modifications, and RNA-mediated gene silencing by non-coding RNAs such as miRNA. DNA methylation has been described of specific key components in glycolytic pathways, glycolysis bypass pathways (i.e. gluconeogenesis and pentose phosphate pathway), as well as mitochondrial and oxygen sensing pathways (66). Furthermore many of the intermediates of cellular metabolic pathways participate in the chemical modifications that epigenetically modify DNA and histones (67). A synopsis of these epigenetic changes that have been described in tumors, shown in Figure 2, underscore the growing evidence for heterogeneous drivers of altered tumor metabolism. In addition to epigenetic changes within the tumors, epigenetic changes also occur in the tumor stroma. Distinct epigenetic alterations occur in epithelial and myoepithelial cells, and stromal fibroblasts occur in a tumor stage- and cell type-specific manner (68). Based on unsupervised analysis three methylation patterns of breast cancer (luminal A, luminal B and basal-like molecular subtypes) were identified, whereas HER2-enriched and normal-like subtypes were distributed among the three groups (69). The luminal B were most methylated and basal-like tumors least frequently methylated. BRCA2-mutated tumors were highly methylated. A large fraction of genes reported as having subtype-specific expression patterns might be regulated through methylation (69).
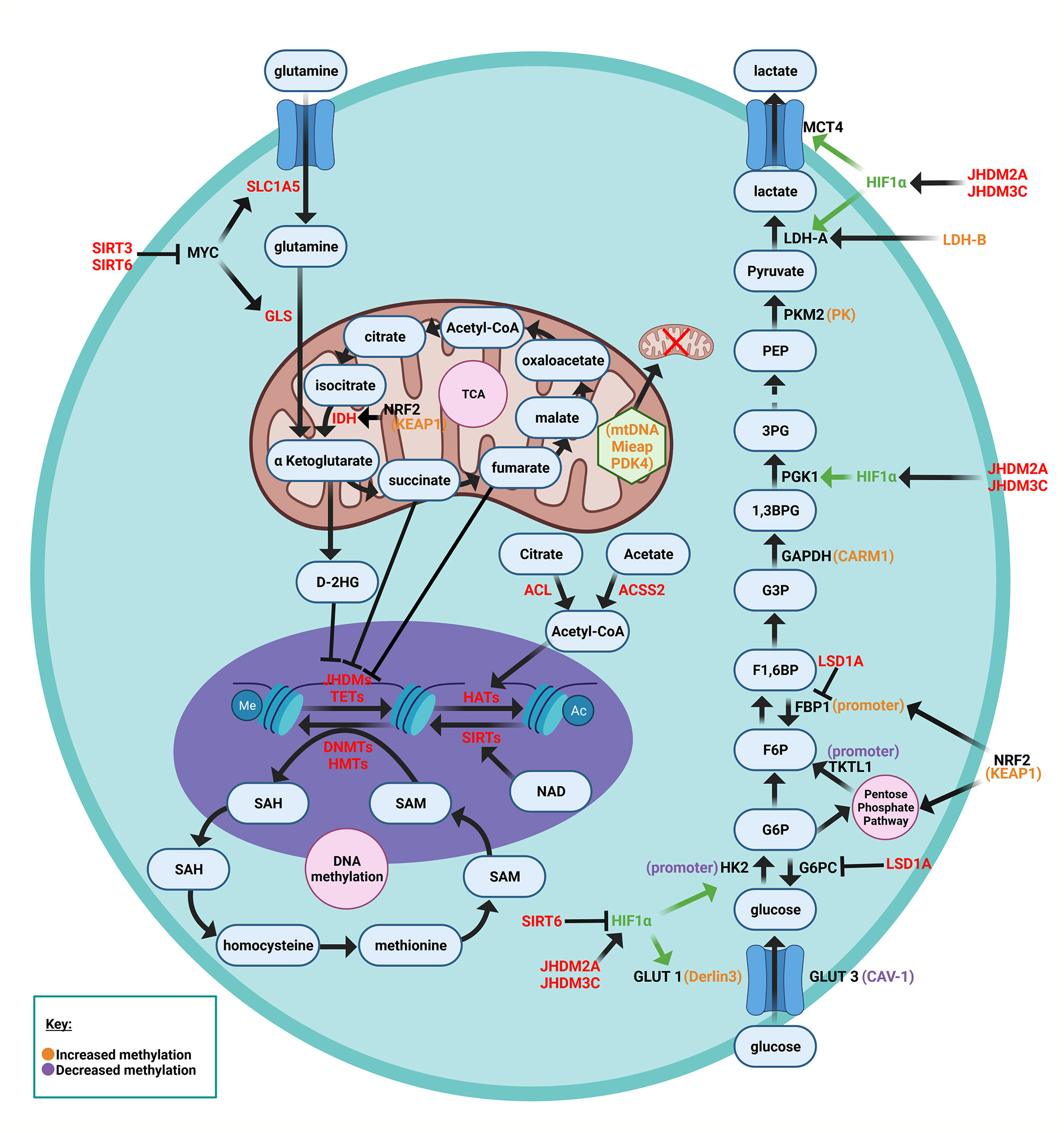
Figure 2 Schematic representation of metabolic nodes governed by epigenetic modification. Metabolic enzymes and nutrient transporters are governed by modification by DNA methylation and histone modifications. Bidirectional feedback occurs as the metabolic substrates generated in turn regulate epigenetic modifications. α-ketoglutarate is a co-substrate for JHDM (The Jumonji-domain histone demethylase) histone demethylase and the TET family methylcytosine dioxygenases, thereby governing demethylation of histone and other proteins, and DNA. Fumarate, succinate and 2-HG compete with a ketoglutarate. HIF1α (hypoxia inducible factor 1α), KEAP1 (Kelch-like ECH-associated protein 1), NRF2 (nuclear factor erythroid 2-related factor 2), FBP1 (fructose-1,6-bisphosphate isoform 1), TKTL1 (transketolase (TKT) like-1 gene), SAM (S-adenosyl methionine), TCA (tricarboxylic acid), The abundance of GLUT3 and GLUT1 are regulated by CAV-1 and Derlin3 respectively.
Epigenetic Modification of the Tumor
Within the tumor, DNA methylation affects key glycolytic components, including glucose transporters (GLUT1, GLUT3), lactate dehydrogenase genes (LDH-A, LDH-B), the hexokinase 2 isoform (HK2), glyceraldehyde-3-phosphate dehydrogenase (GAPDH), and the pyruvate kinase (PK) isoform M2 (PKM2), each of which contribute to the Warburg effect (66). DNA hypermethylation-mediated inactivation of the Derlin-3 gene, which normally contributes to GLUT1 degradation, leads to increased GLUT1 expression (70). Increased GLUT3 expression is regulated by methylation (71). DNA hypermethylation of the LDH-B gene in breast cancer (which interconverts lactate and pyruvate), increases the LDH-A to LDH-B ratio (72). Increased LDH-A mediated conversion of pyruvate into lactate promotes aerobic glycolysis (73). LDH-A activity is crucial to the Warburg effect since it oxidizes NADH and regenerates NAD+, without which aerobic glycolysis could not continue (74). Aerobic glycolysis is induced by upregulation of the hexokinase isoform HK2 via hypomethylation of its promoter (75) and hypomethylation within intron 1 of the pyruvate kinase (PK gene) (76). GAPDH upregulation, via coactivator-associated arginine methyltransferase 1 (CARM1)-mediated methylation also enhances aerobic glycolysis (77). The pyruvate kinase (PK) isoform M2 undergoes DNA methylation at exon 10 of the PK gene, correlating with increased PKM2 expression in breast cancer cells (78–80). Binding of Brother of Regulator of Imprinted Sites (BORIS) to the alternative exon 10 is thereby enhanced. Inhibiting DNA methylation, depleting BORIS or eliminating the BORIS binding site, switched splicing toward generating the normal PKM1 isoform. Loss of BORIS also suppresses the Warburg effect and growth of breast cancer cells (79).
DNA methylation of mitochondrial components, such as mitochondrial DNA (mtDNA), the mitochondrial quality control protein Mieap, and pyruvate dehydrogenase (PDH) kinase 4 (PDK4), causes mitochondrial dysfunction in cancer cells, triggering the Warburg effect (81). Methylation of mtDNA specifically causes dysfunction of oxidative phosphorylation, which promotes aerobic glycolysis as the primary method for rapid ATP synthesis in cancer cells (82). Mieap normally functions to induce intramitochondrial recruitment of lysosome-like organelles in order to eliminate oxidized mitochondrial proteins while maintaining mitochondrial structural integrity. Methylation of the Mieap promoter reduces Mieap abundance, leading to ROS accumulation and mitochondrial destruction (83–85).
DNA methylation changes affect activity of nuclear factor erythroid 2-related factor 2 (NRF2), which in turn regulates expression of transketolase (TKT) like-1 gene (TKT L1), and the fructose-1,6-bisphosphate isoform 1 (FBP1) (66). Methylation in the KEAP1 promoter reduces KEAP1 expression, and thereby abrogates NRF2 degradation (86–88). NRF2 is a transcriptional activator of genes in the pentose phosphate pathway [G6PDH, 6-phosphogluconate dehydrogenase (6PGD), transketolase (TKT), transaldolase and IDH (89)].
In basal-like breast cancer (42) and other cancer types (66), reduced Fructose-1,6-bisphosphatase (FBP1) expression, is due to FBP1 promoter methylation (42). Reduced FBP activity increases glycolysis, enhancing glucose uptake and reducing OXPHOS. The FBP1’s substrate, fructose-1,6-bisphosphate, is an allosteric activator of PKM2, providing a link between reduced FBP1 activity and the Warburg effect (90).
DNA methylation regulates HIF1 abundance and key components in the oxygen sensing pathway (91), including the tumor suppressor proteins WW-domain containing oxidoreductase (Wwox), carboxy-terminal domain 4 (CITED4), the LIM domain containing protein (LIMD1), and von Hippel-Lindau (VHL) (66). In breast cancer GLUT1 expression inversely correlated with Wwox (92). DNA methylation regulates Wwox expression, which modulates glucose metabolism (93). Under aerobic conditions, loss of Wwox reduces mitochondrial respiration and activates glycolytic gene expression, thereby inducing the Warburg effect (94). This is linked to accumulation of Wwox associates with HIF1-α, facilitating hydroxylation by prolyl hydroxylase 2 (PHD2) (92). CITED4 inhibits the HIF complex. Hypermethylation of the CITED4 promoter, reduces CITED4 expression in breast cancer, thereby increasing the expression of HIF and its target genes (95).
In melanoma cells and head and neck cancer cells, DNA hypomethylation of the TKT L1 gene promoter increases TKT L1 expression and activity, promoting HIF1-α accumulation and stability (96) and inducing the Warburg effect (96, 97). LIMD1 acts as a scaffold protein to bind PHD2 and VHL, which degrade HIF by ubiquitination (98) and increased methylation of LIMD1 and VHL are associated with upregulation of HIF1-α in cervical cancer (99).
Epigenetic Modification of the Tumor Microenvironment
Epigenetic modification of the tumor microenvironment also contributes to tumor metabolic heterogeneity. Epigenetic reprogramming in CAFs are biomarkers for cancer progression and promote cancer epithelial progression via paracrine signaling (100). Multiple epigenetic mechanisms, including DNA methylation, histone modification, and chromatin remodeling, together shape and reprogram the phenotypes of CAFs during tumorigenesis (101). Altered DNA methylation status of genes occurs in CAFs isolated from breast cancer tissues (68) and the prostate (102). CAF-secreted factors and stromal content of breast tumors regulated specific genes characterized by a DNA methylation pattern: hypermethylation at transcription start site and shore regions (103). CAFs from localized prostate cancer display distinct genome-wide changes in DNA methylation, significantly at enhancers and promoters, compared to nonmalignant prostate fibroblasts (NPFs) (104). In pancreatic cancer CAFs adopt unique DNA methylation and expression patterns upon interaction with PDA tumor cells (105). Fibroblasts can be reprogrammed to adopt a pro-invasive phenotype by leukemia inducible factor (LIF), which induced methylation through DNMT3B of the promoter region in the protein phosphatase regulator Src homology 2 domain-containing protein tyrosine phosphatase 1 (Shp-1) gene (106).
Immune cells, including tumor-associated macrophages (TAMs), participate in breast cancer onset and progression and contribute to the TME metabolic ecosystem to enhance tumor growth. TAMs generally show increased aerobic glycolysis but may use OXPHOS to generate energy. Bidirectional metabolic feedback occurs between macrophages and breast cancer cells in which M2 like macrophages induce sodium/glucose cotransporter 1 (SGLT1) in breast cancer cells and SGLT1 enhances lactic acid secretion to promote M2 macrophage polarization (107) CCL5 activates the CCR5 receptor, which participates in metastasis of breast (9, 108) and other cancers (109–111). Lactate induces the TAM phenotype, inducing CCL5 expression which promotes breast cancer cellular EMT and aerobic glycolysis via AMPK (112). Human mesenchymal stem cells (MSCs) induce the DNA methylation of the IL1A and IL1B genes when co-cultured with pancreatic ductal adenocarcinoma cells (PDAC) (113). The process of T-cell exhaustion is also controlled by epigenetic regulation and enrichment of T lymphocytes within the TME is a prerequisite for successful cancer immunotherapy (101).
Metabolic substrates from the tumor microenvironment in turn regulate methylation of stromal CAFs (114). The loss of cytosine methylation in de-novo generated CAFs is associated with the induction of inflammatory transcripts. Lactate produced by tumor cells leads to increased production of alpha-ketoglutarate (αKG) within mesenchymal stem cells (MSCs). In turn, αKG mediates activation of the demethylase TET enzyme leading to decreased cytosine methylation and increased hydroxymethylation during de novo differentiation of MSCs to CAF. Thus, in PDAC, a tumor-mediated lactate flux is associated with widespread epigenomic reprogramming that is seen during CAF formation.
The Demand for Glutamine
Glutamine is generally required for proliferation of normal and cancer cells (115), however cancer cells have an increased demand for glutamine as a source of nitrogen. Glutamine is used for the synthesis of amino acids and nucleotides (31) (Figure 3). In early studies, the optimal growth of cultured HeLa cells was shown to require a 10- to a 100-fold molar excess of glutamine in culture medium compared to other amino acids (116). Furthermore, glutamine is the most rapidly used amino acid (116). The increased use of glutamine has been established in the tumor microenvironment in vivo (117–120) and tumors demonstrate increased uptake of 18F-labeled glutamine using positron emission tomography (121). Breast cancer and other cell lines may develop resistance to this metabolic dependency (122). Glutamine is required for a variety of different cellular functions in proliferating cells, providing a source of nitrogen for the synthesis of purine and pyrimidine nucleotides and for the synthesis of other amino acids (123). Glutamine is deaminated by glutaminase to generate glutamate, which is converted to α-ketoglutarate. Within the mitochondria, α-ketoglutarate is converted to oxaloacetate, citrate and malate which in turn contribute to other anabolic pathways (31) (Figure 2). Uptake of cellular amino acids is also affected by glutamine as intracellular glutamine exchanges with extracellular leucine via LAT, a plasma membrane-localized antiporter for neutral amino acids (124). The cystine/glutamate antiporter, which imports cystine to provide cysteine for protein and glutathione synthesis is also regulated by glutamate which serves as the intracellular substrate for the plasma membrane antiporter (125).
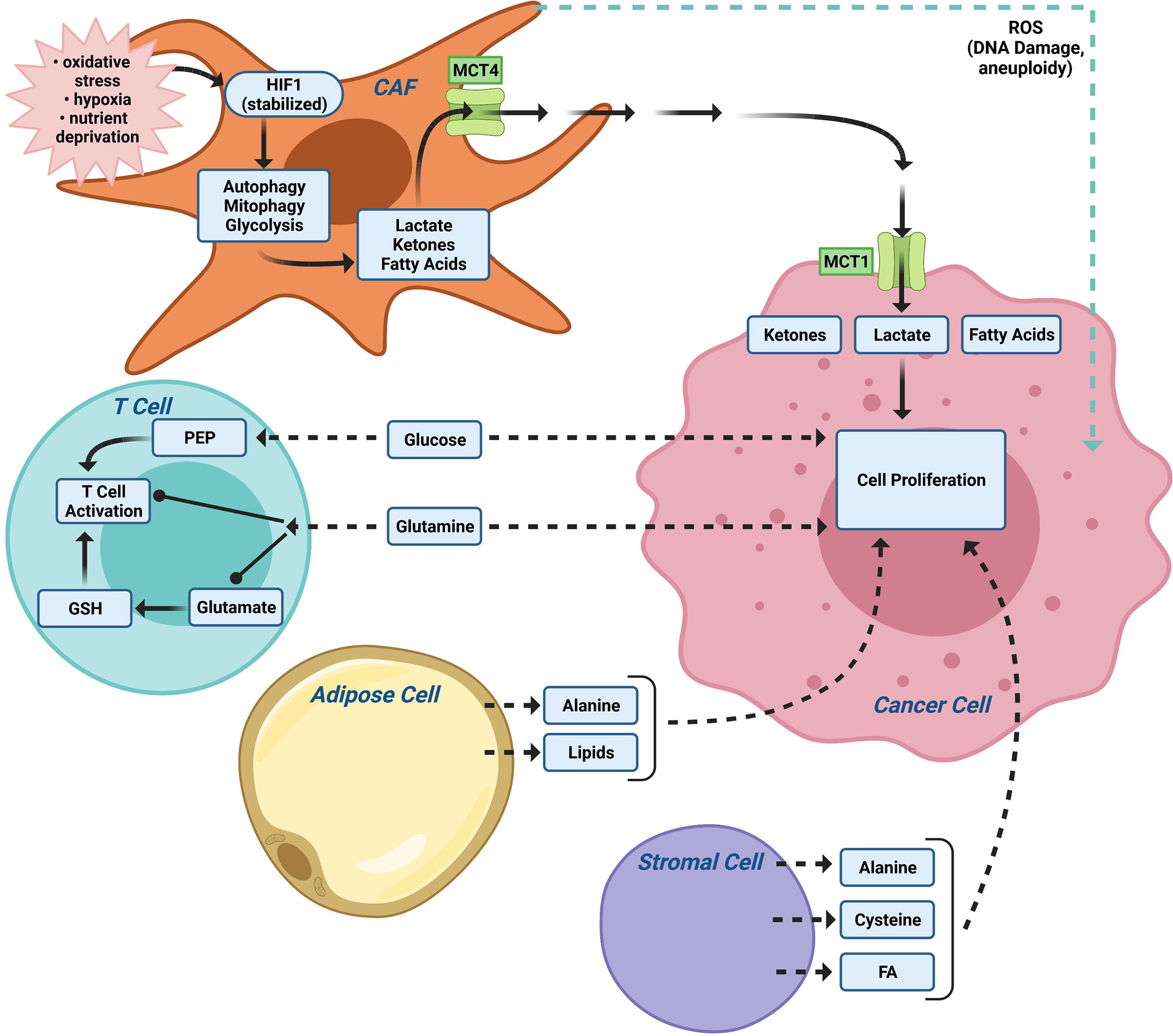
Figure 3 The tumor microenvironment (TME) contributes to metabolic tumor heterogeneity. In addition to CAFs (Figure 2), the local TME, including immune cells and adipocytes, provide nutrients for tumor metabolism. The relative importance of the different TME cellular subtypes varies between patients thereby contributing to additional levels of tumor heterogeneity. PEP (the glycolytic metabolite phosphoenolpyruvate).
Glutamine metabolism is under physiological control by the serine-threonine kinase, mammalian target of rapamycin (mTOR) pathway. mTOR governs several important cellular functions including cellular growth, survival, protein translation and autophagy. mTOR upregulates glutaminase (GLS) thereby increasing the conversion of glutamine to glutamate. The consequent increased production of α-ketoglutarate is then used within the TCA cycle (126). Restraint of mTOR activity also increases the ability of a cell to obtain extracellular proteins as a source of amino acids (127). Induction of mTOR correlates with increased HIF and VEGF which contribute to angiogenesis. Increased mTOR activity therefore collectively stimulates glutamine uptake, glutaminolysis, glycolysis, and angiogenesis in cancer cells.
c-myc governs glutamine homeostasis, with direct effects and indirect actions on cellular uptake via transporters ASCT2 (SLC1AS) (Figure 2) and SN2 (SLC38A5) (128). This enhanced uptake is associated with increased conversion to α-ketoglutarate (129) and incorporation into nucleic acid synthesis (130). The dependence of cancer cells survival on glutamine has led to testing of transport inhibitors targeting ASCT2 and the glutaminase (GLS) (Figure 2) inhibitors, CB-839 and BPTES, for anticancer therapies (131–133).
Heterogeneous Oxidative Metabolism in the Breast Tumor and the Tumor Microenvironment Provides Diverse Nutrients for Tumor Growth
Continued tumor growth requires the development of mechanisms to enhance access to diverse intracellular and extracellular nutrients (134, 135). Tumor cells retain a high level of metabolic plasticity, allowing them to both establish and subsequently adapt to the extracellular environment of a developing tumor. Heterogeneous tumor nutrients can be derived from tumor cells with different metabolic characteristics, in part driven by heterogeneous oxygenation within the tumor. In oxygenated tumor cells MCT1, which is expressed in breast cancers, serves as the prominent pathway for lactate uptake, which in turn serves as a substrate for tumor metabolism (47) Thus, there is a symbiosis by which glycolytic and oxidative tumor cells mutually regulate their access to energy metabolites based on heterogeneous oxygenation within the tumor (136).
Autophagy
Autophagy provides intracellular nutrients and is upregulated in dormant breast cancer cells promoting cancer cell survival under metabolic stress (137–139). Autophagy includes, macroautophagy, microautophagy and chaperone-mediated autophagy. During macroautophagy intracellular components are enveloped in double-membraned vesicles. Lysosomes fuse with autophagososomes resulting in degradation and recycling of these substrates in the cytosol (140). The autophagic process may either enhance or retrain tumor progression depending upon the stage of tumorigenesis. In a genome-wide screen, genes that negatively regulated autophagy were also involved in cellular growth and proliferation (141). Strong evidence for an association between mitogenic signaling in the restraint of autophagy led to studies wherein the pro-mitogenic, collaborative oncogene cyclin D1, was shown to restrain autophagy in breast cancer cells by modulating the activation of AMPK (142). AMPK enhanced autophagy and in human breast cancer cells cyclin D1 restrained AMPK activity (142). Cyclin D1 reduced activation of AMPK (pT172), via cyclin D1-Cdk4/Cdk6 phosphorylation of LKB1, thereby inhibiting mitochondrial function and promoting glycolysis (40, 41).
Tumor Microenvironment and Alternative Nutrients
Alternatively, the tumor microenvironment (TME) provides a rich source of distinct nutrients. Distinct cell types within the TME (cancer associated fibroblasts (CAFs), adipocytes, immune cells, tissue plasma/interstitial fluid) provide distinct nutrients to fuel tumor metabolism (Figure 3). Cancer-associated fibroblasts (CAFs) and adipocytes (143) can support malignant cells by providing nutrients such as alanine and lipids (143). Macrophages participate in TME metabolism (144) and MCT4 is expressed in macrophages (145). Understanding the source of nutrients for a particular tumor may provide an alternative therapeutic opportunity. Alternative substrates fueling tumor growth, include branched chain amino acids for de novo nucleotide and non-essential amino acid (NEAA) biosynthesis (146), acetate for acetyl-CoA and fatty acid synthesis (147), scavenging of extracellular lysophospholipids to bypass de novo lipogenesis (148), and macropinocytotic uptake and degradation of extracellular protein to maintain amino acid supply and bioenergetics (149, 150). Macropinocytotic uptake is induced by Ras (149, 151) and other oncogenic stimuli [reviewed in (152)]. Although the relative importance of scavenging pathways in breast cancer remains to be more fully understood, necrosis is a common feature of invasive breast cancer and breast tumor growth often outstrips the vasculature leaving tumor cells in nutrient-limited environment (153). Desmoplasia, a form of excessive fibrosis that limits perfusion, may favor the outgrowth of breast cancer cells that are capable of nutrient scavenging (154).
Cancer Associated Fibroblasts
The concept of scavenging alternative substrates to fuel tumor growth is illustrated by the “Reverse Warburg Effect” that was initially characterized in breast cancer cells (44, 47, 48). In the “Reverse Warburg” effect, anabolic cancer cells import lactate, ketones and fatty acids released by either adjacent cancer associated fibroblasts (CAF), other stromal cell types or catabolic cancer cells, in response to oxidative stress (21, 155). The “Autophagic Tumor Stroma Model of Cancer” proposes aerobic glycolysis in cancer associated fibroblasts (CAFs) generates energy-rich metabolites (such as lactate, ketone bodies and pyruvate) that are transferred to adjacent cancer cells, where they then enter the TCA cycle, promoting oxidative phosphorylation and increased ATP production (156–165) (Figure 4). In this model, hypoxia, nutrient deprivation and oxidative stress are thought to stabilize HIF1, which in turn causes catabolic autophagy, mitophagy and glycolysis, together with expression of the monocarboxylate transporter (MCT) 4 that exports lactate (21).
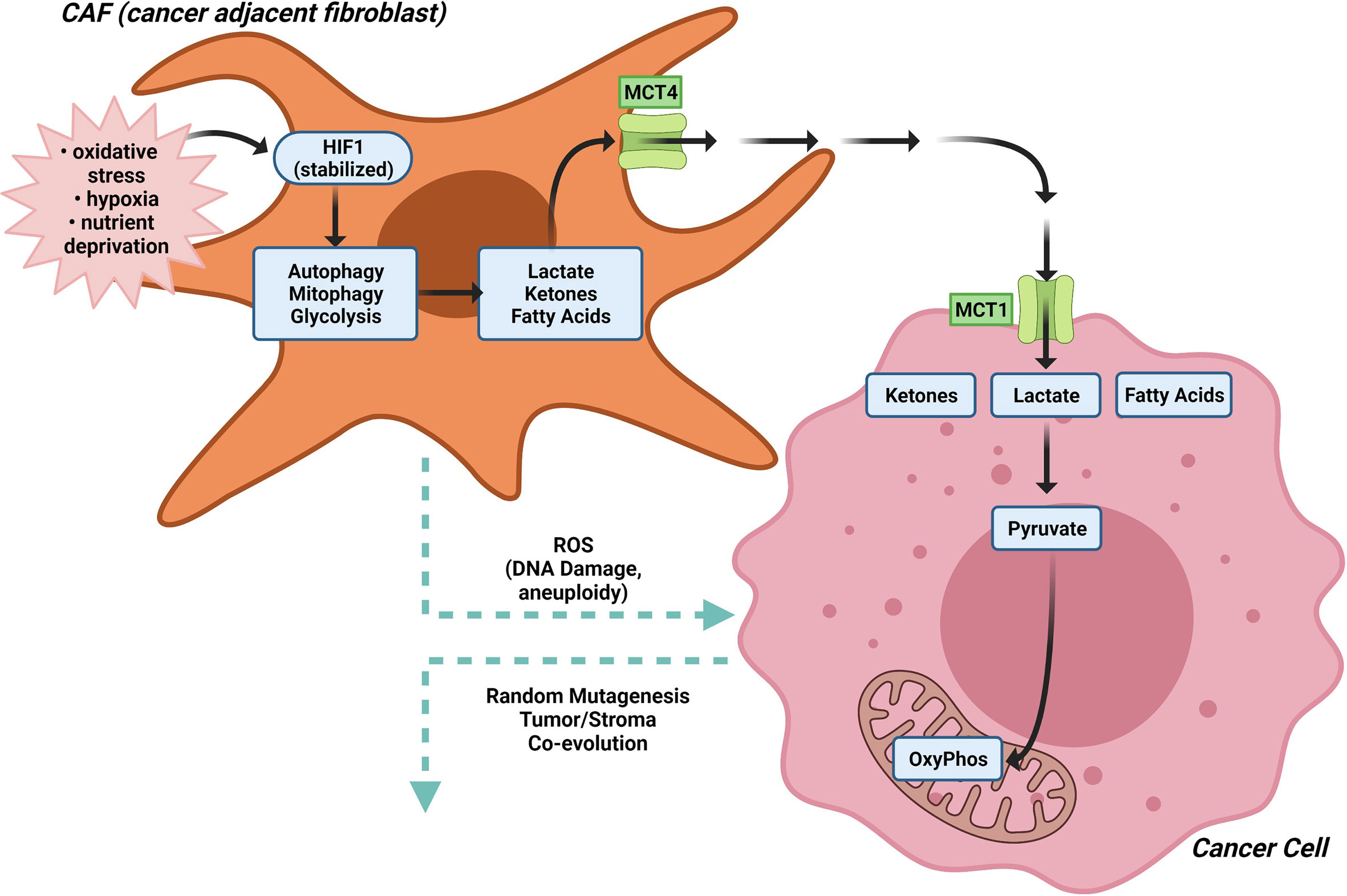
Figure 4 The Reverse Warburg effect. In the “Reverse Warburg Effect”, which was initially characterized in breast cancer cells, anabolic cancer cells import lactate, ketones and fatty acids released by either adjacent cancer associated fibroblasts (CAF), other stromal cell types or catabolic cancer cells, in response to oxidative stress. In CAFs, hypoxia, nutrient deprivation and oxidative stress stabilize HIF1α, which in turn causes catabolic autophagy, mitophagy and glycolysis, together with expression of the monocarboxylate transporter (MCT) 4 that exports lactate. Aerobic glycolysis in cancer associated fibroblasts (CAFs) generates energy-rich metabolites (such as lactate, ketone bodies and pyruvate) that are transferred to adjacent cancer cells, where they then enter the TCA cycle, promoting oxidative phosphorylation and increased ATP production.
The molecular drivers governing the CAF metabolic phenotype may involve downregulation of caveolin-1 (Cav-1) (157). Low expression of stromal Cav-1 correlates with a high rate of tumor recurrence, metastasis, tamoxifen resistance, and poor clinical outcome in breast carcinoma (166, 167). Oxidative stress in the tumor micro-environment then activates an autophagic program, governed in part by the tumor vasculature, leading to the production of recycled nutrients that can then be used as “fuel” to promote the anabolic growth and aggressive progression of tumor epithelial cells (Figure 3). Autophagy in cancer-associated fibroblasts protects tumor cells against apoptotic cell death, in part through the provision of recycled nutrients. Oxidative stress in the tumor microenvironment also has mutagenic consequences (157). ROS production in cancer-associated fibroblasts, induces DNA damage and aneuploidy in adjacent epithelial cancer cells serving as a catalyst for the random mutagenesis of tumor cells and for tumor-stroma co-evolution. Bidirectional metabolic interactions are also observed with glutamine metabolism. Co-targeting glutamine synthetase in stroma and glutaminase in cancer cells reduces tumor weight, nodules, and metastasis (168).
Additional substrates participating in tumor stroma metabolic cross talk have been described in pancreatic cancer for the use of branched-chain amino acids (BCAA) (169). Pancreatic ductal cancer (PDAC)-induces branch chain amino acid transaminase 1 (BCAT1) in CAFs which govern internalization of the extracellular matrix from the tumor microenvironment to supply amino-acid precursors for branched-chain α-ketoacid (BCKA). BCKA secretion by CAF are utilized by PDCA for protein synthesis and oxidative phosphorylation (169).
Tumor-Associated Macrophages
Tumor-associated macrophages (TAMs) and cancer cells co-exist in the context of a complex, bidirectional metabolic relationship. M1-like macrophages displaying enhanced glycolysis and reduced oxidative phosphorylation in contrast with more oxidative M2-like macrophages (170). TAMs exposed to hypoxia or lactate secrete multiple cytokines with metabolic functions, including IL6, TNF, C-C motif chemokine ligand 5 (CCL5) (112), and CCL18 (171). CCL5, and CCL18 boost the synthesis of multiple pro-glycolytic factors including HXK2, PGK1, lactate dehydrogenase A (LDHA), glucose-6-phosphate dehydrogenase (G6PD), pyruvate kinase M1/2 (PKM), pyruvate dehydrogenase kinase 1 (PDK1), pyruvate dehydrogenase (PDH), solute carrier family 2 member 1 (SLC2A1, best known as GLUT1), and vascular cell adhesion molecule 1 (VCAM1) and display lower glyceraldehyde 3-phosphate dehydrogenase (GAPDH) and succinate dehydrogenase (SDH) activity than normal macrophages. There are important consequences of tumor metabolites on immune function as Lactate secreted by glycolytic cancer cells, favors the polarization of immune cells to an immunosuppressive phenotype. Inhibiting glutamine synthetase activity in M2 macrophages skews their polarization toward an HIF1α-mediated M1 state, which impairs cytotoxic T cell recruitment and angiogenesis (172).
Stromal Adipose Cells, Extracellular Fluids and Exosomes
Stromal adipose cells contribute to the breast tumor metabolic microenvironment. In silico deconvolution estimates of cell type composition and molecular profiles of constituent cell types in the context of breast tumors applied to the TCGA data revealed metabolic coupling occurs between the epithelial and stroma cell types (173). A less adipose dense stroma displayed lower levels of mitochondrial activity and were associated with tumor cells with higher levels of oxidative metabolism. An adipokine. omental cell-derived circulating ITLN1 (intelectin-1, or omentin), induced a metabolic shift in metastatic ovarian cancer cell and decrease in tumor growth rates (174). Reduced glycolysis was observed in the cancer cells in vivo in mice given intraperitoneally injections of ITLN1, while increased glycolysis was observed in the adjacent cancer-associated adipocytes (174).
Tissue plasma and interstitial fluid contains soluble proteins that are normally not utilized as sources of amino acids. Tumor cells may activate processes to utilize these nutrients including entosis (175), [the engulfment and degradation of entire live cells), macropinocytosis, (the bulk uptake of extracellular fluid into large vesicles (176, 177)], and micropinocytosis (178). Micropinocytosis is augmented in cancer cells, through mutations including K-Ras, and c-Src, and activation of the phosphoinositide 3-kinase (PI3 kinase) (149, 179, 180) or Hippo pathway effectors Yap and Taz (181). Pancreatic and prostate cancers bearing oncogenic mutations in KRAS or PTEN, respectively, use amino acids derived from engulfed extracellular proteins to proliferate in nutrient-limiting environment (127, 182–184).
An additional mechanism providing tumor nutrients involves CAF-derived exosomes which contain intact metabolites, including amino acids, lipids, and TCA-cycle intermediates that are avidly utilized by cancer cells for central carbon metabolism. These metabolites promote tumor growth under nutrient deprivation or nutrient stressed conditions and inhibit mitochondrial oxidative phosphorylation increasing glycolysis and glutamine-dependent reductive carboxylation in cancer cells (185).
Altered Lipid Metabolism Within the Breast Tumor Epithelium and Tumor Microenvironment
Lipid synthesis increases in cancer cells, corresponding to an increased requirement for membrane synthesis during proliferation and cell division, cellular signaling and synthesis of hormones. Acetyl CoA carboxylase (ACC) activity is essential for breast cancer cell survival (186). Acetyl CoA carboxylase (ACC) and fatty acid synthase complex (FASN) are commonly upregulated in cancer cells (187, 188). ACC converts acetyl CoA (Figure 3) to malonyl CoA, rather than citrate, which in turn is converted by FAS to saturated fatty acids (SFA). Chemical inhibitors of ACC or genetic ablation of FASN by RNAi have shown some efficacy in cancer treatment (189). Lipid precursors are made available through glycolysis and through mitochondrial metabolism of glutamine through αketoglutarate to citrate (Figure 3). Increased glycolysis in cancer cells ensures the availability of dihydroxyacetone phosphate (DHAP) for conversion by glycerol-3-phosphate dehydrogenase 1 (GPD1) to glycerol-3-phosphate and thence phospholipids for cell membrane synthesis (190).
Expression of peroxisome proliferator-activated receptor gamma (PPARγ), a key regulator of lipogenesis, is altered in breast cancer. PPARγ expression is a positive prognostic factor in luminal and ductal breast cancer (191). PPARγ levels are inversely correlated with tumor size, grade and TNM staging (192, 193). PPARγ agonists trigger apoptosis, inhibit cell growth, decrease breast cancer cell motility and inhibit invasion of breast cancer cells (194). Ligands of PPARγ inhibit the expression of several cell cycle regulators thereby reducing cancer cell proliferation (195). The synthetic PPARγ ligands, rosiglitazone and troglitazone and endogenous 15dPGJ2 inhibit cyclin D1 gene expression via repression of cyclin D1 transcription, leading to cell cycle arrest (196). Although the role of PPARγ in tumor progression and metastasis remains controversial, in part because of the potential off target effects of PPARγ ligands (197), consistent with the important role of lipogenesis in breast cancer progression, recent studies showed that genetic deletion of Pparγ1 delayed the onset of tumorigenesis by mammary epithelial cell targeted ErbB2 (198).
Recent studies have identified ferroptosis-related gene expression pathways that predict outcome in breast cancer (199). Ferroptosis is a form of regulated necrosis driven by iron-dependent peroxidation of phospholipids, plays an important role in tumor suppression (200–202). Lipid metabolism can govern ferroptosis via sterol regulatory element-binding protein 1 (SREBP1), a central transcription factor regulating lipid metabolism. SREBP1m targets include gene governing lipogenesis (such as ACLY, ACC, FASN and stearoyl CoA desaturase 1), gluconeogenesis and the pentose phosphate pathway (Figure 2) including pyruvate kinase R isoform (PKLR), phosphoenolpyruvate carboxykinase 1 (PCK1), glucose 6-phosphatase (G6PC), and glucose 6-phosphate dehydrogenase (G6PDH). Sustained activation of mechanistic target of rapamycin complex 1 (mTORC1) through oncogenic activation of the PI3K-AKT pathway induces SREBP1 and provides resistance to ferroptosis in breast tumors in mice (203).
Cell Cycle Regulators Govern Tumor Metabolism
The cell-cycle governs cellular metabolism and, reciprocally, glycolytic enzyme activity can affect cellular proliferation and tumor aggressiveness, including through actions that are additional to their functions within glycolysis (204). Enhanced activity of the glycolytic enzymes, phosphoglycerate mutase (PGM) or glucose phosphate isomerase (GPI) induces proliferation of mouse embryonic fibroblasts and inhibition of these glycolytic enzymes promotes senescence (205). GPI converts glucose 6 phosphate to fructose 6 phosphate (Figure 2)
Regulators of cell cycle progression can also directly affect cellular metabolism. p53 for example downregulates PGM (204). Cyclin D1 overexpression restrains adipogenesis (206), suppresses mitochondrial function and biogenesis, and augments cytosolic glycolysis. The cyclin D1 gene is overexpressed in human breast cancer and is required for oncogene-induced tumorigenesis therefore the mechanism by which cyclin D1 governs tumor metabolism is of broad interest. Cyclin D1 encodes the regulatory subunit of the holoenzyme that phosphorylates and inactivates the RB protein. Early observations in cyclin D1 anti-sense transgenic mice targeting the mammary gland showed induction of mitochondrial and lipogenic regulatory gene clusters in vivo (41). The induction of cyclin D1 antisense in the mammary epithelial cell of transgenic mice induced acetyl-CoA carboxylase, fatty acid synthase, hexokinase II, and pyruvate kinase (Figure 2). A detailed gene expression analysis evidenced the impact of increased cyclin D1 to enhance the Warburg effect (207).
Several additional mechanisms have been described by which cyclin D1 regulates cytosolic glycolysis and induces the Warburg effect. Firstly, the cyclin D1/cdk4 complex phosphorylates NRF1 at a canonical cyclin D1/CDK4 phosphorylation site. NRF1 is a key nuclear transcription factor governing mitochondrial function with targets that include mitochondrial transcription factor A (mTFA). Consequences included reduced D loop transcriptional activity in mitochondrial DNA. Deletion of the cyclin D1 gene increased mitochondrial mass and mitochondrial activity function (40, 208). Secondly, in hepatocytes, cyclin D1–cyclin dependent kinase-4 (Cdk4) phosphorylates and activates the histone acetyltransferase, general control non-repressed protein 5 (GCN5), which then acetylates and inhibits peroxisome-proliferator-activated receptor-γ coactivator-1α (PGC-1α) activity at gluconeogenic genes (209). Thirdly, cyclin D1 increased phosphorylation of AKTSer 473 in breast cancer cells and animal models, augmented AKT1 activity (210), which in turn simulates the Warburg effect (211). Collectively these studies illustrate cyclin D1 promotes the Warburg effect in tissue culture and in vivo.
Mutations of Metabolic Genes in Cancer
As noted above, a number of genes and proteins that have direct roles in metabolism are regulated by oncogenes (c-Myc, cyclin D1, Ras, AKT/PI3K/mTOR), or tumor suppressors (p53) (212). Additionally, germ line and somatic mutations have been described in genes encoding enzymes that have direct roles in metabolism. Familial germline mutations in succinate dehydrogenase (213–215), and somatic mutations in isocitrate dehydrogenase 1 and 2 (IDH1 and IDH2), fumarate hydrase (FH) and isoforms of succinate dehydrogenase (SDH) are found in a variety of human cancers (216). IDH1 and IDH2 catalyze the decarboxylation of isocitrate to α-ketoglutarate (Figure 2). Fumarate hydratase catalyzes the reversible hydration of fumarate to malate. The multi-component SDH complex catalyzes the oxidation of succinate to fumarate, in concert with reducing ubiquinone in the electron transport chain. Succinate in turn may induce DNA hypermethylation (Figure 2). Tumors that accumulate succinate, show inhibition of 2-oxoglutarate-dependent histone and DNA demethylase enzymes, resulting in epigenetic silencing (217).
The metabolite profile itself drives oncogenesis. In the case of the IDH1 and IDH2 mutations, there is reduced production of αKG from isocitrate. αKG is a rate-limiting substrate for α-ketoglutarate-dependent dioxygenases that catalyze demethylation of DNA, histones and mRNA, and regulate HIF1α (212) (Figure 2). IDH1 mutations in some gliomas, and IDH1 and IDH2 mutations in some acute myeloblastic leukemias, convert αKG to R-2-hydroxyglutarate (2HG). 2HG can then suppress activity in α-ketoglutarate-dependent dioxygenases through competition with αKG. Inhibition of some dioxygenases by succinate or fumarate has also been rationalized as an effector pathway for loss of function of mutations in fumarate hydratase (FH), succinate dehydrogenase (31). Fumarate derivatization of cysteine residues within the Kelch-like ECH-associated protein 1 (KEAP1) may also occur, freeing NRF2 from KEAP1-mediated degradation (218).
Recent studies of over 900 cell lines revealed diverse metabolic changes with associated potential therapeutic potential. Hypermethylation of the gene encoding asparagine synthetase showed sensitivity to L-asparaginase (219). A comprehensive proteomic analysis combined with metabalomic and gene methylation analysis revealed the metabolic heterogeneity of the cancer cell lines (220). Analysis of 225 metabolites in 928 cell lines from 20 cancer types revealed several broad principles firstly, previously described mutations (IDH1, KEAP1) revealed the predicted change in metabolites. Secondly, that common oncogenic events (EGFR, KRAS, NRAS, TP53, PTEN, TSC1, TSC2) had weak to non-significant associations with profiled metabolites. Thirdly, that DNA hypermethylation influence metabolite production via suppressing degradation pathways. For example, methylation of SLC25A20 (carnitine/acylcarnitine translocase) in breast cancer cell lines led to accumulation of long chain acylcarnitine species. Fourthly, DNA hypermethylation regulates metabolite levels by limiting components of biosynthetic pathways. For example, hypermethylation of the PYCR gene, an enzyme that converts pyrroline-5-carboxylate to proline, was associated with reduced proline levels.
Epigenetic Regulation of EMT Governs Breast Cancer Metabolism
Carcinoma cells undergo an epithelial-to-mesenchymal transition (EMT) although the transition is considered a spectrum of changes, rather than a binary event (221). EMT-inducing transcription factor (EMT-TF) regulate the induction of EMT by repressing the transcription of epithelial genes while activating mesenchymal genes. EMT-TFs are regulated at a transcriptional level by DNA methylation, histone modifications, and RNA-mediated epigenetic regulation (222). Genetic regulators of EMT also directly regulate BCa cellular metabolism (223). Many pathways link EMT-TFs expression with glycolysis, mitochondrial metabolism, glutaminolysis and lipid metabolism (224), providing the rational basis for metabolic targeting of BCa cancer EMT (223). MDA-MB-231 is a mesenchymal basal-like breast cell line with decreased mitochondrial respiration compared to the epithelial luminal-like breast cell line, MCF-7. The decrease in oxidative phosphorylation correlated with the down regulation of succinate dehydrogenase B (SDHB, complex II), the core catalytic subunit of SDH in MDA-MB-231 cells (225). Decreased SDHB expression leads to metabolic reprogramming and migration and invasion of tumor cells by promoting EMT (226–228).
Most BCa cells express both epithelial and mesenchymal traits. When epithelial cancer cells lose their epithelial features and acquire a mesenchymal phenotype this promotes motility and invasion through loss of cell polarity, disruption E-cadherin/β-catenin leading to loss of cell-cell adhesion involved in cancer invasion and metastasis (225). This E/M hybrid state is facilitated by the differential expression of Snail (Snai1 and Snai2), bHLH (Twist1 and Twist2), and zinc finger and E-box binding (Zeb1 and Zeb2), collectively termed EMT-inducing transcription factors (EMT-TFs). The mesenchymal-like phenotype is accompanied by the expression of adult stem cell programs, notably, active canonical Wnt signaling.
The EMT transition in BCa is regulated by altered expression of the transcription factors SNAIL/SLUG (229), TGF-β (230), Twist, and Goosecoid and the cell-cycle control proteins [p21CIP1 (231), cyclin D1 (232)]. These EMT inducing agents in turn have been shown to regulate cellular metabolism (reviewed in (233). For example, the EMT TFs Slug/Twist suppresses succinate dehydrogenase (SDH), thereby repressing mitochondrial respiration, leading to the accumulation of succinate, which suppresses TET2, causes causing DNA hypermethylation, further promoting EMT in paraganglioma (234). Recent studies identified a novel role for the cell fate determination pathway in restraining EMT. Loss of DACH1 expression, a helix-turn helix protein of the Forkhead family that is a key determinant of the cell fate determination pathway, is a predictor of metastasis and poor survival in BCa (235). The DACH1 gene is silenced by methylation (236), and DACH1 in turns restrains the EMT program (237).
Emerging Questions in Metabolic Heterogeneity
The increased resolution of investigative technology has provided evidence for distinct sources of metabolic heterogeneity in BCa. Metabolic heterogeneity has been identified between genetic subtypes of breast cancer and within the components of the tumor microenvironment for an individual patient’s tumor. Several key questions have emerged as a consequence of the emerging understanding that tumors are highly heterogeneous (238). How can we best harness the knowledge that genetic mutations can alter a particular patent’s tumor metabolic in order to identify therapeutic vulnerabilities?
Analysis of large compendiums of tumor cell lines has identified correlates between altered genetic changes and metabolite production (220). However, evidence suggests that most human tumors acquire hundreds of somatic mutations in coding regions (239). Taking a broader definition of a metabolic genes to include the known upstream regulators of the enzymes that actually carry out the metabolic transformation, though, reveals the extent to which mutation or altered copy number pervades human cancers (240). Metabolic gene alterations are frequent and determine tumor aggressiveness and therapy responses (219, 240). High metabolic gene abnormality frequency correlated with worse prognosis (240). The most frequent metabolic gene abnormalities in breast cancer involve lipid metabolism (240). Bystander gene deletion may also contribute to tumor metabolic heterogeneity as metabolic genes may reside in proximity to known tumor suppressor genes that are deleted in cancer. The MTAP (methylthioadenosine phosphorylase gene for example may be co-deleted with CDKN2A, resulting in elevated methyl thioadenosine which sensitizes cells to PRMT5 inhibitors (241). How then to discern the functional significance of such ubiquitous mutational loads of metabolic genes in a tumor?
Intratumoral heterogeneity subclonal driver mutations that govern tumor metabolism have been identified in breast cancer (TP53, SMAD4) (238), consistent with studies of subclonal diversification of primary breast cancer revealed by multi region sequencing of the coding region (238), the non-coding region (242) and evidence for further genetic evolution upon relapse (SWI-SNF and JAK2-STAT3) (243). What metabolic vulnerabilities emerge in tumors with metabolically diverse subclonal populations?
Mathematical modeling approaches have been developed to understand the metabolic impact of altered gene expression on tumor metabolism. Modeling analysis of epithelial-to-mesenchymal transition has been conducted, in which metabolic pathway signatures have been used to quantify the activities of glycolysis, and the citric acid cycle with corresponding analysis of enzymes governing the metabolic processes in tumor samples (233, 244). Because tumors exhibit a spectrum of EMT and a spectrum of metabolic changes which may be topologically distinct, for example in the leading vs. the trailing edge of an invasive tumors, more accurate mathematical predictive models are required to provide precise metabolic therapeutics.
Linking the complex patterns of metabolic genetic alterations that occurs within a tumor to therapeutic co-extinction paradigms for individualized patient treatment remains a key challenge for future research.
Author Contributions
All authors listed have made a substantial, direct, and intellectual contribution to the work, and approved it for publication.
Funding
This work was supported in part by NIH R01CA132115, R21CA235139-01 RP and a Breakthrough Breast Cancer Research Program grant award from Department of Defense (W81XWH1810605) RP.
Conflict of Interest
RGP holds ownership interests in the companies ProstaGene, CytoDyn, LightSeed, Inc., and EcoGenome. RGP holds ownership interests (value unknown) of several patents and submitted patent applications.
The remaining authors declare that the research was conducted in the absence of any commercial or financial relationships that could be construed as a potential conflict of interest.
Publisher’s Note
All claims expressed in this article are solely those of the authors and do not necessarily represent those of their affiliated organizations, or those of the publisher, the editors and the reviewers. Any product that may be evaluated in this article, or claim that may be made by its manufacturer, is not guaranteed or endorsed by the publisher.
References
1. Siegel RL, Miller KD, Jemal A. Cancer Statistics, 2017. CA Cancer J Clin (2017) 67(1):7–30. doi: 10.3322/caac.21387
2. Gilabert M, Bertucci F, Esterni B, Madroszyk A, Tarpin C, Jacquemier J, et al. Capecitabine After Anthracycline and Taxane Exposure in HER2-Negative Metastatic Breast Cancer Patients: Response, Survival and Prognostic Factors. Anticancer Res (2011) 31(3):1079–86.
3. Cancer Genome Atlas N. Comprehensive Molecular Portraits of Human Breast Tumours. Nature (2012) 490(7418):61–70. doi: 10.1038/nature11412
4. Perou CM, Sorlie T, Eisen MB, van de Rijn M, Jeffrey SS, Rees CA, et al. Molecular Portraits of Human Breast Tumours. Nature (2000) 406(6797):747–52. doi: 10.1038/35021093
5. Cancer Genome Atlas NetworkComprehensive Molecular Portraits of Human Breast Tumours. Nature (2012) 490(7418):61–70. doi: 10.1038/nature11412
6. Shimelis H, LaDuca H, Hu C, Hart SN, Na J, Thomas A, et al. Triple-Negative Breast Cancer Risk Genes Identified by Multigene Hereditary Cancer Panel Testing. J Natl Cancer Inst (2018) 110(8):855–62. doi: 10.1093/jnci/djy106
7. Mittendorf EA, Philips AV, Meric-Bernstam F, Qiao N, Wu Y, Harrington S, et al. PD-L1 Expression in Triple-Negative Breast Cancer. Cancer Immunol Res (2014) 2(4):361–70. doi: 10.1158/2326-6066.CIR-13-0127
8. Velasco-Velazquez M, Jiao X, de la Fuente M, Pestell TG, Ertel A, Lisanti MP, et al. CCR5 Antagonist Blocks Metastasis of Basal Breast Cancer Cells. Cancer Res (2012) 72(15):3839–50. doi: 10.1158/0008-5472.CAN-11-3917
9. Jiao X, Velasco-Velazquez MA, Wang M, Li Z, Rui H, Peck AR, et al. CCR5 Governs DNA Damage Repair and Breast Cancer Stem Cell Expansion. Cancer Res (2018) 78(7):1657–71. doi: 10.1158/0008-5472.CAN-17-0915
10. Wang G, Gormley M, Qiao J, Zhao Q, Wang M, Di Sante G, et al. Cyclin D1-Mediated microRNA Expression Signature Predicts Breast Cancer Outcome. Theranostics (2018) 8(8):2251–63. doi: 10.7150/thno.23877
11. Cedro-Tanda A, Rios-Romero M, Romero-Cordoba S, Cisneros-Villanueva M, Rebollar-Vega RG, Alfaro-Ruiz LA, et al. A lncRNA Landscape in Breast Cancer Reveals a Potential Role for AC009283.1 in Proliferation and Apoptosis in HER2-Enriched Subtype. Sci Rep (2020) 10(1):13146. doi: 10.1038/s41598-020-69905-z
12. Volinia S, Galasso M, Sana ME, Wise TF, Palatini J, Huebner K, et al. Breast Cancer Signatures for Invasiveness and Prognosis Defined by Deep Sequencing of microRNA. Proc Natl Acad Sci USA (2012) 109(8):3024–9. doi: 10.1073/pnas.1200010109
13. Timmerman LA, Holton T, Yuneva M, Louie RJ, Padro M, Daemen A, et al. Glutamine Sensitivity Analysis Identifies the xCT Antiporter as a Common Triple-Negative Breast Tumor Therapeutic Target. Cancer Cell (2013) 24(4):450–65. doi: 10.1016/j.ccr.2013.08.020
14. Kung HN, Marks JR, Chi JT. Glutamine Synthetase Is a Genetic Determinant of Cell Type-Specific Glutamine Independence in Breast Epithelia. PloS Genet (2011) 7(8):e1002229. doi: 10.1371/journal.pgen.1002229
15. Lukey MJ, Cluntun AA, Katt WP, Lin MJ, Druso JE, Ramachandran S, et al. Liver-Type Glutaminase GLS2 Is a Druggable Metabolic Node in Luminal-Subtype Breast Cancer. Cell Rep (2019) 29(1):76–88.e7. doi: 10.1016/j.celrep.2019.08.076
16. Harbeck N, Penault-Llorca F, Cortes J, Gnant M, Houssami N, Poortmans P, et al. Breast Cancer. Nat Rev Dis Primers (2019) 5(1):66. doi: 10.1038/s41572-019-0111-2
17. Anampa J, Makower D, Sparano JA. Progress in Adjuvant Chemotherapy for Breast Cancer: An Overview. BMC Med (2015) 13:195. doi: 10.1186/s12916-015-0439-8
18. Baldassari F, Zerbinati C, Galasso M, Corra F, Minotti L, Agnoletto C, et al. Screen for MicroRNA and Drug Interactions in Breast Cancer Cell Lines Points to miR-126 as a Modulator of CDK4/6 and PIK3CA Inhibitors. Front Genet (2018) 9:174. doi: 10.3389/fgene.2018.00174
19. Balatti V, Croce CM. MicroRNA Dysregulation and Multi-Targeted Therapy for Cancer Treatment. Adv Biol Regul (2020) 75:100669. doi: 10.1016/j.jbior.2019.100669
20. Pekarsky Y, Croce CM. Noncoding RNA Genes in Cancer Pathogenesis. Adv Biol Regul (2019) 71:219–23. doi: 10.1016/j.jbior.2018.12.002
21. Martinez-Outschoorn UE, Peiris-Pages M, Pestell RG, Sotgia F, Lisanti MP. Cancer Metabolism: A Therapeutic Perspective. Nat Rev Clin Oncol (2017) 14(1):11–31. doi: 10.1038/nrclinonc.2016.60
22. Peiris-Pages M, Martinez-Outschoorn UE, Pestell RG, Sotgia F, Lisanti MP. Cancer Stem Cell Metabolism. Breast Cancer Res (2016) 18(1):55. doi: 10.1186/s13058-016-0712-6
23. DeBerardinis RJ, Lum JJ, Hatzivassiliou G, Thompson CB. The Biology of Cancer: Metabolic Reprogramming Fuels Cell Growth and Proliferation. Cell Metab (2008) 7(1):11–20. doi: 10.1016/j.cmet.2007.10.002
24. Warburg O. On the Origin of Cancer Cells. Science (1956) 123(3191):309–14. doi: 10.1126/science.123.3191.309
25. Schworer S, Vardhana SA, Thompson CB. Cancer Metabolism Drives a Stromal Regenerative Response. Cell Metab (2019) 29(3):576–91. doi: 10.1016/j.cmet.2019.01.015
26. Strickaert A, Saiselet M, Dom G, De Deken X, Dumont JE, Feron O, et al. Cancer Heterogeneity Is Not Compatible With One Unique Cancer Cell Metabolic Map. Oncogene (2017) 36(19):2637–42. doi: 10.1038/onc.2016.411
27. Hensley CT, Faubert B, Yuan Q, Lev-Cohain N, Jin E, Kim J, et al. Metabolic Heterogeneity in Human Lung Tumors. Cell (2016) 164(4):681–94. doi: 10.1016/j.cell.2015.12.034
28. Kim J, DeBerardinis RJ. Mechanisms and Implications of Metabolic Heterogeneity in Cancer. Cell Metab (2019) 30(3):434–46. doi: 10.1016/j.cmet.2019.08.013
29. Rao AD, DeBerardinis RJ. Metabolic Vulnerability in Tumours Illuminated. Nature (2019) 575(7782):296–7. doi: 10.1038/d41586-019-03239-3
30. Boroughs LK, DeBerardinis RJ. Metabolic Pathways Promoting Cancer Cell Survival and Growth. Nat Cell Biol (2015) 17(4):351–9. doi: 10.1038/ncb3124
31. Pavlova NN, Thompson CB. The Emerging Hallmarks of Cancer Metabolism. Cell Metab (2016) 23(1):27–47. doi: 10.1016/j.cmet.2015.12.006
32. Baek S, Choi CM, Ahn SH, Lee JW, Gong G, Ryu JS, et al. Exploratory Clinical Trial of (4S)-4-(3-[18F]Fluoropropyl)-L-Glutamate for Imaging xC- Transporter Using Positron Emission Tomography in Patients With Non-Small Cell Lung or Breast Cancer. Clin Cancer Res (2012) 18(19):5427–37. doi: 10.1158/1078-0432.CCR-12-0214
33. Xin Y, Cai H. Improved Radiosynthesis and Biological Evaluations of L- and D-1-[(18)F]Fluoroethyl-Tryptophan for PET Imaging of IDO-Mediated Kynurenine Pathway of Tryptophan Metabolism. Mol Imaging Biol (2017) 19(4):589–98. doi: 10.1007/s11307-016-1024-z
34. Kole AC, Nieweg OE, Pruim J, Paans AM, Plukker JT, Hoekstra HJ, et al. Standardized Uptake Value and Quantification of Metabolism for Breast Cancer Imaging With FDG and L-[1-11C]Tyrosine PET. J Nucl Med (1997) 38(5):692–6.
35. Haukaas TH, Euceda LR, Giskeodegard GF, Bathen TF. Metabolic Portraits of Breast Cancer by HR MAS MR Spectroscopy of Intact Tissue Samples. Metabolites (2017) 7(2):18. doi: 10.3390/metabo7020018
36. Leskinen-Kallio S, Nagren K, Lehikoinen P, Ruotsalainen U, Joensuu H. Uptake of 11C-Methionine in Breast Cancer Studied by PET. An Association With the Size of S-Phase Fraction. Br J Cancer (1991) 64(6):1121–4. doi: 10.1038/bjc.1991.475
37. Jager PL, Plaat BE, de Vries EG, Molenaar WM, Vaalburg W, Piers DA, et al. Imaging of Soft-Tissue Tumors Using L-3-[Iodine-123]Iodo-Alpha-Methyl-Tyrosine Single Photon Emission Computed Tomography: Comparison With Proliferative and Mitotic Activity, Cellularity, and Vascularity. Clin Cancer Res (2000) 6(6):2252–9.
38. Prendergast GC, Malachowski WP, DuHadaway JB, Muller AJ. Discovery of IDO1 Inhibitors: From Bench to Bedside. Cancer Res (2017) 77(24):6795–811. doi: 10.1158/0008-5472.CAN-17-2285
39. Muller AJ, DuHadaway JB, Donover PS, Sutanto-Ward E, Prendergast GC. Inhibition of Indoleamine 2,3-Dioxygenase, an Immunoregulatory Target of the Cancer Suppression Gene Bin1, Potentiates Cancer Chemotherapy. Nat Med (2005) 11(3):312–9. doi: 10.1038/nm1196
40. Wang C, Li Z, Lu Y, Du R, Katiyar S, Yang J, et al. Cyclin D1 Repression of Nuclear Respiratory Factor 1 Integrates Nuclear DNA Synthesis and Mitochondrial Function. Proc Natl Acad Sci USA (2006) 103(31):11567–72. doi: 10.1073/pnas.0603363103
41. Sakamaki T, Casimiro MC, Ju X, Quong AA, Katiyar S, Liu M, et al. Cyclin D1 Determines Mitochondrial Function In Vivo. Mol Cell Biol (2006) 26(14):5449–69. doi: 10.1128/MCB.02074-05
42. Dong C, Yuan T, Wu Y, Wang Y, Fan TW, Miriyala S, et al. Loss of FBP1 by Snail-Mediated Repression Provides Metabolic Advantages in Basal-Like Breast Cancer. Cancer Cell (2013) 23(3):316–31. doi: 10.1016/j.ccr.2013.01.022
43. Luo W, Chang R, Zhong J, Pandey A, Semenza GL. Histone Demethylase JMJD2C Is a Coactivator for Hypoxia-Inducible Factor 1 That Is Required for Breast Cancer Progression. Proc Natl Acad Sci USA (2012) 109(49):E3367–76. doi: 10.1073/pnas.1217394109
44. Cox E, Bonner J. Ecology. The Advantages of Togetherness. Science (2001) 292(5516):448–9. doi: 10.1126/science.1060456
45. Gomez-Cebrian N, Rojas-Benedicto A, Albors-Vaquer A, Lopez-Guerrero JA, Pineda-Lucena A, Puchades-Carrasco L. Metabolomics Contributions to the Discovery of Prostate Cancer Biomarkers. Metabolites (2019) 9(3):48. doi: 10.3390/metabo9030048
46. Giunchi F, Fiorentino M, Loda M. The Metabolic Landscape of Prostate Cancer. Eur Urol Oncol (2019) 2(1):28–36. doi: 10.1016/j.euo.2018.06.010
47. Sonveaux P, Vegran F, Schroeder T, Wergin MC, Verrax J, Rabbani ZN, et al. Targeting Lactate-Fueled Respiration Selectively Kills Hypoxic Tumor Cells in Mice. J Clin Invest (2008) 118(12):3930–42. doi: 10.1172/JCI36843
48. Pavlides S, Whitaker-Menezes D, Castello-Cros R, Flomenberg N, Witkiewicz AK, Frank PG, et al. The Reverse Warburg Effect: Aerobic Glycolysis in Cancer Associated Fibroblasts and the Tumor Stroma. Cell Cycle (2009) 8(23):3984–4001. doi: 10.4161/cc.8.23.10238
49. Ying H, Kimmelman AC, Lyssiotis CA, Hua S, Chu GC, Fletcher-Sananikone E, et al. Oncogenic Kras Maintains Pancreatic Tumors Through Regulation of Anabolic Glucose Metabolism. Cell (2012) 149(3):656–70. doi: 10.1016/j.cell.2012.01.058
50. Jiang P, Du W, Wang X, Mancuso A, Gao X, Wu M, et al. P53 Regulates Biosynthesis Through Direct Inactivation of Glucose-6-Phosphate Dehydrogenase. Nat Cell Biol (2011) 13(3):310–6. doi: 10.1038/ncb2172
51. Wahlstrom T, Henriksson MA. Impact of MYC in Regulation of Tumor Cell Metabolism. Biochim Biophys Acta (2015) 1849(5):563–9. doi: 10.1016/j.bbagrm.2014.07.004
52. Pate KT, Stringari C, Sprowl-Tanio S, Wang K, TeSlaa T, Hoverter NP, et al. Wnt Signaling Directs a Metabolic Program of Glycolysis and Angiogenesis in Colon Cancer. EMBO J (2014) 33(13):1454–73. doi: 10.15252/embj.201488598
53. Vander Heiden MG, Cantley LC, Thompson CB. Understanding the Warburg Effect: The Metabolic Requirements of Cell Proliferation. Science (2009) 324(5930):1029–33. doi: 10.1126/science.1160809
54. Brand K, Leibold W, Luppa P, Schoerner C, Schulz A. Metabolic Alterations Associated With Proliferation of Mitogen-Activated Lymphocytes and of Lymphoblastoid Cell Lines: Evaluation of Glucose and Glutamine Metabolism. Immunobiology (1986) 173(1):23–34. doi: 10.1016/S0171-2985(86)80086-9
55. Pfeiffer T, Schuster S, Bonhoeffer S. Cooperation and Competition in the Evolution of ATP-Producing Pathways. Science (2001) 292(5516):504–7. doi: 10.1126/science.1058079
56. Fan J, Ye J, Kamphorst JJ, Shlomi T, Thompson CB, Rabinowitz JD. Quantitative Flux Analysis Reveals Folate-Dependent NADPH Production. Nature (2014) 510(7504):298–302. doi: 10.1038/nature13236
57. Possemato R, Marks KM, Shaul YD, Pacold ME, Kim D, Birsoy K, et al. Functional Genomics Reveal That the Serine Synthesis Pathway Is Essential in Breast Cancer. Nature (2011) 476(7360):346–50. doi: 10.1038/nature10350
58. Locasale JW, Grassian AR, Melman T, Lyssiotis CA, Mattaini KR, Bass AJ, et al. Phosphoglycerate Dehydrogenase Diverts Glycolytic Flux and Contributes to Oncogenesis. Nat Genet (2011) 43(9):869–74. doi: 10.1038/ng.890
59. Wang T, Marquardt C, Foker J. Aerobic Glycolysis During Lymphocyte Proliferation. Nature (1976) 261(5562):702–5. doi: 10.1038/261702a0
60. Porporato PE, Payen VL, De Saedeleer CJ, Preat V, Thissen JP, Feron O, et al. Lactate Stimulates Angiogenesis and Accelerates the Healing of Superficial and Ischemic Wounds in Mice. Angiogenesis (2012) 15(4):581–92. doi: 10.1007/s10456-012-9282-0
61. Constant JS, Feng JJ, Zabel DD, Yuan H, Suh DY, Scheuenstuhl H, et al. Lactate Elicits Vascular Endothelial Growth Factor From Macrophages: A Possible Alternative to Hypoxia. Wound Repair Regener (2000) 8(5):353–60. doi: 10.1111/j.1524-475X.2000.00353.x
62. Stern R, Shuster S, Neudecker BA, Formby B. Lactate Stimulates Fibroblast Expression of Hyaluronan and CD44: The Warburg Effect Revisited. Exp Cell Res (2002) 276(1):24–31. doi: 10.1006/excr.2002.5508
63. Rothberg JM, Bailey KM, Wojtkowiak JW, Ben-Nun Y, Bogyo M, Weber E, et al. Acid-Mediated Tumor Proteolysis: Contribution of Cysteine Cathepsins. Neoplasia (2013) 15(10):1125–37. doi: 10.1593/neo.13946
64. Estrella V, Chen T, Lloyd M, Wojtkowiak J, Cornnell HH, Ibrahim-Hashim A, et al. Acidity Generated by the Tumor Microenvironment Drives Local Invasion. Cancer Res (2013) 73(5):1524–35. doi: 10.1158/0008-5472.CAN-12-2796
65. Vegran F, Boidot R, Michiels C, Sonveaux P, Feron O. Lactate Influx Through the Endothelial Cell Monocarboxylate Transporter MCT1 Supports an NF-Kappab/IL-8 Pathway That Drives Tumor Angiogenesis. Cancer Res (2011) 71(7):2550–60. doi: 10.1158/0008-5472.CAN-10-2828
66. Zhu X, Xuan Z, Chen J, Li Z, Zheng S, Song P. How DNA Methylation Affects the Warburg Effect. Int J Biol Sci (2020) 16(12):2029–41. doi: 10.7150/ijbs.45420
67. Schvartzman JM, Thompson CB, Finley LWS. Metabolic Regulation of Chromatin Modifications and Gene Expression. J Cell Biol (2018) 217(7):2247–59. doi: 10.1083/jcb.201803061
68. Hu M, Yao J, Cai L, Bachman KE, van den Brule F, Velculescu V, et al. Distinct Epigenetic Changes in the Stromal Cells of Breast Cancers. Nat Genet (2005) 37(8):899–905. doi: 10.1038/ng1596
69. Holm K, Hegardt C, Staaf J, Vallon-Christersson J, Jonsson G, Olsson H, et al. Molecular Subtypes of Breast Cancer Are Associated With Characteristic DNA Methylation Patterns. Breast Cancer Res (2010) 12(3):R36. doi: 10.1186/bcr2590
70. Lopez-Serra P, Marcilla M, Villanueva A, Ramos-Fernandez A, Palau A, Leal L, et al. A DERL3-Associated Defect in the Degradation of SLC2A1 Mediates the Warburg Effect. Nat Commun (2014) 5:3608. doi: 10.1038/ncomms4608
71. Ha TK, Her NG, Lee MG, Ryu BK, Lee JH, Han J, et al. Caveolin-1 Increases Aerobic Glycolysis in Colorectal Cancers by Stimulating HMGA1-Mediated GLUT3 Transcription. Cancer Res (2012) 72(16):4097–109. doi: 10.1158/0008-5472.CAN-12-0448
72. Yen CY, Huang HW, Shu CW, Hou MF, Yuan SS, Wang HR, et al. DNA Methylation, Histone Acetylation and Methylation of Epigenetic Modifications as a Therapeutic Approach for Cancers. Cancer Lett (2016) 373(2):185–92. doi: 10.1016/j.canlet.2016.01.036
73. Brown NJ, Higham SE, Perunovic B, Arafa M, Balasubramanian S, Rehman I. Lactate Dehydrogenase-B Is Silenced by Promoter Methylation in a High Frequency of Human Breast Cancers. PloS One (2013) 8(2):e57697. doi: 10.1371/journal.pone.0057697
74. San-Millan I, Brooks GA. Reexamining Cancer Metabolism: Lactate Production for Carcinogenesis Could be the Purpose and Explanation of the Warburg Effect. Carcinogenesis (2017) 38(2):119–33. doi: 10.1093/carcin/bgw127
75. Thakur C, Chen F. Connections Between Metabolism and Epigenetics in Cancers. Semin Cancer Biol (2019) 57:52–8. doi: 10.1016/j.semcancer.2019.06.006
76. Desai S, Ding M, Wang B, Lu Z, Zhao Q, Shaw K, et al. Tissue-Specific Isoform Switch and DNA Hypomethylation of the Pyruvate Kinase PKM Gene in Human Cancers. Oncotarget (2014) 5(18):8202–10. doi: 10.18632/oncotarget.1159
77. Zhong XY, Yuan XM, Xu YY, Yin M, Yan WW, Zou SW, et al. CARM1 Methylates GAPDH to Regulate Glucose Metabolism and Is Suppressed in Liver Cancer. Cell Rep (2018) 24(12):3207–23. doi: 10.1016/j.celrep.2018.08.066
78. Christofk HR, Vander Heiden MG, Harris MH, Ramanathan A, Gerszten RE, Wei R, et al. The M2 Splice Isoform of Pyruvate Kinase Is Important for Cancer Metabolism and Tumour Growth. Nature (2008) 452(7184):230–3. doi: 10.1038/nature06734
79. Singh S, Narayanan SP, Biswas K, Gupta A, Ahuja N, Yadav S, et al. Intragenic DNA Methylation and BORIS-Mediated Cancer-Specific Splicing Contribute to the Warburg Effect. Proc Natl Acad Sci USA (2017) 114(43):11440–5. doi: 10.1073/pnas.1708447114
80. Luo W, Semenza GL. Emerging Roles of PKM2 in Cell Metabolism and Cancer Progression. Trends Endocrinol Metab (2012) 23(11):560–6. doi: 10.1016/j.tem.2012.06.010
81. Gaowa S, Futamura M, Tsuneki M, Kamino H, Tajima JY, Mori R, et al. Possible Role of P53/Mieap-Regulated Mitochondrial Quality Control as a Tumor Suppressor in Human Breast Cancer. Cancer Sci (2018) 109(12):3910–20. doi: 10.1111/cas.13824
82. Menga A, Palmieri EM, Cianciulli A, Infantino V, Mazzone M, Scilimati A, et al. SLC25A26 Overexpression Impairs Cell Function via mtDNA Hypermethylation and Rewiring of Methyl Metabolism. FEBS J (2017) 284(6):967–84. doi: 10.1111/febs.14028
83. Miyamoto Y, Kitamura N, Nakamura Y, Futamura M, Miyamoto T, Yoshida M, et al. Possible Existence of Lysosome-Like Organella Within Mitochondria and Its Role in Mitochondrial Quality Control. PloS One (2011) 6(1):e16054. doi: 10.1371/journal.pone.0016054
84. Tsuneki M, Nakamura Y, Kinjo T, Nakanishi R, Arakawa H. Mieap Suppresses Murine Intestinal Tumor via Its Mitochondrial Quality Control. Sci Rep (2015) 5:12472. doi: 10.1038/srep12472
85. Nakamura Y, Arakawa H. Discovery of Mieap-Regulated Mitochondrial Quality Control as a New Function of Tumor Suppressor P53. Cancer Sci (2017) 108(5):809–17. doi: 10.1111/cas.13208
86. Muscarella LA, Barbano R, D’Angelo V, Copetti M, Coco M, Balsamo T, et al. Regulation of KEAP1 Expression by Promoter Methylation in Malignant Gliomas and Association With Patient’s Outcome. Epigenetics (2011) 6(3):317–25. doi: 10.4161/epi.6.3.14408
87. Muscarella LA, Parrella P, D’Alessandro V, la Torre A, Barbano R, Fontana A, et al. Frequent Epigenetics Inactivation of KEAP1 Gene in Non-Small Cell Lung Cancer. Epigenetics (2011) 6(6):710–9. doi: 10.4161/epi.6.6.15773
88. Zhang P, Singh A, Yegnasubramanian S, Esopi D, Kombairaju P, Bodas M, et al. Loss of Kelch-Like ECH-Associated Protein 1 Function in Prostate Cancer Cells Causes Chemoresistance and Radioresistance and Promotes Tumor Growth. Mol Cancer Ther (2010) 9(2):336–46. doi: 10.1158/1535-7163.MCT-09-0589
89. Taguchi K, Motohashi H, Yamamoto M. Molecular Mechanisms of the Keap1-Nrf2 Pathway in Stress Response and Cancer Evolution. Genes Cells (2011) 16(2):123–40. doi: 10.1111/j.1365-2443.2010.01473.x
90. Yang W, Lu Z. Regulation and Function of Pyruvate Kinase M2 in Cancer. Cancer Lett (2013) 339(2):153–8. doi: 10.1016/j.canlet.2013.06.008
91. Ristic B, Bhutia YD, Ganapathy V. Cell-Surface G-Protein-Coupled Receptors for Tumor-Associated Metabolites: A Direct Link to Mitochondrial Dysfunction in Cancer. Biochim Biophys Acta Rev Cancer (2017) 1868(1):246–57. doi: 10.1016/j.bbcan.2017.05.003
92. Abu-Remaileh M, Aqeilan RI. The Tumor Suppressor WW Domain-Containing Oxidoreductase Modulates Cell Metabolism. Exp Biol Med (Maywood) (2015) 240(3):345–50. doi: 10.1177/1535370214561956
93. Ekizoglu S, Bulut P, Karaman E, Kilic E, Buyru N. Epigenetic and Genetic Alterations Affect the WWOX Gene in Head and Neck Squamous Cell Carcinoma. PloS One (2015) 10(1):e0115353. doi: 10.1371/journal.pone.0115353
94. Abu-Remaileh M, Aqeilan RI. Tumor Suppressor WWOX Regulates Glucose Metabolism via HIF1alpha Modulation. Cell Death Differ (2014) 21(11):1805–14. doi: 10.1038/cdd.2014.95
95. Huang KT, Takano EA, Mikeska T, Byrne DJ, Dobrovic A, Fox SB. Aberrant DNA Methylation But Not Mutation of CITED4 Is Associated With Alteration of HIF-Regulated Genes in Breast Cancer. Breast Cancer Res Treat (2011) 130(1):319–29. doi: 10.1007/s10549-011-1657-1
96. Sun W, Liu Y, Glazer CA, Shao C, Bhan S, Demokan S, et al. TKTL1 Is Activated by Promoter Hypomethylation and Contributes to Head and Neck Squamous Cell Carcinoma Carcinogenesis Through Increased Aerobic Glycolysis and HIF1alpha Stabilization. Clin Cancer Res (2010) 16(3):857–66. doi: 10.1158/1078-0432.CCR-09-2604
97. Jayachandran A, Lo PH, Chueh AC, Prithviraj P, Molania R, Davalos-Salas M, et al. Transketolase-Like 1 Ectopic Expression Is Associated With DNA Hypomethylation and Induces the Warburg Effect in Melanoma Cells. BMC Cancer (2016) 16:134. doi: 10.1186/s12885-016-2185-5
98. Foxler DE, Bridge KS, James V, Webb TM, Mee M, Wong SC, et al. The LIMD1 Protein Bridges an Association Between the Prolyl Hydroxylases and VHL to Repress HIF-1 Activity. Nat Cell Biol (2012) 14(2):201–8. doi: 10.1038/ncb2424
99. Chakraborty C, Mitra S, Roychowdhury A, Samadder S, Dutta S, Roy A, et al. Deregulation of LIMD1-VHL-HIF-1alpha-VEGF Pathway Is Associated With Different Stages of Cervical Cancer. Biochem J (2018) 475(10):1793–806. doi: 10.1042/BCJ20170649
100. Mishra R, Haldar S, Suchanti S, Bhowmick NA. Epigenetic Changes in Fibroblasts Drive Cancer Metabolism and Differentiation. Endocr Relat Cancer (2019) 26(12):R673–88. doi: 10.1530/ERC-19-0347
101. Pan X, Zheng L. Epigenetics in Modulating Immune Functions of Stromal and Immune Cells in the Tumor Microenvironment. Cell Mol Immunol (2020) 17(9):940–53. doi: 10.1038/s41423-020-0505-9
102. Hanson JA, Gillespie JW, Grover A, Tangrea MA, Chuaqui RF, Emmert-Buck MR, et al. Gene Promoter Methylation in Prostate Tumor-Associated Stromal Cells. J Natl Cancer Inst (2006) 98(4):255–61. doi: 10.1093/jnci/djj051
103. Mathot P, Grandin M, Devailly G, Souaze F, Cahais V, Moran S, et al. DNA Methylation Signal has a Major Role in the Response of Human Breast Cancer Cells to the Microenvironment. Oncogenesis (2017) 6(10):e390. doi: 10.1038/oncsis.2017.88
104. Pidsley R, Lawrence MG, Zotenko E, Niranjan B, Statham A, Song J, et al. Enduring Epigenetic Landmarks Define the Cancer Microenvironment. Genome Res (2018) 28(5):625–38. doi: 10.1101/gr.229070.117
105. Xiao Q, Zhou D, Rucki AA, Williams J, Zhou J, Mo G, et al. Cancer-Associated Fibroblasts in Pancreatic Cancer Are Reprogrammed by Tumor-Induced Alterations in Genomic DNA Methylation. Cancer Res (2016) 76(18):5395–404. doi: 10.1158/0008-5472.CAN-15-3264
106. Albrengues J, Bourget I, Pons C, Butet V, Hofman P, Tartare-Deckert S, et al. LIF Mediates Proinvasive Activation of Stromal Fibroblasts in Cancer. Cell Rep (2014) 7(5):1664–78. doi: 10.1016/j.celrep.2014.04.036
107. Niu X, Ma J, Li J, Gu Y, Yin L, Wang Y, et al. Sodium/glucose Cotransporter 1-Dependent Metabolic Alterations Induce Tamoxifen Resistance in Breast Cancer by Promoting Macrophage M2 Polarization. Cell Death Dis (2021) 12(6):509. doi: 10.1038/s41419-021-03781-x
108. Jiao X, Wang M, Zhang Z, Li Z, Ni D, Ashton AW, et al. Leronlimab, a Humanized Monoclonal Antibody to CCR5, Blocks Breast Cancer Cellular Metastasis and Enhances Cell Death Induced by DNA Damaging Chemotherapy. Breast Cancer Res (2021) 23(1):11. doi: 10.1186/s13058-021-01391-1
109. Sicoli D, Jiao X, Ju X, Velasco-Velazquez M, Ertel A, Addya S, et al. CCR5 Receptor Antagonists Block Metastasis to Bone of V-Src Oncogene-Transformed Metastatic Prostate Cancer Cell Lines. Cancer Res (2014) 74(23):7103–14. doi: 10.1158/0008-5472.CAN-14-0612
110. Jiao X, Nawab O, Patel T, Kossenkov AV, Halama N, Jaeger D, et al. Recent Advances Targeting CCR5 for Cancer and Its Role in Immuno-Oncology. Cancer Res (2019) 79(19):4801–7. doi: 10.1158/0008-5472.CAN-19-1167
111. Novak M, Koprivnikar Krajnc M, Hrastar B, Breznik B, Majc B, Mlinar M, et al. CCR5-Mediated Signaling Is Involved in Invasion of Glioblastoma Cells in Its Microenvironment. Int J Mol Sci (2020) 21(12):4199. doi: 10.3390/ijms21124199
112. Lin S, Sun L, Lyu X, Ai X, Du D, Su N, et al. Lactate-Activated Macrophages Induced Aerobic Glycolysis and Epithelial-Mesenchymal Transition in Breast Cancer by Regulation of CCL5-CCR5 Axis: A Positive Metabolic Feedback Loop. Oncotarget (2017) 8(66):110426–43. doi: 10.18632/oncotarget.22786
113. Ohlund D, Handly-Santana A, Biffi G, Elyada E, Almeida AS, Ponz-Sarvise M, et al. Distinct Populations of Inflammatory Fibroblasts and Myofibroblasts in Pancreatic Cancer. J Exp Med (2017) 214(3):579–96. doi: 10.1084/jem.20162024
114. Bhagat TD, Von Ahrens D, Dawlaty M, Zou Y, Baddour J, Achreja A, et al. Lactate-Mediated Epigenetic Reprogramming Regulates Formation of Human Pancreatic Cancer-Associated Fibroblasts. Elife (2019) 8:e50663. doi: 10.7554/eLife.50663
115. DeBerardinis RJ, Mancuso A, Daikhin E, Nissim I, Yudkoff M, Wehrli S, et al. Beyond Aerobic Glycolysis: Transformed Cells can Engage in Glutamine Metabolism That Exceeds the Requirement for Protein and Nucleotide Synthesis. Proc Natl Acad Sci USA (2007) 104(49):19345–50. doi: 10.1073/pnas.0709747104
116. Eagle H. The Minimum Vitamin Requirements of the L and HeLa Cells in Tissue Culture, the Production of Specific Vitamin Deficiencies, and Their Cure. J Exp Med (1955) 102(5):595–600. doi: 10.1084/jem.102.5.595
117. Urbano-Marquez A, Estruch R, Navarro-Lopez F, Grau JM, Mont L, Rubin E. The Effects of Alcoholism on Skeletal and Cardiac Muscle. N Engl J Med (1989) 320(7):409–15. doi: 10.1056/NEJM198902163200701
118. Rivera SC, Hazen TC, Toranzos GA. Isolation of Fecal Coliforms From Pristine Sites in a Tropical Rain Forest. Appl Environ Microbiol (1988) 54(2):513–7. doi: 10.1128/aem.54.2.513-517.1988
119. Roberts E, Frankel S. Free Amino Acids in Normal and Neoplastic Tissues of Mice as Studied by Paper Chromatography. Cancer Res (1949) 9(11):645–8, 3 pl.
120. Yuneva MO, Fan TW, Allen TD, Higashi RM, Ferraris DV, Tsukamoto T, et al. The Metabolic Profile of Tumors Depends on Both the Responsible Genetic Lesion and Tissue Type. Cell Metab (2012) 15(2):157–70. doi: 10.1016/j.cmet.2011.12.015
121. Lieberman BP, Ploessl K, Wang L, Qu W, Zha Z, Wise DR, et al. PET Imaging of Glutaminolysis in Tumors by 18F-(2S,4R)4-Fluoroglutamine. J Nucl Med (2011) 52(12):1947–55. doi: 10.2967/jnumed.111.093815
122. Kung CP, Budina A, Balaburski G, Bergenstock MK, Murphy M. Autophagy in Tumor Suppression and Cancer Therapy. Crit Rev Eukaryot Gene Expr (2011) 21(1):71–100. doi: 10.1615/CritRevEukarGeneExpr.v21.i1.50
123. Curi R, Lagranha CJ, Doi SQ, Sellitti DF, Procopio J, Pithon-Curi TC, et al. Molecular Mechanisms of Glutamine Action. J Cell Physiol (2005) 204(2):392–401. doi: 10.1002/jcp.20339
124. Yanagida O, Kanai Y, Chairoungdua A, Kim DK, Segawa H, Nii T, et al. Human L-Type Amino Acid Transporter 1 (LAT1): Characterization of Function and Expression in Tumor Cell Lines. Biochim Biophys Acta (2001) 1514(2):291–302. doi: 10.1016/S0005-2736(01)00384-4
125. Lo E, Nicolle L, Classen D, Arias KM, Podgorny K, Anderson DJ, et al. Strategies to Prevent Catheter-Associated Urinary Tract Infections in Acute Care Hospitals. Infect Control Hosp Epidemiol (2008) 29 Suppl 1:S41–50. doi: 10.1086/591066
126. Jin L, Alesi GN, Kang S. Glutaminolysis as a Target for Cancer Therapy. Oncogene (2016) 35(28):3619–25. doi: 10.1038/onc.2015.447
127. Palm W, Park Y, Wright K, Pavlova NN, Tuveson DA, Thompson CB. The Utilization of Extracellular Proteins as Nutrients Is Suppressed by Mtorc1. Cell (2015) 162(2):259–70. doi: 10.1016/j.cell.2015.06.017
128. Li B, Simon MC. Molecular Pathways: Targeting MYC-Induced Metabolic Reprogramming and Oncogenic Stress in Cancer. Clin Cancer Res (2013) 19(21):5835–41. doi: 10.1158/1078-0432.CCR-12-3629
129. Gao P, Tchernyshyov I, Chang TC, Lee YS, Kita K, Ochi T, et al. C-Myc Suppression of miR-23a/B Enhances Mitochondrial Glutaminase Expression and Glutamine Metabolism. Nature (2009) 458(7239):762–5. doi: 10.1038/nature07823
130. Mannava S, Grachtchouk V, Wheeler LJ, Im M, Zhuang D, Slavina EG, et al. Direct Role of Nucleotide Metabolism in C-MYC-Dependent Proliferation of Melanoma Cells. Cell Cycle (2008) 7(15):2392–400. doi: 10.4161/cc.6390
131. Xiang Y, Stine ZE, Xia J, Lu Y, O’Connor RS, Altman BJ, et al. Targeted Inhibition of Tumor-Specific Glutaminase Diminishes Cell-Autonomous Tumorigenesis. J Clin Invest (2015) 125(6):2293–306. doi: 10.1172/JCI75836
132. Gross AE, Van Schooneveld TC, Olsen KM, Rupp ME, Bui TH, Forsung E, et al. Epidemiology and Predictors of Multidrug-Resistant Community-Acquired and Health Care-Associated Pneumonia. Antimicrob Agents Chemother (2014) 58(9):5262–8. doi: 10.1128/AAC.02582-14
133. Land SC, Tee AR. Hypoxia-Inducible Factor 1alpha Is Regulated by the Mammalian Target of Rapamycin (mTOR) via an mTOR Signaling Motif. J Biol Chem (2007) 282(28):20534–43. doi: 10.1074/jbc.M611782200
134. Finicle BT, Jayashankar V, Edinger AL. Nutrient Scavenging in Cancer. Nat Rev Cancer (2018) 18(10):619–33. doi: 10.1038/s41568-018-0048-x
135. Palm W, Thompson CB. Nutrient Acquisition Strategies of Mammalian Cells. Nature (2017) 546(7657):234–42. doi: 10.1038/nature22379
136. Kennedy KM, Scarbrough PM, Ribeiro A, Richardson R, Yuan H, Sonveaux P, et al. Catabolism of Exogenous Lactate Reveals It as a Legitimate Metabolic Substrate in Breast Cancer. PloS One (2013) 8(9):e75154. doi: 10.1371/journal.pone.0075154
137. Kimmelman AC, White E. Autophagy and Tumor Metabolism. Cell Metab (2017) 25(5):1037–43. doi: 10.1016/j.cmet.2017.04.004
138. Flynn AB, Schiemann WP. Autophagy in Breast Cancer Metastatic Dormancy: Tumor Suppressing or Tumor Promoting Functions? J Cancer Metastasis Treat (2019) 5:43. doi: 10.20517/2394-4722.2019.13
139. Huo Y, Cai H, Teplova I, Bowman-Colin C, Chen G, Price S, et al. Autophagy Opposes P53-Mediated Tumor Barrier to Facilitate Tumorigenesis in a Model of PALB2-Associated Hereditary Breast Cancer. Cancer Discov (2013) 3(8):894–907. doi: 10.1158/2159-8290.CD-13-0011
140. Levine B, Klionsky DJ. Development by Self-Digestion: Molecular Mechanisms and Biological Functions of Autophagy. Dev Cell (2004) 6(4):463–77. doi: 10.1016/S1534-5807(04)00099-1
141. Lipinski MM, Hoffman G, Ng A, Zhou W, Py BF, Hsu E, et al. A Genome-Wide siRNA Screen Reveals Multiple Mtorc1 Independent Signaling Pathways Regulating Autophagy Under Normal Nutritional Conditions. Dev Cell (2010) 18(6):1041–52. doi: 10.1016/j.devcel.2010.05.005
142. Casimiro MC, Di Sante G, Di Rocco A, Loro E, Pupo C, Pestell TG, et al. Cyclin D1 Restrains Oncogene-Induced Autophagy by Regulating the AMPK-LKB1 Signaling Axis. Cancer Res (2017) 77(13):3391–405. doi: 10.1158/0008-5472.CAN-16-0425
143. Zhang M, Di Martino JS, Bowman RL, Campbell NR, Baksh SC, Simon-Vermot T, et al. Adipocyte-Derived Lipids Mediate Melanoma Progression via FATP Proteins. Cancer Discov (2018) 8(8):1006–25. doi: 10.1158/2159-8290.CD-17-1371
144. Vitale I, Manic G, Coussens LM, Kroemer G, Galluzzi L. Macrophages and Metabolism in the Tumor Microenvironment. Cell Metab (2019) 30(1):36–50. doi: 10.1016/j.cmet.2019.06.001
145. Tan Z, Xie N, Banerjee S, Cui H, Fu M, Thannickal VJ, et al. The Monocarboxylate Transporter 4 Is Required for Glycolytic Reprogramming and Inflammatory Response in Macrophages. J Biol Chem (2015) 290(1):46–55. doi: 10.1074/jbc.M114.603589
146. Mayers JR, Torrence ME, Danai LV, Papagiannakopoulos T, Davidson SM, Bauer MR, et al. Tissue of Origin Dictates Branched-Chain Amino Acid Metabolism in Mutant Kras-Driven Cancers. Science (2016) 353(6304):1161–5. doi: 10.1126/science.aaf5171
147. Schug SA, Palmer GM, Scott DA, Halliwell R, Trinca J. Acute Pain Management: Scientific Evidence, Fourth Edition, 2015. Med J Aust (2016) 204(8):315–7. doi: 10.5694/mja16.00133
148. Kamphorst JJ, Cross JR, Fan J, de Stanchina E, Mathew R, White EP, et al. Hypoxic and Ras-Transformed Cells Support Growth by Scavenging Unsaturated Fatty Acids From Lysophospholipids. Proc Natl Acad Sci USA (2013) 110(22):8882–7. doi: 10.1073/pnas.1307237110
149. Commisso C, Davidson SM, Soydaner-Azeloglu RG, Parker SJ, Kamphorst JJ, Hackett S, et al. Macropinocytosis of Protein Is an Amino Acid Supply Route in Ras-Transformed Cells. Nature (2013) 497(7451):633–7. doi: 10.1038/nature12138
150. Rajeshkumar NV, Dutta P, Yabuuchi S, de Wilde RF, Martinez GV, Le A, et al. Therapeutic Targeting of the Warburg Effect in Pancreatic Cancer Relies on an Absence of P53 Function. Cancer Res (2015) 75(16):3355–64. doi: 10.1158/0008-5472.CAN-15-0108
151. Yoo DY, Barros SA, Brown GC, Rabot C, Bar-Sagi D, Arora PS. Macropinocytosis as a Key Determinant of Peptidomimetic Uptake in Cancer Cells. J Am Chem Soc (2020) 142(34):14461–71. doi: 10.1021/jacs.0c02109
152. Recouvreux MV, Commisso C. Macropinocytosis: A Metabolic Adaptation to Nutrient Stress in Cancer. Front Endocrinol (Lausanne) (2017) 8:261. doi: 10.3389/fendo.2017.00261
153. Gilchrist KW, Gray R, Fowble B, Tormey DC, Taylor SGT. Tumor Necrosis Is a Prognostic Predictor for Early Recurrence and Death in Lymph Node-Positive Breast Cancer: A 10-Year Follow-Up Study of 728 Eastern Cooperative Oncology Group Patients. J Clin Oncol (1993) 11(10):1929–35. doi: 10.1200/JCO.1993.11.10.1929
154. Walker RA. The Complexities of Breast Cancer Desmoplasia. Breast Cancer Res (2001) 3(3):143–5. doi: 10.1186/bcr287
156. Pavlides S, Tsirigos A, Migneco G, Whitaker-Menezes D, Chiavarina B, Flomenberg N, et al. The Autophagic Tumor Stroma Model of Cancer: Role of Oxidative Stress and Ketone Production in Fueling Tumor Cell Metabolism. Cell Cycle (2010) 9(17):3485–505. doi: 10.4161/cc.9.17.12721
157. Martinez-Outschoorn UE, Balliet RM, Rivadeneira DB, Chiavarina B, Pavlides S, Wang C, et al. Oxidative Stress in Cancer Associated Fibroblasts Drives Tumor-Stroma Co-Evolution: A New Paradigm for Understanding Tumor Metabolism, the Field Effect and Genomic Instability in Cancer Cells. Cell Cycle (2010) 9(16):3256–76. doi: 10.4161/cc.9.16.12553
158. Martinez-Outschoorn UE, Trimmer C, Lin Z, Whitaker-Menezes D, Chiavarina B, Zhou J, et al. Autophagy in Cancer Associated Fibroblasts Promotes Tumor Cell Survival: Role of Hypoxia, HIF1 Induction and NFkappaB Activation in the Tumor Stromal Microenvironment. Cell Cycle (2010) 9(17):3515–33. doi: 10.4161/cc.9.17.12928
159. Chiavarina B, Whitaker-Menezes D, Migneco G, Martinez-Outschoorn UE, Pavlides S, Howell A, et al. HIF1-Alpha Functions as a Tumor Promoter in Cancer Associated Fibroblasts, and as a Tumor Suppressor in Breast Cancer Cells: Autophagy Drives Compartment-Specific Oncogenesis. Cell Cycle (2010) 9(17):3534–51. doi: 10.4161/cc.9.17.12908
160. Martinez-Outschoorn UE, Curry JM, Ko YH, Lin Z, Tuluc M, Cognetti D, et al. Oncogenes and Inflammation Rewire Host Energy Metabolism in the Tumor Microenvironment: RAS and NFkappaB Target Stromal MCT4. Cell Cycle (2013) 12(16):2580–97. doi: 10.4161/cc.25510
161. Salem AF, Whitaker-Menezes D, Lin Z, Martinez-Outschoorn UE, Tanowitz HB, Al-Zoubi MS, et al. Two-Compartment Tumor Metabolism: Autophagy in the Tumor Microenvironment and Oxidative Mitochondrial Metabolism (OXPHOS) in Cancer Cells. Cell Cycle (2012) 11(13):2545–56. doi: 10.4161/cc.20920
162. Sotgia F, Whitaker-Menezes D, Martinez-Outschoorn UE, Flomenberg N, Birbe RC, Witkiewicz AK, et al. Mitochondrial Metabolism in Cancer Metastasis: Visualizing Tumor Cell Mitochondria and the "Reverse Warburg Effect" in Positive Lymph Node Tissue. Cell Cycle (2012) 11(7):1445–54. doi: 10.4161/cc.19841
163. Pavlides S, Tsirigos A, Vera I, Flomenberg N, Frank PG, Casimiro MC, et al. Loss of Stromal Caveolin-1 Leads to Oxidative Stress, Mimics Hypoxia and Drives Inflammation in the Tumor Microenvironment, Conferring the "Reverse Warburg Effect": A Transcriptional Informatics Analysis With Validation. Cell Cycle (2010) 9(11):2201–19. doi: 10.4161/cc.9.11.11848
164. Bonuccelli G, Whitaker-Menezes D, Castello-Cros R, Pavlides S, Pestell RG, Fatatis A, et al. The Reverse Warburg Effect: Glycolysis Inhibitors Prevent the Tumor Promoting Effects of Caveolin-1 Deficient Cancer Associated Fibroblasts. Cell Cycle (2010) 9(10):1960–71. doi: 10.4161/cc.9.10.11601
165. Pavlides S, Tsirigos A, Vera I, Flomenberg N, Frank PG, Casimiro MC, et al. Transcriptional Evidence for the "Reverse Warburg Effect" in Human Breast Cancer Tumor Stroma and Metastasis: Similarities With Oxidative Stress, Inflammation, Alzheimer’s Disease, and "Neuron-Glia Metabolic Coupling". Aging (Albany NY) (2010) 2(4):185–99. doi: 10.18632/aging.100134
166. Del Galdo F, Lisanti MP, Jimenez SA. Caveolin-1, Transforming Growth Factor-Beta Receptor Internalization, and the Pathogenesis of Systemic Sclerosis. Curr Opin Rheumatol (2008) 20(6):713–9. doi: 10.1097/BOR.0b013e3283103d27
167. Del Galdo F, Sotgia F, de Almeida CJ, Jasmin JF, Musick M, Lisanti MP, et al. Decreased Expression of Caveolin 1 in Patients With Systemic Sclerosis: Crucial Role in the Pathogenesis of Tissue Fibrosis. Arthritis Rheum (2008) 58(9):2854–65. doi: 10.1002/art.23791
168. Yang L, Achreja A, Yeung TL, Mangala LS, Jiang D, Han C, et al. Targeting Stromal Glutamine Synthetase in Tumors Disrupts Tumor Microenvironment-Regulated Cancer Cell Growth. Cell Metab (2016) 24(5):685–700. doi: 10.1016/j.cmet.2016.10.011
169. Zhu Z, Achreja A, Meurs N, Animasahun O, Owen S, Mittal A, et al. Tumour-Reprogrammed Stromal BCAT1 Fuels Branched-Chain Ketoacid Dependency in Stromal-Rich PDAC Tumours. Nat Metab (2020) 2(8):775–92. doi: 10.1038/s42255-020-0226-5
170. Biswas SK, Mantovani A. Macrophage Plasticity and Interaction With Lymphocyte Subsets: Cancer as a Paradigm. Nat Immunol (2010) 11(10):889–96. doi: 10.1038/ni.1937
171. Ye H, Zhou Q, Zheng S, Li G, Lin Q, Wei L, et al. Tumor-Associated Macrophages Promote Progression and the Warburg Effect via CCL18/NF-Kb/VCAM-1 Pathway in Pancreatic Ductal Adenocarcinoma. Cell Death Dis (2018) 9(5):453. doi: 10.1038/s41419-018-0486-0
172. Palmieri EM, Menga A, Martin-Perez R, Quinto A, Riera-Domingo C, De Tullio G, et al. Pharmacologic or Genetic Targeting of Glutamine Synthetase Skews Macrophages Toward an M1-Like Phenotype and Inhibits Tumor Metastasis. Cell Rep (2017) 20(7):1654–66. doi: 10.1016/j.celrep.2017.07.054
173. Onuchic V, Hartmaier RJ, Boone DN, Samuels ML, Patel RY, White WM, et al. Epigenomic Deconvolution of Breast Tumors Reveals Metabolic Coupling Between Constituent Cell Types. Cell Rep (2016) 17(8):2075–86. doi: 10.1016/j.celrep.2016.10.057
174. Au-Yeung CL, Yeung TL, Achreja A, Zhao H, Yip KP, Kwan SY, et al. ITLN1 Modulates Invasive Potential and Metabolic Reprogramming of Ovarian Cancer Cells in Omental Microenvironment. Nat Commun (2020) 11(1):3546. doi: 10.1038/s41467-020-17383-2
175. Overholtzer M, Mailleux AA, Mouneimne G, Normand G, Schnitt SJ, King RW, et al. A Nonapoptotic Cell Death Process, Entosis, That Occurs by Cell-in-Cell Invasion. Cell (2007) 131(5):966–79. doi: 10.1016/j.cell.2007.10.040
176. Sivanand S, Vander Heiden MG. Transcriptional Activation of Macropinocytosis by the Hippo Pathway Following Nutrient Limitation. Genes Dev (2020) 34(19-20):1253–5. doi: 10.1101/gad.343632.120
177. Bryant DM, Kerr MC, Hammond LA, Joseph SR, Mostov KE, Teasdale RD, et al. EGF Induces Macropinocytosis and SNX1-Modulated Recycling of E-Cadherin. J Cell Sci (2007) 120(Pt 10):1818–28. doi: 10.1242/jcs.000653
178. Kapara A, Brunton V, Graham D, Faulds K. Investigation of Cellular Uptake Mechanism of Functionalised Gold Nanoparticles Into Breast Cancer Using SERS. Chem Sci (2020) 11(22):5819–29. doi: 10.1039/D0SC01255F
179. Kim SM, Nguyen TT, Ravi A, Kubiniok P, Finicle BT, Jayashankar V, et al. PTEN Deficiency and AMPK Activation Promote Nutrient Scavenging and Anabolism in Prostate Cancer Cells. Cancer Discov (2018) 8(7):866–83. doi: 10.1158/2159-8290.CD-17-1215
180. Palm W, Araki J, King B, DeMatteo RG, Thompson CB. Critical Role for PI3-Kinase in Regulating the Use of Proteins as an Amino Acid Source. Proc Natl Acad Sci USA (2017) 114(41):E8628–36. doi: 10.1073/pnas.1712726114
181. King B, Araki J, Palm W, Thompson CB. Yap/Taz Promote the Scavenging of Extracellular Nutrients Through Macropinocytosis. Genes Dev (2020) 34(19-20):1345–58. doi: 10.1101/gad.340661.120
182. Davidson SM, Jonas O, Keibler MA, Hou HW, Luengo A, Mayers JR, et al. Direct Evidence for Cancer-Cell-Autonomous Extracellular Protein Catabolism in Pancreatic Tumors. Nat Med (2017) 23(2):235–41. doi: 10.1038/nm.4256
183. Kamphorst JJ, Nofal M, Commisso C, Hackett SR, Lu W, Grabocka E, et al. Human Pancreatic Cancer Tumors Are Nutrient Poor and Tumor Cells Actively Scavenge Extracellular Protein. Cancer Res (2015) 75(3):544–53. doi: 10.1158/0008-5472.CAN-14-2211
184. Redelman-Sidi G, Binyamin A, Gaeta I, Palm W, Thompson CB, Romesser PB, et al. The Canonical Wnt Pathway Drives Macropinocytosis in Cancer. Cancer Res (2018) 78(16):4658–70. doi: 10.1158/0008-5472.CAN-17-3199
185. Zhao H, Yang L, Baddour J, Achreja A, Bernard V, Moss T, et al. Tumor Microenvironment Derived Exosomes Pleiotropically Modulate Cancer Cell Metabolism. Elife (2016) 5:e10250. doi: 10.7554/eLife.10250
186. Chajes V, Cambot M, Moreau K, Lenoir GM, Joulin V. Acetyl-CoA Carboxylase Alpha Is Essential to Breast Cancer Cell Survival. Cancer Res (2006) 66(10):5287–94. doi: 10.1158/0008-5472.CAN-05-1489
187. Menendez JA, Lupu R. Fatty Acid Synthase and the Lipogenic Phenotype in Cancer Pathogenesis. Nat Rev Cancer (2007) 7(10):763–77. doi: 10.1038/nrc2222
188. Santos CR, Schulze A. Lipid Metabolism in Cancer. FEBS J (2012) 279(15):2610–23. doi: 10.1111/j.1742-4658.2012.08644.x
189. Rohrig F, Schulze A. The Multifaceted Roles of Fatty Acid Synthesis in Cancer. Nat Rev Cancer (2016) 16(11):732–49. doi: 10.1038/nrc.2016.89
190. Migita T, Narita T, Nomura K, Miyagi E, Inazuka F, Matsuura M, et al. ATP Citrate Lyase: Activation and Therapeutic Implications in Non-Small Cell Lung Cancer. Cancer Res (2008) 68(20):8547–54. doi: 10.1158/0008-5472.CAN-08-1235
191. Papadaki I, Mylona E, Giannopoulou I, Markaki S, Keramopoulos A, Nakopoulou L. PPARgamma Expression in Breast Cancer: Clinical Value and Correlation With ERbeta. Histopathology (2005) 46(1):37–42. doi: 10.1111/j.1365-2559.2005.02056.x
192. Jiang Y, Zou L, Zhang C, He S, Cheng C, Xu J, et al. PPARgamma and Wnt/beta-Catenin Pathway in Human Breast Cancer: Expression Pattern, Molecular Interaction and Clinical/Prognostic Correlations. J Cancer Res Clin Oncol (2009) 135(11):1551–9. doi: 10.1007/s00432-009-0602-8
193. Abduljabbar R, Al-Kaabi MM, Negm OH, Jerjees D, Muftah AA, Mukherjee A, et al. Prognostic and Biological Significance of Peroxisome Proliferator-Activated Receptor-Gamma in Luminal Breast Cancer. Breast Cancer Res Treat (2015) 150(3):511–22. doi: 10.1007/s10549-015-3348-9
194. Chou FS, Wang PS, Kulp S, Pinzone JJ. Effects of Thiazolidinediones on Differentiation, Proliferation, and Apoptosis. Mol Cancer Res (2007) 5(6):523–30. doi: 10.1158/1541-7786.MCR-06-0278
195. Tachibana K, Yamasaki D, Ishimoto K, Doi T. The Role of PPARs in Cancer. PPAR Res (2008) 2008:102737. doi: 10.1155/2008/102737
196. Wang C, Fu M, D’Amico M, Albanese C, Zhou JN, Brownlee M, et al. Inhibition of Cellular Proliferation Through IkappaB Kinase-Independent and Peroxisome Proliferator-Activated Receptor Gamma-Dependent Repression of Cyclin D1. Mol Cell Biol (2001) 21(9):3057–70. doi: 10.1128/MCB.21.9.3057-3070.2001
197. Augimeri G, Giordano C, Gelsomino L, Plastina P, Barone I, Catalano S, et al. The Role of PPARgamma Ligands in Breast Cancer: From Basic Research to Clinical Studies. Cancers (Basel) (2020) 12(9):2623. doi: 10.3390/cancers12092623
198. Jiao X, Tian L, Zhang Z, Balcerek J, Kossenkov AV, Casimiro MC, et al. Ppargamma1 Facilitates ErbB2-Mammary Adenocarcinoma in Mice. Cancers (Basel) (2021) 13(9):2171. doi: 10.3390/cancers13092171
199. Wu ZH, Tang Y, Yu H, Li HD. The Role of Ferroptosis in Breast Cancer Patients: A Comprehensive Analysis. Cell Death Discov (2021) 7(1):93. doi: 10.1038/s41419-020-03329-5
200. Zhang Y, Shi J, Liu X, Feng L, Gong Z, Koppula P, et al. BAP1 Links Metabolic Regulation of Ferroptosis to Tumour Suppression. Nat Cell Biol (2018) 20(10):1181–92. doi: 10.1038/s41556-018-0178-0
201. Jiang L, Kon N, Li T, Wang SJ, Su T, Hibshoosh H, et al. Ferroptosis as a P53-Mediated Activity During Tumour Suppression. Nature (2015) 520(7545):57–62. doi: 10.1038/nature14344
202. Gao M, Yi J, Zhu J, Minikes AM, Monian P, Thompson CB, et al. Role of Mitochondria in Ferroptosis. Mol Cell (2019) 73(2):354–63.e3. doi: 10.1016/j.molcel.2018.10.042
203. Yi J, Zhu J, Wu J, Thompson CB, Jiang X. Oncogenic Activation of PI3K-AKT-mTOR Signaling Suppresses Ferroptosis via SREBP-Mediated Lipogenesis. Proc Natl Acad Sci USA (2020) 117(49):31189–97. doi: 10.1073/pnas.2017152117
204. Lu Z, Hunter T. Metabolic Kinases Moonlighting as Protein Kinases. Trends Biochem Sci (2018) 43(4):301–10. doi: 10.1016/j.tibs.2018.01.006
205. Kondoh H, Lleonart ME, Gil J, Wang J, Degan P, Peters G, et al. Glycolytic Enzymes can Modulate Cellular Life Span. Cancer Res (2005) 65(1):177–85.
206. Wang C, Pattabiraman N, Zhou JN, Fu M, Sakamaki T, Albanese C, et al. Cyclin D1 Repression of Peroxisome Proliferator-Activated Receptor Gamma Expression and Transactivation. Mol Cell Biol (2003) 23(17):6159–73. doi: 10.1128/MCB.23.17.6159-6173.2003
207. Icard P, Fournel L, Wu Z, Alifano M, Lincet H. Interconnection Between Metabolism and Cell Cycle in Cancer. Trends Biochem Sci (2019) 44(6):490–501. doi: 10.1016/j.tibs.2018.12.007
208. Zhang J, Wang C, Chen X, Takada M, Fan C, Zheng X, et al. EglN2 Associates With the NRF1-PGC1alpha Complex and Controls Mitochondrial Function in Breast Cancer. EMBO J (2015) 34(23):2953–70. doi: 10.15252/embj.201591437
209. Lee Y, Dominy JE, Choi YJ, Jurczak M, Tolliday N, Camporez JP, et al. Cyclin D1-Cdk4 Controls Glucose Metabolism Independently of Cell Cycle Progression. Nature (2014) 510(7506):547–51. doi: 10.1038/nature13267
210. Chen K, Jiao X, Di Rocco A, Shen D, Xu S, Ertel A, et al. Endogenous Cyclin D1 Promotes the Rate of Onset and Magnitude of Mitogenic Signaling via Akt1 Ser473 Phosphorylation. Cell Rep (2020) 32(11):108151. doi: 10.1016/j.celrep.2020.108151
211. Robey RB, Hay N. Is Akt the "Warburg Kinase"?-Akt-Energy Metabolism Interactions and Oncogenesis. Semin Cancer Biol (2009) 19(1):25–31. doi: 10.1016/j.semcancer.2008.11.010
212. Oermann EK, Wu J, Guan KL, Xiong Y. Alterations of Metabolic Genes and Metabolites in Cancer. Semin Cell Dev Biol (2012) 23(4):370–80. doi: 10.1016/j.semcdb.2012.01.013
213. Astuti D, Latif F, Dallol A, Dahia PL, Douglas F, George E, et al. Gene Mutations in the Succinate Dehydrogenase Subunit SDHB Cause Susceptibility to Familial Pheochromocytoma and to Familial Paraganglioma. Am J Hum Genet (2001) 69(1):49–54. doi: 10.1086/321282
214. Janeway KA, Kim SY, Lodish M, Nose V, Rustin P, Gaal J, et al. Defects in Succinate Dehydrogenase in Gastrointestinal Stromal Tumors Lacking KIT and PDGFRA Mutations. Proc Natl Acad Sci USA (2011) 108(1):314–8. doi: 10.1073/pnas.1009199108
215. Tomlinson IP, Alam NA, Rowan AJ, Barclay E, Jaeger EE, Kelsell D, et al. Germline Mutations in FH Predispose to Dominantly Inherited Uterine Fibroids, Skin Leiomyomata and Papillary Renal Cell Cancer. Nat Genet (2002) 30(4):406–10. doi: 10.1038/ng849
216. Baysal BE, Ferrell RE, Willett-Brozick JE, Lawrence EC, Myssiorek D, Bosch A, et al. Mutations in SDHD, A Mitochondrial Complex II Gene, in Hereditary Paraganglioma. Science (2000) 287(5454):848–51. doi: 10.1126/science.287.5454.848
217. Yang M, Pollard PJ. Succinate: A New Epigenetic Hacker. Cancer Cell (2013) 23(6):709–11. doi: 10.1016/j.ccr.2013.05.015
218. Adam TC, Schmitt RJ, Holbrook SJ, Brooks AJ, Edmunds PJ, Carpenter RC, et al. Herbivory, Connectivity, and Ecosystem Resilience: Response of a Coral Reef to a Large-Scale Perturbation. PloS One (2011) 6(8):e23717. doi: 10.1371/journal.pone.0023717
219. Li H, Ning S, Ghandi M, Kryukov GV, Gopal S, Deik A, et al. The Landscape of Cancer Cell Line Metabolism. Nat Med (2019) 25(5):850–60. doi: 10.1038/s41591-019-0404-8
220. Nusinow DP, Szpyt J, Ghandi M, Rose CM, McDonald ER 3rd, Kalocsay M, et al. Quantitative Proteomics of the Cancer Cell Line Encyclopedia. Cell (2020) 180(2):387–402.e16. doi: 10.1016/j.cell.2019.12.023
221. Kroger C, Afeyan A, Mraz J, Eaton EN, Reinhardt F, Khodor YL, et al. Acquisition of a Hybrid E/M State Is Essential for Tumorigenicity of Basal Breast Cancer Cells. Proc Natl Acad Sci USA (2019) 116(15):7353–62. doi: 10.1073/pnas.1812876116
222. Skrypek N, Goossens S, De Smedt E, Vandamme N, Berx G. Epithelial-To-Mesenchymal Transition: Epigenetic Reprogramming Driving Cellular Plasticity. Trends Genet (2017) 33(12):943–59. doi: 10.1016/j.tig.2017.08.004
223. Georgakopoulos-Soares I, Chartoumpekis DV, Kyriazopoulou V, Zaravinos A. and Metabolic Pathways in Cancer. Front Oncol (2020) 10:499. doi: 10.3389/fonc.2020.00499
224. Sciacovelli M, Frezza C. Metabolic Reprogramming and Epithelial-to-Mesenchymal Transition in Cancer. FEBS J (2017) 284(19):3132–44. doi: 10.1111/febs.14090
225. Lunetti P, Di Giacomo M, Vergara D, De Domenico S, Maffia M, Zara V, et al. Metabolic Reprogramming in Breast Cancer Results in Distinct Mitochondrial Bioenergetics Between Luminal and Basal Subtypes. FEBS J (2019) 286(4):688–709. doi: 10.1111/febs.14756
226. Xiao Z, Liu S, Ai F, Chen X, Li X, Liu R, et al. SDHB Downregulation Facilitates the Proliferation and Invasion of Colorectal Cancer Through AMPK Functions Excluding Those Involved in the Modulation of Aerobic Glycolysis. Exp Ther Med (2018) 15(1):864–72. doi: 10.3892/etm.2017.5482
227. Wang H, Chen Y, Wu G. SDHB Deficiency Promotes TGFbeta-Mediated Invasion and Metastasis of Colorectal Cancer Through Transcriptional Repression Complex SNAIL1-Smad3/4. Transl Oncol (2016) 9(6):512–20. doi: 10.1016/j.tranon.2016.09.009
228. Aspuria PP, Lunt SY, Varemo L, Vergnes L, Gozo M, Beach JA, et al. Succinate Dehydrogenase Inhibition Leads to Epithelial-Mesenchymal Transition and Reprogrammed Carbon Metabolism. Cancer Metab (2014) 2:21. doi: 10.1186/2049-3002-2-21
229. Vega S, Morales AV, Ocana OH, Valdes F, Fabregat I, Nieto MA. Snail Blocks the Cell Cycle and Confers Resistance to Cell Death. Genes Dev (2004) 18(10):1131–43. doi: 10.1101/gad.294104
230. Morikawa M, Derynck R, Miyazono K. TGF-Beta and the TGF-Beta Family: Context-Dependent Roles in Cell and Tissue Physiology. Cold Spring Harb Perspect Biol (2016) 8(5):a021873. doi: 10.1101/cshperspect.a021873
231. Liu M, Casimiro MC, Wang C, Shirley LA, Jiao X, Katiyar S, et al. P21cip1 Attenuates Ras- and C-Myc-Dependent Breast Tumor Epithelial Mesenchymal Transition and Cancer Stem Cell-Like Gene Expression In Vivo. Proc Natl Acad Sci USA (2009) 106(45):19035–9. doi: 10.1073/pnas.0910009106
232. Ju X, Casimiro MC, Gormley M, Meng H, Jiao X, Katiyar S, et al. Identification of a Cyclin D1 Network in Prostate Cancer That Antagonizes Epithelial-Mesenchymal Restraint. Cancer Res (2014) 74(2):508–19. doi: 10.1158/0008-5472.CAN-13-1313
233. Jia D, Park JH, Kaur H, Jung KH, Yang S, Tripathi S, et al. Towards Decoding the Coupled Decision-Making of Metabolism and Epithelial-to-Mesenchymal Transition in Cancer. Br J Cancer (2021) 124(12):1902–11. doi: 10.1038/s41416-021-01385-y
234. Letouze E, Martinelli C, Loriot C, Burnichon N, Abermil N, Ottolenghi C, et al. SDH Mutations Establish a Hypermethylator Phenotype in Paraganglioma. Cancer Cell (2013) 23(6):739–52. doi: 10.1016/j.ccr.2013.04.018
235. Wu GA, Prochnik S, Jenkins J, Salse J, Hellsten U, Murat F, et al. Sequencing of Diverse Mandarin, Pummelo and Orange Genomes Reveals Complex History of Admixture During Citrus Domestication. Nat Biotechnol (2014) 32(7):656–62. doi: 10.1038/nbt.2906
236. Wu K, Chen K, Wang C, Jiao X, Wang L, Zhou J, et al. Cell Fate Factor DACH1 Represses YB-1-Mediated Oncogenic Transcription and Translation. Cancer Res (2014) 74(3):829–39. doi: 10.1158/0008-5472.CAN-13-2466
237. Popov VM, Wu K, Zhou J, Powell MJ, Mardon G, Wang C, et al. The Dachshund Gene in Development and Hormone-Responsive Tumorigenesis. Trends Endocrinol Metab (2010) 21(1):41–9. doi: 10.1016/j.tem.2009.08.002
238. Dentro SC, Leshchiner I, Haase K, Tarabichi M, Wintersinger J, Deshwar AG, et al. Characterizing Genetic Intra-Tumor Heterogeneity Across 2,658 Human Cancer Genomes. Cell (2021) 184(8):2239–54.e39. doi: 10.1016/j.cell.2021.03.009
239. Abascal F, Harvey LMR, Mitchell E, Lawson ARJ, Lensing SV, Ellis P, et al. Somatic Mutation Landscapes at Single-Molecule Resolution. Nature (2021) 593(7859):405–10. doi: 10.1038/s41586-021-03477-4
240. Sinkala M, Mulder N, Patrick Martin D. Metabolic Gene Alterations Impact the Clinical Aggressiveness and Drug Responses of 32 Human Cancers. Commun Biol (2019) 2:414. doi: 10.1038/s42003-019-0666-1
241. Marjon K, Cameron MJ, Quang P, Clasquin MF, Mandley E, Kunii K, et al. MTAP Deletions in Cancer Create Vulnerability to Targeting of the MAT2A/PRMT5/RIOK1 Axis. Cell Rep (2016) 15(3):574–87. doi: 10.1016/j.celrep.2016.03.043
242. Rheinbay E, Nielsen MM, Abascal F, Wala JA, Shapira O, Tiao G, et al. Analyses of Non-Coding Somatic Drivers in 2,658 Cancer Whole Genomes. Nature (2020) 578(7793):102–11. doi: 10.1038/s41586-020-1965-x
243. Yates LR, Knappskog S, Wedge D, Farmery JHR, Gonzalez S, Martincorena I, et al. Genomic Evolution of Breast Cancer Metastasis and Relapse. Cancer Cell (2017) 32(2):169–84.e7. doi: 10.1016/j.ccell.2017.07.005
Keywords: breast cancer, metabolism, Warburg effect, aerobic glycolysis, reverse Warburg effect, epigenetics, PPAR-γ, Cyclin D1
Citation: Patra S, Elahi N, Armorer A, Arunachalam S, Omala J, Hamid I, Ashton AW, Joyce D, Jiao X and Pestell RG (2021) Mechanisms Governing Metabolic Heterogeneity in Breast Cancer and Other Tumors. Front. Oncol. 11:700629. doi: 10.3389/fonc.2021.700629
Received: 28 April 2021; Accepted: 30 August 2021;
Published: 23 September 2021.
Edited by:
Yong Teng, Emory University, United StatesReviewed by:
Jaromir Gumulec, Masaryk University, CzechiaAbhinav Achreja, University of Michigan, United States
Copyright © 2021 Patra, Elahi, Armorer, Arunachalam, Omala, Hamid, Ashton, Joyce, Jiao and Pestell. This is an open-access article distributed under the terms of the Creative Commons Attribution License (CC BY). The use, distribution or reproduction in other forums is permitted, provided the original author(s) and the copyright owner(s) are credited and that the original publication in this journal is cited, in accordance with accepted academic practice. No use, distribution or reproduction is permitted which does not comply with these terms.
*Correspondence: Xuanmao Jiao, xuanmao.jiao@bblumberg.org
†These authors have contributed equally to this work and share first authorship