- 1Department of Neurosurgery, The First Medical Center of People’s Liberation Army General (PLA) Hospital, Beijing, China
- 2Department of Computer Science, City University of Hong Kong, Hong Kong, Hong Kong, SAR China
- 3School of Public Health, Health Science Center of Xi’an Jiaotong University, Xi’an, China
Background: Primary central nervous system lymphoma (PCNSL) is characterized by a lack of specificity and poor prognosis. Further understanding of the tumor heterogeneity and molecular phenotype of PCNSL is of great significance for improving the diagnosis and treatment of this disease.
Methods: To explore the distinct phenotypic states of PCNSL, transcriptome-wide single-cell RNA sequencing was performed on 34,851 PCNSL cells from patients. The cell types, heterogeneity, and gene subset enrichment of PCNSL were identified. A comparison of the PCNSL cells with 21,250 normal human fetal brain (nHFB) cells was further analyzed to reveal the differences between PCNSL and normal sample.
Results: Six cell populations were mainly identified in the PCNSL tissue, including four types of immune cells—B cell, T cell, macrophage and dendritic cell—and two types of stromal cells: oligodendrocyte and meningeal cell. There are significant cellular interactions between B cells and several other cells. Three subpopulations of B cells indicating diffident functions were identified, as well as a small number of plasma cells. Different subtypes of T cells and dendritic cells also showed significant heterogeneity. It should be noted that, compared with normal, the gene expression and immune function of macrophages in PCNSL were significantly downregulated, which may be another important feature of PCNSL in addition to B cell lesions and may be a potential target for PCNSL therapy.
Introduction
Primary central nervous system (CNS) lymphoma (PCNSL), defined as diffuse large B cell lymphoma (DLBCL) confined to the CNS, can occur in the setting of immunosuppression (HIV/AIDS, post-transplant) or in immunocompetent individuals (1, 2). The incidence of PCNSL accounting for 2% to 3% of all CNS neoplasms (3) has reportedly increased in the immunocompetent population (4). Incidence of PCNSL is recently increasing in the elderly (5). It is not possible to morphologically distinguish PCNSL and peripheral DLBCL. This non-Hodgkin aggressive B-cell lymphoma is distinguished from extra-cerebral DLBCL by its poorer prognosis. The tumor cells are mainly large, with numerous apoptotic cells or widespread necrosis, which commonly hinders diagnosis in small biopsies (6, 7).
The molecular subtype of PCNSL has been studied by different methodological approaches with conflicting conclusions. On the basis of several IHC studies, an ABC-like immunophenotype is typical (8, 9), but immunoglobulin heavy-chain gene mutational signatures also provide evidence for germinal center exposure, indicating that PCNSL develops from a B-cell that has been exposed to a germinal center influence outside the CNS (10–12). In addition, results of immunophenotyping studies suggest that tumor cells originate from a late germinal center to an early postgerminal center stage (13), while gene expression profiling studies indicate that PCNSLs are distributed among the spectrum of systemic DLBCL with roughly equal proportion of ABC and GC cases (14, 15).
Standard chemotherapeutic regimens for systemic DLBCL show little efficacy in PCNSL, likely because of inefficient drug delivery across the blood–brain barrier (16). High-dose methotrexate-based chemotherapy is the standard therapy for PCNSL. Chemotherapy with whole-brain radiation therapy has produced response rates up to 80%–90% and median overall survival up to 5 years. While treatment response rates are high, relapses are frequent and prognosis after recurrence is poor with 5-year survival rates ranging from 22% to 40% (17, 18). Up to 50% of patients with PCNSL will relapse, and 10%–15% demonstrate primary refractory disease, indicating a significant unmet therapeutic need (19). However, PCNSL has a poor prognosis compared with that of DLBCL (20) and the reason for the difference in prognosis between PCNSL and DLBCL has not been elucidated.
The rarity of the disease and the difficulty of obtaining intracranial specimens have hindered understanding of the pathophysiology of PCNSL. The observed overexpression of BCL6 and aberrant somatic hypermutation (aSHM) of many genes, together with expression of immunoglobulin M at the cell surface, have suggested that PCNSL cells may be arrested at the stage of terminal B-cell differentiation (21). The genomic alterations (GAs) underlying PCNSL have not been comprehensively studied. Single-nucleotide mutations in various genes, including MYD88, CD79b, PIM1, and BTG2, have been reported as the most prevalent genetic alterations in PCNSL (22–24).
Genome-wide gene expression in PCNSL compared with non-CNS DLBCL suggests that PCNSL has specific signatures to be distinguished from non-CNS DLBCL and greater molecular heterogeneity (14, 25). Of the genetic changes that lead to PCNSL, very little is known and no characteristic genetic alterations have been defined thus far. However, few retrospective studies have examined the detailed molecular network and cell signaling based on diagnosis with gene mutations and CNVs or the prognosis of patients with PCNSL.
Some vague evidences suggest that CNS lymphoma and peripheral lymphoma are heterogeneous, which may be related to different immune environments and different origins of lymphoma cells. At present, there is no clinical significance to reveal the molecular characteristics of CNS lymphoma.
Here, we performed an analysis of scRNA-seq data of CNS tissue sequenced by 10x Genomics to identify the cell types of CNS cells; we next performed differential expression (DE) analysis on two PCNSL-associated cells (i.e., B cell, plasma cell) and gene set enrichment analysis (GSEA) of their DE genes on the cell type-specific marker genes to examine the cell-specific functionalities in PCNSL development.
Materials and Methods
Single-Cell RNA Sequencing
Human PCNSL tumor samples were obtained surgically from clinical patients confirmed by pathology from our hospital. After sample collection processing and suspensions, we performed scRNA-seq following the manufacturer’s protocol. Briefly, the cells were washed with PBS and resuspended in 500 μl PBS. scRNA-seq libraries were prepared using a Chromium Single cell 3′ Reagent kit, version 2. Amplified cDNA and final libraries were evaluated using a High Sensitivity DNA Kit (Agilent Technologies). Sequencing was performed on NovaSeq 6000 (Illumina) at a depth of approximately 400M reads.
Single-Cell Data Processing
The Cell Ranger software pipeline (version 4.0, http://support.10Xgenomics.com/single-cell-gene-expression/software/overview/welcome) provided by 10x Genomics was used to demultiplex cellular barcodes. Unique molecular identifier (UMI) counts were obtained by mapping reads to the human reference genome (GRCH38 3.1.0) genome and align transcriptomes using the STAR aligner and down-sample reads as required to generate normalized aggregate data across samples. In the end, a matrix of gene counts by cells was produced. We processed the UMI count matrix using the Seurat R package (version 4.0.2), resulting in 34,851 cells with 36,601 genes for PCNSL samples.
We first removed the likely multiplet captures, which is a major concern in microdroplet-base experiments through DoubletFinder. We filtered cells at the cell and gene levels to obtain the reliability results of PCNSL scRNA-seq data, respectively. We removed the low-quality cells with the following criteria: (i) the number of expressed genes was <200 or >2,000; (ii) the number of total counts was > 20,000; and (iii) the percentage of mitochondrial counts > 10%. We only kept the genes detected in at least 20 cells. After applying these quality control criteria, we obtained 20,307 cells with 12,229 genes in total, which were used for downstream analysis.
To analyze the differences between PCNSL and normal brain cells, we referred to a published scRNA-seq data of normal human fetal brain (nHFB). The raw data are from the European Genome-Phenome Archive: EGAS00001004422 (https://ega-archive.org/studies/EGAS00001004422). For accessibility reasons, we directly used the cellranger matrices from HFA567_total.filtered_gene_matrices, HFA570_total.filtered_gene_matrices, and HFA571_total.filtered_gene_matrices (https://datahub-262-c54.p.genap.ca/GBM_paper_data/GBM_cellranger_matrix.tar.gz) (26). The following criteria were used for nHFB scRNA data processing: (i) the number of expressed genes was <100 or >3,000; (ii) the number of total counts was > 20,000; and (iii) the percentage of mitochondrial counts > 10%. We only kept the genes detected in at least 20 cells. After applying these quality control criteria, we obtained 20,015 cells with 16,608 genes from 21,255 cells with 33,694 genes in total, which were used for downstream analysis.
Cell Subpopulation Detection
scRNA-seq data were normalized using log transforms and scaled so that the mean expression across cells is 0 and the variance across cells is 1. Then, we performed dimension reduction and visualization on the single-cell data through the Seurat R package (version 4.0.2) (1, 27). We used the VST method (28) to obtain the top 3,000 highly variable genes and used the PCA method to reduce dimensionality. The top 21 principal components (PCs) were selected for tSNE to visualize the cell clustering (29). We then clustered the cells using the Leiden algorithm (30) implemented in the Seurat package, with the resolution parameter set to be 0.7. There are two methods for annotation cell types: (i) the Wilcox test was used to identify significantly differentially expressed genes (DEGs) between clusters. To identify the marker genes of each cluster, we combined the rest of the cells and set them to control cells. For the process of identifying DEGs between PCNSL and normal samples, we set cells of one cluster in PCNSL samples as the treatment group and the cells of this cluster in normal samples as the control group. We considered the genes with adjusted p-value < 0.05 and |log2FC|> 0.58 for each scRNA-seq cell type as the DEGs. The top 30 genes are used for the annotation cell type manual. According to the detailed intracellular marker gene, we combined with the CellMarker (http://bio-bigdata.hrbmu.edu.cn/CellMarker/) database. (ii) By comparing scRNA-seq with HumanPrimaryCellAtlasData and BlueprintEncodeData data, we utilized both the SingleR R package (version 1.4.1) and manual work to assign cell types to cluster automatic annotation. Finally, we compare the annotation results and determine the cell types.
Gene Function Enrichment Analysis
We then performed gene ontology (GO) enrichment analysis using the database for annotation, visualization, and integrated discovery database (DAVID: version 6.8, with the significant DEGs https://david.ncifcrf.gov/summary.jsp). It is an essential tool for systematically extracting biological information from a set of genes. Adj. p-value < 0.05 was considered statistically significant.
Cell Communication Analysis
In order to concretely prove the direct interaction between cell subpopulations, we used CellPhoneDB (version 2.1.7) to conduct interactive analysis on cell subpopulations. CellPhoneDB is a publicly available repository of selected receptors, ligands, and their interactions (31). Here, we used it (version 2.1.7) to explore the cell–cell communication by immune-related proteins. Here, we focus on the four classes of immune cell subpopulations and immune-related genes. There are eight classes of gene lists collected in our research: chemokines, T helper type 1 genes (Th1), T helper type 2 genes (Th2), T helper 17 genes (Th17), T regulators (Treg), and costimulatory, coinhibitory, and immune niche genes. The circle edge width is proportional to the number of cells in each cell cluster and the communication score between interacting cell clusters, respectively.
Results
Identification of Cell Types in PCNSL and nHFB
After quality filtering, 40,322 cells with 20,452 genes were obtained from the PCNSL and nHFB samples (details in Materials and Methods). All cells were classified into 13 subpopulations by combining the automatic and manual annotation methods. The cell types were identified as B cell, T cell, macrophage, dendritic cell, oligodendrocyte, meningeal cell, excitatory neuron (EN), interneuron (IN), radial glia (RG), inhibitory neuronal progenitor cell (INP), excitatory neuronal progenitor (ENP), astrocyte, and oligodendrocyte progenitor cell (OPC) (Figure 1A and Table 1). The combination comparison (Figures 1A, B) indicated that PCNSL and nHFB had significant differences in cell heterogeneity, and there were certain similarities only in macrophages and meningeal cells. The B cell, T cell, macrophage, and dendritic cell population accounted for the highest proportion in PCNSL (Table 2, Figure 1C). By extracting the average value of top 30 gene expression in each group, and performing Pearson correlation coefficient (PCC) expression correlation analysis, the difference between groups is greater than the difference within the groups, which proves that our subpopulation annotation has high sensitivity and specificity (Figure 1D). The result of the bubble plot reveals that typical marker genes are particularly expressed in the cell subpopulations (Figure 1E). Overall, the annotation results show the complex cell composition in PCNSL tissue.
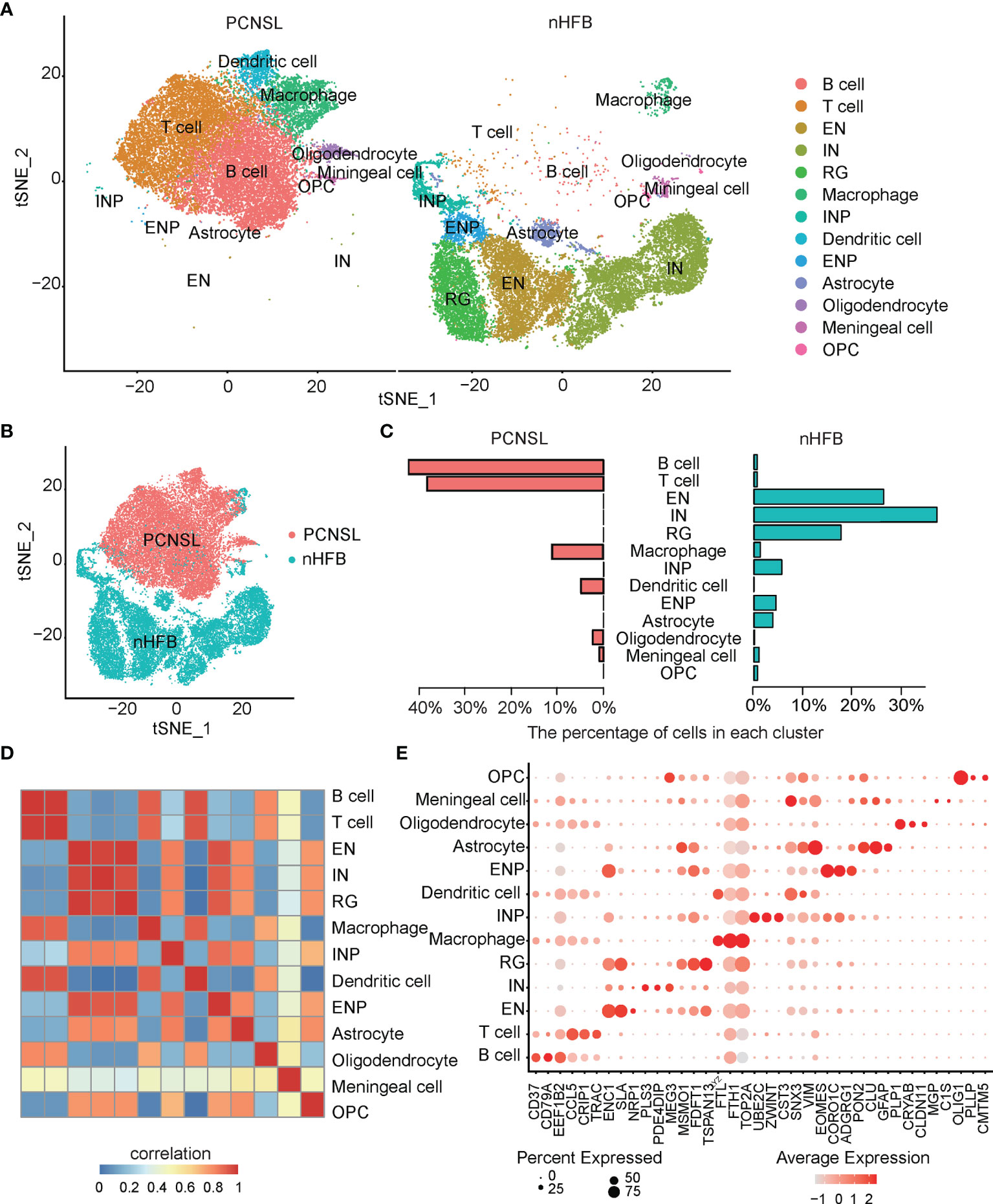
Figure 1 Single-cell analysis of CNS scRNA-seq data sets. (A) The visualization results of cell subpopulations in PCNSL and normal CNS tissue samples. (B) The tSNE displays PCNSL and normal CNS cells: red indicates PCNSL cells, and green indicates normal cells. (C) The barplot represents the proportion of various cell subgroups in PCNSL and normal CNS tissue samples individually. (D) The heatmap describes the PCC values of the average expression values of the top 30 genes in each cluster. (E) The bubble chart shows that three marker genes are selected for each cell subgroup, and their expression in all cell subpopulations is plotted.
The Heterogeneity of B Cells in PCNSL and Their Communication Interaction With Other Immune Cells
Malignant proliferation and abnormal differentiation of B cells (toward plasma cells) are the main pathological characteristics of PCNSL. Molecular targeted therapy targeting B cells has also been considered as a promising way to treat PCNSL in recent years. Nevertheless, the heterogeneity of B cells in PCNSL remains unclear. Therefore, we first analyzed the heterogeneity of B-cell subtypes and genetic characteristics in PCNSL from single-cell resolution. CD79A served as a typical marker gene of the B cell population in total PCNSL cells (Figure 2A). Then, the identical methods with cell population detection were used to re-cluster B cells into four distinct subclusters: B cell-1, B cell-2, B cell-3, and plasma cell (Figure 2B). We identified the marker genes for each subcluster among the B cell population (Figure 2C and Supplementary Table 1). The functional annotations of each subcluster were counted (Supplementary Table 2) and the three significant pathways are shown in Figure 2D. The B cell-1 cluster highly expressed marker genes (e.g., CD79A, CD79B, TCL1A) of the classical B cells. The B cell-2 cluster was significantly marked by, e.g., NCL, LTB, NEAT1, and enriched functional pathways of antigen processing and presentation of endogenous peptide antigen via MHC class I and positive regulation of T cell-mediated cytotoxicity, suggesting that these B cells have strong antigen–antibody immunity and synergistic disturbance with T cells. The B cell-3 cluster (e.g., CST7, CXCL13, MT-ND3) indicated functions of cell–cell adhesion regulation, neuroinflammation response, and hydrogen peroxide biosynthetic process. Plasma cells (e.g., IGHG1, IGHG3, IGHG4) played a significant role in complement activation.
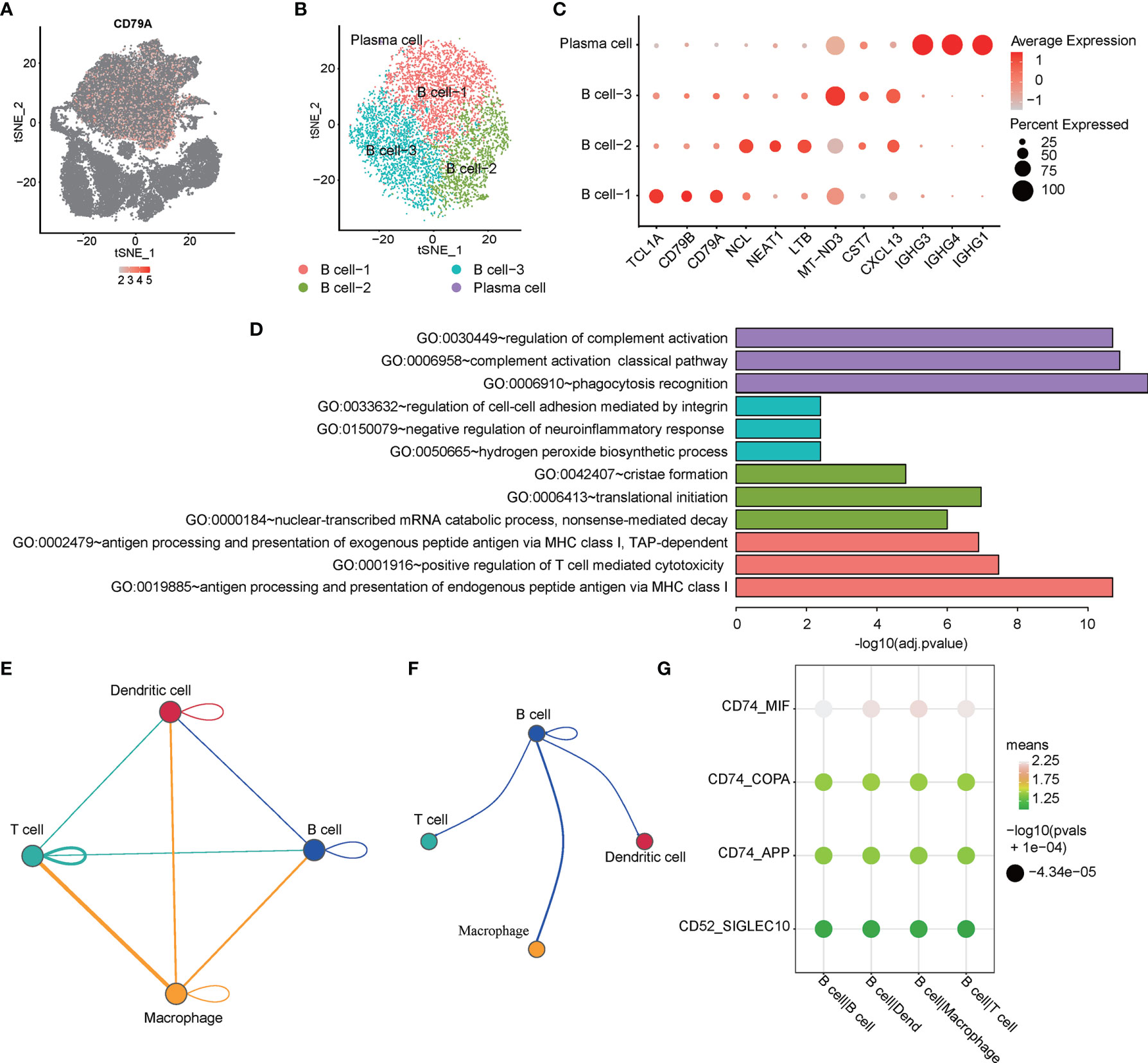
Figure 2 Single-cell analysis of heterogeneity for B cell and cell communication. (A) The expression distribution of the B cell maker gen -- CD79A in all cells. (B) tSNE visualization describes the re-cluster results of B cell subgroups. (C) The bubble chart shows that selected three marker genes are selected for each cell subgroup, and their expression in all cell subpopulations is plotted. (D) The top3 representative GO functional annotation categories of marker genes for each sub-population. (E) The interactions among immune-related cell subpopulations: B cell, T cell, macrophage, and dendritic cell subpopulation. (F) The interaction pairs of B cell with T cell, macrophage, and dendritic cell, respectively. (G) The ligand-receptor pairs are contributing to the signaling from B cells to the other three clusters.
Cell–cell communication is a fundamental process that shapes biological tissue. In particular, ligand–receptor pairs can infer intercellular communication from the coordinated expression of their homologous genes (32). Here, we focused on four classes of immune cell subpopulations and immune-related genes. Figure 2E shows the interactions among immune-related cell subpopulations: B cell, T cell, macrophage, and dendritic cell subpopulation. The circle edge width is proportional to the number of cells in each cell cluster and the communication score between interacting cell clusters, respectively. The circle plot (Figure 2F) showed the interactions of B cell with T cell, macrophage, and dendritic cell, respectively. The bubble plots (Figure 2G) show the ligand–receptor pairs contributing to the signaling from B cells to other three clusters. The circle plots show that the interaction between macrophage and T cell has the most count numbers among others. Interestingly, we identified that significant target CD74 with MIF, COPA, and APP might participate the process of B cell interacting with T cell, macrophage, and dendritic cell based on the CellPhoneDB results, and CD74–MIF interaction was the most significant among these.
The Heterogeneity of T Cells and Dendritic Cells in PCNSL
T cells and dendritic cells have a high proportion in PCNSL and are closely related to the immune microenvironment of PCNSL. The subpopulations of T cells and dendritic cells in PCNSL were analyzed. LTB serves as a marker gene of the T cell population (Figure 3A). Then, T cells were re-clustered into four distinct subclusters: naive T cells, natural killer T (NKT) cells, T helper cells, and MPCs (Figure 3B). Further clustering of the T cell subpopulation in PCNSL gave rise to four subpopulations with specific gene signatures, including T helper cell group (LTB, NEAT1, and IL7R), NKT cell group (DUSP1, CCL5, and JUNB), MPC cell group (UBE2C, STMN1, and BIRC5), and classical T cell group (NCL, HNRNPA3, and DUT). Bubble heatmap showing expression levels of selected signature genes in ESCA. Dot size indicates a fraction of expressing cells, colored based on normalized expression levels (Figure 3C). The proportions of these subtypes are shown in Figure 3D. The marker genes were identified for each subcluster among the T cell population (Supplementary Table 3), and the three typical marker genes for annotation are shown in a bubble plot (Figure 3D). Three significant function pathways enriched by the marker genes of the four subclusters are shown in Figure 3E (Supplementary Table 4). DEGs of MPC were highly enriched in the G2/M transition of the mitotic cell cycle pathway, which was closely related to the proliferation and differentiation of various lymphocytes in PCNSL and might be the main source of tumor germination.
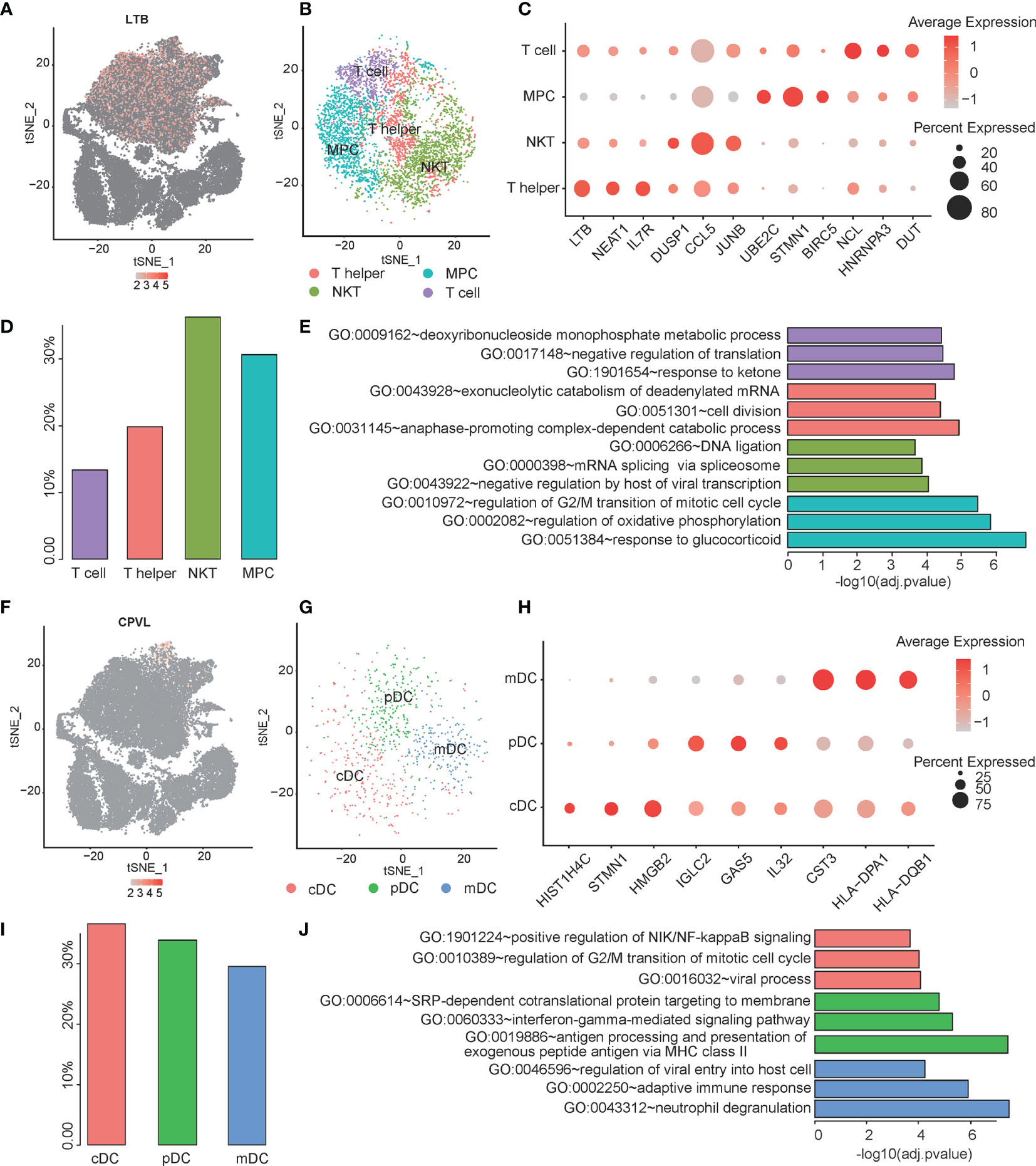
Figure 3 Single-cell analysis of heterogeneity for T cell and Dendritic cell sub-population. (A) The expression distribution of the T cell maker gene -- LTB in all cells. (B) The tSNE visualization describes the re-cluster results of T cell subgroups. (C) The bubble chart shows that selected three marker genes are selected for each cell subgroup, and their expression in all cell subpopulations is plotted. (D) The proportion of each subpopulation for T cell. (E) The top three significant GO categories of marker genes for each subgroup are enriched. (F) The expression distribution of the Dendritic cell maker gene -- CPVL in all cells. (G) The tSNE visualization describes the re-cluster results of dendritic cell subgroups. (H) The bubble chart shows that selected three marker genes are selected for each cell subgroup, and their expression in all cell subpopulations is plotted. (I) The proportion of each subpopulation for dendritic cells. (J) The top three significant GO categories of marker genes for each subgroup are enriched.
The dendritic cell population was labeled by a marker gene CPVL (Figure 3F). Then, the dendritic cells were re-clustered into three distinct clusters: conventional dendritic cell (cDC), myeloid dendritic cell (mDC), and plasmacytoid dendritic cell (pDC) (Figure 3G). We identified the marker genes for each subcluster among the dendritic cell population (Supplementary Table 5). The typical marker genes for annotation are shown in a bubble plot (cDC: e.g., STMN1, HMGB2, HIST1H4C; mDC: e.g., CST3, HLA-DPA1, HLA-DQB1; pDC: IGLC2, GAS5, IL32) (Figure 3H and Supplementary Table 5). The proportions of the subclusters are shown in Figure 3I. Evaluating known pathway expression in every three subpopulations of dendritic cell group using GO enrichment analysis revealed strong enrichment of regulation of viral entry into host cell, adaptive immune response, and neutrophil degranulation for mDC subpopulation; SRP-dependent cotranslational protein targeting to membrane , interferon-gamma-mediated signaling pathway, and antigen processing and presentation of exogenous peptide antigen via MHC class II for pDC; and positive regulation of NIK/NF-kappaB signaling, regulation of G2/M transition of mitotic cell cycle, and viral process in cDC. The function pathways of the marker genes enriched by GO analysis are shown in Figure 3J and Supplementary Table 6.
Identification and Variation Analysis of Macrophage Between PCNSL and Normal Brain
It is noted that macrophage in PCNSL and HFB shows obvious difference in cell proportion and distribution. Then they may also have some differences in function. LYZ was used as a marker gene of the macrophage population (Figure 4A). NFKBIA (log2FC = -0.38; adj. p-value = 1.32E-8), BTG2 (log2FC = -0.59, adj. p-value = 1.44E-18), NFKBIZ (log2FC = -0.28, adj. p-value = 1.18E-20), TLR4 (log2FC = –0.53, adj. p-value = 7.35E-34), and TLR10 (log2FC = -0.54, adj. p-value = 3.69E-40) are selected for visualization of the differentially expressed between PCNSL and nHFB samples (Figure 4B). There are 143 DEGs between cancer and normal cells. Fifty genes are upregulated in cancer cells compared to the normal cells, while 93 genes are downregulated in cancer cells compared to normal cells (Figure 4C and Table 3). Moreover, the top 20 enriched functional pathways of these DEGs are shown in Figures 4D, E. Compared with PCNSL, macrophages in nHFB exhibit normal immune function, and the un-regulated DEGs are mainly enriched in pathways including immune response, neutrophil chemotaxis, and chemokine-mediated signaling pathway (Figure 4D). However, this classic immune function of macrophage in PCNSL was significantly downregulated, and no significant pathways were found (Figure 4E). These results suggest that the immune function of macrophages in PCNSL is significantly suppressed.
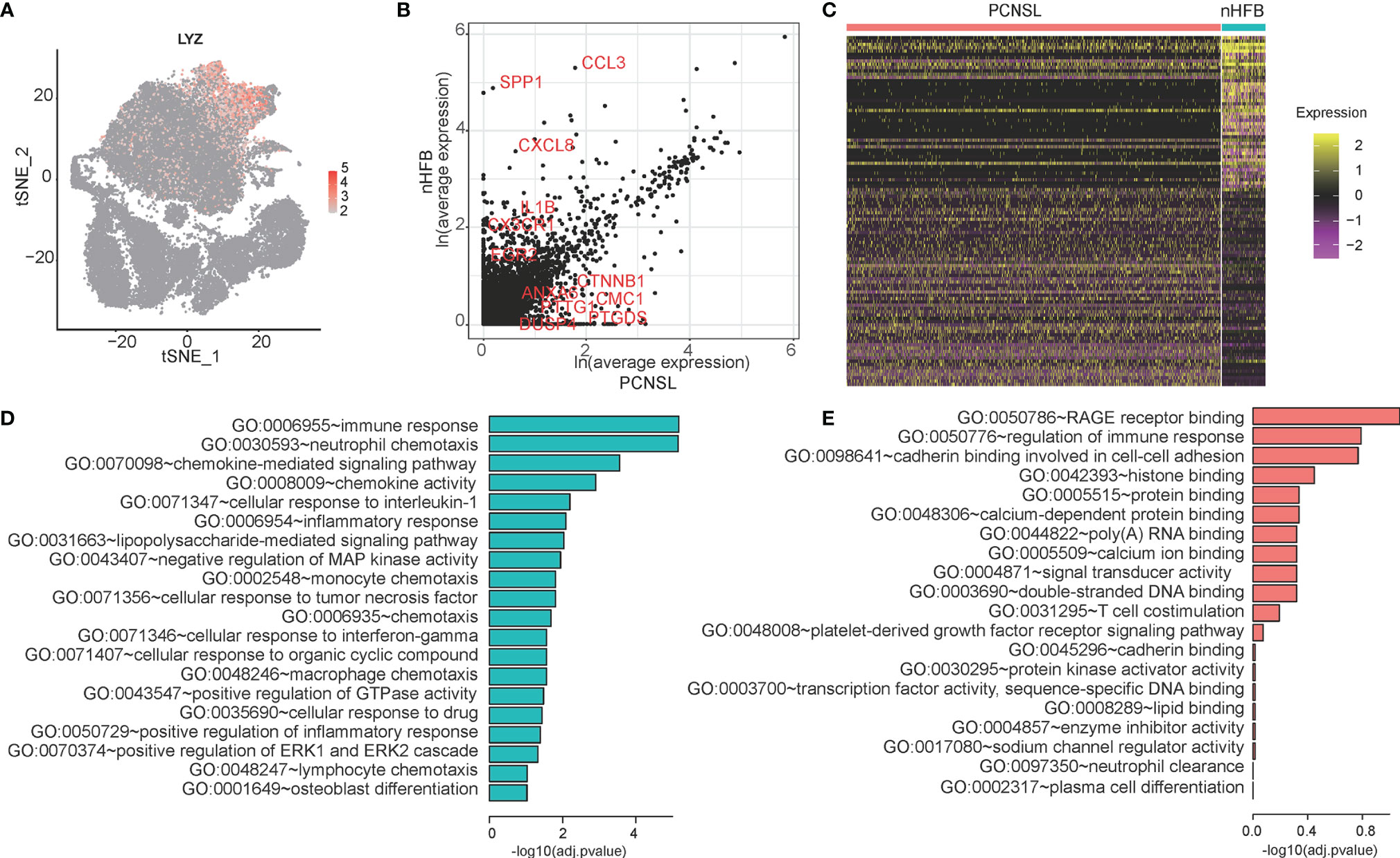
Figure 4 The differential expression analysis of macrophage between cancer and normal samples. (A) The expression distribution of the macrophage cell marker gene -- LYZ in all cells. (B) The scatter plot of genes selected five significant DEGs in the macrophage cell population between cancer and normal samples. (C) The heatmap of DEGs of macrophage. (D, E) The GO categories of down-regulated genes (green) and up-regulated genes (red) in PCNSL samples compared with normal samples.
Discussion
CNS tissue is the key site of PCNSL pathogenesis and progression which consists of diverse types of cells. Understanding the molecular phenotype of each cell type to the PCNSL is an important step to reveal the pathogenesis of PCNSL. The availability of scRNA-seq data allows us to perform molecular and functional heterogeneity analysis for different cells in the PCNSL tissue. In this study, we investigated CNS tissues at a single-cell resolution using gene expression profiling. We mapped the immune microenvironment of PCNSL and identified the cellular markers and functional signals of immune cells such as B cells, macrophages, and dendritic cells in PCNSL, which provided a partial basis for guiding the precise treatment of PCNSL.
Abnormal proliferation and differentiation of B cells are directly related to the pathological basis of PCNSL, and scRNA-seq enables us to directly parse the heterogeneity of B cells from single-cell resolution. We identified that B cells can be divided into three subgroups with completely different gene expression and function, including a small number of terminally differentiated plasma cells (IGHG1, IGHG3, IGHG4). We identified that B cells can be divided into three subgroups with completely different gene expression and function, including a small number of terminally differentiated plasma cells. In addition to the subsets of normal B cells (B cell-1 overexpressed CD79A and CD79B), B cell-2 showed an important role in antigen processing and regulating the cytotoxicity of T cells, and its function was similar to that of dendritic cells, which may be related to the abnormal differentiation of B cells in PCNSL. This also indicated from the molecular phenotype that B cells in PCNSL were in the pathological overactivation state.
Cell–cell communication between B cells and other immune cells is also an important mechanism for the progression of PCNSL disease (12, 15, 33). Surprisingly, we found that CD74 might be a key target to regulate the communication between B cells and the other three types of immune cells (T cell, macrophage, dendritic cell) and CD74–MIF was identified to be the most significant interaction. The macrophage migration inhibitory factor (MIF) receptor CD74 is overexpressed in various neoplasms, mainly in hematologic tumors, and currently investigated in clinical studies. Zeiner et al. summarized that the MIF–CD74A interaction is restricted to macrophages, associated with survival in glioma (34). CD74 is quickly internalized and recycles after antibody binding; therefore, it constitutes an attractive target for antibody-based treatment strategies. CD74 has been further described as one of the most upregulated molecules in human glioblastomas. They also pointed that CD74 expression in human gliomas is restricted to GAMs and positively associated with patient survival. Moreover, CD74 represents a positive prognostic marker most probably because of its association with an M1-polarized immune milieu in high-grade gliomas. Our study strongly suggests that CD74 of B cells in PCNSL may be an important cellular and molecular pathological basis for its coordination and disturbance with other immune cells to induce the disorder of the immune microenvironment. This may provide an important basis for targeted therapy of PCNSL. However, there are still limitations in the above conclusions, namely, that malignant and non-malignant B cells in PCNSL have not been identified and distinguished. These analyses are mainly based on the mixed population of B cells in PCNSL. In this regard, the separated malignant and non-malignant B cells from PCNSL tumors should be accurately analyzed for the tumor cell heterogeneity of PCNSL in the future.
In addition, we also demonstrated that immune cells would be affected by the tumor microenvironment. Heterogeneities were also observed in these cell types. We identified T cell, T helper cell, NKT cell, and MPC subtypes within T cell populations that possessed different ontologies. Moreover, we also identified three subtypes within the dendritic cells, including conventional dendritic cell, myeloid dendritic cell, and plasmacytoid dendritic cell. They also enriched into NF-κB signaling, antigen processing and presentation, and neutrophil degranulation pathways, respectively. Further studies on the immune regulation mechanism of these subtypes of T cells and dendritic cells might provide a new strategy for the treatment of PCNSL.
In conclusion, our single-cell analysis enabled us to study the cellular heterogeneity and gene expression characteristics of PCNSL at a single-cell resolution and whole-transcriptome scale. Our study is the first to analyze the cellular and molecular pathological map at single-cell resolution. Despite the small size of single-cell samples in our study, it reveals novel potential for targeted immunotherapy of PCNSL.
Data Availability Statement
The raw and processed data supporting the findings in this study are available from the corresponding author, and has been submitted to the Gene Expression Omnibus (GEO) database under the accession code GSE181304 (https://www.ncbi.nlm.nih.gov/geo/query/acc.cgi?acc=GSE181304).
Ethics Statement
Written informed consent was not obtained from the individual(s) for the publication of any potentially identifiable images or data included in this article.
Author Contributions
Conceptualization, B.Y.W. and Z.L.; Methodology, B.Y.W. and Z.L.; Validation, Y.F. and G.C.; Formal Analysis, B.Y.W. and Z.L.; Resources, B.Y.W., S.W.W., C.D., W.R. and F.Y.; Data Curation, Y.F.; Visualization, Y.F.; Writing-Original Draft, B.Y.W., Z. L. and Y.F.; Writing-Review & Editing, B.Y.W., Z.L, and J.N.Z.; Supervision, G.C., and J.N.Z. All authors contributed to the article and approved the submitted version.
Funding
This study was funded by the Innovation Cultivation Fund of the Sixth Medical Center of PLA General Hospital (CXPY201913) and the Youth Science Foundation Project of the National Natural Science Foundation of China (81801165).
Conflict of Interest
The authors declare that the research was conducted in the absence of any commercial or financial relationships that could be construed as a potential conflict of interest.
Publisher’s Note
All claims expressed in this article are solely those of the authors and do not necessarily represent those of their affiliated organizations, or those of the publisher, the editors and the reviewers. Any product that may be evaluated in this article, or claim that may be made by its manufacturer, is not guaranteed or endorsed by the publisher.
Acknowledgments
We appreciate the support in the study design and data analysis from Professor Dayun Feng (Department of Neurosurgery, Tangdu Hospital Affiliated to Air Force Medical University) and Dr. Yong Zhang (Genergy Bio-technology (Shanghai) Co., Ltd.) for the technical support.
Supplementary Material
The Supplementary Material for this article can be found online at: https://www.frontiersin.org/articles/10.3389/fonc.2021.683007/full#supplementary-material
References
1. Diamond C, Taylor TH, Aboumrad T, Anton-Culver H. Changes in Acquired Immunodeficiency Syndrome-Related Non-Hodgkin Lymphoma in the Era of Highly Active Antiretroviral Therapy: Incidence, Presentation, Treatment, and Survival. Cancer (2006) 106:128–35. doi: 10.1002/cncr.21562
2. Soliman RM, Elhaddad A, Oke J, Eweida W, Sidhom I, Ahmed S, et al. Trends in Primary Central Nervous System Lymphoma Incidence and Survival in the U.S. Br J Haematol (2016) 174:417–24. doi: 10.1111/bjh.14073
3. Korfel A, Schlegel U. Diagnosis and Treatment of Primary CNS Lymphoma. Nat Rev Neurol (2013) 9:317–27. doi: 10.1038/nrneurol.2013.83
4. Olson JE, Janney CA, Rao RD, Cerhan JR, Kurtin PJ, Schiff D, et al. The Continuing Increase in the Incidence of Primary Central Nervous System Non-Hodgkin Lymphoma: A Surveillance, Epidemiology, and End Results Analysis. Cancer (2002) 95:1504–10. doi: 10.1002/cncr.10851
5. Villano JL, Koshy M, Shaikh H, Dolecek TA, McCarthy BJ. Age, Gender, and Racial Differences in Incidence and Survival in Primary CNS Lymphoma. Br J Cancer (2011) 105:1414–8. doi: 10.1038/bjc.2011.357
6. Choi YL, Suh YL, Kim D, Ko YH, Sung CO, Lee JI. Malignant Lymphoma of the Central Nervous System: Difficult Histologic Diagnosis After Glucocorticoid Therapy Prior to Biopsy. Clin Neuropathol (2006) 25:29–36. doi: 10.1080/02699200400016539
7. Commins DL. Pathology of Primary Central Nervous System Lymphoma. Neurosurg Focus (2006) 21:E2. doi: 10.3171/foc.2006.21.5.3
8. Liu J, Wang Y, Liu Y, Liu Z, Cui Q, Ji N, et al. Immunohistochemical Profile and Prognostic Significance in Primary Central Nervous System Lymphoma: Analysis of 89 Cases. Oncol Lett (2017) 14:5505–12. doi: 10.3892/ol.2017.6893
9. Raoux D, Duband S, Forest F, Trombert B, Chambonnière ML, Dumollard JM, et al. Primary Central Nervous System Lymphoma: Immunohistochemical Profile and Prognostic Significance. Neuropathology (2010) 30:232–40. doi: 10.1111/j.1440-1789.2009.01074.x
10. Thompsett AR, Ellison DW, Stevenson FK, Zhu D. V(H) Gene Sequences From Primary Central Nervous System Lymphomas Indicate Derivation From Highly Mutated Germinal Center B Cells With Ongoing Mutational Activity. Blood (1999) 94:1738–46. doi: 10.1182/blood.V94.5.1738
11. Montesinos-Rongen M, Küppers R, Schlüter D, Spieker T, Van Roost D, Schaller C, et al. Primary Central Nervous System Lymphomas Are Derived From Germinal-Center B Cells and Show a Preferential Usage of the V4-34 Gene Segment. Am J Pathol (1999) 155:2077–86. doi: 10.1016/S0002-9440(10)65526-5
12. Larocca LM, Capello D, Rinelli A, Nori S, Antinori A, Gloghini A, et al. The Molecular and Phenotypic Profile of Primary Central Nervous System Lymphoma Identifies Distinct Categories of the Disease and Is Consistent With Histogenetic Derivation From Germinal Center-Related B Cells. Blood (1998) 92:1011–9. doi: 0006-4971/98/9203-0004
13. Sugita Y, Tokunaga O, Nakashima A, Shigemori M. SHP-1 Expression in Primary Central Nervous System B-Cell Lymphomas in Immunocompetent Patients Reflects Maturation Stage of Normal B Cell Counterparts. Pathol Int (2004) 54:659–66. doi: 10.1111/j.1440-1827.2004.01677.x
14. Rubenstein JL, Fridlyand J, Shen A, Aldape K, Ginzinger D, Batchelor T, et al. Gene Expression and Angiotropism in Primary CNS Lymphoma. Blood (2006) 107:3716–23. doi: 10.1182/blood-2005-03-0897
15. Montesinos-Rongen M, Brunn A, Bentink S, Basso K, Lim WK, Klapper W, et al. Gene Expression Profiling Suggests Primary Central Nervous System Lymphomas to be Derived From a Late Germinal Center B Cell. Leukemia (2008) 22:400–5. doi: 10.1038/sj.leu.2405019
16. Mead G M, Bleehen NM, Gregor A, Bullimore J, Shirley D, Rampling RP, et al. A Medical Research Council Randomized Trial in Patients With Primary Cerebral non-Hodgkin Lymphoma: Cerebral Radiotherapy With and Without Cyclophosphamide, Doxorubicin, Vincristine, and Prednisone Chemotherapy. Cancer (2000) 89:1359–70. doi: 10.1002/1097-0142(20000915)89:6<1359::AID-CNCR21>3.0.CO;2-9
17. Gavrilovic IT, Hormigo A, Yahalom J, DeAngelis LM, Abrey LE. Long-Term Follow-Up of High-Dose Methotrexate-Based Therapy With and Without Whole Brain Irradiation for Newly Diagnosed Primary CNS Lymphoma. J Clin Oncol (2006) 24:4570–4. doi: 10.1200/JCO.2006.06.6910
18. Nayak L, Hedvat C, Rosenblum MK, Abrey LE, DeAngelis LM. Late Relapse in Primary Central Nervous System Lymphoma: Clonal Persistence. Neuro Oncol (2011) 13:525–9. doi: 10.1093/neuonc/nor014
19. Langner-Lemercier S, Houillier C, Soussain C, Ghesquières H, Chinot O, Taillandier L, et al. Primary CNS Lymphoma at First Relapse/Progression: Characteristics, Management, and Outcome of 256 Patients From the French LOC Network. Neuro Oncol (2016) 18:1297–303. doi: 10.1093/neuonc/now033
20. Prodduturi P, Bierman PJ. Current and Emerging Pharmacotherapies for Primary CNS Lymphoma. Clin Med Insights Oncol (2012) 6:219–31. doi: 10.4137/CMO.S7752
21. Deckert M, Montesinos-Rongen M, Brunn A, Siebert R. Systems Biology of Primary CNS Lymphoma: From Genetic Aberrations to Modeling in Mice. Acta Neuropathol (2014) 127:175–88. doi: 10.1007/s00401-013-1202-x
22. Zheng M, Perry AM, Bierman P, Loberiza F, Nasr MR, Szwajcer D, et al. Frequency of MYD88 and CD79B Mutations, and MGMT Methylation in Primary Central Nervous System Diffuse Large B-Cell Lymphoma. Neuropathology (2017) 37:509–16. doi: 10.1111/neup.12405
23. Yamada S, Ishida Y, Matsuno A, Yamazaki K. Primary Diffuse Large B-Cell Lymphomas of Central Nervous System Exhibit Remarkably High Prevalence of Oncogenic MYD88 and CD79B Mutations. Leuk Lymphoma (2015) 56:2141–5. doi: 10.3109/10428194.2014.979413
24. Nakamura T, Tateishi K, Niwa T, Matsushita Y, Tamura K, Kinoshita M, et al. Recurrent Mutations of CD79B and MYD88 Are the Hallmark of Primary Central Nervous System Lymphomas. Neuropathol Appl Neurobiol (2016) 42:279–90. doi: 10.1111/nan.12259
25. Tun HW, Personett D, Baskerville KA, Menke DM, Jaeckle KA, Kreinest P, et al. Pathway Analysis of Primary Central Nervous System Lymphoma. Blood (2008) 111:3200–10. doi: 10.1182/blood-2007-10-119099
26. Couturier CP, Ayyadhury S, Le PU, Nadaf J, Monlong J, Riva G, et al. Single-Cell RNA-Seq Reveals That Glioblastoma Recapitulates a Normal Neurodevelopmental Hierarchy. Nat Commun (2020) 11:3406. doi: 10.1038/s41467-020-17186-5
27. Satija R, Farrell JA, Gennert D, Schier AF, Regev A. Spatial Reconstruction of Single-Cell Gene Expression Data. Nat Biotechnol (2015) 33(5):495–502. doi: 10.1038/nbt.3192
28. Hafemeister C, Satija R. Normalization and Variance Stabilization of Single-Cell RNA-Seq Data Using Regularized Negative Binomial Regression. Genome Biol (2019) 20(1):1–15. doi: 10.1186/s13059-019-1874-1
29. McInnes L, Healy J, Melville J. Umap: Uniform Manifold Approximation and Projection for Dimension Reduction. ArXiv (2018). Available at: https://en.wikipedia.org/wiki/ArXiv arXiv preprint arXiv:1802.03426.
30. Traag VA, Waltman L, Van Eck NJ. From Louvain to Leiden: Guaranteeing Well-Connected Communities. Sci Rep (2019) 9(1). doi: 10.1038/s41598-019-41695-z
31. Efremova M, Vento-Tormo M, Teichmann SA, Vento-Tormo R. CellPhoneDB: Inferring Cell-Cell Communication From Combined Expression of Multi-Subunit Ligand-Receptor Complexes. Nat Protoc (2020) 15(4):1484–506. doi: 10.1038/s41596-020-0292-x
32. Chen P, Zhao D, Li J, Liang X, Li J, Chang A, et al. Symbiotic Macrophage-Glioma Cell Interactions Reveal Synthetic Lethality in PTEN-Null Glioma. Cancer Cell (2019) 35(6):868–884 e6. doi: 10.1016/j.ccell.2019.05.003
33. Margold M, Seidel S, Kowalski T, Ladigan S, Baraniskin A, Schroers R, et al. The Value of Bone Marrow Biopsy for Staging of Patients With Primary CNS Lymphoma. Neuro Oncol (2021) 13:noab109. doi: 10.1093/neuonc/noab109
Keywords: primary central nervous system lymphoma (PCNSL), single cell sequencing, cellular heterogeneity, immune microenvironment, pathogenic and therapeutic, cell communication
Citation: Wei BY, Liu Z, Fan Y, Wang SW, Dong C, Rao W, Yang F, Cheng G and Zhang JN (2021) Analysis of Cellular Heterogeneity in Immune Microenvironment of Primary Central Nervous System Lymphoma by Single-Cell Sequencing. Front. Oncol. 11:683007. doi: 10.3389/fonc.2021.683007
Received: 19 March 2021; Accepted: 03 September 2021;
Published: 04 October 2021.
Edited by:
Jun Zhong, National Cancer Institute (NCI), United StatesReviewed by:
Zilu Zhou, University of Pennsylvania, United StatesJunil Kim, Soongsil University, South Korea
Copyright © 2021 Wei, Liu, Fan, Wang, Dong, Rao, Yang, Cheng and Zhang. This is an open-access article distributed under the terms of the Creative Commons Attribution License (CC BY). The use, distribution or reproduction in other forums is permitted, provided the original author(s) and the copyright owner(s) are credited and that the original publication in this journal is cited, in accordance with accepted academic practice. No use, distribution or reproduction is permitted which does not comply with these terms.
*Correspondence: Jianning Zhang, jnzhang2021@163.com; Gang Cheng, yjscg2003@126.com
†These authors have contributed equally to this work and share first authorship