- 1Medical Imaging Key Laboratory of Sichuan Province, Department of Radiology, Medical Research Center, Affiliated Hospital of North Sichuan Medical College, Nanchong, China
- 2Department of Hepatocellular Surgery, Institute of Hepato-Biliary-Intestinal Disease, Affiliated Hospital of North Sichuan Medical College, Nanchong, China
- 3School of Medical Imaging, North Sichuan Medical College, Nanchong, China
Background: Hepatocellular carcinoma (HCC) is the sixth most common cancer in the world and the third leading cause of cancer-related death. Although the diagnostic scheme of HCC is currently undergoing refinement, the prognosis of HCC is still not satisfactory. In addition to certain factors, such as tumor size and number and vascular invasion displayed on traditional imaging, some histopathological features and gene expression parameters are also important for the prognosis of HCC patients. However, most parameters are based on postoperative pathological examinations, which cannot help with preoperative decision-making. As a new field, radiomics extracts high-throughput imaging data from different types of images to build models and predict clinical outcomes noninvasively before surgery, rendering it a powerful aid for making personalized treatment decisions preoperatively.
Objective: This study reviewed the workflow of radiomics and the research progress on magnetic resonance imaging (MRI) radiomics in the diagnosis and treatment of HCC.
Methods: A literature review was conducted by searching PubMed for search of relevant peer-reviewed articles published from May 2017 to June 2021.The search keywords included HCC, MRI, radiomics, deep learning, artificial intelligence, machine learning, neural network, texture analysis, diagnosis, histopathology, microvascular invasion, surgical resection, radiofrequency, recurrence, relapse, transarterial chemoembolization, targeted therapy, immunotherapy, therapeutic response, and prognosis.
Results: Radiomics features on MRI can be used as biomarkers to determine the differential diagnosis, histological grade, microvascular invasion status, gene expression status, local and systemic therapeutic responses, and prognosis of HCC patients.
Conclusion: Radiomics is a promising new imaging method. MRI radiomics has high application value in the diagnosis and treatment of HCC.
Introduction
Hepatocellular carcinoma (HCC) is the sixth most common cancer and the third leading cause of cancer-related death worldwide (1). Although the diagnostic criteria of HCC continue to improve, its prognosis remains unsatisfactory (2). In addition to certain factors, such as tumor size and number and vascular invasion displayed on traditional imaging, some histo-pathological features and gene expression parameters are also important in the prognoses of patients with HCC. However, many current staging systems for HCC have not taken into consideration the above-mentioned histopathological features or genetic traits beyond the size and number and vascular invasion of the tumor (3, 4). Most parameters are based on postoperative pathological examinations, which cannot help with preoperative decision-making. To better stratify HCC patients before surgery, make more accurate treatment decisions, and improve the prognoses of patients, there is an urgent need for a noninvasive method that can accurately predict the histo-pathological features and gene expression parameters before surgery. The rapid development of artificial intelligence has played an important role in personalized precision medicine (5). Radiomics, a new technology, can transform the potential histopathological and physiological information in images into high-dimensional quantitative image features that can be mined (6, 7).The study of radiomics will contribute to the early diagnosis and treatment of HCC and ultimately improve survival (8, 9). In recent years, many studies have confirmed the application values of magnetic resonance imaging (MRI) radiomics in the diagnosis and differentiation (10, 11), histological grading (12, 13), microvascular invasion (MVI)assessment (14, 15), radiogenomics (16, 17),prediction of relapse and prognosis after surgical resection (18–20), response to transarterial chemoembolization(TACE) (21, 22) and systemic treatment efficacy of HCC (23).
To better understand the research hotspots and trends of MRI radiomics in HCC, we used PubMed to identify important recent publications on MRI radiomics in HCC, selected research articles and reviews and used bibliometric method to visually analyze the countries, institution, authors, and keywords of MRI radiomics in HCC. Meanwhile, this study reviews the radiomics workflow from image acquisition and reconstruction, segmentation, feature extraction, feature selection and modeling to model validation, and the research progress of MRI radiomics in HCC.
Bibliometrics of MRI Radiomics in HCC
The authors conducted a literature review using PubMed to identify important recent publications and determine the current status of radiomics in HCC. A comprehensive list of MeSH terms and keywords was included in the search: HCC, MRI, radiomics, deep learning(DL), artificial intelligence, machine learning, neural network, texture analysis, diagnosis, histopathology, microvascular invasion, surgical resection, radiofrequency, recurrence, relapse, TACE, targeted therapy, immunotherapy, therapeutic response, and prognosis. The inclusion criteria were as follows: (1) original research articles and review articles published in the English language between May 2017 and June 2021; (2) literature related to MRI radiomics or DL; and (3) literature related to diagnosis and differentiation, histological grading, MVI assessment, radiogenomics, prediction of relapse and prognosis after surgical resection, response to TACE and systemic treatment efficacy of HCC. Articles that were not published in English and those containing irrelevant information on the subject were excluded. We also excluded articles that were published before May 2017 and after June 2021. In total, 129 articles were ultimately retrieved. After screening the titles, abstracts and full texts (if appropriate), only 84 papers met the criteria for inclusion (Figure 1). Those 84 papers were then downloaded with the record content of “Full Record and Cited References” and the file format of “Plain Text”. As CiteSpace can only recognize files named “download *.txt”, the files were renamed accordingly. The bibliometric software CiteSpace5.7.R2 (64 bits) was utilized for this study to visually analyze the countries, institution, authors, and keywords draw relevant charts. The articles originated from a total of 12 countries, and the top five countries were China (24), the USA (13), South Korea (5), Germany (3), and France (2). A total of 30 institutions published manuscripts independently or cooperatively. The top five institutions were the Chinese Academy of Sciences (12), Fudan University (10), GE Healthcare (8), Sun Yat-Sen University (7), and Sichuan University (5). Bin Song and Xin Li were the most prolific authors. Meng-Su Zeng, Jie Tian, and Dong-Sheng Gu were also active in this field. “Hepatocellular carcinoma” was the most important term, followed by “radiomics”, “recurrence”, and “microvascular invasion”. According to the link strength of keyword cooccurrence, the network was divided into eight clusters, and the largest cluster was “tumor differentiation (#0)” (Figure 2).
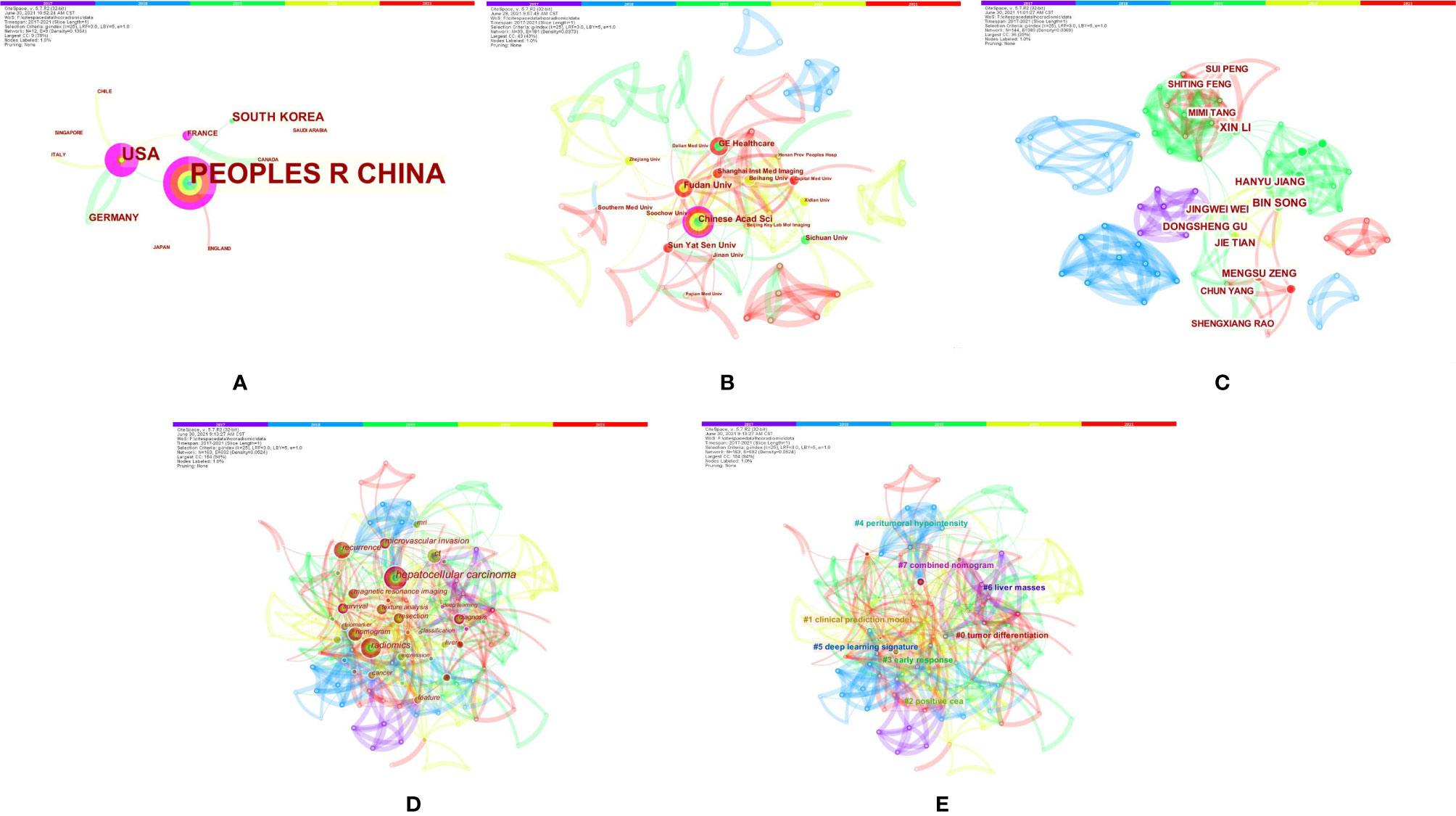
Figure 2 Bibliometrics of magnetic resonance imaging radiomics in hepatocellular carcinoma. (A) Co-occurrence map of countries. (B) Co-occurrence map of institutions. (C) Co-occurrence map of authors. (D) Terms in theco-occurrence network. (E) Terms in theco-occurrence clusters.
Radiomics Workflow
Radiomics extracts high-throughput features from images and transforms imaging data into high-resolution mining data spaces through machine learning (25). Quantitative radiological data can therefore be extracted and applied to clinical decision-making (25). The workflow of radiomics usually includes five steps (6), which are described below.
Image Acquisition and Reconstruction
Imaging techniques that can be used for radiomics include MRI, computed tomography (CT), positron-emission tomography, and ultrasound. Among them, MRI has the advantage of depicting more soft-tissue features. Radiomics is an imaging analysis method; thus, it is vital to standardize high-quality images (26–28). This makes it necessary to preprocess the imaging data; otherwise, a widely promoted standard scanning protocol is needed to reduce the variability in radiomic features and improve the performance of radiomic models (25, 29).
Image Segmentation
Manual, automatic, and semiautomatic segmentation are often used to segment the volume or region of interest in a target tissue (30). Manual segmentation is most reliable, but it involves intraobserver and interobserver variability. Its labor and time cost are high. The segmentation of an image often requires multiple clinicians or the same clinician at multiple times. The intraobserver and interobserver variability can be improved by screening the intraobserver and interobserver consistency. The purpose of automatic segmentation is to mark the regions of interest automatically by a computer. Semiautomatic segmentation involves manual corrections. Automatic segmentation algorithms include image segmentation based on thresholds, image segmentation based on region growing, and image segmentation based on edge detection. Some classical algorithms perform well at delineating liver lesions (31, 32).
Image Feature Extraction
Image features include semantic features and nonsemanticfeatures (33). Semantic features include qualitative (shapes, boundaries, etc.) and quantitative features, and their analysis depends on the radiologist’s knowledge. Nonsemantic features are quantitative descriptors extracted from tissues of interest, including shape and statistical features (34). The shape features of objects in images include topological features, distances, perimeters, areas, geometric features, and descriptions of shape and orientation. Statistical features can be further divided into first-order, second-order, and high-order features. First-order features are usually called density features, which involve gray-level histogram information simply describing the global distribution of gray levels in an image. Such features cannot describe the local distribution of gray levels in an image or the spatial position of each gray level (35). Second-order features are often called texture features. These reflect the relationships between adjacent voxels. High-order features are usually called filtering features and are generated by wavelet and Laplacian Gaussian filtering, for example, in addition to first-order and second-order features.
Feature Selection and Modeling
Many features can be extracted from a high-throughput image, but using all the features to analyze an image will lead to overfitting. The best features can be selected by dimensionality reduction to improve the efficiency of the model. The methods of feature selection can be divided into three categories: filter, wrapper, and embedded (36). The goal of radiomics is to establish a prediction model for clinical outcomes from selected features. The modeling methods include logistic regression, k-nearest neighbor, decision trees, ensemble learning, and support vector machines. It is recommended to test the effectiveness of several forecasting models to select the model with the best performance (37).
Model Validation
The prediction model can be validated by internal cross-validation, such that the model can be further optimized and the prediction performance can be maximized. Validation of the model should be carried out in a separate cohort (37). For differentiation analysis, the receiver operating characteristic (ROC) curve is the most commonly used method to evaluate the performance of the model. The area under the ROC curve (AUC) or the sensitivity and specificity of the model can be used to evaluate whether the model can predict clinical outcomes. For survival analysis, the concordance index (C-index) and the time-related ROC curve are usually used for validation (38).
Diagnosis and Differentiation
At present, the diagnosis of HCC is mainly based on imaging methods such as MRI, CT, and ultrasound. Because HCC has a typical enhancement mode, contrast-enhanced CT and dynamic contrast-enhanced MRI play important roles in the diagnosis of HCC (39–42). The European Association for the Study of the Liver standard (40) and the Liver Imaging Reporting and Data System (43) are widely recognized. However, the evaluation of imaging features may be subjective because radiologists have different experiences and different familiarities with the system (44, 45). Radiomics has important application value in the diagnosis of solid tumors because it uses advanced image processing technology to extract high-throughput data and quantitative analysis of tumor behavior and heterogeneity (6, 46–51).
Radiomics signatures based on conventional precontrast T1-weighted imaging, postcontrast T1-weighted imaging, T2-weighted imaging, diffusion-weighted imaging (DWI), and intravoxel incoherent motion (IVIM), whether alone or in combination with clinical data, are all valuable for HCC differentiation (52–59), and their differentiation efficiency is almost equal to that of experienced radiologists (10-year experience) (52). HCC, intrahepatic cholangiocarcinoma (ICC), and HCC-ICC have common risk factors (60, 61), and their typical qualitative MRI features may overlap (24, 62–64). Therefore, the conventional MRI diagnosis of HCC is still uncertain. According to Liu et al. (54), the imaging features extracted from MR images have great potential to differentiate combined hepatocellular cholangiocarcinoma from cholangiocarcinoma and HCC, showing a maximum AUC of 0.77. Recently, Zhu et al. (56) studied the application value of histogram features on IVIM-DWI in the differential diagnosis of HCC. They found that the histogram parameters of IVIM-DWI could distinguish hepatic hemangiomas, hepatic cysts, and HCC and that the volume of the pseudodiffusion coefficient and perfusion fraction had better diagnostic value than other histogram parameters (56).
In recent years, DL technology has been developed and has achieved excellent performance in the classification of hepatic lesions (65–71). Hamm CA et al. (65) developed a proof-of-concept convolutional neural network (CNN)-based DL system and classified 494 hepatic lesions from six categories on MRI. The system demonstrated 92% accuracy, 92% sensitivity and 98% specificity, and their results showed a 90% sensitivity for classifying HCC compared to 60%/70% for radiologists.
Histological Grading
The histological grading of HCC is key to determining the best treatment scheme and prognosis of a patient. High-grade HCC patients have a higher intrahepatic relapse rate than low-grade HCC patients (72, 73), and most high-grade HCC patients need larger safe resection margins and more frequent postoperative follow-up visits (74, 75). The radiomic features of precontrast T1-weighted imaging, postcontrast T1-weighted imaging, and T2-weighted imaging, whether alone or in combination with clinical data (76), are all valuable for identifying poorly differentiated HCC (13, 76–80). In addition, recent studies have shown the application value of functional MRI radiomics based on IVIM-DWI in predicting the pathological grade of HCC (12, 81, 82). Shi et al. (82)performed MRI on 52 HCC patients and extracted histogram indices from IVIM parameter maps. Eighteen IVIM histogram indices showed the capacity to differentiate histopathological grades. By establishing a diagnostic model based on logistic regression and integrating different histogram indices showing significant differences between different subgroups, the maximum diagnostic power for distinguishing HCC histological grades was obtained (AUC=0.917). This study indicated that histogram indices extracted from IVIM parameter maps had great potential in predicting histopathological grade (82). Geng et al. (12) extracted 107 radiomic features from SWI images of 53 HCC patients and Spearman correlation coefficients were used to evaluate the correlation between SWI radiomic features and histopathology. They found that the SWI radiomic features were significantly correlated with histopathological grades.
MVI
MVI is diagnosed depending on postoperative tissue specimens, but detection by conventional imaging is difficult. The presence of MVI indicates that the tumor has strong biological invasiveness, which can increase the relapse rate of HCC more than fourfold (83, 84). Accurate preoperative prediction of MVI of HCC can help doctors adjust treatment strategies in a timely manner (such as expanding the resection range), optimize treatment plans, reduce the risks of postoperative relapse, and improve the prognosis (84, 85). Enhanced MRI is helpful to predict MVI in HCC (83, 86–91). MRI-based radiomics (15, 92–102) and DL systems (103–106) have shown good performance in predicting MVI in HCC. The increase in clinicopathological risk factors and qualitative imaging features can improve the prediction efficiency of the model (14, 98, 107, 108). Li et al. (101) found that tumor volume–based IVIM histogram analysis can be used to predict MVI and that the fifth percentile of the true diffusion coefficient is most beneficial to predict MVI of HCC. Zhang et al. (107) extracted imaging features based on preoperative multimodal MR images and constructed an MVI prediction model (combined model) by combining the clinical features and qualitative imaging features of patients with HCC. The AUC in the validation cohort of their combined model was 0.858, which was higher than the AUC (0.820) in the validation cohort of the model constructed from individual radiomic features, indicating that the prediction efficiency of the combined model was higher. Song D et al. (104) predicted MVI using radiomics and DL in 601 patients with HCC based on preoperative MRI. Their results showed that the radiomics model achieved an AUC of 0.731, the DL model based only on MRI images achieved an AUC of 0.915, and a DL model combined with clinical parameters achieved an AUC of 0.931. These studies indicated that the model combining radiomics, DL, and clinical parameters showed the best predictive performance.
Radiogenomics
The biological behavior of a tumor is closely related to its gene expression profile. Biopsy is a widely used method to evaluate gene expression before surgery, but biopsy is an invasive examination that may cause bleeding and other complications. Therefore, patients are often unwilling to undergo this examination. In recent years, radiogenomics has gradually become more widely applied in HCC research. The purpose of radiogenomics is to determine the relationship between semantic and quantitative image data and genomic and molecular measurements, thus constructing correlation diagrams related to results or other clinical measurements (33, 109, 110). Segal et al. (111) evaluated the correlation between radiogenomic features and the liver cancer gene phenotype and reported that 78% of liver cancer gene expression profiles could be reconstructed by this combination of features. To date, radiogenomics studies have described the semantic features obtained from MRI (112–115). MRI radiogenomics has the value of predicting gene features with prognostic and therapeutic significance (16, 17, 116–123). Taouli et al. (113) found that there was a strong connection between imaging features, such as the “infiltrative pattern”, “mosaic appearance”, and “presence of macrovascular invasion”, and an aggressive genomic signature determined previously. Shi et al. (82) found that histogram indices extracted from IVIM parameter maps could predict Ki-67 expression. Jun et al. (124) used an immunohistochemical method to detect the expression of programmed cell death-1 (PD-1) and programmed cell death ligand-1 (PD-L1) in 98 ICC patients and extracted radiological features from the arterial phase and portal venous phase of preoperative MR images. The results indicated that the AUCs of the models for predicting PD-1 and PD-L1 expression were 0.897 and 0.897, respectively. The prognoses of PD-1-positive and PD-L1-positive patients were worse than those of PD-1-negative and PD-L1-negative patients, and their 5-year survival rates were 12.5%, 48.3%, 21.9%, and 39.4%, respectively (P < 0.05). The results indicated that MRI radiomics could be used as a noninvasive biomarker to evaluate the expression of PD-1 and PD-L1 and the prognosis of ICC patients (Table 1).
Prediction of Relapse and Prognosis After Surgical Resection
Surgical resection is still the main treatment for patients with early HCC (125). However, tumor relapse is still the main cause of postoperative death, and the 5-year relapse rate after surgery is close to 70% (126). Improving the ability to preoperatively identify these high-risk patients will guide surgical management, postoperative monitoring, and treatment intervention (127, 128). The radiomic model based on preoperative MRI can be used as a new tool to predict early relapse (18, 19, 129–134), relapse-free survival (135) and overall survival (OS) (136, 137) in patients with HCC after surgery. Hui et al. (130) used preoperative MRI to extract 290 texture parameters to predict the relapse of HCC patients within 730 days after surgical resection. The results showed that the prediction accuracy of texture features based on dynamic contrast-enhanced MRI in the equilibrium phase was 84%. Combining clinical, laboratory, and radiomic data can improve the performance of quantitative models (20, 129, 135, 136, 138). According to Kim et al. (135), the combined clinical and radiomic model had the same performance as the clinicopathological model in predicting early relapse. Zhang et al. evaluated the effectiveness of contrast-enhanced MRI radiomic features in predicting the OS of HCC patients after resection. Their results showed that preoperative clinical features and semantic imaging features were significantly correlated with survival rate; the Barcelona Clinic Liver Cancer stage, uneven tumor margin, and combined rad-score were independently correlated with OS; and the combined model incorporating radiological and radiomic features had a better prediction performance than the clinic-radiological model (136).
Prediction of Response to TACE
TACE is recognized as an effective treatment for advanced HCC (125), but its long-term efficacy needs to be further improved (139–141). MRI radiomics can be used to predict the response to TACE treatment and provide a reference for the formulation of individualized treatment plans (21, 22, 142–148). Sun et al. (142)predicted the risk of early postoperative progression based on multiparameter MRI data before TACE. The results showed that the AUC of the model based on DWI features was 0.786 and 0.729 when b=0 and b=500, respectively, followed by the AUC of T2-weighted imaging features (0.729) and the apparent diffusion coefficient (0.714). Compared with any single MRI signal, the MP-MRI signal had a higher AUC, at 0.800. Song et al. (143) revealed that their combined model incorporating radiomic features and clinical radiation risk factors had the best predictive value (C = 0.802).
Prediction of the Systemic Treatment Efficacy
The treatment of HCC has been a challenge. Systemic therapies for HCC are current research hotspots. Targeted therapy with sorafenib (149) and lenvatinib (150) and immunotherapy with immune checkpoint inhibitors, especially antibodies against PD-1/PD-L1 pathway members (nivolumab and pembrolizumab), have achieved excellent clinical results (151–158). These results strongly indicated that immune checkpoint inhibitor-based strategies will soon be primary method in the treatment of advanced HCC, and immunotherapy will introduce a new era of HCC therapy. Traditional contrast-enhanced CT and MRI, including functional imaging, are the most commonly used biomarkers for evaluating the therapeutic response in clinical practice (159–172). Research based on contrast-enhanced CT and MR images has shown the value of radiomics and DL in predicting systemic treatment efficacy for advanced HCC (23, 173–175). Mulé et al. (174) analyzed the CT texture features of 92 patients before receiving sorafenib and found that the entropy of portal phase-derived entropy at fine texture scales was an independent predictor of OS, which was confirmed in their validation cohort. Yuan et al. (173) established a radiomics nomogram and measured its ability to evaluate the therapeutic efficacy of anti-PD-1antibodies in the treatment of HCC by combining pretreatment contrast-enhanced CT images and clinical risk factors. The results indicated that the AUCs of the radiomics nomogram were 0.894 and 0.883 in the training and validation cohorts, respectively.
In recent years, MRI radiomics has gradually become more widely applied to systemic treatment evaluation of brain tumors (176, 177). There are still no reports on using MRI radiomics to evaluate the systemic treatments of patients with HCC. We believe that as research progresses, MRI radiomics will play an important role in the evaluation of systemic treatments for HCC in the near future.
Conclusion
As a new technology, radiomics can improve the diagnosis and differentiation of HCC, as well as predictions of the stage, histological grade, MVI, gene expression, treatment response, and prognosis of HCC. This is because it allows us to analyze the relationship between high-dimensional quantitative imaging features and clinical and genetic data. Moreover, it is a powerful tool for making personalized treatment decisions before surgery. With the rapid development of targeted therapy and immunotherapy for HCC, radiomics is expected to become a reliable radiological marker for predicting the therapeutic targets and therapeutic responses of HCC patients.
There are still some challenges and limitations in the clinical application of radiomics. First, a key challenge is to ensure that the academic community can obtain high-quality radiological and clinical resources that involve the establishment and promotion of imaging and clinical data acquisition protocols. Second, the analytical methods of radiomics need to be standardized. Third, many radiomics studies are retrospective, whereas a prospective research design is ideal. As technology advances and research progresses, MRI radiomics will play a more important and even irreplaceable role in the diagnosis and treatment of HCC.
Author Contributions
X-QG and LY wrote the paper. Y-YT, Y-KW, NinL, XY, RW, JZ, and GY contributed to the literature search and manuscript preparation. NiaL and X-HH revised the paper. X-QW performed the data analysis and created graphs. X-MZ and J-DL designed the research. All authors contributed to the article and approved the submitted version.
Funding
This work was supported by the Project of Medical Association of Sichuan Province (No. S20070) and the Project of City-University Science and Technology Strategic Cooperation of Nanchong City (North Sichuan Medical College) (No. 20SXQT0324).
Conflict of Interest
The authors declare that the research was conducted in the absence of any commercial or financial relationships that could be construed as a potential conflict of interest.
Publisher’s Note
All claims expressed in this article are solely those of the authors and do not necessarily represent those of their affiliated organizations, or those of the publisher, the editors and the reviewers. Any product that may be evaluated in this article, or claim that may be made by its manufacturer, is not guaranteed or endorsed by the publisher.
References
1. Sung H, Ferlay J, Siegel RL, Laversanne M, Soerjomataram I, Jemal A, et al. Global Cancer Statistics 2020: GLOBOCAN Estimates of Incidence and Mortality Worldwide for 36 Cancers in 185 Countries. CA Cancer J Clin (2021) 71:209–49. doi: 10.3322/caac.21660
2. Hiley C, de Bruin EC, McGranahan N, Swanton C. Deciphering Intratumor Heterogeneity and Temporal Acquisition of Driver Events to Refine Precision Medicine. Genome Biol (2014) 15:453. doi: 10.1186/s13059-014-0453-8
3. Hao K, Lamb J, Zhang C, Xie T, Wang K, Zhang B, et al. Clinicopathologic and Gene Expression Parameters Predict Liver Cancer Prognosis. BMC Cancer (2011) 11:481. doi: 10.1186/1471-2407-11-481
4. Hao K, Luk JM, Lee NP, Mao M, Zhang C, Ferguson MD, et al. Predicting Prognosis in Hepatocellular Carcinoma After Curative Surgery With Common Clinicopathologic Parameters. BMC Cancer (2009) 9:389. doi: 10.1186/1471-2407-9-389
5. Hood L, Friend SH. Predictive, Personalized, Preventive, Participatory (P4) Cancer Medicine. Nat Rev Clin Oncol (2011) 8:184–7. doi: 10.1038/nrclinonc.2010.227
6. Lambin P, Rios-Velazquez E, Leijenaar R, Carvalho S, van Stiphout RG, Granton P, et al. Radiomics: Extracting More Information From Medical Images Using Advanced Feature Analysis. Eur J Cancer (2012) 48:441–6. doi: 10.1016/j.ejca.2011.11.036
7. Aerts HJ, Velazquez ER, Leijenaar RT, Parmar C, Grossmann P, Carvalho S, et al. Decoding Tumour Phenotype by Noninvasive Imaging Using a Quantitative Radiomics Approach. Nat Commun (2014) 5:4006. doi: 10.1038/ncomms5006
8. Sagir KA. Radiomics in Hepatocellular Carcinoma. J Gastrointest Cancer (2020) 51:1165–8. doi: 10.1007/s12029-020-00493-x
9. Wei J, Jiang H, Gu D, Niu M, Fu F, Han Y, et al. Radiomics in Liver Diseases: Current Progress and Future Opportunities. Liver Int (2020) 40:2050–63. doi: 10.1111/liv.14555
10. Masokano IB, Liu W, Xie S, Marcellin D, Pei Y, Li W. The Application of Texture Quantification in Hepatocellular Carcinoma Using CT and MRI: A Review of Perspectives and Challenges. Cancer Imag (2020) 20:67. doi: 10.1186/s40644-020-00341-y
11. Wakabayashi T, Ouhmich F, Gonzalez-Cabrera C, Felli E, Saviano A, Agnus V, et al. Radiomics in Hepatocellular Carcinoma: A Quantitative Review. Hepatol Int (2019) 13:546–59. doi: 10.1007/s12072-019-09973-0
12. Geng Z, Zhang Y, Wang S, Li H, Zhang C, Yin S, et al. Radiomics Analysis of Susceptibility Weighted Imaging for Hepatocellular Carcinoma: Exploring the Correlation Between Histopathology and Radiomics Features. Magn Reson Med Sci (2020) 20:253–63. doi: 10.2463/mrms.mp.2020-0060
13. Feng M, Zhang M, Liu Y, Jiang N, Meng Q, Wang J, et al. Texture Analysis of MR Images to Identify the Differentiated Degree in Hepatocellular Carcinoma: A Retrospective Study. BMC Cancer (2020) 20:611. doi: 10.1186/s12885-020-07094-8
14. Chong HH, Yang L, Sheng RF, Yu YL, Wu DJ, Rao SX, et al. Multi-Scale and Multi-Parametric Radiomics of Gadoxetate Disodium-Enhanced MRI Predicts Microvascular Invasion and Outcome in Patients With Solitary Hepatocellular Carcinoma ≤ 5 Cm. Eur Radiol (2021) 31:4824–38. doi: 10.1007/s00330-020-07601-2
15. Chen Y, Xia Y, Tolat PP, Long L, Jiang Z, Huang Z, et al. Comparison of Conventional Gadoxetate Disodium-Enhanced MRI Features and Radiomics Signatures With Machine Learning for Diagnosing Microvascular Invasion. AJR Am J Roentgenol (2021) 216:1510–20. doi: 10.2214/AJR.20.23255
16. Fan Y, Yu Y, Wang X, Hu M, Du M, Guo L, et al. Texture Analysis Based on Gd-EOB-DTPA-Enhanced MRI for Identifying Vessels Encapsulating Tumor Clusters (VETC)-Positive Hepatocellular Carcinoma. J Hepatocell Carcinoma (2021) 8:349–59. doi: 10.2147/JHC.S293755
17. Wang W, Gu D, Wei J, Ding Y, Yang L, Zhu K, et al. A Radiomics-Based Biomarker for Cytokeratin 19 Status of Hepatocellular Carcinoma With Gadoxetic Acid-Enhanced MRI. Eur Radiol (2020) 30:3004–14. doi: 10.1007/s00330-019-06585-y
18. Wen L, Weng S, Yan C, Ye R, Zhu Y, Zhou L, et al. A Radiomics Nomogram for Preoperative Prediction of Early Recurrence of Small Hepatocellular Carcinoma After Surgical Resection or Radiofrequency Ablation. Front Oncol (2021) 11:657039. doi: 10.3389/fonc.2021.657039
19. Chong H, Gong Y, Pan X, Liu A, Chen L, Yang C, et al. Peritumoral Dilation Radiomics of Gadoxetate Disodium-Enhanced MRI Excellently Predicts Early Recurrence of Hepatocellular Carcinoma Without Macrovascular Invasion After Hepatectomy. J Hepatocell Carcinoma (2021) 8:545–63. doi: 10.2147/JHC.S309570
20. Zhang L, Hu J, Hou J, Jiang X, Guo L, Tian L. Radiomics-Based Model Using Gadoxetic Acid Disodium-Enhanced MR Images: Associations With Recurrence-Free Survival of Patients With Hepatocellular Carcinoma Treated by Surgical Resection. Abdom Radiol (NY) (2021) 46:3845–54. doi: 10.1007/s00261-021-03034-7
21. Kuang Y, Li R, Jia P, Ye W, Zhou R, Zhu R, et al. MRI-Based Radiomics: Nomograms Predicting the Short-Term Response After Transcatheter Arterial Chemoembolization (TACE) in Hepatocellular Carcinoma Patients With Diameter Less Than 5 Cm. Abdom Radiol (NY) (2021) 46:3772–89. doi: 10.1007/s00261-021-02992-2
22. Zhao Y, Wang N, Wu J, Zhang Q, Lin T, Yao Y, et al. Radiomics Analysis Based on Contrast-Enhanced MRI for Prediction of Therapeutic Response to Transarterial Chemoembolization in Hepatocellular Carcinoma. Front Oncol (2021) 11:582788. doi: 10.3389/fonc.2021.582788
23. Zhang L, Xia W, Yan ZP, Sun JH, Zhong BY, Hou ZH, et al. Deep Learning Predicts Overall Survival of Patients With Unresectable Hepatocellular Carcinoma Treated by Transarterial Chemoembolization Plus Sorafenib. Front Oncol (2020) 10:593292. doi: 10.3389/fonc.2020.593292
24. Huang B, Wu L, Lu XY, Xu F, Liu CF, Shen WF, et al. Small Intrahepatic Cholangiocarcinoma and Hepatocellular Carcinoma in Cirrhotic Livers May Share Similar Enhancement Patterns at Multiphase Dynamic MR Imaging. Radiology (2016) 281:150–7. doi: 10.1148/radiol.2016151205
25. Lambin P, Leijenaar R, Deist TM, Peerlings J, de Jong E, van Timmeren J, et al. Radiomics: The Bridge Between Medical Imaging and Personalized Medicine. Nat Rev Clin Oncol (2017) 14:749–62. doi: 10.1038/nrclinonc.2017.141
26. Saha A, Yu X, Sahoo D, Mazurowski MA. Effects of MRI Scanner Parameters on Breast Cancer Radiomics. Expert Syst Appl (2017) 87:384–91. doi: 10.1016/j.eswa.2017.06.029
27. Maruyama T, Hayashi N, Sato Y, Hyuga S, Wakayama Y, Watanabe H, et al. Comparison of Medical Image Classification Accuracy Among Three Machine Learning Methods. J Xray Sci Technol (2018) 26:885–93. doi: 10.3233/XST-18386
28. Lu L, Ehmke RC, Schwartz LH, Zhao B. Assessing Agreement Between Radiomic Features Computed for Multiple CT Imaging Settings. PloS One (2016) 11:e0166550. doi: 10.1371/journal.pone.0166550
29. Ger RB, Zhou S, Chi PM, Lee HJ, Layman RR, Jones AK, et al. Comprehensive Investigation on Controlling for CT Imaging Variabilities in Radiomics Studies. Sci Rep (2018) 8:13047. doi: 10.1038/s41598-018-31509-z
30. Polan DF, Brady SL, Kaufman RA. Tissue Segmentation of Computed Tomography Images Using a Random Forest Algorithm: A Feasibility Study. Phys Med Biol (2016) 61:6553–69. doi: 10.1088/0031-9155/61/17/6553
31. Massoptier L, Casciaro S. A New Fully Automatic and Robust Algorithm for Fast Segmentation of Liver Tissue and Tumors From CT Scans. Eur Radiol (2008) 18:1658–65. doi: 10.1007/s00330-008-0924-y
32. Häme Y, Pollari M. Semi-Automatic Liver Tumor Segmentation With Hidden Markov Measure Field Model and Non-Parametric Distribution Estimation. Med Image Anal (2012) 16:140–9. doi: 10.1016/j.media.2011.06.006
33. Gillies RJ, Kinahan PE, Hricak H. Radiomics: Images Are More Than Pictures, They Are Data. Radiology (2016) 278:563–77. doi: 10.1148/radiol.2015151169
34. Yang F, Ford JC, Dogan N, Padgett KR, Breto AL, Abramowitz MC, et al. Magnetic Resonance Imaging (MRI)-Based Radiomics for Prostate Cancer Radiotherapy. Transl Androl Urol (2018) 7:445–58. doi: 10.21037/tau.2018.06.05
35. Just N. Improving Tumour Heterogeneity MRI Assessment With Histograms. Br J Cancer (2014) 111:2205–13. doi: 10.1038/bjc.2014.512
36. Bagherzadeh-Khiabani F, Ramezankhani A, Azizi F, Hadaegh F, Steyerberg EW, Khalili D. A Tutorial on Variable Selection for Clinical Prediction Models: Feature Selection Methods in Data Mining Could Improve the Results. J Clin Epidemiol (2016) 71:76–85. doi: 10.1016/j.jclinepi.2015.10.002
37. Lewis S, Hectors S, Taouli B. Radiomics of Hepatocellular Carcinoma. Abdom Radiol (NY) (2021) 1:111–23. doi: 10.1007/s00261-019-02378-5
38. Liu Z, Wang S, Dong D, Wei J, Fang C, Zhou X, et al. The Applications of Radiomics in Precision Diagnosis and Treatment of Oncology: Opportunities and Challenges. Theranostics (2019) 9:1303–22. doi: 10.7150/thno.30309
39. Marrero JA, Kulik LM, Sirlin CB, Zhu AX, Finn RS, Abecassis MM, et al. Diagnosis, Staging, and Management of Hepatocellular Carcinoma: 2018 Practice Guidance by the American Association for the Study of Liver Diseases. Hepatology (2018) 68:723–50. doi: 10.1002/hep.29913
40. EASL Clinical Practice Guidelines. Management of Hepatocellular Carcinoma. J Hepatol (2018) 69:182–236. doi: 10.1016/j.jhep.2018.03.019
41. Kokudo N, Hasegawa K, Akahane M, Igaki H, Izumi N, Ichida T, et al. Evidence-Based Clinical Practice Guidelines for Hepatocellular Carcinoma: The Japan Society of Hepatology 2013 Update (3rd JSH-HCC Guidelines). Hepatol Res (2015) 45:123–7. doi: 10.1111/hepr.12464
42. Omata M, Cheng AL, Kokudo N, Kudo M, Lee JM, Jia J, et al. Asia-Pacific Clinical Practice Guidelines on the Management of Hepatocellular Carcinoma: A 2017 Update. Hepatol Int (2017) 11:317–70. doi: 10.1007/s12072-017-9799-9
43. Chernyak V, Fowler KJ, Kamaya A, Kielar AZ, Elsayes KM, Bashir MR, et al. Liver Imaging Reporting and Data System (LI-RADS) Version 2018: Imaging of Hepatocellular Carcinoma in At-Risk Patients. Radiology (2018) 289:816–30. doi: 10.1148/radiol.2018181494
44. Fraum TJ, Tsai R, Rohe E, Ludwig DR, Salter A, Nalbantoglu I, et al. Differentiation of Hepatocellular Carcinoma From Other Hepatic Malignancies in Patients at Risk: Diagnostic Performance of the Liver Imaging Reporting and Data System Version 2014. Radiology (2018) 286:158–72. doi: 10.1148/radiol.2017170114
45. Kierans AS, Makkar J, Guniganti P, Cornman-Homonoff J, Lee MJ, Pittman M, et al. Validation of Liver Imaging Reporting and Data System 2017 (LI-RADS) Criteria for Imaging Diagnosis of Hepatocellular Carcinoma. J Magn Reson Imag (2019) 49:e205–15. doi: 10.1002/jmri.26329
46. Jian J, Li Y, Pickhardt PJ, Xia W, He Z, Zhang R, et al. MR Image-Based Radiomics to Differentiate Type ι and Type ιι Epithelial Ovarian Cancers. Eur Radiol (2021) 31:403–10. doi: 10.1007/s00330-020-07091-2
47. Song XL, Ren JL, Zhao D, Wang L, Ren H, Niu J. Radiomics Derived From Dynamic Contrast-Enhanced MRI Pharmacokinetic Protocol Features: The Value of Precision Diagnosis Ovarian Neoplasms. Eur Radiol (2021) 31:368–78. doi: 10.1007/s00330-020-07112-0
48. Xia W, Hu B, Li H, Geng C, Wu Q, Yang L, et al. Multiparametric-MRI-Based Radiomics Model for Differentiating Primary Central Nervous System Lymphoma From Glioblastoma: Development and Cross-Vendor Validation. J Magn Reson Imag (2021) 53:242–50. doi: 10.1002/jmri.27344
49. Tagliafico AS, Piana M, Schenone D, Lai R, Massone AM, Houssami N. Overview of Radiomics in Breast Cancer Diagnosis and Prognostication. Breast (2020) 49:74–80. doi: 10.1016/j.breast.2019.10.018
50. Mokrane FZ, Lu L, Vavasseur A, Otal P, Peron JM, Luk L, et al. Radiomics Machine-Learning Signature for Diagnosis of Hepatocellular Carcinoma in Cirrhotic Patients With Indeterminate Liver Nodules. Eur Radiol (2020) 30:558–70. doi: 10.1007/s00330-019-06347-w
51. Jiang H, Liu X, Chen J, Wei Y, Lee JM, Cao L, et al. Man or Machine? Prospective Comparison of the Version 2018 EASL, LI-RADS Criteria and a Radiomics Model to Diagnose Hepatocellular Carcinoma. Cancer Imag (2019) 19:84. doi: 10.1186/s40644-019-0266-9
52. Wu J, Liu A, Cui J, Chen A, Song Q, Xie L. Radiomics-Based Classification of Hepatocellular Carcinoma and Hepatic Haemangioma On Precontrast Magnetic Resonance Images. BMC Med Imag (2019) 19:23. doi: 10.1186/s12880-019-0321-9
53. Li J, Xue F, Xu X, Wang Q, Zhang X. Dynamic Contrast-Enhanced MRI Differentiates Hepatocellular Carcinoma From Hepatic Metastasis of Rectal Cancer by Extracting Pharmacokinetic Parameters and Radiomic Features. Exp Ther Med (2020) 20:3643–52. doi: 10.3892/etm.2020.9115
54. Liu X, Khalvati F, Namdar K, Fischer S, Lewis S, Taouli B, et al. Can Machine Learning Radiomics Provide Pre-Operative Differentiation of Combined Hepatocellular Cholangiocarcinoma From Hepatocellular Carcinoma and Cholangiocarcinoma to Inform Optimal Treatment Planning? Eur Radiol (2021) 31:244–55. doi: 10.1007/s00330-020-07119-7
55. Oyama A, Hiraoka Y, Obayashi I, Saikawa Y, Furui S, Shiraishi K, et al. Hepatic Tumor Classification Using Texture and Topology Analysis of Non-Contrast-Enhanced Three-Dimensional T1-Weighted MR Images With a Radiomics Approach. Sci Rep (2019) 9:8764. doi: 10.1038/s41598-019-45283-z
56. Ai Z, Han Q, Huang Z, Wu J, Xiang Z. The Value of Multiparametric Histogram Features Based on Intravoxel Incoherent Motion Diffusion-Weighted Imaging (IVIM-DWI) for the Differential Diagnosis of Liver Lesions. Ann Transl Med (2020) 8:1128. doi: 10.21037/atm-20-5109
57. Zhong X, Guan T, Tang D, Li J, Lu B, Cui S, et al. Differentiation of Small (≤ 3 Cm) Hepatocellular Carcinomas From Benign Nodules in Cirrhotic Liver: The Added Additive Value of MRI-Based Radiomics Analysis to LI-RADS Version 2018 Algorithm. BMC Gastroenterol (2021) 21:155. doi: 10.1186/s12876-021-01710-y
58. Liang W, Shao J, Liu W, Ruan S, Tian W, Zhang X, et al. Differentiating Hepatic Epithelioid Angiomyolipoma From Hepatocellular Carcinoma and Focal Nodular Hyperplasia via Radiomics Models. Front Oncol (2020) 10:564307. doi: 10.3389/fonc.2020.564307
59. Li Z, Mao Y, Huang W, Li H, Zhu J, Li W, et al. Texture-Based Classification of Different Single Liver Lesion Based on SPAIR T2W MRI Images. BMC Med Imag (2017) 17:42. doi: 10.1186/s12880-017-0212-x
60. Nakanuma Y, Xu J, Harada K, Sato Y, Sasaki M, Ikeda H, et al. Pathological Spectrum of Intrahepatic Cholangiocarcinoma Arising in Non-Biliary Chronic Advanced Liver Diseases. Pathol Int (2011) 61:298–305. doi: 10.1111/j.1440-1827.2011.02665.x
61. Portolani N, Baiocchi GL, Coniglio A, Piardi T, Grazioli L, Benetti A, et al. Intrahepatic Cholangiocarcinoma and Combined Hepatocellular-Cholangiocarcinoma: A Western Experience. Ann Surg Oncol (2008) 15:1880–90. doi: 10.1245/s10434-008-9933-y
62. Fowler KJ, Sheybani A, Parker RR, Doherty S, BE M, Chapman WC, et al. Combined Hepatocellular and Cholangiocarcinoma (Biphenotypic) Tumors: Imaging Features and Diagnostic Accuracy of Contrast-Enhanced CT and MRI. AJR Am J Roentgenol (2013) 201:332–9. doi: 10.2214/AJR.12.9488
63. Potretzke TA, Tan BR, Doyle MB, Brunt EM, Heiken JP, Fowler KJ. Imaging Features of Biphenotypic Primary Liver Carcinoma (Hepatocholangiocarcinoma) and the Potential to Mimic Hepatocellular Carcinoma: LI-RADS Analysis of CT and MRI Features in 61 Cases. AJR Am J Roentgenol (2016) 207:25–31. doi: 10.2214/AJR.15.14997
64. Sheng RF, Xie YH, Ji Y, Chen CZ, Yang L, Jin KP, et al. MR Comparative Study of Combined Hepatocellular-Cholangiocarcinoma in Normal, Fibrotic, and Cirrhotic Livers. Abdom Radiol (NY) (2016) 41:2102–14. doi: 10.1007/s00261-016-0811-y
65. Hamm CA, Wang CJ, Savic LJ, Ferrante M, Schobert I, Schlachter T, et al. Deep Learning for Liver Tumor Diagnosis Part I: Development of a Convolutional Neural Network Classifier for Multi-Phasic MRI. Eur Radiol (2019) 29:3338–47. doi: 10.1007/s00330-019-06205-9
66. Azer SA. Deep Learning With Convolutional Neural Networks for Identification of Liver Masses and Hepatocellular Carcinoma: A Systematic Review. World J Gastrointest Oncol (2019) 11:1218–30. doi: 10.4251/wjgo.v11.i12.1218
67. Oestmann PM, Wang CJ, Savic LJ, Hamm CA, Stark S, Schobert I, et al. Deep Learning-Assisted Differentiation of Pathologically Proven Atypical and Typical Hepatocellular Carcinoma (HCC) Versus Non-HCC on Contrast-Enhanced MRI of the Liver. Eur Radiol (2021) 31:4981–90. doi: 10.1007/s00330-020-07559-1
68. Bousabarah K, Letzen B, Tefera J, Savic L, Schobert I, Schlachter T, et al. Automated Detection and Delineation of Hepatocellular Carcinoma on Multiphasic Contrast-Enhanced MRI Using Deep Learning. Abdom Radiol (NY) (2021) 46:216–25. doi: 10.1007/s00261-020-02604-5
69. Kim J, Min JH, Kim SK, Shin SY, Lee MW. Detection of Hepatocellular Carcinoma in Contrast-Enhanced Magnetic Resonance Imaging Using Deep Learning Classifier: A Multi-Center Retrospective Study. Sci Rep (2020) 10:9458. doi: 10.1038/s41598-020-65875-4
70. Zheng R, Wang L, Wang C, Yu X, Chen W, Li Y, et al. Feasibility of Automatic Detection of Small Hepatocellular Carcinoma (≤2 Cm) in Cirrhotic Liver Based on Pattern Matching and Deep Learning. Phys Med Biol (2021) 66:5014. doi: 10.1088/1361-6560/abf2f8
71. Wu Y, White GM, Cornelius T, Gowdar I, Ansari MH, Supanich MP, et al. Deep Learning LI-RADS Grading System Based on Contrast Enhanced Multiphase MRI for Differentiation Between LR-3 and LR-4/LR-5 Liver Tumors. Ann Transl Med (2020) 8:701. doi: 10.21037/atm.2019.12.151
72. Sasaki A, Kai S, Iwashita Y, Hirano S, Ohta M, Kitano S. Microsatellite Distribution and Indication for Locoregional Therapy in Small Hepatocellular Carcinoma. Cancer-Am Cancer Soc (2005) 103:299–306. doi: 10.1002/cncr.20798
73. Ng IO, Lai EC, Fan ST, Ng MM, So MK. Prognostic Significance of Pathologic Features of Hepatocellular Carcinoma. A Multivariate Analysis of 278 Patients. Cancer-Am Cancer Soc (1995) 76:2443–8. doi: 10.1002/1097-0142(19951215)76:12<2443::aid-cncr2820761207>3.0.co;2-f
74. Okusaka T, Okada S, Ueno H, Ikeda M, Shimada K, Yamamoto J, et al. Satellite Lesions in Patients With Small Hepatocellular Carcinoma With Reference to Clinicopathologic Features. Cancer-Am Cancer Soc (2002) 95:1931–7. doi: 10.1002/cncr.10892
75. Bruix J, Sherman M. Management of Hepatocellular Carcinoma. Hepatology (2005) 42:1208–36. doi: 10.1002/hep.20933
76. Wu M, Tan H, Gao F, Hai J, Ning P, Chen J, et al. Predicting the Grade of Hepatocellular Carcinoma Based on Non-Contrast-Enhanced MRI Radiomics Signature. Eur Radiol (2019) 29:2802–11. doi: 10.1007/s00330-018-5787-2
77. Zhou W, Zhang L, Wang K, Chen S, Wang G, Liu Z, et al. Malignancy Characterization of Hepatocellular Carcinomas Based on Texture Analysis Of Contrast-Enhanced MR Images. J Magn Reson Imag (2017) 45:1476–84. doi: 10.1002/jmri.25454
78. Yang X, Yuan C, Zhang Y, Wang Z. Magnetic Resonance Radiomics Signatures for Predicting Poorly Differentiated Hepatocellular Carcinoma: A SQUIRE-Compliant Study. Med (Baltimore) (2021) 100:e25838. doi: 10.1097/MD.0000000000025838
79. Choi JM, Yu JS, Cho ES, Kim JH, Chung JJ. Texture Analysis of Hepatocellular Carcinoma on Magnetic Resonance Imaging: Assessment for Performance in Predicting Histopathologic Grade. J Comput Assist Tomogr (2020) 44:901–10. doi: 10.1097/RCT.0000000000001087
80. Zhou Q, Zhou Z, Chen C, Fan G, Chen G, Heng H, et al. Grading of Hepatocellular Carcinoma Using 3D SE-DenseNet in Dynamic Enhanced MR Images. Comput Biol Med (2019) 107:47–57. doi: 10.1016/j.compbiomed.2019.01.026
81. Xu YS, Liu HF, Xi DL, Li JK, Liu Z, Yan RF, et al. Whole-Lesion Histogram Analysis Metrics of the Apparent Diffusion Coefficient: A Correlation Study With Histological Grade of Hepatocellular Carcinoma. Abdom Radiol (NY) (2019) 44:3089–98. doi: 10.1007/s00261-019-02109-w
82. Shi G, Han X, Wang Q, Ding Y, Liu H, Zhang Y, et al. Evaluation of Multiple Prognostic Factors of Hepatocellular Carcinoma With Intra-Voxel Incoherent Motions Imaging by Extracting the Histogram Metrics. Cancer Manag Res (2020) 12:6019–31. doi: 10.2147/CMAR.S262973
83. Gouw AS, Balabaud C, Kusano H, Todo S, Ichida T, Kojiro M. Markers for Microvascular Invasion in Hepatocellular Carcinoma: Where Do We Stand? Liver Transpl (2011) 17 Suppl 2:S72–80. doi: 10.1002/lt.22368
84. Shi M, Guo RP, Lin XJ, Zhang YQ, Chen MS, Zhang CQ, et al. Partial Hepatectomy With Wide Versus Narrow Resection Margin for Solitary Hepatocellular Carcinoma: A Prospective Randomized Trial. Ann Surg (2007) 245:36–43. doi: 10.1097/01.sla.0000231758.07868.71
85. Sun JJ, Wang K, Zhang CZ, Guo WX, Shi J, Cong WM, et al. Postoperative Adjuvant Transcatheter Arterial Chemoembolization After R0 Hepatectomy Improves Outcomes of Patients Who Have Hepatocellular Carcinoma With Microvascular Invasion. Ann Surg Oncol (2016) 23:1344–51. doi: 10.1245/s10434-015-5008-z
86. Renzulli M, Brocchi S, Cucchetti A, Mazzotti F, Mosconi C, Sportoletti C, et al. Can Current Preoperative Imaging Be Used to Detect Microvascular Invasion of Hepatocellular Carcinoma? Radiology (2016) 279:432–42. doi: 10.1148/radiol.2015150998
87. Lee S, Kim SH, Lee JE, Sinn DH, Park CK. Preoperative Gadoxetic Acid-Enhanced MRI for Predicting Microvascular Invasion in Patients With Single Hepatocellular Carcinoma. J Hepatol (2017) 67:526–34. doi: 10.1016/j.jhep.2017.04.024
88. Ahn SY, Lee JM, Joo I, Lee ES, Lee SJ, Cheon GJ, et al. Prediction of Microvascular Invasion of Hepatocellular Carcinoma Using Gadoxetic Acid-Enhanced MR and (18)F-FDG PET/CT. Abdom Imag (2015) 40:843–51. doi: 10.1007/s00261-014-0256-0
89. Kim JY, Kim MJ, Kim KA, Jeong HT, Park YN. Hyperintense HCC on Hepatobiliary Phase Images of Gadoxetic Acid-Enhanced MRI: Correlation With Clinical and Pathological Features. Eur J Radiol (2012) 81:3877–82. doi: 10.1016/j.ejrad.2012.07.021
90. Kim KA, Kim MJ, Jeon HM, Kim KS, Choi JS, Ahn SH, et al. Prediction of Microvascular Invasion of Hepatocellular Carcinoma: Usefulness of Peritumoral Hypointensity Seen on Gadoxetate Disodium-Enhanced Hepatobiliary Phase Images. J Magn Reson Imag (2012) 35:629–34. doi: 10.1002/jmri.22876
91. Huang M, Liao B, Xu P, Cai H, Huang K, Dong Z, et al. Prediction of Microvascular Invasion in Hepatocellular Carcinoma: Preoperative Gd-EOB-DTPA-Dynamic Enhanced MRI and Histopathological Correlation. Contrast Media Mol Imag (2018) 2018:9674565. doi: 10.1155/2018/9674565
92. Meng XP, Wang YC, Zhou JY, Yu Q, Lu CQ, Xia C, et al. Comparison of MRI and CT for the Prediction of Microvascular Invasion in Solitary Hepatocellular Carcinoma Based on a Non-Radiomics and Radiomics Method: Which Imaging Modality Is Better? J Magn Reson Imag (2021) 54:526–36. doi: 10.1002/jmri.27575
93. Zhang Y, Shu Z, Ye Q, Chen J, Zhong J, Jiang H, et al. Preoperative Prediction of Microvascular Invasion in Hepatocellular Carcinoma via Multi-Parametric MRI Radiomics. Front Oncol (2021) 11:633596. doi: 10.3389/fonc.2021.633596
94. Dai H, Lu M, Huang B, Tang M, Pang T, Liao B, et al. Considerable Effects of Imaging Sequences, Feature Extraction, Feature Selection, and Classifiers on Radiomics-Based Prediction of Microvascular Invasion in Hepatocellular Carcinoma Using Magnetic Resonance Imaging. Quant Imaging Med Surg (2021) 11:1836–53. doi: 10.21037/qims-20-218
95. Zhang S, Xu G, Duan C, Zhou X, Wang X, Yu H, et al. Radiomics Analysis of MR Imaging With Gd-EOB-DTPA for Preoperative Prediction of Microvascular Invasion in Hepatocellular Carcinoma: Investigation and Comparison of Different Hepatobiliary Phase Delay Times. BioMed Res Int (2021) 2021:6685723. doi: 10.1155/2021/6685723
96. Tang M, Zhou Q, Huang M, Sun K, Wu T, Li X, et al. Nomogram Development and Validation to Predict Hepatocellular Carcinoma Tumor Behavior by Preoperative Gadoxetic Acid-Enhanced MRI. Eur Radiol (2021). doi: 10.1007/s00330-021-07941-7
97. Wilson GC, Cannella R, Fiorentini G, Shen C, Borhani A, Furlan A, et al. Texture Analysis on Preoperative Contrast-Enhanced Magnetic Resonance Imaging Identifies Microvascular Invasion in Hepatocellular Carcinoma. HPB (Oxford) (2020) 22:1622–30. doi: 10.1016/j.hpb.2020.03.001
98. Nebbia G, Zhang Q, Arefan D, Zhao X, Wu S. Pre-Operative Microvascular Invasion Prediction Using Multi-Parametric Liver MRI Radiomics. J Digit Imag (2020) 33:1376–86. doi: 10.1007/s10278-020-00353-x
99. Yang L, Gu D, Wei J, Yang C, Rao S, Wang W, et al. A Radiomics Nomogram for Preoperative Prediction of Microvascular Invasion in Hepatocellular Carcinoma. Liver Cancer (2019) 8:373–86. doi: 10.1159/000494099
100. Zhu YJ, Feng B, Wang S, Wang LM, Wu JF, Ma XH, et al. Model-Based Three-Dimensional Texture Analysis of Contrast-Enhanced Magnetic Resonance Imaging as a Potential Tool for Preoperative Prediction of Microvascular Invasion in Hepatocellular Carcinoma. Oncol Lett (2019) 18:720–32. doi: 10.3892/ol.2019.10378
101. Li H, Zhang J, Zheng Z, Guo Y, Chen M, Xie C, et al. Preoperative Histogram Analysis of Intravoxel Incoherent Motion (IVIM) for Predicting Microvascular Invasion in Patients With Single Hepatocellular Carcinoma. Eur J Radiol (2018) 105:65–71. doi: 10.1016/j.ejrad.2018.05.032
102. Peng J, Zhang J, Zhang Q, Xu Y, Zhou J, Liu L. A Radiomics Nomogram for Preoperative Prediction of Microvascular Invasion Risk in Hepatitis B Virus-Related Hepatocellular Carcinoma. Diagn Interv Radiol (2018) 24:121–7. doi: 10.5152/dir.2018.17467
103. Song D, Wang Y, Wang W, Wang Y, Cai J, Zhu K, et al. Using Deep Learning to Predict Microvascular Invasion in Hepatocellular Carcinoma Based on Dynamic Contrast-Enhanced MRI Combined With Clinical Parameters. J Cancer Res Clin Oncol (2021). doi: 10.1007/s00432-021-03617-3
104. Zhang Y, Lv X, Qiu J, Zhang B, Zhang L, Fang J, et al. Deep Learning With 3d Convolutional Neural Network for Noninvasive Prediction of Microvascular Invasion in Hepatocellular Carcinoma. J Magn Reson Imag (2021) 54:134–43. doi: 10.1002/jmri.27538
105. Wei J, Jiang H, Zeng M, Wang M, Niu M, Gu D, et al. Prediction of Microvascular Invasion in Hepatocellular Carcinoma via Deep Learning: A Multi-Center and Prospective Validation Study. Cancers (Basel) (2021) 13:2368. doi: 10.3390/cancers13102368
106. Zhou W, Jian W, Cen X, Zhang L, Guo H, Liu Z, et al. Prediction of Microvascular Invasion of Hepatocellular Carcinoma Based on Contrast-Enhanced MR and 3D Convolutional Neural Networks. Front Oncol (2021) 11:588010. doi: 10.3389/fonc.2021.588010
107. Zhang R, Xu L, Wen X, Zhang J, Yang P, Zhang L, et al. A Nomogram Based on Bi-Regional Radiomics Features From Multimodal Magnetic Resonance Imaging for Preoperative Prediction of Microvascular Invasion in Hepatocellular Carcinoma. Quant Imaging Med Surg (2019) 9:1503–15. doi: 10.21037/qims.2019.09.07
108. Feng ST, Jia Y, Liao B, Huang B, Zhou Q, Li X, et al. Preoperative Prediction of Microvascular Invasion in Hepatocellular Cancer: A Radiomics Model Using Gd-EOB-DTPA-Enhanced MRI. Eur Radiol (2019) 29:4648–59. doi: 10.1007/s00330-018-5935-8
109. Bai HX, Lee AM, Yang L, Zhang P, Davatzikos C, Maris JM, et al. Imaging Genomics in Cancer Research: Limitations and Promises. Br J Radiol (2016) 89:20151030. doi: 10.1259/bjr.20151030
110. Pinker K, Shitano F, Sala E, Do RK, Young RJ, Wibmer AG, et al. Background, Current Role, and Potential Applications of Radiogenomics. J Magn Reson Imag (2018) 47:604–20. doi: 10.1002/jmri.25870
111. Segal E, Sirlin CB, Ooi C, Adler AS, Gollub J, Chen X, et al. Decoding Global Gene Expression Programs in Liver Cancer by Noninvasive Imaging. Nat Biotechnol (2007) 25:675–80. doi: 10.1038/nbt1306
112. Hectors SJ, Wagner M, Bane O, Besa C, Lewis S, Remark R, et al. Quantification of Hepatocellular Carcinoma Heterogeneity With Multiparametric Magnetic Resonance Imaging. Sci Rep (2017) 7:2452. doi: 10.1038/s41598-017-02706-z
113. Taouli B, Hoshida Y, Kakite S, Chen X, Tan PS, Sun X, et al. Imaging-Based Surrogate Markers of Transcriptome Subclasses and Signatures in Hepatocellular Carcinoma: Preliminary Results. Eur Radiol (2017) 27:4472–81. doi: 10.1007/s00330-017-4844-6
114. Kitao A, Matsui O, Yoneda N, Kozaka K, Kobayashi S, Sanada J, et al. Hepatocellular Carcinoma With β-Catenin Mutation: Imaging and Pathologic Characteristics. Radiology (2015) 275:708–17. doi: 10.1148/radiol.14141315
115. Yoneda N, Matsui O, Kobayashi S, Kitao A, Kozaka K, Inoue D, et al. Current Status of Imaging Biomarkers Predicting the Biological Nature of Hepatocellular Carcinoma. Jpn J Radiol (2019) 37:191–208. doi: 10.1007/s11604-019-00817-3
116. Hectors SJ, Lewis S, Besa C, King MJ, Said D, Putra J, et al. MRI Radiomics Features Predict Immuno-Oncological Characteristics of Hepatocellular Carcinoma. Eur Radiol (2020) 30:3759–69. doi: 10.1007/s00330-020-06675-2
117. Gu D, Xie Y, Wei J, Li W, Ye Z, Zhu Z, et al. MRI-Based Radiomics Signature: A Potential Biomarker for Identifying Glypican 3-Positive Hepatocellular Carcinoma. J Magn Reson Imag (2020) 52:1679–87. doi: 10.1002/jmri.27199
118. Ye Z, Jiang H, Chen J, Liu X, Wei Y, Xia C, et al. Texture Analysis on Gadoxetic Acid Enhanced-MRI for Predicting Ki-67 Status in Hepatocellular Carcinoma: A Prospective Study. Chin J Cancer Res (2019) 31:806–17. doi: 10.21147/j.issn.1000-9604.2019.05.10
119. Li Y, Yan C, Weng S, Shi Z, Sun H, Chen J, et al. Texture Analysis of Multi-Phase MRI Images to Detect Expression of Ki67 in Hepatocellular Carcinoma. Clin Radiol (2019) 74:813.e19–27. doi: 10.1016/j.crad.2019.06.024
120. Wang HQ, Yang C, Zeng MS, Rao SX, Ji Y, Weng X, et al. Magnetic Resonance Texture Analysis for the Identification of Cytokeratin 19-Positive Hepatocellular Carcinoma. Eur J Radiol (2019) 117:164–70. doi: 10.1016/j.ejrad.2019.06.016
121. Fan Y, Yu Y, Wang X, Hu M, Hu C. Radiomic Analysis of Gd-EOB-DTPA-Enhanced MRI Predicts Ki-67 Expression in Hepatocellular Carcinoma. BMC Med Imag (2021) 21:100. doi: 10.1186/s12880-021-00633-0
122. Huang X, Long L, Wei J, Li Y, Xia Y, Zuo P, et al. Radiomics for Diagnosis of Dual-Phenotype Hepatocellular Carcinoma Using Gd-EOB-DTPA-Enhanced MRI and Patient Prognosis. J Cancer Res Clin Oncol (2019) 145:2995–3003. doi: 10.1007/s00432-019-03062-3
123. Chen S, Feng S, Wei J, Liu F, Li B, Li X, et al. Pretreatment Prediction of Immunoscore in Hepatocellular Cancer: A Radiomics-Based Clinical Model Based on Gd-EOB-DTPA-Enhanced MRI Imaging. Eur Radiol (2019) 29:4177–87. doi: 10.1007/s00330-018-5986-x
124. Zhang J, Wu Z, Zhang X, Liu S, Zhao J, Yuan F, et al. Machine Learning: An Approach to Preoperatively Predict PD-1/PD-L1 Expression and Outcome in Intrahepatic Cholangiocarcinoma Using MRI Biomarkers. ESMO Open (2020) 5:e000910. doi: 10.1136/esmoopen-2020-000910
125. Bruix J, Reig M, Sherman M. Evidence-Based Diagnosis, Staging, and Treatment of Patients With Hepatocellular Carcinoma. Gastroenterology (2016) 150:835–53. doi: 10.1053/j.gastro.2015.12.041
126. Kulik L, El-Serag HB. Epidemiology and Management of Hepatocellular Carcinoma. Gastroenterology (2019) 156:477–91.e1. doi: 10.1053/j.gastro.2018.08.065
127. Chen Y, Liu Z, Mo Y, Li B, Zhou Q, Peng S, et al. Prediction of Post-Hepatectomy Liver Failure in Patients With Hepatocellular Carcinoma Based on Radiomics Using Gd-EOB-DTPA-Enhanced MRI: The Liver Failure Model. Front Oncol (2021) 11:605296. doi: 10.3389/fonc.2021.605296
128. Zhu WS, Shi SY, Yang ZH, Song C, Shen J. Radiomics Model Based on Preoperative Gadoxetic Acid-Enhanced MRI for Predicting Liver Failure. World J Gastroenterol (2020) 26:1208–20. doi: 10.3748/wjg.v26.i11.1208
129. Zhang Z, Jiang H, Chen J, Wei Y, Cao L, Ye Z, et al. Hepatocellular Carcinoma: Radiomics Nomogram on Gadoxetic Acid-Enhanced MR Imaging for Early Postoperative Recurrence Prediction. Cancer Imag (2019) 19:22. doi: 10.1186/s40644-019-0209-5
130. Hui T, Chuah TK, Low HM, Tan CH. Predicting Early Recurrence of Hepatocellular Carcinoma With Texture Analysis of Preoperative MRI: A Radiomics Study. Clin Radiol (2018) 73:1056.e11–1056.e16. doi: 10.1016/j.crad.2018.07.109
131. Hu C, Song Y, Zhang J, Dai L, Tang C, Li M, et al. Preoperative Gadoxetic Acid-Enhanced MRI Based Nomogram Improves Prediction of Early HCC Recurrence After Ablation Therapy. Front Oncol (2021) 11:649682. doi: 10.3389/fonc.2021.649682
132. Zhao Y, Wu J, Zhang Q, Hua Z, Qi W, Wang N, et al. Radiomics Analysis Based on Multiparametric MRI for Predicting Early Recurrence in Hepatocellular Carcinoma After Partial Hepatectomy. J Magn Reson Imag (2021) 53:1066–79. doi: 10.1002/jmri.27424
133. Zhang J, Liu X, Zhang H, He X, Liu Y, Zhou J, et al. Texture Analysis Based on Preoperative Magnetic Resonance Imaging (MRI) and Conventional MRI Features for Predicting the Early Recurrence of Single Hepatocellular Carcinoma After Hepatectomy. Acad Radiol (2019) 26:1164–73. doi: 10.1016/j.acra.2018.10.011
134. Ahn SJ, Kim JH, Park SJ, Kim ST, Han JK. Hepatocellular Carcinoma: Preoperative Gadoxetic Acid-Enhanced MR Imaging Can Predict Early Recurrence After Curative Resection Using Image Features and Texture Analysis. Abdom Radiol (NY) (2019) 44:539–48. doi: 10.1007/s00261-018-1768-9
135. Kim S, Shin J, Kim DY, Choi GH, Kim MJ, Choi JY. Radiomics on Gadoxetic Acid-Enhanced Magnetic Resonance Imaging for Prediction of Postoperative Early and Late Recurrence of Single Hepatocellular Carcinoma. Clin Cancer Res (2019) 25:3847–55. doi: 10.1158/1078-0432.CCR-18-2861
136. Zhang Z, Chen J, Jiang H, Wei Y, Zhang X, Cao L, et al. Gadoxetic Acid-Enhanced MRI Radiomics Signature: Prediction of Clinical Outcome in Hepatocellular Carcinoma After Surgical Resection. Ann Transl Med (2020) 8:870. doi: 10.21037/atm-20-3041
137. Zhang J, Wang X, Zhang L, Yao L, Xue X, Zhang S, et al. Radiomics Predict Postoperative Survival of Patients With Primary Liver Cancer With Different Pathological Types. Ann Transl Med (2020) 8:820. doi: 10.21037/atm-19-4668
138. Wang XH, Long LH, Cui Y, Jia AY, Zhu XG, Wang HZ, et al. MRI-Based Radiomics Model for Preoperative Prediction of 5-Year Survival in Patients With Hepatocellular Carcinoma. Br J Cancer (2020) 122:978–85. doi: 10.1038/s41416-019-0706-0
139. Bargellini I, Sacco R, Bozzi E, Bertini M, Ginanni B, Romano A, et al. Transarterial Chemoembolization in Very Early and Early-Stage Hepatocellular Carcinoma Patients Excluded From Curative Treatment: A Prospective Cohort Study. Eur J Radiol (2012) 81:1173–8. doi: 10.1016/j.ejrad.2011.03.046
140. Song YG, Shin SW, Cho SK, Choi D, Rhim H, Lee MW, et al. Transarterial Chemoembolization as First-Line Therapy for Hepatocellular Carcinomas Infeasible for Ultrasound-Guided Radiofrequency Ablation: A Retrospective Cohort Study of 116 Patients. Acta Radiol (2015) 56:70–7. doi: 10.1177/0284185114520857
141. Terzi E, Golfieri R, Piscaglia F, Galassi M, Dazzi A, Leoni S, et al. Response Rate and Clinical Outcome of HCC After First and Repeated cTACE Performed "on Demand". J Hepatol (2012) 57:1258–67. doi: 10.1016/j.jhep.2012.07.025
142. Sun Y, Bai H, Xia W, Wang D, Zhou B, Zhao X, et al. Predicting the Outcome of Transcatheter Arterial Embolization Therapy for Unresectable Hepatocellular Carcinoma Based on Radiomics of Preoperative Multiparameter MRI. J Magn Reson Imag (2020) 52:1083–90. doi: 10.1002/jmri.27143
143. Song W, Yu X, Guo D, Liu H, Tang Z, Liu X, et al. MRI-Based Radiomics: Associations With the Recurrence-Free Survival of Patients With Hepatocellular Carcinoma Treated With Conventional Transcatheter Arterial Chemoembolization. J Magn Reson Imag (2020) 52:461–73. doi: 10.1002/jmri.26977
144. Yu JY, Zhang HP, Tang ZY, Zhou J, He XJ, Liu YY, et al. Value of Texture Analysis Based on Enhanced MRI for Predicting an Early Therapeutic Response to Transcatheter Arterial Chemoembolisation Combined With High-Intensity Focused Ultrasound Treatment in Hepatocellular Carcinoma. Clin Radiol (2018) 73:758.e9–18. doi: 10.1016/j.crad.2018.04.013
145. Weng W, Lü XL, Zhang QQ, Zhao XM, Chen CM, Kong CL, et al. Prediction of Short-Term Prognosis of Hepatocellular Carcinoma After TACE Surgery Based on MRI Texture Analysis Technology. Zhonghua Yi Xue Za Zhi (2020) 100:828–32. doi: 10.3760/cma.j.cn112137-20190705-01502
146. Kong C, Zhao Z, Chen W, Lv X, Shu G, Ye M, et al. Prediction of Tumor Response via a Pretreatment MRI Radiomics-Based Nomogram in HCC Treated With TACE. Eur Radiol (2021). doi: 10.1007/s00330-021-07910-0
147. Abajian A, Murali N, Savic LJ, Laage-Gaupp FM, Nezami N, Duncan JS, et al. Predicting Treatment Response to Intra-Arterial Therapies for Hepatocellular Carcinoma With the Use of Supervised Machine Learning-An Artificial Intelligence Concept. J Vasc Interv Radiol (2018) 29:850–7.e1. doi: 10.1016/j.jvir.2018.01.769
148. Liu J, Pei Y, Zhang Y, Wu Y, Liu F, Gu S. Predicting the Prognosis of Hepatocellular Carcinoma With the Treatment of Transcatheter Arterial Chemoembolization Combined With Microwave Ablation Using Pretreatment MR Imaging Texture Features. Abdom Radiol (NY) (2021) 46:3748–57. doi: 10.1007/s00261-020-02891-y
149. Llovet JM, Ricci S, Mazzaferro V, Hilgard P, Gane E, Blanc JF, et al. Sorafenib in Advanced Hepatocellular Carcinoma. N Engl J Med (2008) 359:378–90. doi: 10.1056/NEJMoa0708857
150. Kudo M, Finn RS, Qin S, Han KH, Ikeda K, Piscaglia F, et al. Lenvatinib Versus Sorafenib in First-Line Treatment of Patients With Unresectable Hepatocellular Carcinoma: A Randomised Phase 3 Non-Inferiority Trial. Lancet (2018) 391:1163–73. doi: 10.1016/S0140-6736(18)30207-1
151. Bouattour M, Mehta N, He AR, Cohen EI, Nault JC. Systemic Treatment for Advanced Hepatocellular Carcinoma. Liver Cancer (2019) 8:341–58. doi: 10.1159/000496439
152. Brown ZJ, Greten TF, Heinrich B. Adjuvant Treatment of Hepatocellular Carcinoma: Prospect of Immunotherapy. Hepatology (2019) 70:1437–42. doi: 10.1002/hep.30633
153. Rimassa L, Pressiani T, Merle P. Systemic Treatment Options in Hepatocellular Carcinoma. Liver Cancer (2019) 8:427–46. doi: 10.1159/000499765
154. Finn RS, Qin S, Ikeda M, Galle PR, Ducreux M, Kim TY, et al. Atezolizumab Plus Bevacizumab in Unresectable Hepatocellular Carcinoma. N Engl J Med (2020) 382:1894–905. doi: 10.1056/NEJMoa1915745
155. Finn RS, Zhu AX. Evolution of Systemic Therapy for Hepatocellular Carcinoma. Hepatology (2020) 73:150–7. doi: 10.1002/hep.31306
156. Kelley RK. Atezolizumab Plus Bevacizumab - A Landmark in Liver Cancer. N Engl J Med (2020) 382:1953–5. doi: 10.1056/NEJMe2004851
157. Lee MS, Ryoo BY, Hsu CH, Numata K, Stein S, Verret W, et al. Atezolizumab With or Without Bevacizumab in Unresectable Hepatocellular Carcinoma (GO30140): An Open-Label, Multicentre, Phase 1b Study. Lancet Oncol (2020) 21:808–20. doi: 10.1016/S1470-2045(20)30156-X
158. Liu Z, Lin Y, Zhang J, Zhang Y, Li Y, Liu Z, et al. Molecular Targeted and Immune Checkpoint Therapy for Advanced Hepatocellular Carcinoma. J Exp Clin Cancer Res (2019) 38:447. doi: 10.1186/s13046-019-1412-8
159. Zhao YL, Guo QQ, Yang GR, Wang QD. Early Changes in Apparent Diffusion Coefficient as an Indicator of Response to Sorafenib in Hepatocellular Carcinoma. J Zhejiang Univ Sci B (2014) 15:713–9. doi: 10.1631/jzus.B1400010
160. Lee Y, Lee SS, Cheong H, Lee CK, Kim N, Son WC, et al. Intravoxel Incoherent Motion MRI for Monitoring the Therapeutic Response of Hepatocellular Carcinoma to Sorafenib Treatment in Mouse Xenograft Tumor Models. Acta Radiol (2017) 58:1045–53. doi: 10.1177/0284185116683576
161. Nakamura Y, Kawaoka T, Higaki T, Fukumoto W, Honda Y, Iida M, et al. Hepatocellular Carcinoma Treated With Sorafenib: Arterial Tumor Perfusion in Dynamic Contrast-Enhanced CT as Early Imaging Biomarkers for Survival. Eur J Radiol (2018) 98:41–9. doi: 10.1016/j.ejrad.2017.10.017
162. Kim B, Kim SS, Cho SW, Cheong JY, Huh J, Kim JK, et al. Liver Stiffness in Magnetic Resonance Elastography Is Prognostic for Sorafenib-Treated Advanced Hepatocellular Carcinoma. Eur Radiol (2020) 31:2507–17. doi: 10.1007/s00330-020-07357-9
163. Qayyum A, Hwang KP, Stafford J, Verma A, Maru DM, Sandesh S. Immunotherapy Response Evaluation With Magnetic Resonance Elastography (MRE) in Advanced HCC. J Immunother Cancer (2019) 7:329. doi: 10.1186/s40425-019-0766-y
164. Yang SH, Lin J, Lu F, Han ZH, Fu CX, Lv P, et al. Evaluation of Antiangiogenic and Antiproliferative Effects of Sorafenib by Sequential Histology and Intravoxel Incoherent Motion Diffusion-Weighted Imaging in An Orthotopic Hepatocellular Carcinoma Xenograft Model. J Magn Reson Imag (2017) 45:270–80. doi: 10.1002/jmri.25344
165. Shirota N, Saito K, Sugimoto K, Takara K, Moriyasu F, Tokuuye K. Intravoxel Incoherent Motion MRI as a Biomarker of Sorafenib Treatment for Advanced Hepatocellular Carcinoma: A Pilot Study. Cancer Imag (2016) 16:1. doi: 10.1186/s40644-016-0059-3
166. Hsu CY, Shen YC, Yu CW, Hsu C, Hu FC, Hsu CH, et al. Dynamic Contrast-Enhanced Magnetic Resonance Imaging Biomarkers Predict Survival and Response in Hepatocellular Carcinoma Patients Treated With Sorafenib and Metronomic Tegafur/Uracil. J Hepatol (2011) 55:858–65. doi: 10.1016/j.jhep.2011.01.032
167. Salvatore V, Baron Toaldo M, Marinelli S, Milazzo M, Palamà C, Venerandi L, et al. Early Prediction of Treatment Response to Sorafenib With Elastosonography in a Mice Xenograft Model of Hepatocellular Carcinoma: A Proof-of-Concept Study. Ultraschall Med (2013) 34:541–9. doi: 10.1055/s-0033-1355834
168. Jiang T, Kambadakone A, Kulkarni NM, Zhu AX, Sahani DV. Monitoring Response to Antiangiogenic Treatment and Predicting Outcomes in Advanced Hepatocellular Carcinoma Using Image Biomarkers, CT Perfusion, Tumor Density, and Tumor Size (RECIST). Invest Radiol (2012) 47:11–7. doi: 10.1097/RLI.0b013e3182199bb5
169. Jeon MY, Lee HW, Kim BK, Park JY, Kim DY, Ahn SH, et al. Reproducibility of European Association for the Study of the Liver Criteria and Modified Response Evaluation Criteria in Solid Tumors in Patients Treated With Sorafenib. Liver Int (2018) 38:1655–63. doi: 10.1111/liv.13731
170. Lencioni R, Montal R, Torres F, Park JW, Decaens T, Raoul JL, et al. Objective Response by mRECIST as a Predictor and Potential Surrogate End-Point of Overall Survival in Advanced HCC. J Hepatol (2017) 66:1166–72. doi: 10.1016/j.jhep.2017.01.012
171. Takada J, Hidaka H, Nakazawa T, Kondo M, Numata K, Tanaka K, et al. Modified Response Evaluation Criteria in Solid Tumors Is Superior to Response Evaluation Criteria in Solid Tumors for Assessment of Responses to Sorafenib in Patients With Advanced Hepatocellular Carcinoma. BMC Res Notes (2015) 8:609. doi: 10.1186/s13104-015-1565-2
172. Edeline J, Boucher E, Rolland Y, Vauléon E, Pracht M, Perrin C, et al. Comparison of Tumor Response by Response Evaluation Criteria in Solid Tumors (RECIST) and Modified RECIST in Patients Treated With Sorafenib for Hepatocellular Carcinoma. Cancer-Am Cancer Soc (2012) 118:147–56. doi: 10.1002/cncr.26255
173. Yuan G, Song Y, Li Q, Hu X, Zang M, Dai W, et al. Development and Validation of a Contrast-Enhanced CT-Based Radiomics Nomogram for Prediction of Therapeutic Efficacy of Anti-PD-1 Antibodies in Advanced HCC Patients. Front Immunol (2020) 11:613946. doi: 10.3389/fimmu.2020.613946
174. Mulé S, Thiefin G, Costentin C, Durot C, Rahmouni A, Luciani A, et al. Advanced Hepatocellular Carcinoma: Pretreatment Contrast-Enhanced CT Texture Parameters as Predictive Biomarkers of Survival in Patients Treated With Sorafenib. Radiology (2018) 288:445–55. doi: 10.1148/radiol.2018171320
175. Aerts HJ. The Potential of Radiomic-Based Phenotyping in Precision Medicine: A Review. JAMA Oncol (2016) 2:1636–42. doi: 10.1001/jamaoncol.2016.2631
176. Galldiks N, Kocher M, Ceccon G, Werner JM, Brunn A, Deckert M, et al. Imaging Challenges of Immunotherapy and Targeted Therapy in Patients With Brain Metastases: Response, Progression, and Pseudoprogression. Neuro Oncol (2020) 22:17–30. doi: 10.1093/neuonc/noz147
Keywords: hepatocellular carcinoma, magnetic resonance imaging, intravoxel incoherent motion, radiomics, immune checkpoint inhibitors, target therapies, therapeutic response, diagnosis
Citation: Gong X-Q, Tao Y-Y, Wu Y–K, Liu N, Yu X, Wang R, Zheng J, Liu N, Huang X-H, Li J-D, Yang G, Wei X-Q, Yang L and Zhang X-M (2021) Progress of MRI Radiomics in Hepatocellular Carcinoma. Front. Oncol. 11:698373. doi: 10.3389/fonc.2021.698373
Received: 21 April 2021; Accepted: 31 August 2021;
Published: 20 September 2021.
Edited by:
Omar Sultan Al-Kadi, The University of Jordan, JordanCopyright © 2021 Gong, Tao, Wu, Liu, Yu, Wang, Zheng, Liu, Huang, Li, Yang, Wei, Yang and Zhang. This is an open-access article distributed under the terms of the Creative Commons Attribution License (CC BY). The use, distribution or reproduction in other forums is permitted, provided the original author(s) and the copyright owner(s) are credited and that the original publication in this journal is cited, in accordance with accepted academic practice. No use, distribution or reproduction is permitted which does not comply with these terms.
*Correspondence: Lin Yang, linyangmd@163.com