- 1Department of Oncology, The First Affiliated Hospital of Nanchang University, Nanchang, China
- 2Department of Burn, The First Affiliated Hospital of Nanchang University, Nanchang, China
- 3Department of Pathology, The First Affiliated Hospital of Nanchang University, Nanchang, China
Purpose: Multiple factors have been shown to be tied to the prognosis of individuals with parotid cancer (PC); however, there are limited numbers of reliable as well as straightforward tools available for clinical estimation of individualized mortality. Here, a competing risk nomogram was established to assess the risk of cancer-specific deaths (CSD) in individuals with PC.
Methods: Data of PC patients analyzed in this work were retrieved from the Surveillance, Epidemiology, and End Results (SEER) data repository and the First Affiliated Hospital of Nanchang University (China). Univariate Lasso regression coupled with multivariate Cox assessments were adopted to explore the predictive factors influencing CSD. The cumulative incidence function (CIF) coupled with the Fine-Gray proportional hazards model was employed to determine the risk indicators tied to CSD as per the univariate, as well as multivariate analyses conducted in the R software. Finally, we created and validated a nomogram to forecast the 3- and 5-year CSD likelihood.
Results: Overall, 1,467 PC patients were identified from the SEER data repository, with the 3- and 5-year CSD CIF after diagnosis being 21.4% and 24.1%, respectively. The univariate along with the Lasso regression data revealed that nine independent risk factors were tied to CSD in the test dataset (n = 1,035) retrieved from the SEER data repository. Additionally, multivariate data of Fine-Gray proportional subdistribution hazards model illustrated that N stage, Age, T stage, Histologic, M stage, grade, surgery, and radiation were independent risk factors influencing CSD in an individual with PC in the test dataset (p < 0.05). Based on optimization performed using the Bayesian information criterion (BIC), six variables were incorporated in the prognostic nomogram. In the internal SEER data repository verification dataset (n = 432) and the external medical center verification dataset (n = 473), our nomogram was well calibrated and exhibited considerable estimation efficiency.
Conclusion: The competing risk nomogram presented here can be used for assessing cancer-specific mortality in PC patients.
Introduction
Parotid cancers are responsible for about 70% of malignant tumors in the salivary gland, characterized by pathological/histological differences (1, 2). The present crude incidence of primary cancers of the salivary is 0.9 per 100,000, of which approximately 80% of these cases arise in the parotid salivary gland (3). The prognosis of individuals with PC differs significantly, with some clinical features considerably influencing the disease-free survival (DSF) along with (OS) overall survival. Its mortality rate has remained the same over the past decade, with a 5-year OS of approximately 60% dependent on the histological type, as well as the anatomical site, and specifically the treatment option (4, 5). Presently, the AJCC staging criteria are the main approach to estimating prognosis in individuals with parotid cancer. Nonetheless, remarkable differences in the clinical outcomes among individuals with parotid cancer at the same stage receiving similar treatments have been reported (6). This demonstrates that the AJCC staging method is far from being a perfect system for making a prognosis, as well as treatment decisions. Such a method is only ideal for estimating distant metastasis (M stage), tumor size along with extension (T stage), and lymph node (LN) involvement without taking into account other factors, e.g., histological types, demographical factors, and treatments. Recently, numerous researches have documented the prognosis of common head and neck cancer, such as laryngeal carcinoma (7) and nasopharyngeal carcinoma (8), but few have addressed PC. The survival of individuals with PC has been investigated by other research groups; nonetheless, most investigations are from single institutions lacking the assessment of CSD risk factors. Hence, it is pivotal to conduct more research on PC prognosis.
Surveillance, Epidemiology, and End Results (SEER), a data repository based on populations, represents an estimated 28% of the US population. Therefore, the datasets retrieved from the SEER data repository provide adequate cases of creating prognostic models, particularly for rare cancers (9). The data of PC cases utilized in this research were retrieved from the SEER data repository, which can guarantee the authenticity and sufficiency of the data. Overall, cancer patients frequently experience more than two events, but only one event occurs (10). The events excluding that of interest are termed as competing risks. In traditional survival assessment, censoring of competing risks is done and can be enhanced through competing risk assessment.
A nomogram visualizes the linear prognosis of a disease (11). Each characteristic value on the nomogram plot signifies a score, with the total score mapping the survival estimate. In many studies, survival outcomes are determined using the Kaplan-Meier approach coupled with the Cox proportional hazard, although the population-based approaches are also applied (12, 13). Nonetheless, a significance of the studies analyzed the OS along with the cancer-specific survival assessment, while neglecting the role played by other competing causes of death in the prognosis of nonmetastatic PC. Prolonged survival is dependent on the competing risks of death to a remarkable degree. The competing risk should be considered when forecasting survival outcomes.
In this work, we aimed to construct a competing risk nomogram using data retrieved from the SEER data repository to assist in predicting death linked to PC. The nomogram will help clinicians in making patient-specific decisions in treating PC as well as precise predictions of disease outcomes.
Material and Methods
SEER Database Patients
We retrospectively analyzed data from the SEER data repository spanning from 1992 to 2017. The SEER data repository (https://seer.cancer.gov/) is publicly accessible.
A selection of SEER 13 Regs Custom Data (with additional treatment fields) uploaded in November 2019 (1992–2017 varying) was done. All subjects with primary PC diagnosis (site recode NM7/CS v0204+ Schema of “parotid gland” along with the ICD-O-3 behavior recode of “malignant”) were enrolled in the analysis. Exclusion criteria consisted of PC individuals who were less than 5 years old, those with a survival time of ≤1 month, and patients lacking complete data or a pathological diagnosis.
Our Medical Center Patients
We collected data from 473 individuals with PC who were admitted to the First Affiliated Hospital of Nanchang University (China) spanning from 2006 to 2017. The subjects confirmed by pathology had no history of other malignant tumors. The Ethics Committee of the First Affiliated Hospital of Nanchang University approved this retrospective cohort study. The principles of the Helsinki Declaration were followed with regards to data confidentiality.
Variable Selection
Factors including age, T stage, AJCC stage, N stage, race, M stage, sex, histological type, surgery, radiation, grade, follow-up time, and survival outcomes were retrieved from the SEER data repository. We adopted the X-tile software to determine the optimal threshold values. The age of the subjects at diagnosis was classified into two classes, i.e., ≥70 and <70 years. The AJCC stage was employed as the staging approach. The ICD-O-3 codes were adopted to categorize the PC histological type into two classes, i.e., mucoepidermoid carcinoma (MEC) and none MEC (squamous cell carcinoma, myoepithelial carcinoma, polymorphous adenocarcinoma NOS, adenoid cystic carcinoma, and acinic cell carcinoma, among others) as per the WHO categorization approach. Cancer-distinct survival constituted the primary end-point and included the time beginning from cancer diagnosis to death emerging from PC or a censored risk. Deaths linked to accidents or diseases excluding PC constituted the competition risks.
Statistical Analyses
We conducted all the analysis using R (V.4.0.4: survivial, crrstep, cmprsk, pec, rms, riskRegression, mstate, and foreign packages) to perform the statistical analyses. Two-sided and p < 0.05 defined statistical significance. Firstly, we computed the CIF for 3 to 5 years. We further carried out subgroup analysis between diverse subgroups, and matching CIF curves were created for these variables. Gray’s test was implemented to determine the drastic differences in values of CIF among subgroups. Secondly, patients from the SEER data repository were split at random into a test data set along with the verification data set, with a 7:3 ratio. Patients recruited from our hospital served as the external verification dataset. The test dataset was employed to create the prediction nomogram for estimating of CSD, whereas the verification datasets were employed to validate the efficiency of our nomogram. Univariate coupled with the Lasso Cox regression model assessments were implemented to explore the independent predictors of CSDs in the test dataset. All different variables were further identified by AIC and BIC models. The Fine-Gray proportional hazards model was adopted to develop the competing risk nomogram.
The performance of our nomogram was first explored in the test cohort and subsequently in the verification cohorts with respect to the C-index, AUC, and the calibration curve. The estimation capacity of our nomogram was quantified with the C-index and ranged from 0.5 to 1.0, representing a random probability from indicating no discrimination to indicating optimal discrimination (14). The AUC reflects the overall estimation value for all the thresholds (15), with a perfect prediction value exhibiting an AUC of 1.0. We adopted decision curve analysis (DCA) to determine the clinical net benefit of different probability thresholds for a possible clinical consequence (16) and explored the nomogram efficiency in contrast with the AJCC staging approach visually.
Results
Baseline Features of Participants
As illustrated in Figure 1, we initially retrieved 2,304 patient cases from the SEER data repository. Strict screening was carried out, yielding 1,467 patient cases with PC who were recruited in the study. The subjects’ median age was 50.7 years (5–85) at diagnosis with males accounting for 43.6%. Most participants were white (n = 1,142, 77.8%). Of the 1,467 PC cases, 621 (42.3%) were MEC, consisting of 599 (40.8%) incidences of moderate differentiation. Besides, stage I constituted the most frequent tumor stage (n = 454, 30.9%), followed by stages IV (n = 420, 28.6%), II (n = 334, 22.8%), and III (n = 259, 17.7%). Most PC subjects were classified as T1 (35.0%), followed by T2 (28.9%), T3 (19.9%), and T4 (16.2%). More than half of the PC subjects lacked lymph node (LN) metastases (N0, 72.1%), and most patients did not exhibit distant metastases (M0, 96.4%). A significant number of the PC individuals were treated using surgical therapy (n = 1,375, 93.7%) and 35.5% of the patients received radiotherapy. The detailed demographic, as well as clinical characteristics of the recruited participants, are given in Table 1.
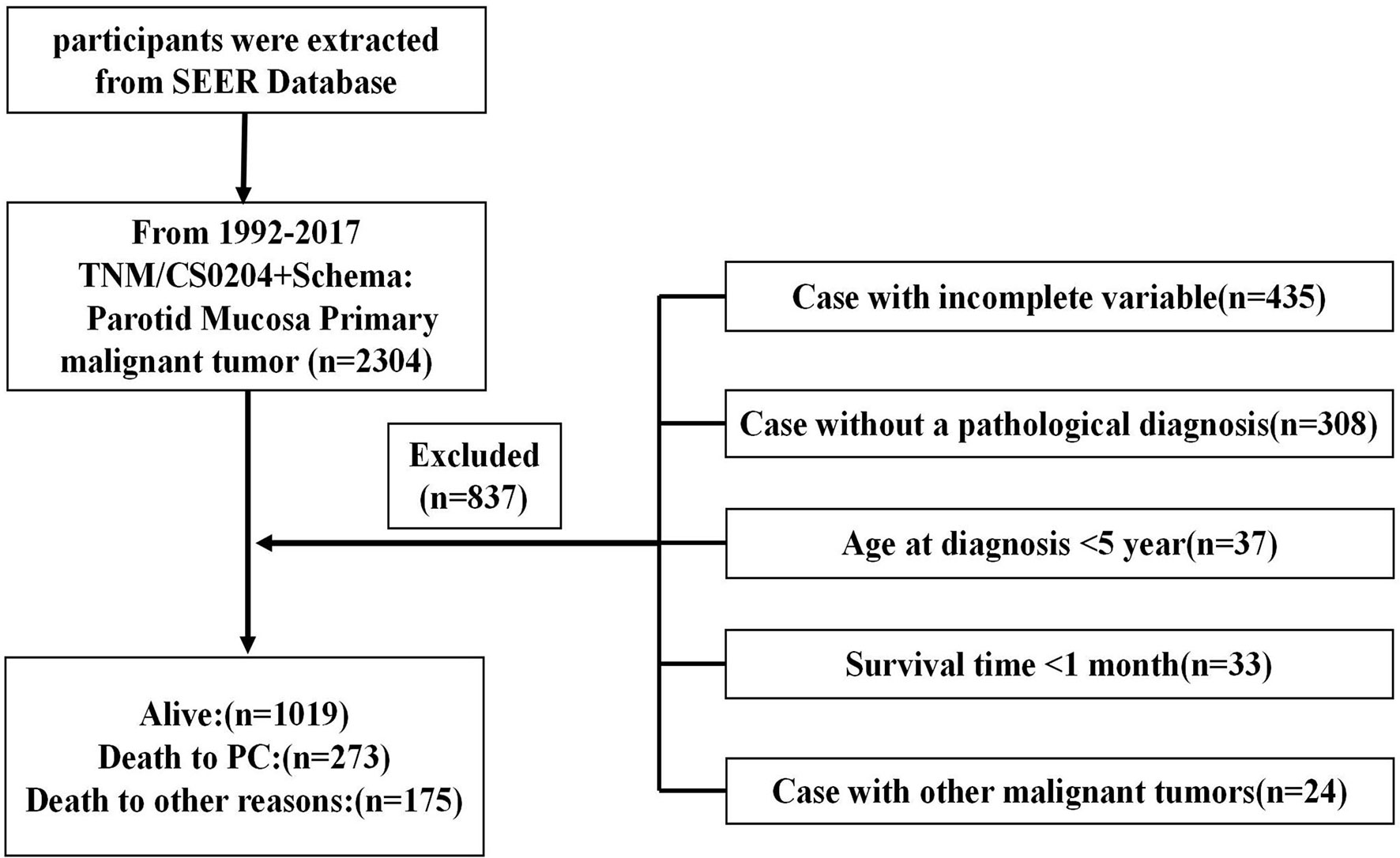
Figure 1 Flow diagram illustrating recruitment of patients. SEER, Surveillance, Epidemiology, and End Results; PC, parotid cancer.
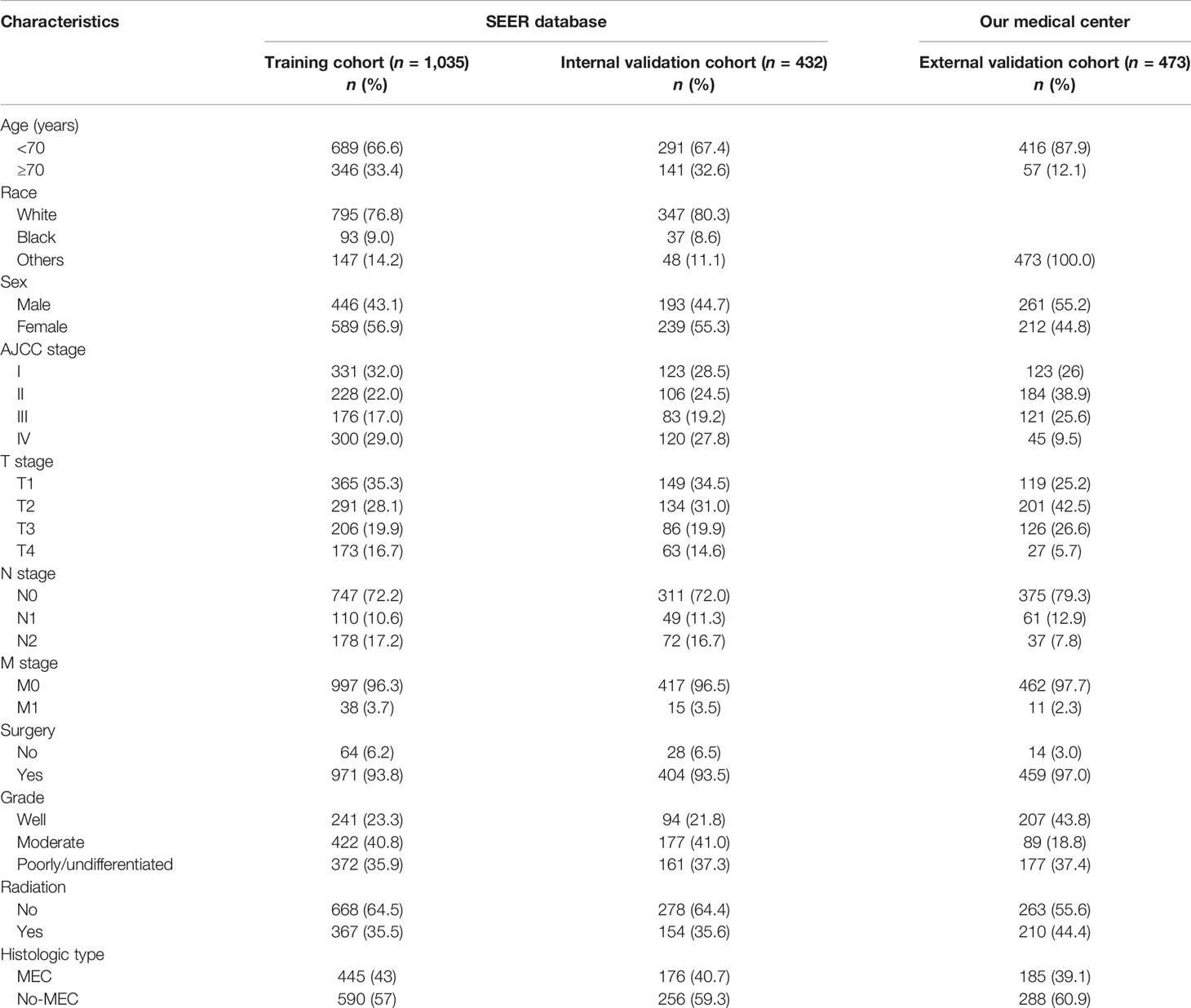
Table 1 Basic characteristics of parotid cancer patients in the training, internal validation, and external validation cohorts.
CIF Survival Analysis
The median follow-up time was 43 months (1–95) based on the results of the nomogram (Table 2). In total, 448 patients (30.5%) had died by the end of follow-up, among which 273 (60.9%) patients died from cancer and 175 (39.1%) patients died from other causes. The 3- and 5-year CSD CIF was 21.4% and 24.1%, respectively. The CIF subgroup assessment data exhibited that high CSD majorly occurred in individuals with PC aged ≥70 years (Figure 2A) with advanced AJCC stage (Figure 2B), advanced T stage (Figure 2C), advanced N stage (Figure 2D), along with M1 stage (Figure 2E), as well as the patients who did not undergo surgical treatment (Figure 2F), radiation treatment (Figure 2H), and with undifferentiated/poor grade (Figure 2G) and MEC (Figure 2I). Nevertheless, no considerable difference in CSD was reported and race and sex subgroup assessments (Figures 2J, K).
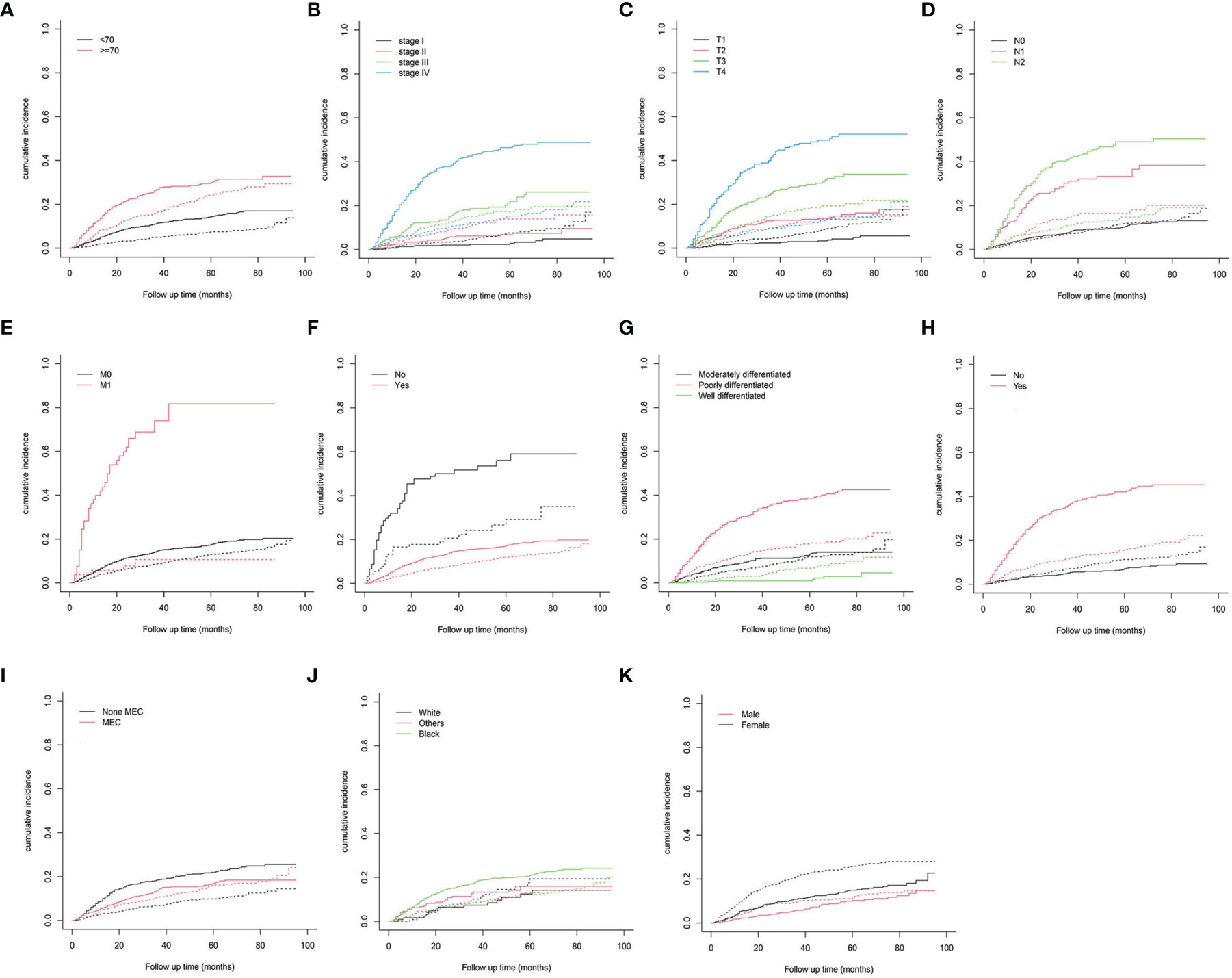
Figure 2 Cumulative incidence predictions of CSD in parotid carcinoma. (A) Age; (B) AJCC stage; (C) T stage; (D) N stage; (E) M stage; (F) surgery; (G) grade; (H) radiation; (I) histologic type; (J) race; (K) sex. Solid line designates CSD; dotted line designates other causes of death. AJCC, American Joint Committee on Cancer; MEC, mucoepidermoid carcinoma.
Nomogram Development
As illustrated in Table 1, the patients from the SEER data repository were stratified at random into a test group (n = 1,035) and a verification group (n = 432) at a ratio of 7:3. We implemented univariate and Lasso Cox assessments in the test dataset to determine independent predictors affecting CDS. A total of nine predictive factors (AJCC stage, surgery, age, T stage, M stage, grade, N stage, histologic, and radiation) were incorporated in the predictive model (Figures 3A, B). All variables were further identified by the multivariate assessment of Fine-Gray proportional subdistribution hazards model. As per the AIC assessment, age, T stage, surgery, N stage, histologic, M stage, grade, as well as radiation were independent predictors influencing cancer-distinct death in individuals with PC of the test cohort (p < 0.05). Following the optimization of the nomogram as per the BIC, we finally incorporated six variables in the estimation model (Table 3). A competing event nomogram was created to assess the 3- and 5-year chances of CSD by using these variables (Figure 4). Each patient’s likelihood of death caused by PC at various time points was computed via this model through the addition of the scores of each of the integrated variables.
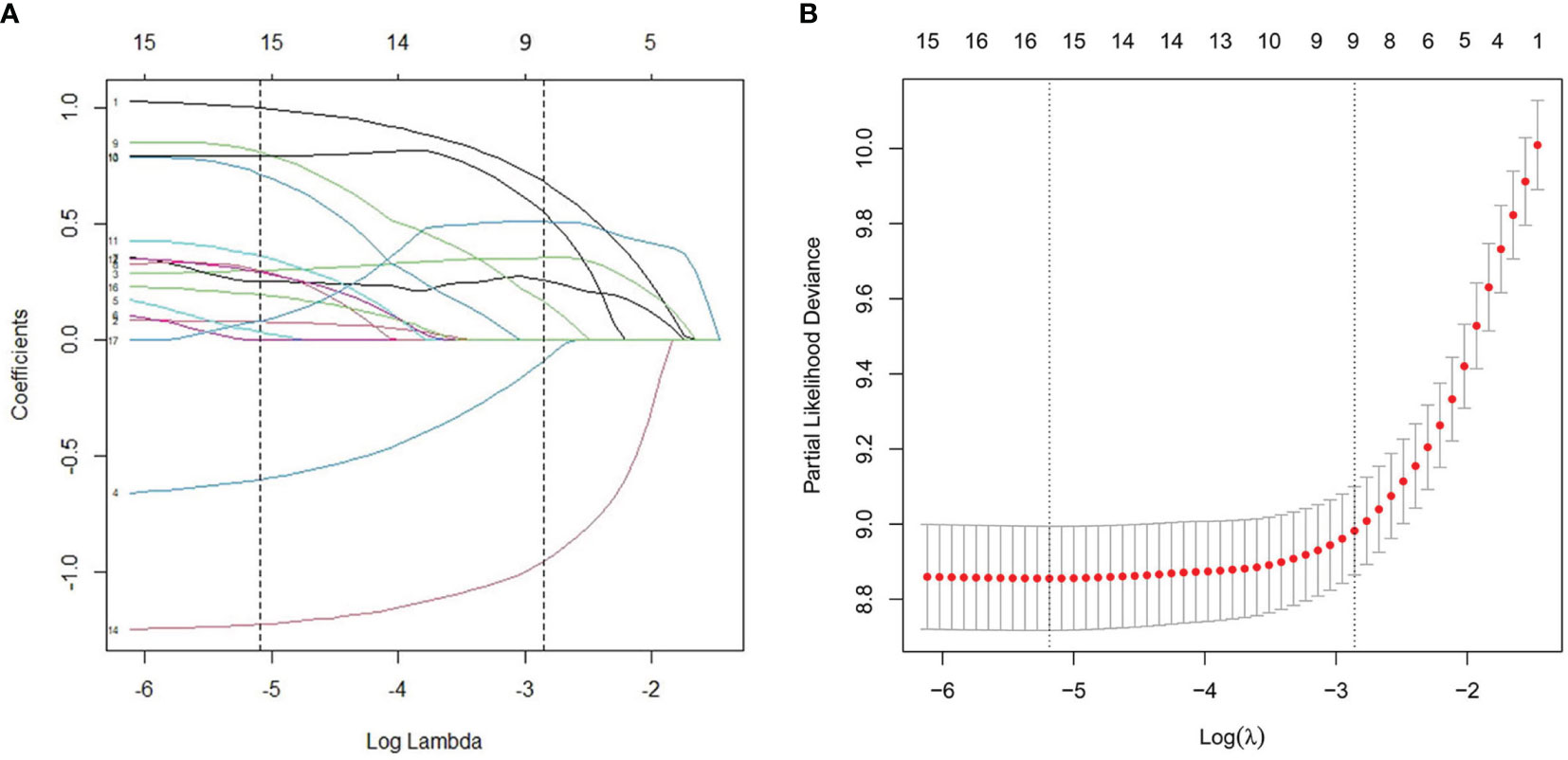
Figure 3 L1-penalized (Lasso) regression model were adopted to determine further predictive variables in test dataset. (A) LASSO coefficient patterns of the features. (B) Ten-time cross-verification for tuning parameter selection in the Lasso model.
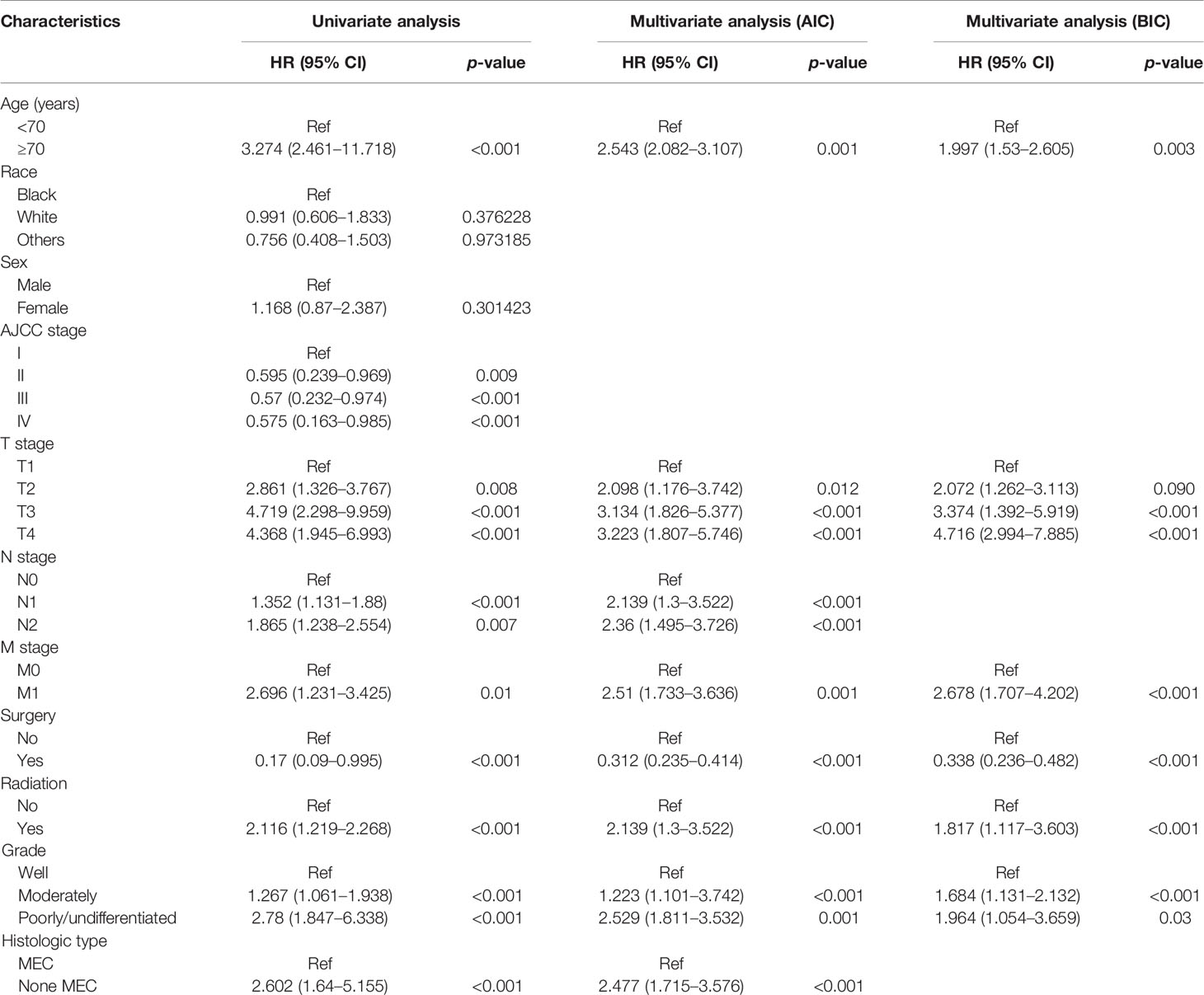
Table 3 Results of univariate and multivariate analyses by Fine-Gray proportional subdistribution hazards model in the training cohort.
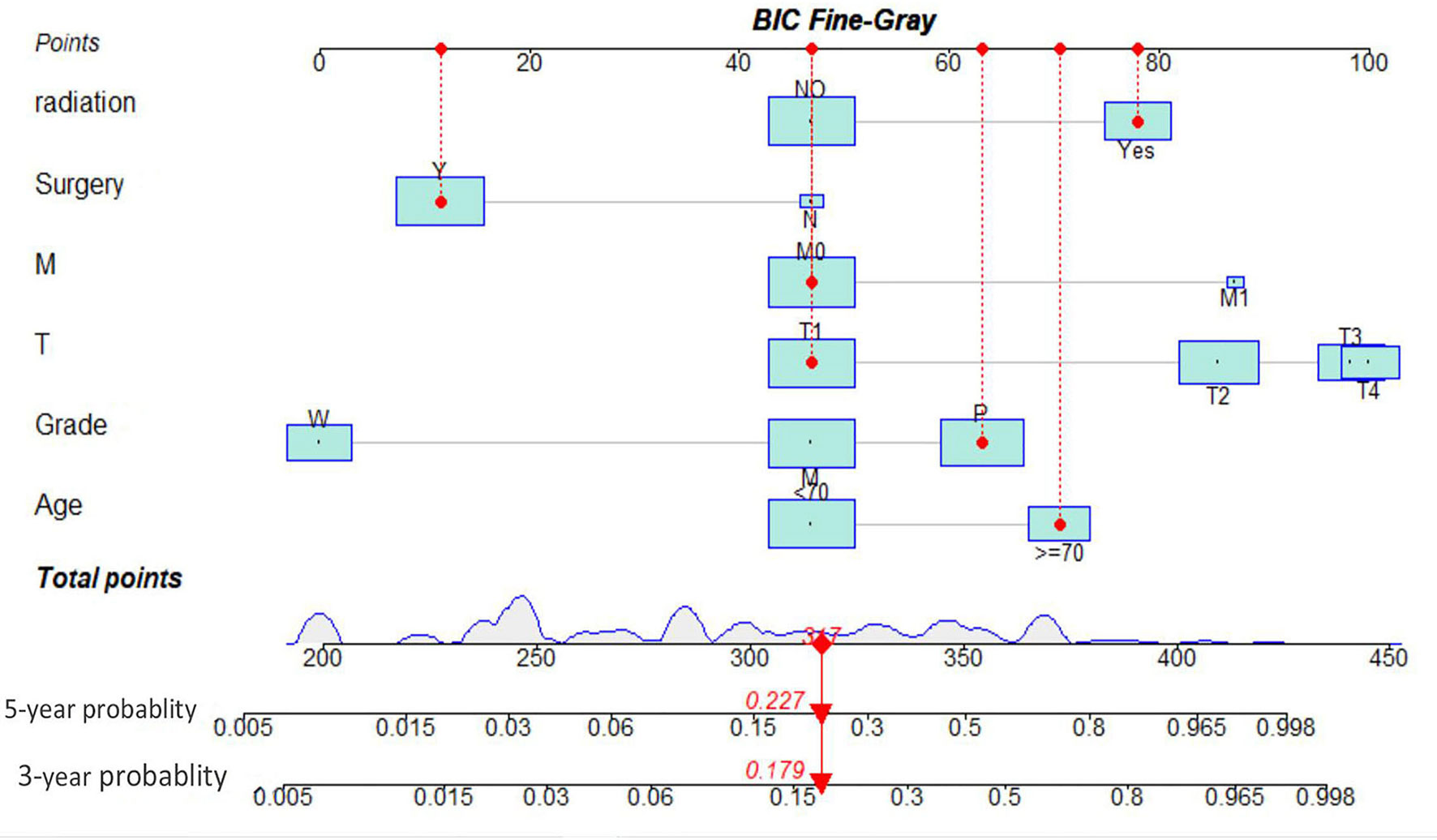
Figure 4 Interactive nomogram for predicting 3- and 5-year likelihoods of CSD in parotid carcinoma. BIC, Bayesian information criterion; MEC, mucoepidermoid carcinoma.
Nomogram Verification
The C-indexes of the developed nomogram for prediction of the likelihood of CSD in the test data set were 0.862, and the internal verification datasets were 0.843 and 0.795 in the external verification. The AUC of the competing risk nomogram model for forecasting 3- and 5-year likelihoods of CSD was 0.851 and 0.861 in the test cohort, 0.834 and 0.843 in the internal verification cohort, and 0.761 and 0.751 in the external verification cohort. The calibration plots demonstrated optimal consistency of the actual likelihood with the nomogram-forecasted likelihoods in the test (Figures 5A, D), as well as verification datasets (Figures 5B, C, E, F). The above data illustrated the good estimation potential along with the high confidence of our nomogram.
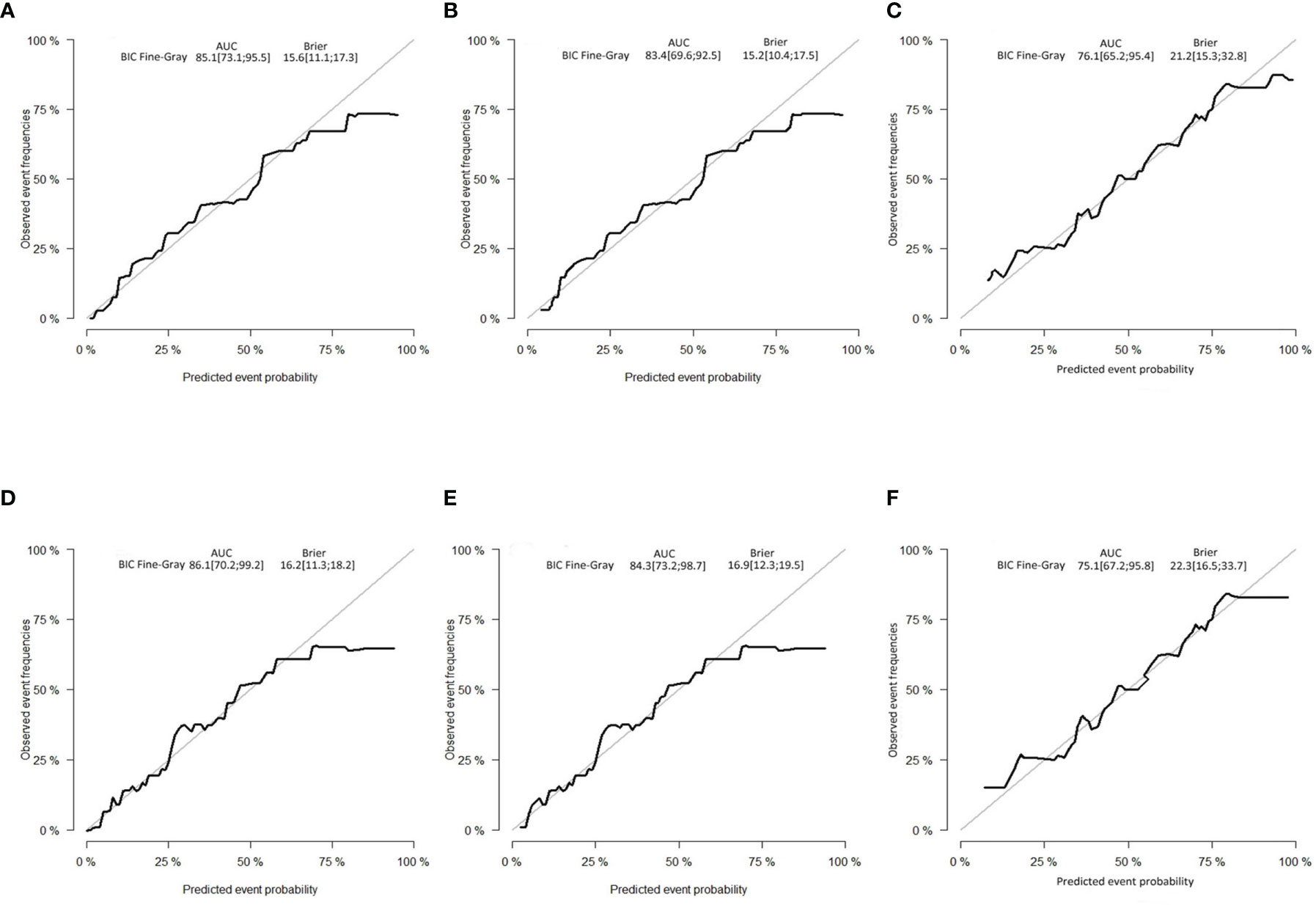
Figure 5 Calibration curves. (A) Three-year and (D) 5-year likelihoods of CSD in the test dataset. (B) Three-year and (E) 5-year likelihoods of CSD in the internal verification dataset. (C) Three-year and (F) 5-year likelihoods of CSD in the external verification dataset. BIC, Bayesian information criterion; AUC, area under the curve.
Decision Curve Analysis
The DCA was carried out in the test, internal verification, and external verification datasets. The estimation model exhibited an elevated net benefit coupled with a wide range of cutoff likelihood in contrast with the AJCC categorization criteria, illustrating that our prognostic model exhibited a high clinical application value (Figures 6A–F).
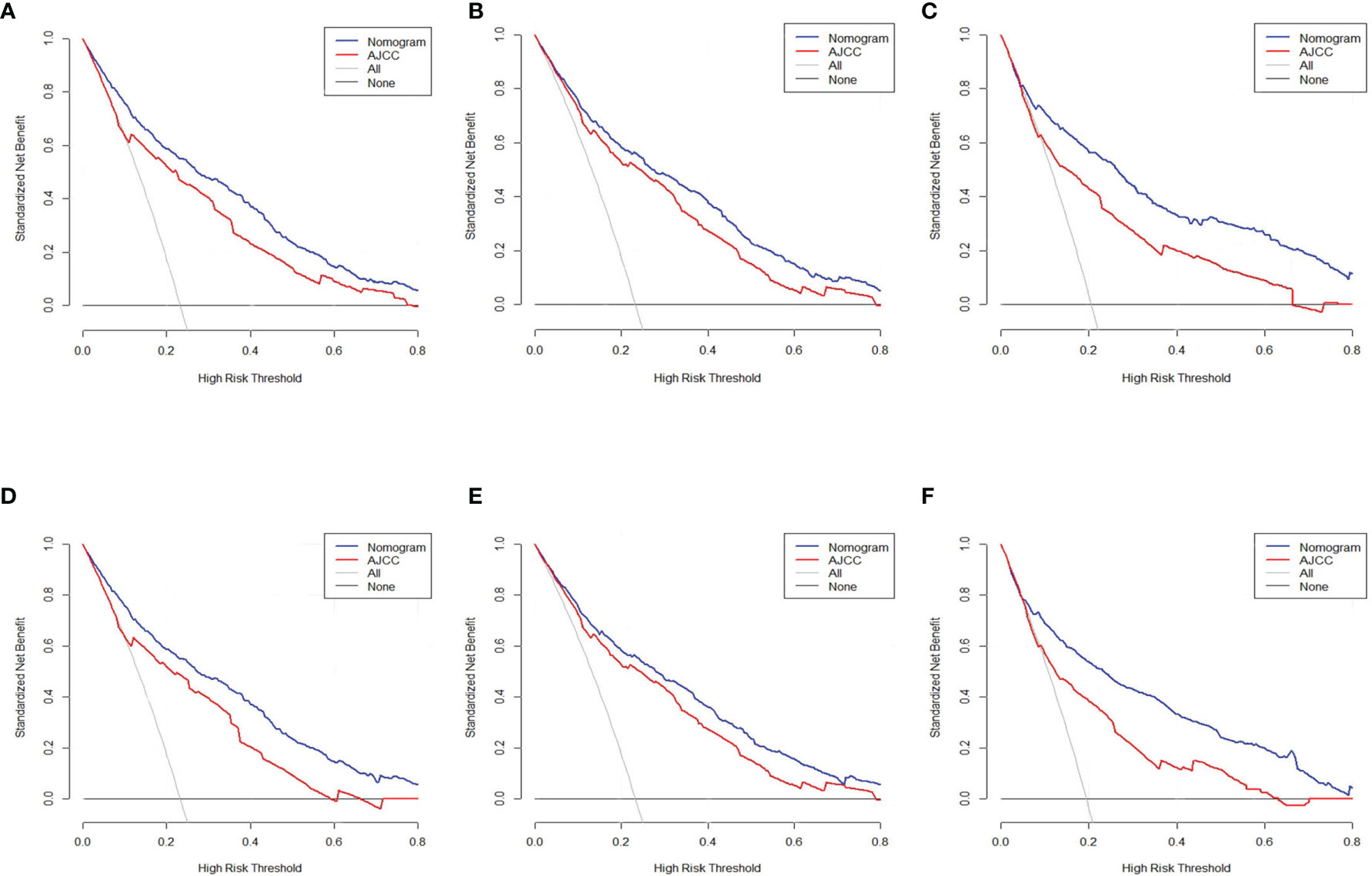
Figure 6 Decision curve assessment of the nomogram along with the AJCC staging approach in the estimation of the CSD of individuals with PC at 3 and 5 years in the test cohort (A, D), internal verification (B, E), and external verification (C, F) cohorts.
Discussion
Salivary gland tumors (SGT) are rare, representing less than 3% of all head and neck tumors (17). On the basis of literature, 22%–35% of SGT are malignant, with the percentage of malignant SGT in the parotid being 15%–25% (1, 18). The pathological types of PC are very complex. Different types of tumors have different clinical and imaging manifestations, treatment, and prognosis. Herein, for the first time, a nomogram for the prognosis of persons with PC was created in a competitive risk nomogram and determined more precise indicators. The large-sized samples available in the SEER data repository reduce errors in this research. Relative to prevailing tools for assessing survival outcomes, the developed nomogram ensures that the chosen variables can be directly associated with a prognosis of cancer. Currently, the most widely used prognostic tool for all solid tumors, including salivary gland tumors, is the TNM staging system (19), but this staging system did not include treatment options such as surgery, chemotherapy, and radiotherapy. However, a nomogram can allow individualized examination of patient prognosis since it incorporates numerous variables.
Of the 11 variables determined in this study, these predictors have been proven in other studies (20, 21). Nine (age, AJCC stage, radiation, T stage, M stage, grade, surgery, N stage, and histological type) were established as indicators of CSD in persons with PC via univariate coupled with the Lasso Cox regression model competing risk assessment. Sex and race were excluded in the univariate assessment, illustrating that they do not influence CSD in persons with PC. Assessment of multiple variables using the competing nomogram showed that the AJCC stage was not an independent indicator of patient prognosis. Finally, six variables (age, T stage, M stage, surgery, radiation, and grade) were used to construct the nomogram.
Similar to a study by Sun et al., age was found to independently influence the prognosis of parotid gland mucoepidermoid carcinoma (20). Lyu et al. investigated staging of PC and documented that patient age, favoring 40–60-year-old patients, which is a considerable independent indicator after adjusting for other confounders, might be because older patients have more comorbidities coupled with elevated perioperative risks (22, 23). This finding was congruent with Sun et al. who documented that the prognosis was not remarkably different across races (20) and survival differences between races are not remarkable. Fang et al. documented that sex had no effect on cancer-distinct survival of PC patients, which is congruent with our work (24). The findings also showed that histology type and N stage were independent indicators for PC patients, which is in agreement with previous findings in malignant salivary gland tumor research (25). To prevent overfitting, the aforementioned factors were omitted using PC to improve the performance of the model.
Surgical treatment is the most frequently used therapy for PC at all stages, although according to the guidelines published by the NCCN, surgery is highly recommended for resectable PC (T1–T4a) (26). The data herein demonstrated that surgical therapy could remarkably diminish the tumor-distinct risk of death in individuals with PC; this has been confirmed by most clinicians. However, neck dissection is a controversial subject in parotid malignant tumors. In the presence of a clinically palpable lymph node, there is a consensus on the application of elective neck dissection with a primary parotid surgery (27), some authors support elective neck dissection depending on the tumor histology, size, and grade (28, 29). However, in the study by Ali et al., they suggested that the neck is susceptible to be a target region for metastatic diseases; therefore, complete neck dissection between levels I to V is recommended (30). We established that radiation therapy could remarkably suppress deaths in PC patients, but whether radiotherapy can significantly improve the prognosis of patients is still controversial. In the study by Kaur et al., they believe that postoperative radiotherapy (PORT) has shown a survival benefit in patients with major salivary gland carcinoma (31). For patients with resected T1–2 tumors, the present protocols advocate radiation treatment after operation for individuals with adenoid cystic pathology, close (<1 mm) perineural, or lymphovascular infiltration, or positive margins, lymph node metastasis, as well as intermediate- or high-grade histology (26). In a parotid gland infiltrating ductal carcinoma (IDC) research, they found that PORT only enhanced survival of individuals with parotid gland IDC within T3–4, N1, and TNM III subgroups (32).
Mucoepidermoid carcinoma (MEC), the most prevalent type of PC, constitutes approximately 30–50% of malignant salivary glands (33, 34) However, there is no prognostic analysis of different pathological types of parotid carcinoma. Herein, MEC accounted for 42.3% of all PC cases, and we exhibited that the risk of CSD in persons with MEC was not remarkably higher relative to that in other types of PC such as adenoid cystic carcinoma and polymorphous adenocarcinoma, adenocarcinoma NOS, and myoepithelial carcinoma. This is consistent with the result of Filho OVO et in a retrospective analysis of 193 patients (25). Nevertheless, Baddour et al. and Kokemueller et al. revealed higher survival rates at 5, 10, and 15 years for MEC in relation to other types of PC (35, 36). We think that this should be related to the difference in diagnosis and treatment level between different regions.
Previous investigation on parotid carcinoma based on the SEER data repository focused on incidence, along with survival trends (37, 38), while we focused on creating a prognostic nomogram herein. The clinical therapy of PC and the evaluation of prognosis currently depends on the AJCC staging method. Our prognostic model is suitable for all persons with PC and could be extensively applied in all levels of medical centers. The comprehensive nature of the nomogram may cover the shortcomings of the AJCC staging method, and allow individualized treatment, as well as the precise examination of the prognosis of individuals with PC. Besides, the user-friendly graphic interface of the nomogram could promote the interaction of clinicians with patients. Additionally, a verification data set was utilized for external verification, and the data were drastically congruent with actual survival likelihoods.
However, this research had some shortcomings. In the first place, the SEER data repository lacks some pivotal factors tied to prognosis, including perineural invasion, smoking history, chronic parotitis history, comorbidities, and lack of genetic records of patients. Besides, we adopted the sixth or seventh edition of the AJCC staging method, which lacks two pivotal variables (depth of invasion, as well as an extranodal extension) in contrast with the eighth edition. Moreover, the SEER repository lacks data on tumor volume, which is considered a significant prognostic factor for Salivary gland tumors. Even though this work incorporated the data on chemotherapy and radiotherapy, but the SEER database lacks detailed data on cycles number and doses of chemotherapy, the radiotherapy approaches, and the follow-up treatment after relapse. These variables can also influence the prognosis. Lastly, even though the SEER data repository provided an extensive range of samples for this analysis, errors exist when this database is utilized in the global context. Besides, the data of the external verification are only from a single province in China. It has been reported that even in China, there are different epidemiological differences between the north and the south (1, 39). Therefore larger-sample multicenter study should be conducted to further improve our estimation model and validate its clinical application significance.
Conclusion
We have created a competing risk nomogram for PC patients using the data retrieved from the SEER data repository and carried out external verification to show the precision and reliability of our nomogram. This well-calibrated nomogram could be utilized in making clinical decisions regarding the prognosis as well as personalized treatment of PC patients.
Data Availability Statement
The original contributions presented in the study are included in the article/supplementary material. Further inquiries can be directed to the corresponding authors.
Ethics Statement
Written informed consent was obtained from the individual(s) for the publication of any potentially identifiable images or data included in this article.
Author Contributions
XL and MH were responsible for the conception and design of the study, assisted with the statistical analysis, and wrote and revised the manuscript. MH and WG were involved in the collecting of data and follow up of the patients. SC and JM contributed their help on the data analysis, revised the English language and grammar, and corrected parts of the discussion. DL has made the primary contribution in the later stage in the writing and modification of the paper and the review of the finalized article. All authors contributed to the article and approved the submitted version.
Funding
This study was supported by the National Natural Science Foundation of China (No. 81560410) and the Postgraduate Innovation Special Foundation of Jiangxi Province (YC2020-B043).
Conflict of Interest
The authors declare that the research was conducted in the absence of any commercial or financial relationships that could be construed as a potential conflict of interest.
Publisher’s Note
All claims expressed in this article are solely those of the authors and do not necessarily represent those of their affiliated organizations, or those of the publisher, the editors and the reviewers. Any product that may be evaluated in this article, or claim that may be made by its manufacturer, is not guaranteed or endorsed by the publisher.
References
1. Gao M, Hao Y, Huang MX, Ma DQ, Chen Y, Luo HY, et al. Salivary Gland Tumours in a Northern Chinese Population: A 50-Year Retrospective Study of 7190 Cases. Int J Oral Maxillofac Surg (2017) 46(3):343–9. doi: 10.1016/j.ijom.2016.09.021
2. da Silva LP, Serpa MS, Viveiros SK, Sena DAC, de Carvalho Pinho RF, de Abreu Guimarães LD, et al. Salivary Gland Tumors in a Brazilian Population: A 20-Year Retrospective and Multicentric Study of 2292 Cases. J Craniomaxillofac Surg (2018) 46(12):2227–33. doi: 10.1016/j.jcms.2018.09.028
3. de Ridder M, Balm AJ, Smeele LE, Wouters MW, van Dijk BA. An Epidemiological Evaluation of Salivary Gland Cancer in the Netherlands (1989–2010). Cancer Epidemiol (2015) 39(1):14–20. doi: 10.1016/j.canep.2014.10.007
4. McHugh CH, Roberts DB, El-Naggar AK, Hanna EY, Garden AS, Kies MS, et al. Prognostic Factors in Mucoepidermoid Carcinoma of the Salivary Glands. Cancer (2012) 118:3928–36. doi: 10.1002/cncr.26697
5. Chen AM, Lau VH, Farwell DG, Luu Q, Donald PJ. Mucoepidermoid Carcinoma of the Parotid Gland Treated by Surgery and Postoperative Radiation Therapy: Clinicopathologic Correlates of Outcome. Laryngoscope (2013) 123(12):3049–55. doi: 10.1002/lary.24238
6. Ali S, Palmer FL, Yu C, DiLorenzo M, Shah JP, Kattan MW, et al. A Predictive Nomogram for Recurrence of Carcinoma of the Major Salivary Glands. JAMA Otolaryngol Head Neck Surg (2013) 139(7):698–705. doi: 10.1001/jamaoto.2013.3347
7. Cui J, Wang L, Zhong W, Chen Z, Tan X, Yang H, et al. Development and Validation of Nomogram to Predict Risk of Survival in Patients With Laryngeal Squamous Cell Carcinoma. Biosci Rep (2020) 40(8):BSR20200228. doi: 10.1042/BSR20200228
8. Li J, Chen S, Peng S, Liu Y, Xing S, He X, et al. Prognostic Nomogram for Patients With Nasopharyngeal Carcinoma Incorporating Hematological Biomarkers and Clinical Characteristics. Int J Biol Sci (2018) 14(5):549–56. doi: 10.7150/ijbs.24374
9. Doll KM, Rademaker A, Sosa JA. Practical Guide to Surgical Data Sets: Surveillance, Epidemiology, and End Results (SEER) Database. JAMA Surg (2018) 153(6):588–9. doi: 10.1001/jamasurg.2018.0501
10. Dignam JJ, Zhang Q, Kocherginsky M. The Use and Interpretation of Competing Risks Regression Models. Clin Cancer Res (2012) 18(8):2301–8. doi: 10.1158/1078-0432.CCR-11-2097
11. Li J, Zheng Q, Zhao X, Zhao J, An T, Wu M, et al. Nomogram Model for Predicting Cause-Specific Mortality in Patients With Stage I Small-Cell Lung Cancer: A Competing Risk Analysis. BMC Cancer (2020) 20(1):793. doi: 10.1186/s12885-020-07271-9
12. Yang Y, Chen ZJ, Yan S. The Incidence, Risk Factors and Predictive Nomograms for Early Death Among Patients With Stage IV Gastric Cancer: A Population-Based Study. J Gastrointest Oncol (2020) 11(5):964–82. doi: 10.21037/jgo-20-217
13. Dibas M, Ghozy S, Morsy S, Salah Abbas A, Alkahtani S, Bin-Jumah M, et al. Novel Nomograms Predicting Overall and Cancer-Specific Survival of Malignant Ependymoma Patients: A Population-Based Study. J Neurosurg Sci (2020). doi: 10.23736/S0390-5616.20.05033-X
14. Li J, Li X, Gu J, Ma X, Xue F. A Competing-Risks Nomogram for Predicting Probability of Death From CRC in Chinese Han Patients With Stage I-III CRC. Jpn J Clin Oncol (2018) 48(12):1088–95. doi: 10.1093/jjco/hyy136
15. van Steenbeek CD, van Maaren MC, Siesling S, Witteveen A, Verbeek XAAM, Koffijberg H. Facilitating Validation of Prediction Models: A Comparison of Manual and Semi-Automated Validation Using Registry-Based Data of Breast Cancer Patients in the Netherlands. BMC Med Res Methodol (2019) 19(1):117. doi: 10.1186/s12874-019-0761-5
16. Fei Z, Qiu X, Li M, Chen C, Li Y, Huang Y. Prognosis Viewing for Nasopharyngeal Carcinoma Treated With Intensity-Modulated Radiation Therapy: Application of Nomogram and Decision Curve Analysis. Jpn J Clin Oncol (2020) 50(2):159–68. doi: 10.1093/jjco/hyz165
17. Ito FA, Ito K, Vargas PA, de Almeida OP, Lopes MA. Salivary Gland Tumors in a Brazilian Population: A Retrospective Study of 496 Cases. Int J Oral Maxillofac Surg (2005) 34(5):533–6. doi: 10.1016/j.ijom.2005.02.005
18. Israel Y, Rachmiel A, Ziv G, Nagler R. Benign and Malignant Salivary Gland Tumors - Clinical and Demographic Characteristics. Anticancer Res (2016) 36(8):4151–4.
19. Webber C, Gospodarowicz M, Sobin LH, Wittekind C, Greene FL, Mason MD, et al. Improving the TNM Classification: Findings From a 10-Year Continuous Literature Review. Int J Cancer (2014) 135(2):371–8. doi: 10.1002/ijc.28683
20. Sun J, Sun Y, Yang F, Zhou Q, Liu W, Cheng Y. Nomogram to Predict the Prognosis of Parotid Gland Mucoepidermoid Carcinoma: A Population-Based Study of 1306 Cases. Peer J (2019) 7:e7237. doi: 10.7717/peerj.7237
21. Chakrabarti S, Nair D, Malik A, Qayyumi B, Nair S, Agrawal JP, et al. Prognostic Factors in Parotid Cancers: Clinicopathological and Treatment Factors Influencing Outcomes. Indian J Cancer (2018) 55(1):98–104. doi: 10.4103/ijc.IJC_503_17
22. Lyu HX, Wang ZR, Gao YQ, Yu M, Li BQ, Zhang ZB. Clinical Pathologic Analysis on 3 724 Cases of Salivary Gland Tumors. Zhonghua Kou Qiang Yi Xue Za Zhi (2019) 54(1):10–6. doi: 10.3760/cma.j.issn.1002-0098.2019.01.003
23. Nakano T, Yasumatsu R, Kogo R, Hashimoto K, Asai K, Ohga S, et al. Parotid Gland Carcinoma: 32 Years' Experience From a Single Institute. J Laryngol Otol (2019) 133(7):604–9. doi: 10.1017/S0022215119001130
24. Fang Q, Wu J, Liu F. Oncologic Outcome and Potential Prognostic Factors in Primary Squamous Cell Carcinoma of the Parotid Gland. BMC Cancer (2019) 19(1):752. doi: 10.1186/s12885-019-5969-6
25. Filho OVO, Rêgo TJRD, Mendes FHO, Dantas TS, Cunha MDPSS, Malta CEN, et al. Prognostic Factors and Overall Survival in a 15-Year Followup of Patients With Malignant Salivary Gland Tumors: A Retrospective Analysis of 193 Patients. Braz J Otorhinolaryngol (2020) S1808-8694(20):30116–6. doi: 10.1016/j.bjorl.2020.06.016
26. Pfister DG, Spencer S, Adelstein D, Adkins D, Anzai Y, Brizel DM, et al. Head and Neck Cancers, Version 2.2020, NCCN Clinical Practice Guidelines in Oncology. J Natl Compr Canc Netw (2020) 18(7):873–98. doi: 10.6004/jnccn.2020.0031
27. Hanna EYN, Suen JY. Malignant Tumors of the Salivary Glands. In: Myers EN, Suen JY, Myers JN, Hanna EYN, editors. Cancer of the Head and Neck, 4th ed. Philadelphia: Saunders (2003). p. 475–510. doi: 10.1007/0-387-21701-0_9
28. Armstrong JG, Harrison LB, Thaler HT, Friedlander-Klar H, Fass DE, Zelefsky MJ, et al. The Indications for Elective Treatment of the Neck in Cancer of the Major Salivary Glands. Cancer (1992) 69(3):615–9. doi: 10.1002/1097-0142(19920201)69:3<615::aid-cncr2820690303>3.0.co;2-9
29. Lloyd S, Yu JB, Ross DA, Wilson LD, Decker RH. A Prognostic Index for Predicting Lymph Node Metastasis in Minor Salivary Gland Cancer. Int J Radiat Oncol Biol Phys (2010) 76(1):169–75. doi: 10.1016/j.ijrobp.2009.01.021
30. Ali S, Palmer FL, DiLorenzo M, Shah JP, Patel SG, Ganly I. Treatment of the Neck in Carcinoma of the Parotid Gland. Ann Surg Oncol (2014) 21(9):3042–8. doi: 10.1245/s10434-014-3681-y
31. Kaur J, Goyal S, Muzumder S, Bhasker S, Mohanti BK, Rath GK. Outcome of Surgery and Post-Operative Radiotherapy for Major Salivary Gland Carcinoma: Ten Year Experience From a Single Institute. Asian Pac J Cancer Prev (2014) 15(19):8259–63. doi: 10.7314/apjcp.2014.15.19.8259
32. Lv T, Wang Y, Wang X. Subgroups of Parotid Gland Infiltrating Ductal Carcinoma Benefit From Postoperative Radiotherapy: A Population-Based Study. Future Oncol (2019) 15(8):885–95. doi: 10.2217/fon-2018-0495
33. Brandwein MS, Ivanov K, Wallace DI, Hille JJ, Wang B, Fahmy A, et al. Mucoepidermoid Carcinoma: A Clinicopathologic Study of 80 Patients With Special Reference to Histological Grading. Am J Surg Pathol (2001) 25(7):835–45. doi: 10.1097/00000478-200107000-00001
34. Lydiatt WM, Patel SG, O'Sullivan B, Brandwein MS, Ridge JA, Migliacci JC, et al. Head and Neck Cancers-Major Changes in the American Joint Committee on Cancer Eighth Edition Cancer Staging Manual. CA Cancer J Clin (2017) 67(2):122–37. doi: 10.3322/caac.21389
35. Baddour HM Jr, Fedewa SA, Chen AY. Five- and 10-Year Cause-Specific Survival Rates in Carcinoma of the Minor Salivary Gland. JAMA Otolaryngol Head Neck Surg (2016) 142(1):67–73. doi: 10.1001/jamaoto.2015.2805
36. Kokemueller H, Swennen G, Brueggemann N, Brachvogel P, Eckardt A, Hausamen JE. Epithelial Malignancies of the Salivary Glands: Clinical Experience of a Single Institution-a Review. Int J Oral Maxillofac Surg (2004) 33(5):423–32. doi: 10.1016/j.ijom.2004.02.007
37. Chen MM, Roman SA, Sosa JA, Judson BL. Histologic Grade as Prognostic Indicator for Mucoepidermoid Carcinoma: A Population-Level Analysis of 2400 Patients. Head Neck (2014) 36(2):158–63. doi: 10.1002/hed.23256
38. Rutt AL, Hawkshaw MJ, Lurie D, Sataloff RT. Salivary Gland Cancer in Patients Younger Than 30 Years. Ear Nose Throat J (2011) 90(4):174–84. doi: 10.1177/014556131109000409
Keywords: SEER, parotid cancer, cancer-specific death, competing risk, nomogram
Citation: Li X, Hu M, Gu W, Liu D, Mei J and Chen S (2021) Nomogram Predicting Cancer-Specific Death in Parotid Carcinoma: a Competing Risk Analysis. Front. Oncol. 11:698870. doi: 10.3389/fonc.2021.698870
Received: 22 April 2021; Accepted: 17 September 2021;
Published: 13 October 2021.
Edited by:
Yong Yin, Shandong Cancer Hospital, ChinaReviewed by:
Peiguo Wang, Tianjin Medical University Cancer Institute and Hospital, ChinaJingao Li, Jiangxi Provincial Cancer Hospital, China
Copyright © 2021 Li, Hu, Gu, Liu, Mei and Chen. This is an open-access article distributed under the terms of the Creative Commons Attribution License (CC BY). The use, distribution or reproduction in other forums is permitted, provided the original author(s) and the copyright owner(s) are credited and that the original publication in this journal is cited, in accordance with accepted academic practice. No use, distribution or reproduction is permitted which does not comply with these terms.
*Correspondence: Shaoqing Chen, 1075017867@qq.com; Jinhong Mei, mjhdoctor@126.com; Dewu Liu, dewuliu2@126.com
†These authors have contributed equally to this work