- Department of Otolaryngology Head and Neck Surgery, Shengjing Hospital of China Medical University, Shenyang, China
Objective: Our purpose was to develop and verify an immune-related signature for predicting recurrence risk of patients with laryngeal cancer.
Methods: RNA-seq data of 51 recurrence and 81 non-recurrence laryngeal cancer samples were downloaded from TCGA database, as the training set. Microarray data of 34 recurrence and 75 non-recurrence cancer samples were obtained from GEO dataset, as the validation set. Single factor cox regression was utilized to screen prognosis-related immune genes. After LASSO regression analysis, an immune-related signature was constructed. Recurrence free survival (RFS) between high- and low- recurrence risk patients was presented, followed by ROC. We also evaluated the correlation between immune infiltration and the signature using the CIBERSORT algorithm. The genes in the signature were validated in laryngeal cancer tissues by western blot or RT-qPCR. After RCN1 knockdown, migration and invasion of laryngeal cancer cells were investigated.
Results: Totally, 43 prognosis-related immune genes were identified for laryngeal cancer. Among them, eight genes were used for constructing a prognostic signature. High risk group exhibited a higher recurrence risk than low risk group. The AUC for 1-year was separately 0.803 and 0.715 in the training and verification sets, suggesting its well efficacy for predicting the recurrence. Furthermore, this signature was closely related to distinct immune cell infiltration. RCN1, DNAJA2, LASP1 and IBSP were up-regulated in laryngeal cancer. RCN1 knockdown restrained migrated and invasive abilities of laryngeal cancer cells.
Conclusion: Our findings identify a reliable immune-related signature that can predict the recurrence risk of patients with laryngeal cancer.
Introduction
Laryngeal cancer is a commonly diagnosed head and neck malignancy globally (1). It is induced by various risk factors, such as smoking, drinking and human papillomavirus infection (1). At present, the main treatment strategies for laryngeal cancer are surgery, radiotherapy as well as chemotherapy (2). Under the goals of maintaining speech and swallowing functions, some patients have experienced relapse and require salvage treatment. Recurrence is the main manifestation of treatment failure for laryngeal cancer (3). The prognosis of patients with recurrent laryngeal cancer is very poor. Even if salvage whole laryngectomy or re-radiotherapy is taken, patients who relapse within 2 years after initial treatment may still die from recurrence (3). Some clinical indicators have been reported as prognostic predictors for patients with recurrent head and neck carcinoma such as presence or absence of complications as well as tumor cell differentiation (4). Thus, how to reduce mortality and risk of recurrence remains a major clinical challenge for head and neck surgeons (5). Hence, it is urgent to probe into novel detection indicators that can predict patients’ recurrence.
Tumor, node, metastasis (TNM) stage is the main clinical tool to predict the risk of recurrence (6). However, in clinical practice, its predictive power is limited. Patients in the same stage often have different clinical outcomes due to the heterogeneous clinical pathological characteristics and tumor biology (7). Studies have reported that the TNM staging system cannot accurately evaluate the prognosis of patients with laryngeal cancer (7). In other tumor types, it also shows that including more clinical prognostic factors for prognostic analysis can make the results more accurate and reliable. The immune system plays a vital role in controlling tumor growth and progression. Infiltrating immune cells are highly heterogeneous in laryngeal cancer (8). The abnormal interaction between tumor cells and immune cells in the tumor microenvironment may promote the occurrence of tumors. For example, high density of tumor infiltrating lymphocytes is associated with a better prognosis of laryngeal carcinoma (9). With the development of molecular biology, prognostic models have been developed to predict the prognosis of laryngeal cancer. For example, Zhang et al. constructed a 4-lncRNA prognostic signature for laryngeal cancer (10). Ana Gabriela Jover-Esplá et al. developed a scoring system for predicting laryngeal cancer patients’ 5-year mortality (11). Nevertheless, there is still a lack of reliable molecular models for predicting the recurrence of patients with laryngeal cancer. Thus, in this study, we developed a reliable model to predict the risk of recurrence for patients with laryngeal cancer.
Materials and Methods
Data Collection and Pre-Processing
The level 3 RNA-seq data and the corresponding clinical information of 132 cases of laryngeal cancer (including 51 recurrence samples and 81 non-recurrence samples) were obtained from The Cancer Genome Atlas (TCGA; https://cancergenome.nih.gov). Microarray expression profiling of 109 laryngeal cancer patients was download from the Gene Expression Omnibus (GEO; accession: GSE27020) (12) via the GEOquery package, which was then annotated by the corresponding package from the bioconductor. This dataset was based on the platform of GPL96 [HG-U133A] Affymetrix Human Genome U133A Array. Among them, 34 patients relapsed after surgery. Only patients diagnosed with laryngeal cancer were included in our study. Those without complete clinical data (recurrence endpoint and recurrence time) were excluded. Data from different platforms were normalized by the limma package (13). TCGA cohort was the training set and GEO cohort was the validation set. Table 1 lists the clinical characteristics of laryngeal cancer in the two cohorts.
Immune-Related Genes
The list of 2498 immune-related genes was extracted from the Immunology Database and Analysis Portal (ImmPort) database (https://immport.niaid.nih.gov) (14).
Development and Verification of an Immune-Related Prognostic Signature
Using univariate Cox regression analysis, the correlation between immune-related genes and prognosis was determined in the training set. Genes with p-value<0.001 were screened as prognostic factors. Then, least absolute shrinkage and selection operator (LASSO) regression analysis was presented to obtain the optimal candidate genes utilizing the glmnet package (15). In the training set, multivariate cox hazard regression modeling was constructed. The stepwise method based on AIC was utilized for further variable selection. The risk score was calculated on the basis of the coefficient and expression value of each candidate in the training and validation sets.
Evaluation of the Effectiveness of the Signature in Predicting Recurrence
Time‐dependent receiver operating characteristic (ROC) curve of 1-, 3-, and 5-year was built for the signature via the survivalROC package in the two datasets. The area under the curve (AUC) was calculated to evaluate its predictive effectiveness. The optimal cutoff value was determined, which was utilized to divide high- and low- risk groups. Kaplan‐Meier curves of overall survival (OS) and recurrence‐free survival (RFS) were conducted between the two groups via the survival package. By log‐rank test, the difference in OS and RFS was compared between the two groups. Univariate and multivariate cox regression analysis was also presented to evaluate the predictive independency of this signature in predicting laryngeal cancer outcome.
Immune Infiltration
The Cell type identification by estimating relative subsets of RNA transcripts (CIBERSORT) algorithm (http://cibersort.stanford.edu/) was utilized to evaluate the proportion of 22 kinds of immune cells in each laryngeal cancer samples from TCGA database (16). A total of 1,000 simulations were presented, and p < 0.05 was significant.
Construction of a Prognostic Nomogram
Genes in the prognostic model were incorporated into a nomogram for predicting 1-, 3- and 5-year survival probabilities using the rms package.
Drug Sensitivity Evaluation
The sensitivity to chemotherapy drugs was curated from the Genomics of Drug Sensitivity in Cancer (GDSC; https://www.cancerrxgene.org/) project [15]. The half maximal inhibitory concentration (IC50) was determined with ride regression analysis utilizing the pRRophetic package.
Patients and Specimens
Postoperative tissue specimens of 26 patients with laryngeal cancer from the Department of Otolaryngology Head and Neck Surgery of the Shengjing Hospital of China Medical University were selected between 2019 and 2020. At the same time, corresponding adjacent tissues (>2 cm from the tumor margin) were selected as controls. All the selected tissue specimens were diagnosed as laryngeal squamous cell carcinoma by the Department of Pathology. All patients provided written informed consent. This research obtained the approval by the Ethics Committee of Shengjing Hospital of China Medical University (2019030).
Western Blot
Tissues or cells were added with RIPA lysate to extract total cell protein. Protein concentration was determined by BCA method. The 30 μg protein sample was mixed with the loading buffer thoroughly. The protein was isolated by sodium dodecyl sulfate - polyacrylamide gel electrophoresis (SDS-PAGE). After the reaction, the membrane was transferred and sealed, and the primary protein antibody including RCN1 (1/1000; ab205927; Abcam, USA), DNAJA2 (1/10000; ab168365; Abcam, USA), LASP1 (1/10000; ab156872, USA), IBSP (1/1000; A-AP14114a; Abgent, USA), β-actin (1/5000; ab179467; Abcam, USA), LAT2 (1/1000; ab75610; Abcam, USA), FUZ (1/1000; ab111842; Abcam, USA), HOOK2 (1/1000; ab133691; Abcam, USA), and DAPK2 (1/1000; ab111928; Abcam, USA) was added and incubated at 4°C for 24 h. After TBST washing, IgG secondary antibody (1/5000; ab7090; Abcam, USA) was added. ECL was added, and the protein bands were analyzed by automatic gel imaging system.
Real-Time Quantitative Polymerase Chain Reaction (RT-qPCR)
Total RNA was extracted from laryngeal cancer and paracancerous tissues by Trizol method. The quality of RNA was measured by ultraviolet spectrophotometer, and cDNA was synthesized by reverse transcription kit. The primers of RCN1 (5’-AAACGGGTGCAGAAAAGATACA-3’ (forward) and 5’-AGGTAGTAACCATAGGTGGCTT-3’ (reverse)); DNAJA2 (5’-GTGGCTGACACGAAGCTGTA-3’ (forward) and 5’-AAGACCTTGCTCTCCGTATCT-3’ (reverse)); LASP1 (5’-TGCGGCAAGATCGTGTATCC-3’ (forward) and 5’-GCAGTAGGGCTTCTTCTCGTAG-3’ (reverse)); IBSP (5’-CACTGGAGCCAATGCAGAAGA-3’ (forward) and 5’-TGGTGGGGTTGTAGGTTCAAA-3’ (reverse)); LAT2 (5’-ACAGAGCTTTACGGGGTCC-3’ (forward) and 5’-TGGGGTCTATGTAGGCTTCCT-3’ (reverse)); FUZ (5’-GACTTGAGGGCCAGTTATTGC-3’ (forward) and 5’-GACACCACCAGACTGACGA-3’ (reverse)); HOOK2 (5’- AAGCTGAGCTATGCGGGTC-3’ (forward) and 5’-AGGAGGGGTCTATCTGGTTCA-3’ (reverse)); DAPK2 (5’- CATCCTTGAGCTAGTGTCTGGA-3’ (forward) and 5’-GGATCTGCTTAATGAAGCTGGT-3’ (reverse)) and β-actin (5’-TGCTGTCCCTGTATGCCTC-3’ (forward) and 5’-TGATGTCACGCACGCAGATTT-3’ (reverse)) were synthesized by Shanghai Bioengineering Co., Ltd. (China). The reaction system was prepared according to the instructions of the qRT-PCR detection kit. The system was presented in an ABI 7500 RT-qPCR instrument. The reaction conditions included: pre-denaturation at 95°C for 5 min, denaturation at 95°C for 15 s, annealing at 60°C for 60 s, and extension at 72°C for 30 s, with a total of 40 cycles. β-actin served as an internal control of RCN1. By applying the 2-ΔΔCt method, RCN1 mRNA expression was quantified.
Cell Culture and Transfection
TU686 and TU212 cells (ATCC, USA) were cultured in DMEM medium (Shanghai Yubo Biotechnology Co., Ltd, China) containing 10% FBS and placed in an incubator with 5% CO2 at 37°C. The cells were sub-cultured when the growth density reached about 85%. The cells in logarithmic growth phase were inoculated in a 6-well plate and transfected when the cell fusion reached 70%. The operation was carried out according to the instructions of Lipofectamine2000 reagent (Invitrogen, USA). The culture medium was changed to the medium without FBS 1 h before transfection. The siRNA negative control (si-NC) or siRNA against RCN1 (siRCN1; sequence: 5’-GGAUGAGAAGCUAACUAAAGA-3’) was transfected into the cells. The medium containing FBS was replaced after 6 h transfection and continued for 48 h. The cells were collected for subsequent experiments.
Transwell
The upper chamber at the bottom of the transwell chamber was added with Matrigel gel (100 μL/well). Transfected TU686 and TU212 cells were collected and resuspended (2×105/mL). The resuspended cells were added to the upper chamber of the transwell chamber (200 μl/well). 600 μl DMEM medium containing FBS was added to the lower chamber of transwell. The cells were placed in an incubator with 5% CO2 at 37°C for 24 h. The cells were fixed with paraformaldehyde for 20 min. Then, the cells were washed with PBS. They were stained with crystal violet solution for 15 min. After washing with PBS, the number of invaded cells was observed under the microscope.
Wound Healing
The transfected cells were collected and counted after trypsin digestion. Then, the cells were seeded into 6-well plates (1×104/mL). 24 h after inoculation, the 6-well plate was scratched, and washed with PBS for 3 times. The serum-free medium was replaced. The migration of cells was observed at 0 h and 24 h after scratches.
Statistical Analyses
All data were analyzed using R language (version: 3.6.1; http://www.r-project.org/). Comparisons between groups were presented with student’s t test or Wilcoxon test. Pearson or Spearmon correlation test was presented for evaluating the association between variables. Statistical significance was set as p < 0.05.
Results
Patient Characteristics
RNA-seq data of 51 recurrence laryngeal cancer patients and 81 non-recurrence patients were from TCGA database, as the training set (Table 1). Microarray expression profiles of 34 recurrence laryngeal cancer patients and 75 non-recurrence patients were selected as the validation set. In the training set, 62 patients were aged ≤60. For grade, patients were diagnosed at 1 (n = 6), 2 (n = 18), 3 (n = 30), 4a (n = 74) and 4b (n = 4). In the validation set, 43 patients were aged ≤60. For grade, all patients were diagnosed at 1 (n = 44), 2 (n = 49) and 3 (n = 16).
Development of an Immune Signature for Predicting Laryngeal Cancer Recurrence
Totally, 2498 immune genes were extracted from the IMMPORT database. In the training set, prognosis-related genes with p-value <0.001 were screened by single factor cox regression. A total of 43 immune genes were identified as the most significant factors affecting the prognosis. When the number of variables was 13, the partial likelihood deviation was the smallest (Figure 1A). The regression coefficients of the 13 variables were calculated in the LASSO model (Figure 1B). Multivariate cox hazard regression analysis was then carried out in the training set, and the stepwise method based on AIC was used for further variable selection. Finally, an eight immune-related signature was conducted, composed of RCN1, LAT2, DAPK2, DNAJA2, FUZ, LASP1, IBSP and HOOK2 (Table 2). Among them, RCN1 (hazard ratio (HR): 1.928852, 95% confidence interval (CI): 1.194018-3.115926, p-value = 0.007261) and IBSP (HR: 1.928852, 95% CI: 1.194018-3.115926, p-value = 0.007261) were risk factors for laryngeal cancer. Moreover, LAT2 (HR: 0.525901, 95% CI: 0.320208-0.863728, p-value = 0.011127) and HOOK2 (HR: 0.458976, 95% CI: 0.25448-0.827801, p-value = 0.009654) were protective factors for laryngeal cancer. The risk score of each sample was calculated, as follows: risk score = 0.656925 * the expression level of RCN1 + (-0.64264) * the expression level of LAT2 + (-0.26934) * the expression level of DAPK2 + 0.380614 * the expression level of DNAJA2 + (-0.28219) * the expression level of FUZ + (0.709185) * the expression level of LASP1 + 0.412168 * the expression level of IBSP + (-0.77876) * the expression level of HOOK2.
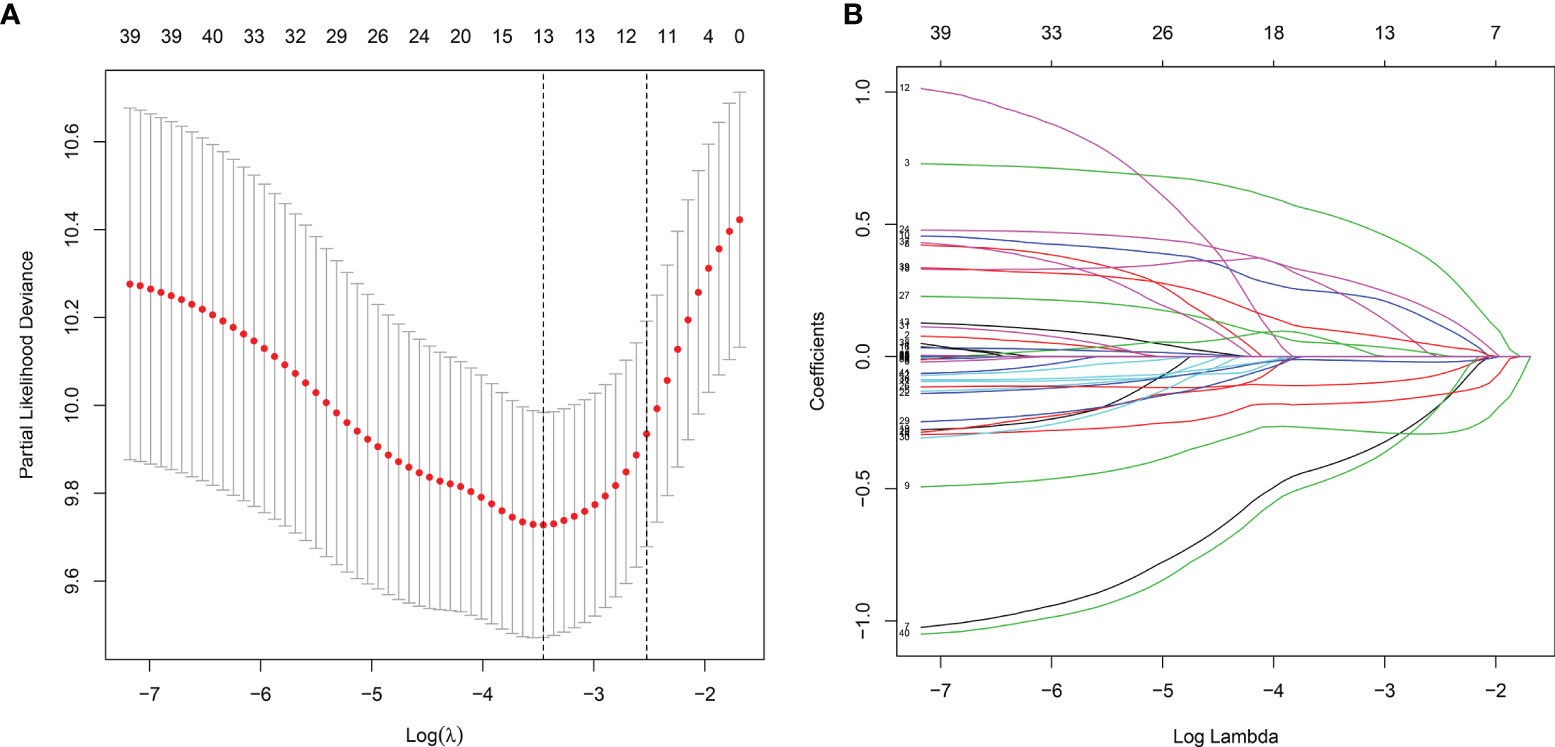
Figure 1 The LASSO coefficient profiles of immune-related genes. The optimal number of variables was determined using LASSO regression analysis. (A) 10-fold cross verification for selecting lambda in the LASSO model on the basis of the minimum criteria. (B) The optimal number of variables was determined using LASSO regression analysis.
Evaluation of the Predictive Efficacy of the Signature for Laryngeal Cancer Recurrence
Heat maps visualized the differences in expression patterns of these eight genes between high-and low- risk score groups in the training set (Figure 2A). We determined the optimal cutoff value to divide the high and low risk groups, and the optimal cutoff was 1.681355 (Figure 2B). As the risk score of patients with laryngeal cancer increased, the number of red dots gradually increased, indicating an increase in the number of patients with recurrence (Figure 2C). Thus, the high-risk population had a higher recurrence rate. Kaplan-Meier RFS results demonstrated that patients with high risk score indicated a higher recurrence risk than those with low risk score (p=8.059-08; Figure 2D). The ROC curve of the model was drawn to evaluate its sensitivity and specificity. In Figure 2E, the AUC of the constructed immune model predicting the patients’ 1-year recurrence rate was 0.803. Additionally, the AUC values at 3- and 5-year recurrence were separately 0.870 and 0.786 (Figure 2F). This indicated that the model exhibited the well efficacy for the prediction of recurrence. We also conducted the ROC curves at 1-, 3- and 5-year OS outcome and our results showed that the AUC values at 1, 3- and 5-year were separately 0.681, 0.822 and 0.750 (Figure 2G), demonstrating that this signature could also predict patients’ OS outcome.
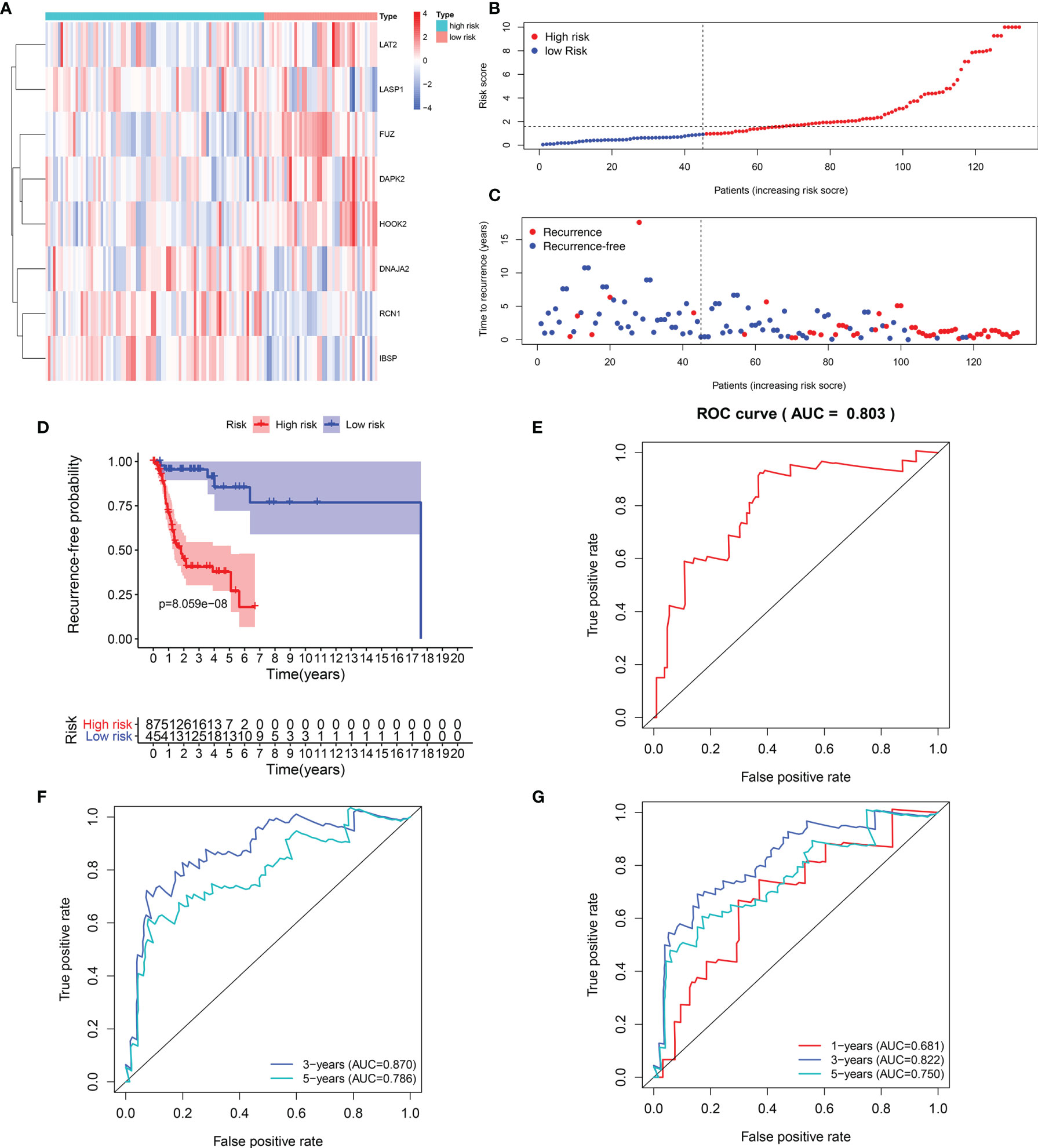
Figure 2 Evaluation of the predictive efficacy of the signature for laryngeal cancer recurrence in the training set. (A) Heat maps depicting the expression patterns of these eight genes including RCN1, LAT2, DAPK2, DNAJA2, FUZ, LASP1, IBSP and HOOK2 between high- and low- risk score group. Red: up-regulation and blue: down-regulation. (B) Ranking of risk scores among all laryngeal cancer patients. (C) Distribution of recurrence time among all laryngeal cancer patients. Red dots express recurrence laryngeal cancer samples and blue dots represent non-recurrence samples. The dotted line indicates the optimal cutoff value of risk score. The left side of the line represents low-risk patients, while the right side represents high-risk patients. (D) Recurrence-free survival between high- and low- risk score groups. (E) ROC curve for predicting the patients’ 1-year recurrence. (F) ROC curves for predicting the patients’ 3 and 5-year recurrence. (G) ROC curves for prediction of 1, 3- and 5-year OS outcome.
Validation of the Predictive Efficacy of the Signature for Predicting Recurrence of Laryngeal Cancer
The efficacy of predicting recurrence of the signature was verified in the validation set. As depicted in the heat maps, we visualized the expression patterns of these eight genes between high-and low- risk score groups in the validation set (Figure 3A). There were distinct differences in their expression between the two groups. Following determination of the optimal cutoff value, 109 laryngeal cancer samples were separated into high- and low- risk score groups (Figure 3B). As the risk score of patients with laryngeal cancer increased, the number of samples with recurrence gradually increased (Figure 3C). Therefore, high-risk score patients exhibited a distinctly higher recurrence risk. Our Kaplan-Meier RFS results suggested that patients with high risk score usually experienced a higher recurrence risk in comparison to those with low risk score (p=8.657-06; Figure 3D). The AUC values of the ROC curve for patients’ 1- (Figure 3E), 3- and 5-year recurrence (Figure 3F) were 0.715, 0.701 and 0.701, indicating that the signature had the well efficacy for predicting recurrence risk of laryngeal cancer. Additionally, our uni- and multivariate cox regression analysis results demonstrated that the prognostic model was a clinically independent prognostic factor (Table 3).
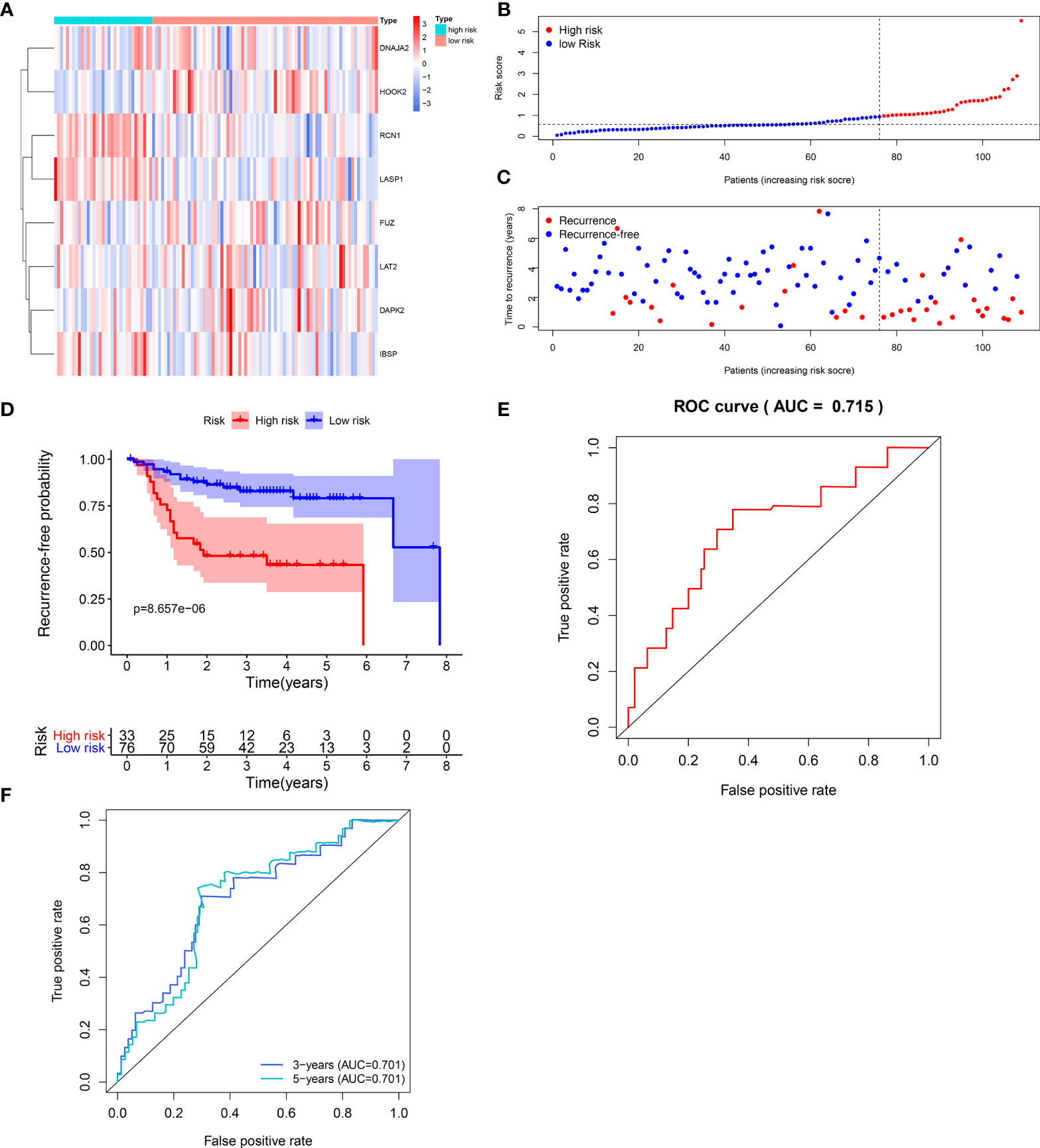
Figure 3 Validation of the predictive efficacy of the signature for predicting recurrence of laryngeal cancer in the verification set. (A) Heat maps visualizing the differences expression patterns of these eight genes including RCN1, LAT2, DAPK2, DNAJA2, FUZ, LASP1, IBSP and HOOK2 between high- and low- risk score group. Red expresses up-regulation and blue indicates down-regulation. (B) Ranking of risk scores among all laryngeal cancer patients. (C) Distribution of recurrence time among all laryngeal cancer patients. Red dots express recurrence laryngeal cancer samples and blue dots represent non-recurrence samples. The dotted line indicates the optimal cutoff value of risk score. The left side of the line represents low-risk patients, while the right side represents high-risk patients. (D) Recurrence-free survival between high- and low- risk score groups. (E) ROC curve for predicting the patients’ 1-year recurrence. (F) ROC curves for predicting the patients’ 3 and 5-year recurrence.
Characteristics of Immune Infiltration of the Signature
The levels of immune infiltration of 22 kinds of immune cells were assessed between high recurrence risk and low recurrence risk groups using the CIBERSORT algorithm (Figure 4A). In Figure 4B, we investigated that the signature was positively correlated to NK cells resting, macrophages M0 and T cells DC4 memory resting but was negatively correlated to NK cells activated, dendritic cells resting, plasma cells, Tregs, T cells CD8 and T cells follicular helper. There were higher infiltration levels of T cells CD4 memory resting (p=4.8e-09; Figure 5A), NK cells (p=7e-08; Figure 5B), macrophage M0 (p=1e-06; Figure 5C) in samples with high recurrence risk in comparison to those with low recurrence risk. Moreover, samples with high recurrence risk exhibited lower infiltration levels of T cells follicular helper (p=0.028; Figure 5D), T cells regulatory (Tregs; p=3.8e-09; Figure 5E), T cells CD8 (p=3.2e-11; Figure 5F), plasma cells (p=8.7e-07; Figure 5G), dendritic cells (p=1.1e-05; Figure 5H), NK cells (p=0.0057; Figure 5I) and mast cells activated (p=2.4e-05; Figure 5J) compared to those with low risk.
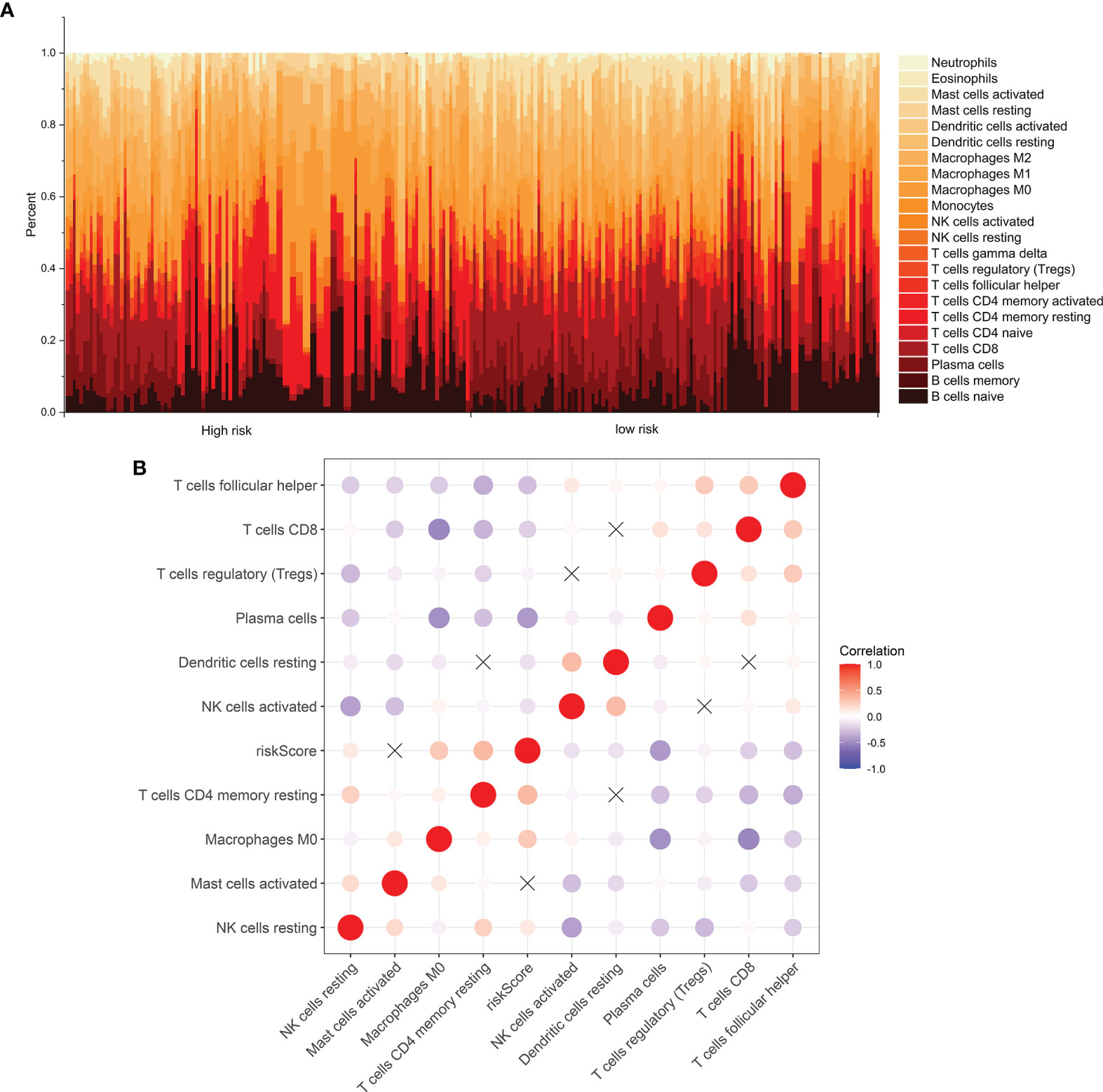
Figure 4 Immune cell infiltration analysis. (A) The distribution of 22 kinds of immune cells between high- and low- risk groups via the CIBERSORT algorithm. (B) Heatmap showing the interaction between immune cells and the risk score among laryngeal cancer patients.
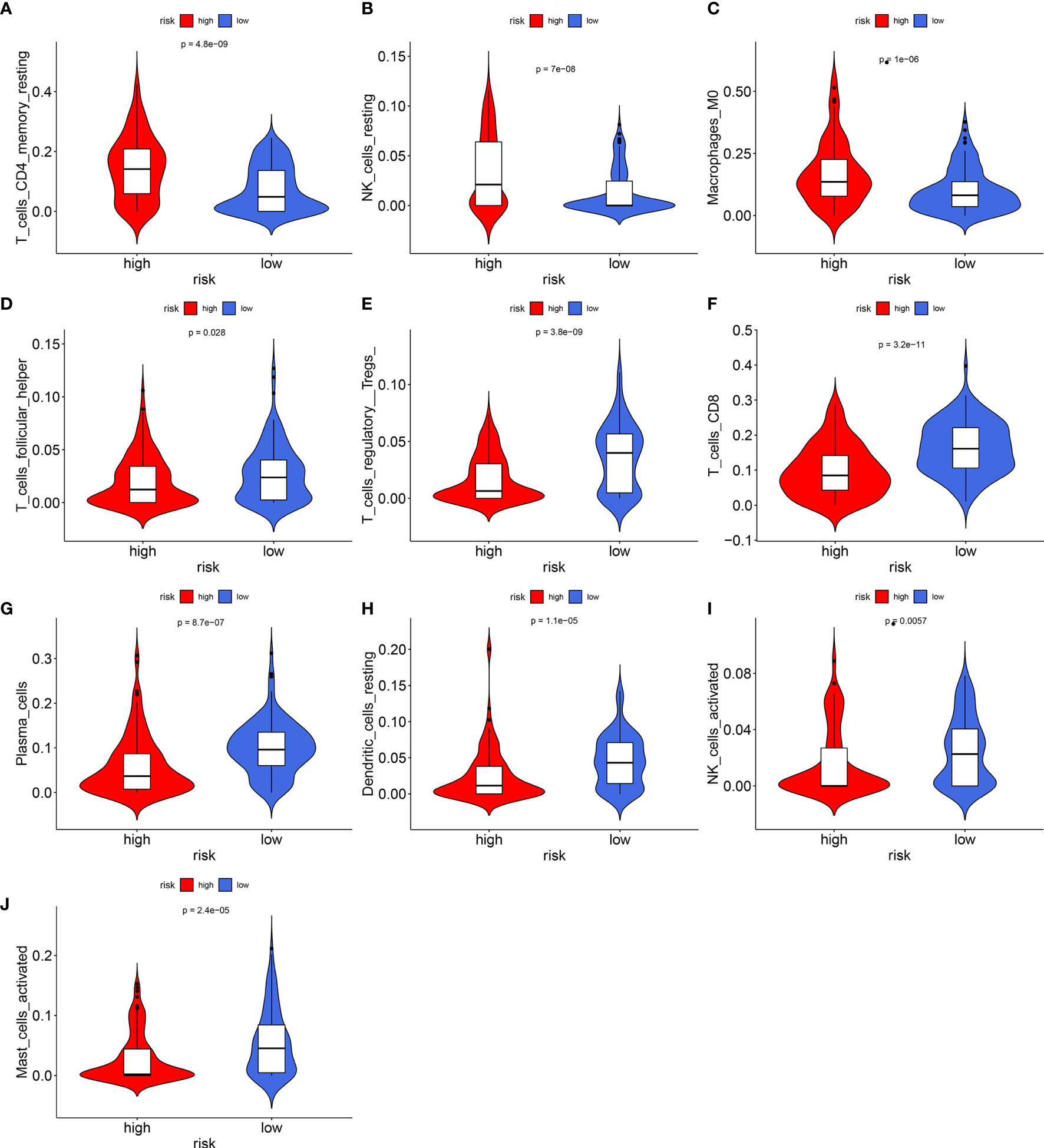
Figure 5 Violin plots showing the relationship between the signature and infiltration levels of 22 kinds of immune cells. (A) T cells CD4 memory resting; (B) NK cells; (C) macrophage M0; (D) T cells follicular helper; (E) T cells regulatory; (F) T cells; (G) plasma cells; (H) dendritic cells; (I) NK cells; (J) mast cells activated. Red indicates high recurrence risk group and blue indicates low recurrence risk group.
Evaluation of the Clinical Application Potential of the Prognostic Signature
ROC curves were conducted to compare the predictive performance of the prognostic signature with that of grade. In the training set, higher AUC values at 1-, 3- and 5-year recurrence were investigated in the prognostic model (Figure 6A) in compared with grade (Figure 6B). The similar results were found in the validation set (Figures 6C, D). This indicated that the prognostic signature possessed the better predictive performance than grade. For facilitating the clinical practice, we established a prognostic nomogram for predicting 1-, 3-, and 5-year survival probabilities based on the genes in the prognostic signature (Figure 6E).
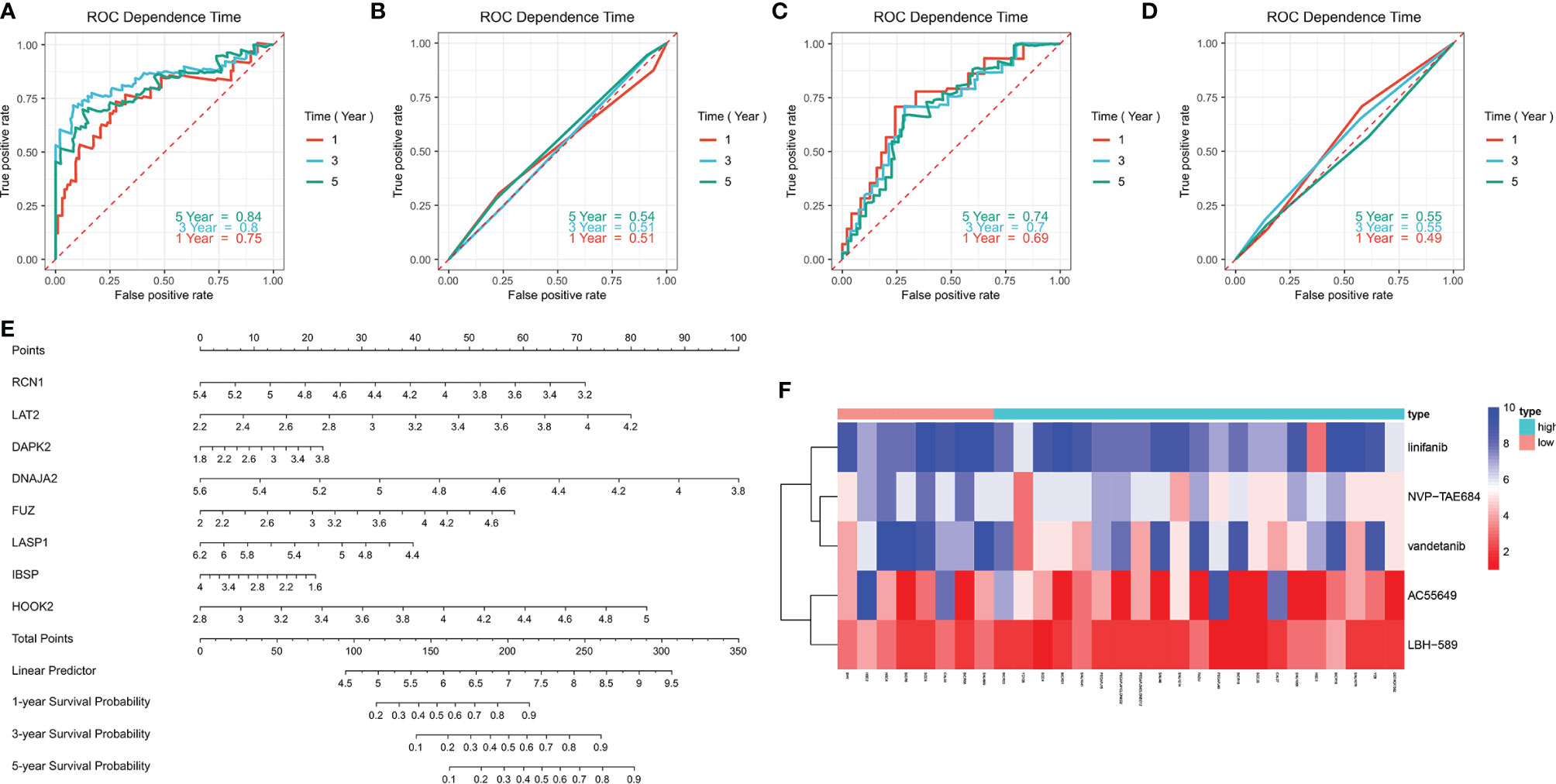
Figure 6 Evaluation of the clinical predictive efficacy of the signature and drug sensitivity. (A, B) Comparison of the predictive efficacy between (A) the signature and (B) grade in the training set in accordance with ROC curves at 1-, 3- and 5-year recurrence. (C, D) Comparison of the predictive efficacy between (C) the signature and (D) grade in the validation set based on ROC curves at 1-, 3- and 5-year recurrence. (E) Construction of a prognostic nomogram for predicting patients’ 1-, 3- and 5-year recurrence. (F) Heatmap showing the difference in drug sensitivity between high- and low-risk groups.
Comparison of the Effects for Patients in High- and Low Risk to Receive Chemotherapy
We also compared the effects for patients in high- and low risk to receive chemotherapy. Our results demonstrated that high-risk group presented significantly lower IC50 values of NVP-TAE684 (p=0.013111), vandetanib (p=0.015979), AC55649 (p=0.025122), LBH-589 (p=0.042172) and linifanib (p=0.045183) compared with low-risk group (Table 4; Figure 6F). This indicated that high-risk patients were more sensitive to above chemotherapeutic drugs.
Validation of the Genes in the Prognostic Signature
This study included 26 laryngeal cancer patients in our study. Table 5 shows the clinical features of above patients. The genes in the signature were detected in three paired laryngeal cancer and normal tissues by western blot (Figure 7A). As a result, RCN1 (p < 0.001; Figure 7B), DNAJA2 (p < 0.05; Figure 7C), LASP1 (p < 0.05; Figure 7D) and IBSP (p < 0.01; Figure 7E) exhibited higher expression while LAT2 (p < 0.01; Figure 7F), FUZ (p < 0.01; Figure 7G), and HOOK2 (p < 0.0001; Figure 7H) presented lower expression in laryngeal cancer than normal tissues. However, no significance difference in DAPK2 expression was found in laryngeal cancer and normal tissues (Figure 7I). We also detected the mRNA expression of above genes in 26 paired laryngeal cancer and normal tissues via RT-qPCR. As a result, RCN1 (p < 0.0001; Figure 7J), DNAJA2 (p < 0.001; Figure 7K), LASP1 (p < 0.001; Figure 7L) and IBSP (p < 0.0001; Figure 7M) were markedly up-regulated while LAT2 (p < 0.0001; Figure 7N) and FUZ (p < 0.0001; Figure 7O) were remarkably down-regulated in laryngeal cancer compared with normal tissues. Nevertheless, there was no significant difference in HOOK2 (Figure 7P) and DAPK2 (Figure 7Q) between laryngeal cancer and normal tissues.
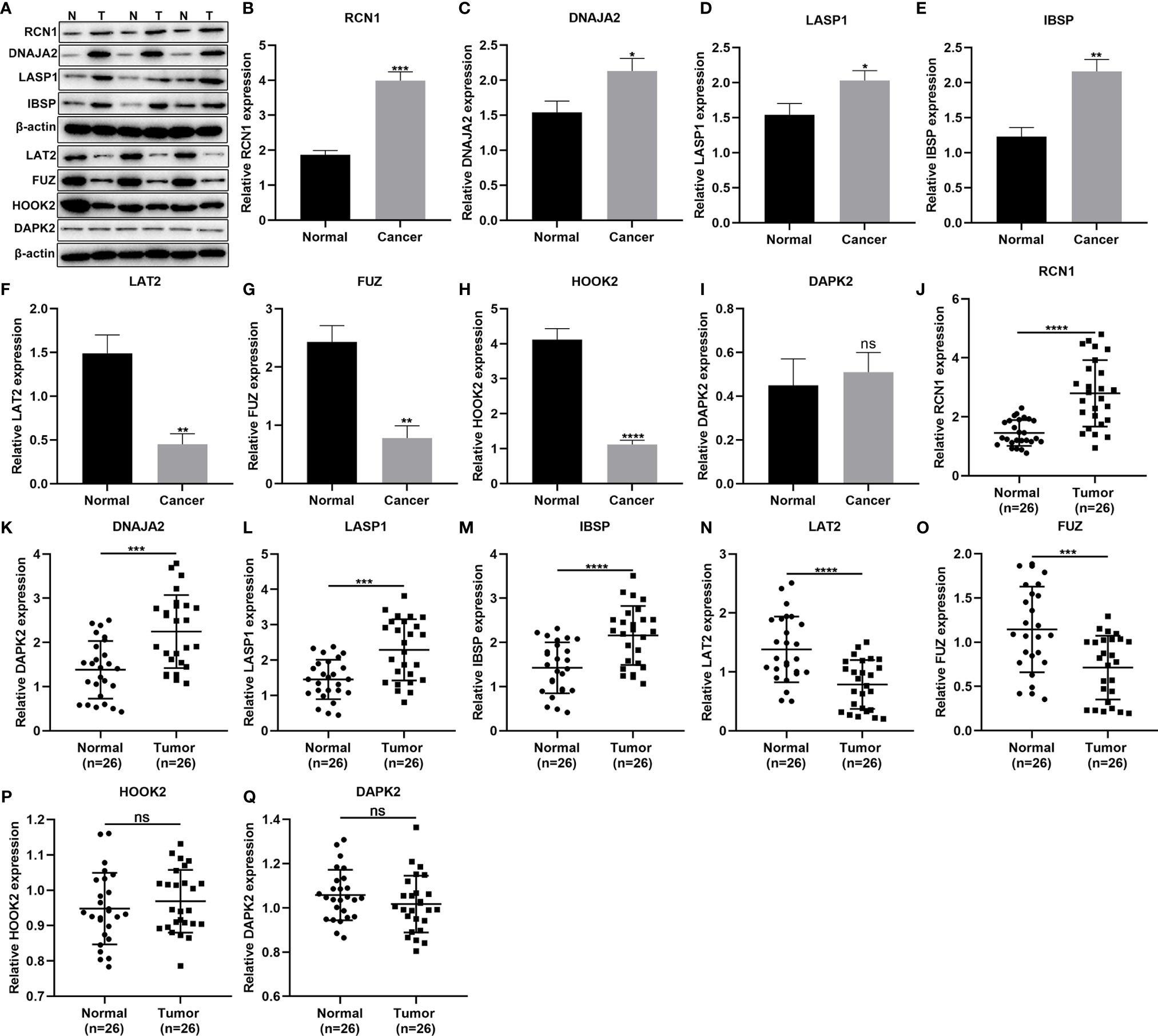
Figure 7 Validation of the genes in the prognostic signature. (A–I) Western blot for detection of the protein expression of (B) RCN1, (C) DNAJA2, (D) LASP1, (E) IBSP, (F) LAT2, (G) FUZ, (H) HOOK2, and (I) DAPK2 in laryngeal cancer and normal tissues. (J–Q) RT-qPCR for detecting the mRNA expression of (J) RCN1, (K) DNAJA2, (L) LASP1, (M) IBSP, (N) LAT2, (O) FUZ, (P) HOOK2, and (Q) DAPK2 in laryngeal cancer and normal tissues. Ns, not significant; *P < 0.05; **p < 0.01; ***p < 0.001; ****p < 0.0001.
Knockdown of RCN1 Lowers Invasion of Laryngeal Cancer Cells
To investigate the functions of RCN1 in laryngeal cancer progression, siRCN1 was transfected into TU686 and TU212 cells. Western blot confirmed that RCN1 expression was distinctly decreased in TU686 (p < 0.0001) and TU212 cells (p < 0.001; Figures 8A–C). After silencing RCN1, invasion of laryngeal cancer cells was assessed through transwell assay. Our data demonstrated that the invasive capacity of TU686 (p < 0.01) and TU212 (p < 0.01) cells was significantly restrained by siRCN1 transfection (Figures 8D–F).
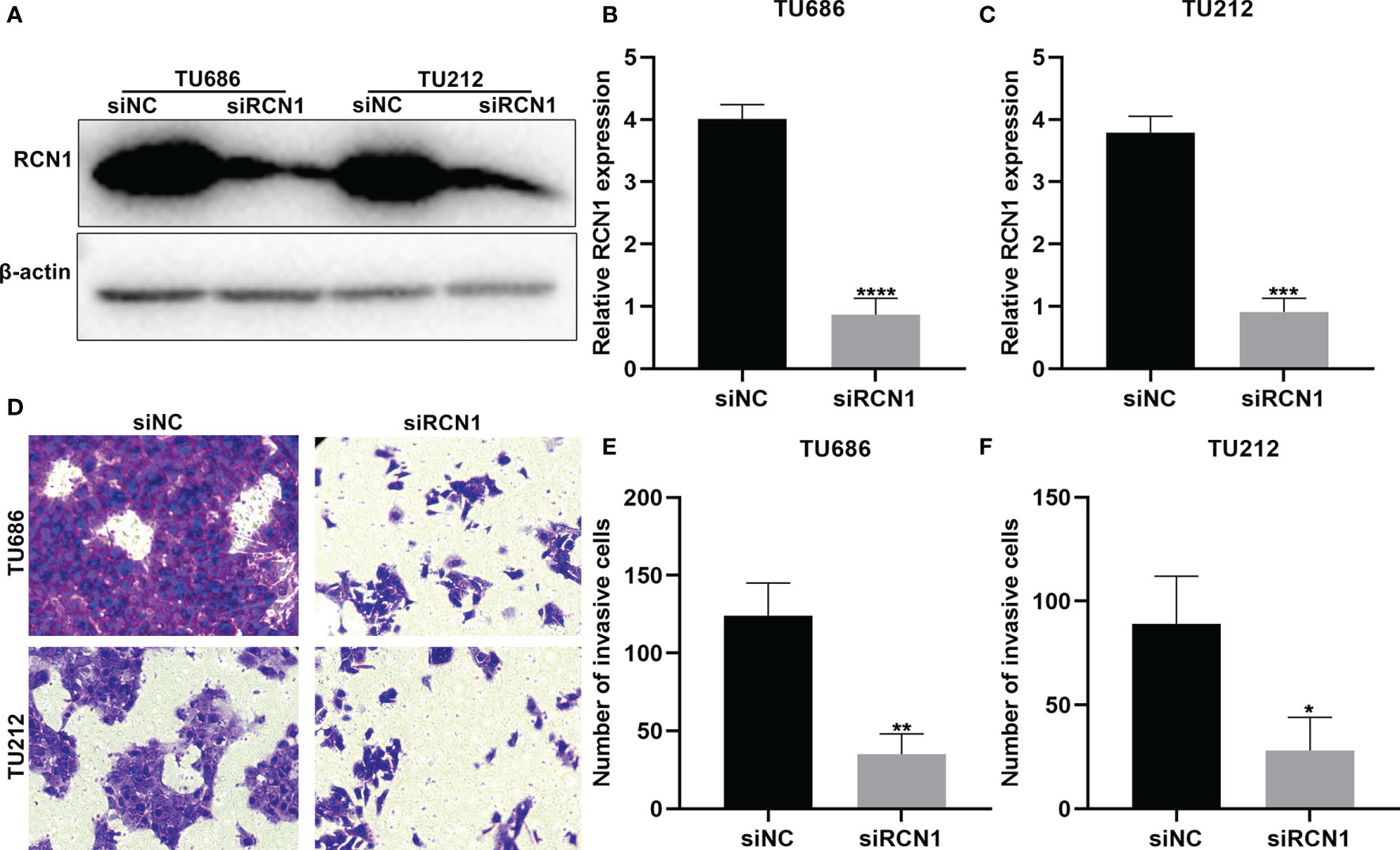
Figure 8 RCNA1 knockdown lowers invasion of laryngeal cancer cells. (A–C) Western blot for detection of RCNA1 expression in TU686 and TU212 cells transfected with siRCNA1. (D–F) Transwell for detection of the invasion of TU686 and TU212 cells transfected with siRCNA1. *P < 0.05; **p < 0.01; ***p < 0.001; ****p < 0.0001.
Knockdown of RCN1 Restrains Migration of Laryngeal Cancer Cells
The migrated ability of laryngeal cancer cells was evaluated by wound healing. As a result, wider wound distance was found in TU686 (p < 0.01) and TU212 (p < 0.05) cells under transfection with siRCN1 (Figures 9A–C). The data demonstrated that silencing RCN1 may restrain migration of laryngeal cancer cells.
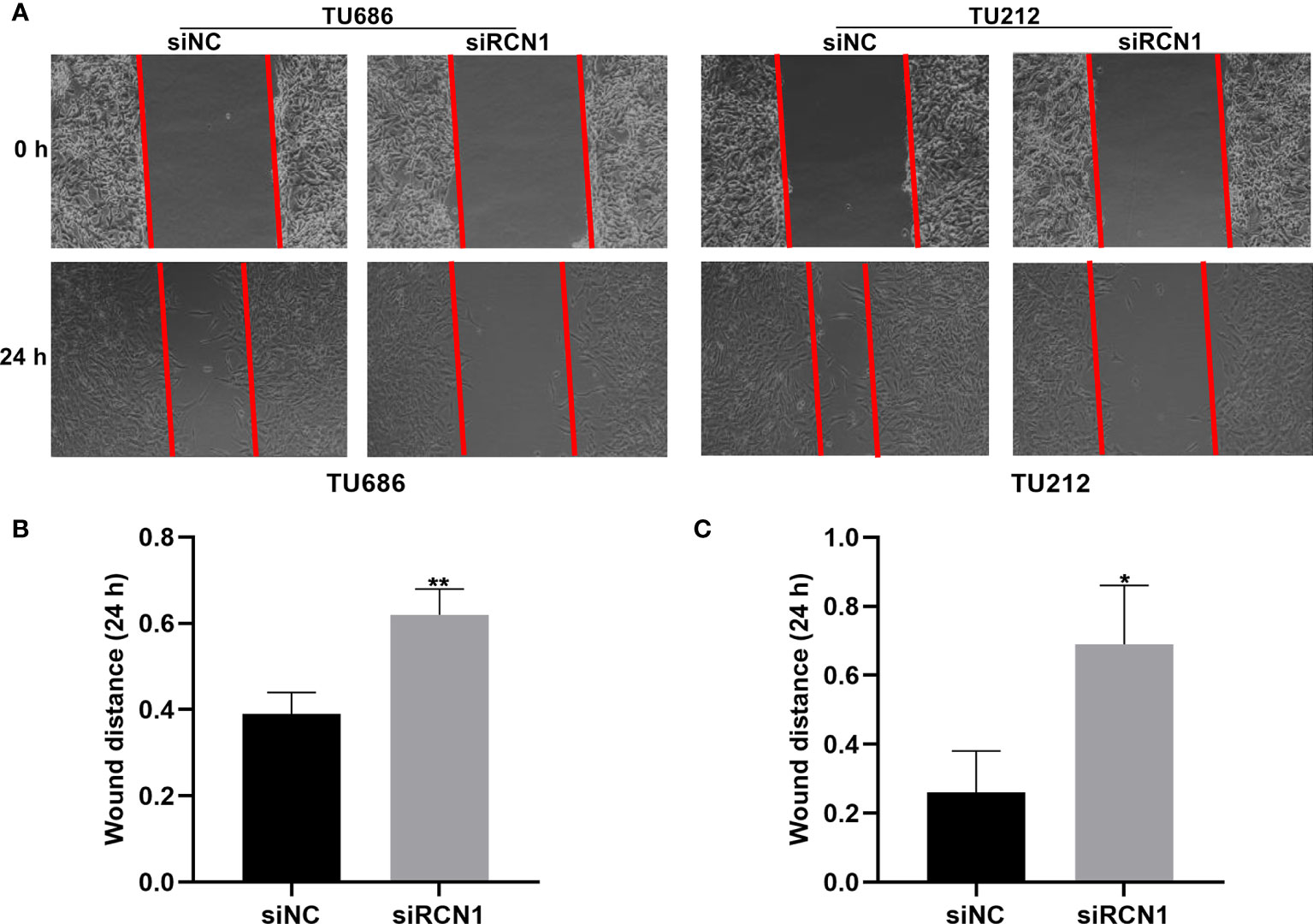
Figure 9 RCNA1 knockdown restrains migration of laryngeal cancer cells. (A) Representative images of wound healing assay. (B, C) Quantification of wound distance of TU686 and TU212 cells transfected with siRCNA1. *P < 0.05; **p < 0.01.
Discussion
Recurrence is the most common cause of death in postoperative patients with laryngeal cancer. There are no reliable clinical tools to identify patients who may benefit from adjuvant therapy (17). Our findings developed and verified an immune-related signature for prediction of laryngeal cancer recurrence. Patients with high risk score had higher risk of recurrence. Thus, these patients should take more radical treatments. Our ROC curve confirmed its well performance for predicting laryngeal cancer recurrence.
Increasing markers related to laryngeal cancer recurrence may be found in recent studies (18). Prognostic guidance has been implemented to determine whether organ is preserved. In patients with recurrent laryngeal cancer, clinical outcome is still not the optimal choice. Most of them may not be suitable for surgery. Due to the importance of immune infiltration in laryngeal cancer recurrence, the newly approved immunotherapy could bring distinct benefit to these patients (18). Patients with recurrence may be the main candidates for immune adjuvant therapy. How to identify this subgroup of patients still faces challenges. In this study, we developed an immune-related signature for predicting laryngeal cancer recurrence. This signature was composed of RCN1, LAT2, DAPK2, DNAJA2, FUZ, LASP1, IBSP and HOOK2. Our data confirmed that RCN1, DNAJA2, LASP1 and IBSP were up-regulated while LAT2, FUZ, HOOK2 and DAPK2 were down-regulated in laryngeal cancer. RCN1 knockdown restrained migrated and invasive abilities of laryngeal cancer cells. Dysregulated RCN1 is found in multiple kinds of cancers including non-small cell lung cancer (NSCLC) (19), prostate cancer (20), renal cell carcinoma (21), nasopharyngeal carcinoma (22), and oral squamous cell carcinoma (23). Its knockdown accelerates tumor cell apoptosis and necrosis (20). Nevertheless, RCN1 expression is still undiscovered in laryngeal cancer. Our results firstly demonstrated that RCN1 was a risk factor for laryngeal cancer. For patients in the high-risk recurrence group, its expression was higher than those in the low-risk recurrence group following validation. The roles of LAT2 have been widely studied in different cancers (24). For example, LAT2 as an oncogene could weaken the sensitivity of pancreatic cancer cells to gemcitabine (25). Consistent with other cancers, its expression is up-regulated in laryngeal cancer patients with high risk recurrence (26). Down-regulated DAPK1 has a relationship to tumor recurrence as well as metastasis (27). DAPK is frequently hypermethylated in laryngeal cancer tissues than controls (28). Hypermethylation of tumor suppressor genes induced by risk factors such as smoking and drinking contribute to progression of laryngeal cancer (29). Herein, we found that DAPK was often lowly expressed in laryngeal cancer patients with high risk recurrence, indicating that the loss of its expression might accelerate recurrence of laryngeal cancer. FUZ promotes the progression and metastasis of NSCLC (30). High FUZ expression implies poor prognosis for NSCLC patients. However, no study has explored the function of FUZ in laryngeal cancer. LASP1 is in relation to a variety of human malignancies (31). It is highly expressed in laryngeal cancer tissues, which promotes the invasion and proliferation of laryngeal cancer cells (32). In this study, patients with high recurrence risk had higher LASP1 expression than those with low risk. Combining previous studies, overexpressed LASP1 could be involved in recurrence of laryngeal cancer. The roles of IBSP in head and neck tumors have been studied. For instance, IBSP is highly expressed in esophageal squamous cell carcinoma (ESCC) tissues (33). Up-regulation of IBSP is positively correlated with lymph node metastasis, TNM stage as well as poor clinical outcomes for ESCC patients (33). Furthermore, it is associated with metastasis and recurrence of ESCC (33) and breast cancer (34). Our research demonstrated that it was highly expressed in laryngeal cancer tissues with high recurrence risk in comparison to low risk. Moreover, it was a risk factor for laryngeal cancer recurrence. HOOK2 is detected in serum of ESCC patients (35). Its low expression was found in laryngeal cancer with high recurrence risk. Also, it was a protective factor for laryngeal cancer recurrence. The molecular mechanisms involving these eight genes remain undiscovered in laryngeal cancer recurrence.
We assessed the immune infiltration levels of 22 kinds of immune cells between high- and low recurrence risk groups using the CIBERSORT algorithm. Laryngeal cancer samples with high recurrence risk exhibited higher infiltration levels of T cells CD4 memory resting, NK cells, macrophage M0 in comparison to those with low recurrence risk. Samples with high recurrence risk exhibited lower infiltration levels of T cells follicular helper, Tregs, T cells CD8, plasma cells, dendritic cells, NK cells and mast cells activated compared to those with low risk. Immune cells in the tumor microenvironment have been confirmed to be related to laryngeal cancer recurrence (18). For example, CD4 and CD8 tumor-infiltrating lymphocytes show the potential to predict the prognosis of patients with recurrent laryngeal cancer (36). Tumor-associated macrophage is an independent factor for prediction of the overall survival of patients with laryngeal cancer (37). Thus, interaction between this signature and immune cells could contribute to recurrence of laryngeal cancer.
The innovations of this research are as follows: (1) Recurrence remains a major obstacle to long-term survival of postoperative patients with laryngeal cancer. Nevertheless, limited genetic models can precisely predict recurrence probability and optimize therapeutic strategies for laryngeal cancer. The immune-related signature we proposed can provide accurate estimations of the recurrence prediction as well as might offer novel research directions and prospects for individualized treatment of patients with laryngeal cancer. (2) This study collected fresh clinical specimens to confirm the application of our model from biological algorithm approaches. (3) The biological function of one gene in the model was confirmed through in vitro experiments. Although our results are encouraging, several limitations should be pointed out. This study was based on the two public databases. The number of laryngeal cancer patients was relatively limited. Furthermore, this was a retrospective study. The immune-related recurrence signature should be verified in a prospective study.
Conclusion
Taken together, we developed and verified an immune-related signature for predicting the recurrence of laryngeal cancer. Patients with high risk score had a higher recurrence risk in comparison to those with low risk score. This signature could be correlated to immune infiltration. More clinical studies will be carried out to validate the performance of this signature for prediction of laryngeal cancer recurrence.
Data Availability Statement
The original contributions presented in the study are included in the article/supplementary material. Further inquiries can be directed to the corresponding author.
Ethics Statement
This research obtained the approval by the Ethics Committee of Shengjing Hospital of China Medical University (2019030). The patients/participants provided their written informed consent to participate in this study.
Author Contributions
WJ conceived and designed the study. HZ conducted most of the experiments and data analysis, and wrote the manuscript. XZ and JW participated in collecting data and helped to draft the manuscript. All authors reviewed and approved the manuscript.
Funding
This work was funded by National Natural Science Foundation of China (81202126, 81072196) and 345 Talent Project (M0710).
Conflict of Interest
The authors declare that the research was conducted in the absence of any commercial or financial relationships that could be construed as a potential conflict of interest.
Publisher’s Note
All claims expressed in this article are solely those of the authors and do not necessarily represent those of their affiliated organizations, or those of the publisher, the editors and the reviewers. Any product that may be evaluated in this article, or claim that may be made by its manufacturer, is not guaranteed or endorsed by the publisher.
Abbreviations
TCGA, The Cancer Genome Atlas; GEO, Gene Expression Omnibus; RFS, recurrence free survival; ROC, receiver operating characteristic; AUC, area under curve; TNM, tumor, node, metastasis; ImmPort, Immunology Database and Analysis Portal; LASSO, a least absolute shrinkage and selection operator; CIBERSORT, Cell type identification by estimating relative subsets of RNA transcripts; coef, coefficients; HR, hazard ratio; CI, confidence interval.
References
1. Zhou L, Li Y, Gao W, Huangfu H, Wen S, Zhang C, et al. Assessment of Tumor-Associated Immune Cells in Laryngeal Squamous Cell Carcinoma. J Cancer Res Clin Oncol (2019) 145(7):1761–72. doi: 10.1007/s00432-019-02936-w
2. Carvalho GB, Kohler HF, Lira RB, Vartanian JG, Kowalski LP. Survival Results of 3786 Patients With Stage I or II Laryngeal Squamous Cell Carcinoma: A Study Based on a Propensity Score. Braz J Otorhinolaryngol (2020) 19:S1808-8694(20)30106-3. doi: 10.1016/j.bjorl.2020.06.007
3. Succo G, Crosetti E. Limitations and Opportunities in Open Laryngeal Organ Preservation Surgery: Current Role of OPHLs. Front Oncol (2019) 9:408. doi: 10.3389/fonc.2019.00408
4. Nachalon Y, Reicher Y, Alkan U, Levi L, Bachar G, Popovtzer A. Prognostic Factors for Survival and Nonfunctional Larynx in Patients With Squamous Cell Carcinoma of the Larynx. Laryngoscope (2020) 130(5):1202–5. doi: 10.1002/lary.28173
5. Zhu X, Heng Y, Zhou L, Tao L, Zhang M. A Prognostic Nomogram for Predicting Risk of Recurrence in Laryngeal Squamous Cell Carcinoma Patients After Tumor Resection to Assist Decision Making for Postoperative Adjuvant Treatment. J Surg Oncol (2019) 120(4):698–706. doi: 10.1002/jso.25614
6. Cui J, Wang L, Tan G, Chen W, He G, Huang H, et al. Development and Validation of Nomograms to Accurately Predict Risk of Recurrence for Patients With Laryngeal Squamous Cell Carcinoma: Cohort Study. Int J Surg (2020) 76:163–70. doi: 10.1016/j.ijsu.2020.03.010
7. Locatello LG, Pietragalla M, Taverna C, Bonasera L, Massi D. Mannelli G. A Critical Reappraisal of Primary and Recurrent Advanced Laryngeal Cancer Staging. Ann Otol Rhinol Laryngol (2019) 128(1):36–43. doi: 10.1177/0003489418806915
8. Goswami KK, Barik S, Banerjee S, Bhowmick AK, Biswas J, Bose A, et al. Supraglottic Laryngeal Tumor Microenvironmental Factors Facilitate STAT3 Dependent Pro-Tumorigenic Switch in Tumor Associated Macrophages to Render Utmost Immune Evasion. Immunol Lett (2013) 156(1-2):7–17. doi: 10.1016/j.imlet.2013.09.003
9. Vassilakopoulou M, Avgeris M, Velcheti V, Kotoula V, Rampias T, Chatzopoulos K, et al. Evaluation of PD-L1 Expression and Associated Tumor-Infiltrating Lymphocytes in Laryngeal Squamous Cell Carcinoma. Clin Cancer Res (2016) 22(3):704–13. doi: 10.1158/1078-0432.Ccr-15-1543
10. Zhang G, Fan E, Zhong Q, Feng G, Shuai Y, Wu M, et al. Identification and Potential Mechanisms of a 4-lncRNA Signature That Predicts Prognosis in Patients With Laryngeal Cancer. Hum Genomics (2019) 13(1):36. doi: 10.1186/s40246-019-0230-6
11. Jover-Esplá AG, Palazón-Bru A, Folgado-de la Rosa DM, de Juan-Herrero J, Gil-Guillén VF. A Scoring System to Predict 5-Year Mortality in Patients Diagnosed With Laryngeal Glottic Cancer. Eur J Cancer Care (Engl) (2018) 27(4):e12860. doi: 10.1111/ecc.12860
12. Fountzilas E, Kotoula V, Angouridakis N, Karasmanis I, Wirtz RM, Eleftheraki AG, et al. Identification and Validation of a Multigene Predictor of Recurrence in Primary Laryngeal Cancer. PloS One (2013) 8(8):e70429. doi: 10.1371/journal.pone.0070429
13. Ritchie ME, Phipson B, Wu D, Hu Y, Law CW, Shi W, et al. Limma Powers Differential Expression Analyses for RNA-Sequencing and Microarray Studies. Nucleic Acids Res (2015) 43(7):e47. doi: 10.1093/nar/gkv007
14. Bhattacharya S, Andorf S, Gomes L, Dunn P, Schaefer H, Pontius J, et al. ImmPort: Disseminating Data to the Public for the Future of Immunology. Immunol Res (2014) 58(2-3):234–9. doi: 10.1007/s12026-014-8516-1
15. Friedman J, Hastie T, Tibshirani R. Regularization Paths for Generalized Linear Models via Coordinate Descent. J Stat Softw (2010) 33(1):1–22. doi: 10.18637/jss.v033.i01
16. Newman AM, Liu CL, Green MR, Gentles AJ, Feng W, Xu Y, et al. Robust Enumeration of Cell Subsets From Tissue Expression Profiles. Nat Methods (2015) 12(5):453–7. doi: 10.1038/nmeth.3337
17. Mann JE, Smith JD, Birkeland AC, Bellile E, Swiecicki P, Mierzwa M, et al. Analysis of Tumor-Infiltrating CD103 Resident Memory T-Cell Content in Recurrent Laryngeal Squamous Cell Carcinoma. Cancer Immunol Immunother (2019) 68(2):213–20. doi: 10.1007/s00262-018-2256-3
18. Dutsch-Wicherek M, Tomaszewska R, Lazar A, Wicherek L, Skladzien J. The Association Between RCAS1 Expression in Laryngeal and Pharyngeal Cancer and Its Healthy Stroma With Cancer Relapse. BMC Cancer (2009) 9:35. doi: 10.1186/1471-2407-9-35
19. Chen X, Shao W, Huang H, Feng X, Yao S, Ke H. Overexpression of RCN1 Correlates With Poor Prognosis and Progression in Non-Small Cell Lung Cancer. Hum Pathol (2019) 83:140–8. doi: 10.1016/j.humpath.2018.08.014
20. Liu X, Zhang N, Wang D, Zhu D, Yuan Q, Zhang X, et al. Downregulation of Reticulocalbin-1 Differentially Facilitates Apoptosis and Necroptosis in Human Prostate Cancer Cells. Cancer Sci (2018) 109(4):1147–57. doi: 10.1111/cas.13541
21. Giribaldi G, Barbero G, Mandili G, Daniele L, Khadjavi A, Notarpietro A, et al. Proteomic Identification of Reticulocalbin 1 as Potential Tumor Marker in Renal Cell Carcinoma. J Proteomics (2013) 91:385–92. doi: 10.1016/j.jprot.2013.07.018
22. Huang ZH, Qiao J, Feng YY, Qiu MT, Cheng T, Wang J, et al. Reticulocalbin-1 Knockdown Increases the Sensitivity of Cells to Adriamycin in Nasopharyngeal Carcinoma and Promotes Endoplasmic Reticulum Stress-Induced Cell Apoptosis. Cell Cycle (2020) 19(13):1576–89. doi: 10.1080/15384101.2020.1733750
23. Ueda S, Hashimoto K, Miyabe S, Hasegawa S, Goto M, Shimizu D, et al. Salivary NUS1 and RCN1 Levels as Biomarkers for Oral Squamous Cell Carcinoma Diagnosis. Vivo (2020) 34(5):2353–61. doi: 10.21873/invivo.12048
24. Xiong G, Liu C, Yang G, Feng M, Xu J, Zhao F, et al. Long Noncoding RNA GSTM3TV2 Upregulates LAT2 and OLR1 by Competitively Sponging Let-7 to Promote Gemcitabine Resistance in Pancreatic Cancer. J Hematol Oncol (2019) 12(1):97. doi: 10.1186/s13045-019-0777-7
25. Feng M, Xiong G, Cao Z, Yang G, Zheng S, Qiu J, et al. LAT2 Regulates Glutamine-Dependent mTOR Activation to Promote Glycolysis and Chemoresistance in Pancreatic Cancer. J Exp Clin Cancer Res (2018) 37(1):274. doi: 10.1186/s13046-018-0947-4
26. Hayashi K, Anzai N. Novel Therapeutic Approaches Targeting L-Type Amino Acid Transporters for Cancer Treatment. World J Gastrointest Oncol (2017) 9(1):21–9. doi: 10.4251/wjgo.v9.i1.21
27. Chen D, Zhou XZ, Lee TH. Death-Associated Protein Kinase 1 as a Promising Drug Target in Cancer and Alzheimer’s Disease. Recent Pat Anticancer Drug Discov (2019) 14(2):144–57. doi: 10.2174/1574892814666181218170257
28. Paluszczak J, Misiak P, Wierzbicka M, Woźniak A, Baer-Dubowska W. Frequent Hypermethylation of DAPK, RARbeta, MGMT, RASSF1A and FHIT in Laryngeal Squamous Cell Carcinomas and Adjacent Normal Mucosa. Oral Oncol (2011) 47(2):104–7. doi: 10.1016/j.oraloncology.2010.11.006
29. Paluszczak J, Krajka-Kuźniak V, Małecka Z, Jarmuż M, Kostrzewska-Poczekaj M, Grenman R, et al. Frequent Gene Hypermethylation in Laryngeal Cancer Cell Lines and the Resistance to Demethylation Induction by Plant Polyphenols. Toxicol Vitro (2011) 25(1):213–21. doi: 10.1016/j.tiv.2010.11.003
30. He M, Li K, Yu C, Lv B, Zhao N, Deng J, et al. In Vitro Study of FUZ as a Novel Potential Therapeutic Target in Non-Small-Cell Lung Cancer. Life Sci (2018) 197:91–100. doi: 10.1016/j.lfs.2018.02.007
31. Dejima T, Imada K, Takeuchi A, Shiota M, Leong J, Tombe T, et al. Suppression of LIM and SH3 Domain Protein 1 (LASP1) Negatively Regulated by Androgen Receptor Delays Castration Resistant Prostate Cancer Progression. Prostate (2017) 77(3):309–20. doi: 10.1002/pros.23269
32. Tan J, Jing YY, Han L, Zheng HW, Shen JX, Zhang LH, et al. MicroRNA-203 Inhibits Invasion and Induces Apoptosis of Laryngeal Cancer Cells via Targeting LASP1. Eur Rev Med Pharmacol Sci (2018) 22(19):6350–7. doi: 10.26355/eurrev_201810_16046
33. Wang M, Liu B, Li D, Wu Y, Wu X, Jiao S, et al. Upregulation of IBSP Expression Predicts Poor Prognosis in Patients With Esophageal Squamous Cell Carcinoma. Front Oncol (2019) 9:1117. doi: 10.3389/fonc.2019.01117
34. Zhang Y, He W, Zhang S. Seeking for Correlative Genes and Signaling Pathways With Bone Metastasis From Breast Cancer by Integrated Analysis. Front Oncol (2019) 9:138. doi: 10.3389/fonc.2019.00138
35. Shimada H, Nakashima K, Ochiai T, Nabeya Y, Takiguchi M, Nomura F, et al. Serological Identification of Tumor Antigens of Esophageal Squamous Cell Carcinoma. Int J Oncol (2005) 26(1):77–86. doi: 10.3892/ijo.26.1.77
36. Hoesli R, Birkeland AC, Rosko AJ, Issa M, Chow KL, Michmerhuizen NL, et al. Proportion of CD4 and CD8 Tumor Infiltrating Lymphocytes Predicts Survival in Persistent/Recurrent Laryngeal Squamous Cell Carcinoma. Oral Oncol (2018) 77:83–9. doi: 10.1016/j.oraloncology.2017.12.003
Keywords: laryngeal cancer, recurrence, immune, signature, prognosis
Citation: Zhang H, Zhao X, Wang J and Ji W (2021) Development and Validation of an Immune-Related Signature for the Prediction of Recurrence Risk of Patients With Laryngeal Cancer. Front. Oncol. 11:683915. doi: 10.3389/fonc.2021.683915
Received: 22 March 2021; Accepted: 30 November 2021;
Published: 16 December 2021.
Edited by:
Yury O. Nunez Lopez, AdventHealth, United StatesReviewed by:
Jinhui Liu, Nanjing Medical University, ChinaZhigang Bai, Beijing Friendship Hospital, China
Copyright © 2021 Zhang, Zhao, Wang and Ji. This is an open-access article distributed under the terms of the Creative Commons Attribution License (CC BY). The use, distribution or reproduction in other forums is permitted, provided the original author(s) and the copyright owner(s) are credited and that the original publication in this journal is cited, in accordance with accepted academic practice. No use, distribution or reproduction is permitted which does not comply with these terms.
*Correspondence: Wenyue Ji, ebhzzy3@sj-hospital.org