- Department of Respiratory and Critical Care Medicine, NHC Key Laboratory of Pulmonary Diseases, Union Hospital, Tongji Medical College, Huazhong University of Science and Technology, Wuhan, China
Background: Suppressive tumor microenvironment is closely related to the progression and poor prognosis of lung adenocarcinoma (LUAD). Novel individual and universal immune-related biomarkers to predict the prognosis and immune landscape of LUAD patients are urgently needed. Two-gene pairing patterns could integrate and utilize various gene expression data.
Methods: The RNA-seq and relevant clinicopathological data of the LUAD project from the TCGA and well-known immune-related genes list from the ImmPort database were obtained. Co-expression analysis followed by an analysis of variance was performed to identify differentially expressed immune-related lncRNA (irlncRNA) (DEirlncRNA) between tumor and normal tissues. Two arbitrary DEirlncRNAs (DEirlncRNAs pair) in a tumor sample underwent pairwise comparison to generate a score (0 or 1). Next, Univariate analysis, Lasso regression and Multivariate analysis were used to screen survival-related DEirlncRNAs pairs and construct a prognostic model. The Acak information standard (AIC) values of the receiver operating characteristic (ROC) curve for 3 years are calculated to determine the cut-off point for high- or low-risk score. Finally, we evaluated the relationship between the risk score and overall survival, clinicopathological features, immune landscape, and chemotherapy efficacy.
Results: Data of 54 normal and 497 tumor samples of LUAD were enrolled. After a strict screening process, 15 survival-independent-related DEirlncRNA pairs were integrated to construct a prognostic model. The AUC value of the 3-year ROC curve was 0.828. Kaplan–Meier analysis showed that patients with low risk lived longer than patients with high risk (p <0.001). Univariate and Multivariate Cox analysis suggested that the risk score was an independent factor of survival. The risk score was negatively associated with most tumor-infiltrating immune cells, immune score, and microenvironment scores. The low-risk group was correlated with increased expression of ICOS. The high-risk group had a connection with lower half inhibitory centration (IC50) of most chemotherapy drugs (e.g., etoposide, paclitaxel, vinorelbine, gemcitabine, and docetaxel) and targeted medicine—erlotinib, but with higher IC50 of methotrexate.
Conclusion: The established irlncRNA pairs-based model is a promising prognostic signature for LUAD patients. Furthermore, the prognostic signature has great potential in the evaluation of tumor immune landscape and guiding individualized treatment regimens.
Introduction
Globally, lung cancer remains the main cause of cancer death (1). Lung adenocarcinoma (LUAD), as the most common pathological type of lung cancer, has brought great burden to the health care systems (2). The prognosis for LUAD is generally poor in virtue of the characteristics of early metastasis. Chemotherapy and molecular targeted therapy are already conventional treatments for LUAD (3). Suppressive tumor microenvironment is closely related to the progression and poor prognosis of lung cancer (4). Immunotherapy targeted to relevant immunological mechanisms especially immune checkpoint inhibitor treatment has brought promising future for cure of LUAD patients (3, 5, 6). However, individual immune heterogeneity, namely, various immune cell compositions and immunoregulatory molecules, are related to different responses to immunotherapy (6, 7), chemotherapy (8), and targeted therapy (9). It is necessary and theoretically feasible to find immune-related biomarkers that can predict the prognosis and treatment sensitivity of LUAD patients.
Long noncoding RNAs (lncRNAs, ncRNAs that are >200 nt in length), a crucial class of pervasive genes playing a variety of cellular and physiologic functions, are known to be related to tumorigenesis and metastasis (10). LncRNAs, especially immune-related lncRNAs, have been indicated to possess great potential as novel biomarkers for the prognosis and treatment effect of lung cancer (11–15). However, the prognostic signatures in these studies were found based on the exact expression level of immune-related lncRNAs. Of these methods, the process of the normalization of lncRNAs expression from different platforms made data processing complicated and might affect the accuracy of the prediction model.
An inspiring research developed and validated an individualized immune prognostic signature for lung cancer using a strategy of immune-related gene (irgene) pairing in each sample. This gene-pairing strategy left out the normalization of data from diverse platforms (16).
In the present study, we retrieved irlncRNA data of LUAD patients from the Cancer Genome Atlas (TCGA) to establish and verify an individualized and multiple-data applicable prediction model for LUAD by applying the irlncRNA-pairing strategy. Furthermore, we investigated the value of the prognostic model in evaluating the immune landscape and prediction of effects of chemotherapy and targeted therapy.
Methods
Data Processing and Extraction of Differentially Expressed Immune-Related lncRNAs
The RNA-seq and matched clinicopathologic data of LUAD were downloaded from the TCGA database. The mRNAs and lncRNAs were distinguished by annotation files from the Ensembl databank for subsequent analysis. The list of confirmed immune-related genes (irgenes) was downloaded from the ImmPort databank. Immune-related lncRNA (IrlncRNAs) were acquired through co-expression analysis between irgenes and lncRNAs with correlation coefficients >0.4 and the p-value <0.001 as thresholds. The “limma” R package was used to discriminate the differential expressed irlncRNAs (DEirlncRNA) between tumor and normal tissues with the thresholds set as log fold change (logFC) >1 and false discovery rate (FDR) <0.05. Patients lacking clinicopathological data and those with survival data <30 days (who may die of other diseases rather than LUAD) were excluded.
Pairing DEirlncRNA
The DEirlncRNAs in each tumor sample were randomly paired. The score of DEirlncRNAs pair (DEirlncRNA 1/DEirlncRNA 2) was assigned to be 1 if the expression of DEirlncRNA 1 was more than DEirlncRNA 2; otherwise, the DEirlncRNAs pair score was 0 (16). Therefore, we constructed a 0-or-1 matrix. DEirlncRNAs pair score with constant values (0 or 1 over 80% frequency or under 20%) was considered not associated with prognosis because a certain rank was necessary for the survival of the discriminating patient (17). Accordingly, only if the frequency of value (0 or 1) of a DEirlncRNA pair score was between 20 and 80% of the total sample, it would be regarded as candidate for prognostic model construction.
Construction and Validation of a Prognostic Model
For screening alternative DEirlncRNA pairs to construct a prognostic model, we first performed a Univariate Cox proportional hazard analysis to screen survival-related DEirlncRNA pairs. Then, a 10-fold cross-validation Lasso regression (18) was performed to further filter meaningful DEirlncRNA pairs (p <0.05 as significance threshold), which were then selected for Multivariate Cox proportional hazard analysis for the construction of the model with the risk-formula: Risk score = score of DEirlncRNA pairs 1 × β1 DEirlncRNA pairs 1 + score of DEirlncRNA pairs 2 × β2 DEirlncRNA pairs 2 +……+ score of DEirlncRNA pairs n × βn DEirlncRNA pairs n. We drew the ROC curves of the model for 1, 3, and 5 years and evaluated the Acak information criterion (AIC) values of each point of the 3-year ROC curve to determine the cut-off point for high- or low-risk score. Kaplan–Meier analysis showing the difference of survival between the high-risk group and low-risk group was performed to verify this critical value. The relation of risk score values to survival status was also explored. The R packages performed in the above steps included glmnet, survival, survminer, survivalROC, pbapply, and pHeatmap.
For the validation of the clinical significance of the constructed model, chi-square test was used to explore the relationship between risk score and clinical data. The band diagram was plotted for visualization (p <0.001 = ***, p <0.01 = **, and p <0.05 = *). We performed Wilcoxon signed-rank test to show the risk score differences among various groups divided by clinical characteristics, which were shown by the box diagram. To confirm whether the risk score can be used as an independent risk-stratification factor, Univariate and Multivariate Cox regression analyses were conducted between the risk score and clinical features. Forest maps were utilized to display the results. These procedures were utilized by the R packages, namely, Survival and pHeatmap.
Evaluation of Tumor Immune Microenvironment Using Prognostic Signature
First, to investigate the association between the risk score and immune cells in the tumor microenvironment, we estimated the infiltrating-immune cells among the samples by acknowledged algorithms, namely, CIBERSORT (19), CIBERSORT-ABS (20), TIMER (21), xCELL (22, 23), MCPcounter (24), QUANTISEQ (25), and EPIC (26). Wilcoxon signed-rank test was used to inspect the differences of the infiltrating immune cells between high- and low-risk groups, of which the results were shown in the box chart. The Spearman correlation analysis between the risk score and the immune cells was performed and the correlation coefficients were shown in a lollipop diagram (p <0.05 as significance threshold). The operation was utilized by the R ggplot 2 package. Second, Wilcoxon signed-rank test was applied to study the differential expression of immune checkpoint-related gene between the high- and low-risk groups. Package “ggstatsplot” was performed and the violin plot was visualized.
Assessment of the Value of the Signature in Predicting Drug Susceptibility
To evaluate the value of the signature in the LUAD treatment efficacy prediction, we counted the IC50 of common chemotherapy and molecular targeted drugs for each sample using pRRophetic (an R package for prediction of clinical chemotherapeutic response from tumor gene expression levels) (27). Antitumor medicines such as etoposide, paclitaxel, vinorelbine, docetaxel, methotrexate, erlotinib, gefitinib, crizotinib, and alectinib are recommended for LUAD treatment in guidelines (3). The difference in the IC50 between the high- and low-risk groups was compared by Wilcoxon signed-rank test and the results are shown as box drawings using a “ggplot2” R package.
Results
Screening of DEirlncRNAs
Figure 1 presents the flow chart of this research. First, we obtained the RNA-seq data of LUAD from the TCGA database, namely, 54 normal and 497 tumor samples. Next, the data were divided into lncRNA and mRNA, and the known irgenes profiles were retrieved from the ImmPort database, then the irlncRNAs were obtained by performing co-expression analysis between irgenes and lncRNAs. Finally, we identified 1,209 irlncRNAs (shown in Table S1) and 160 DEirlncRNAs (Figure 2A and Table S2), among which, 136 were raised, and 24 were reduced in tumor comparing to normal tissues. (Figure 2B).
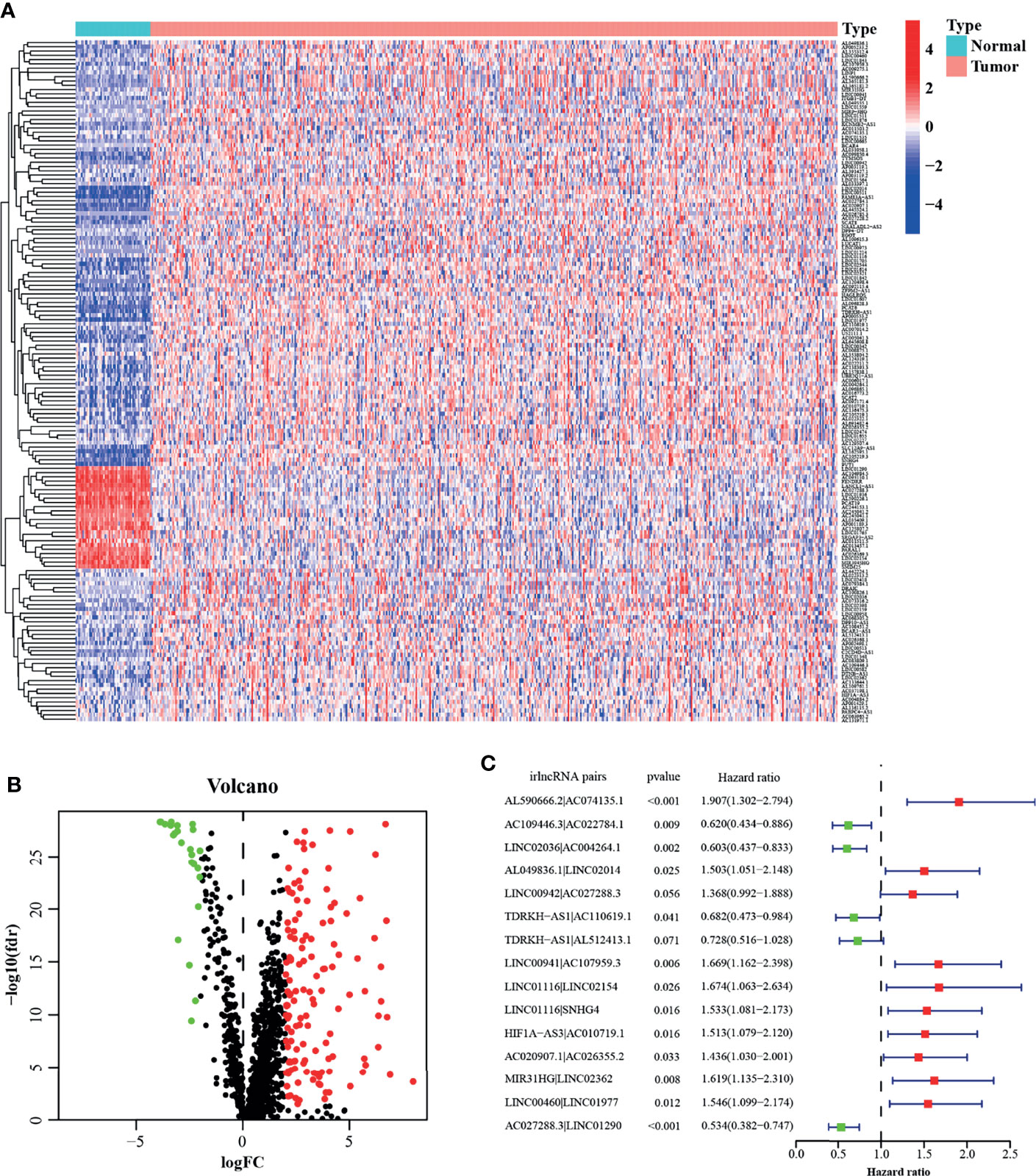
Figure 2 Construction of a prognostic model using DEirlncRNA Pairs. The heatmap (A) and volcano plot (B) of identified differentially expressed immune-related lncRNAs (DEirlncRNAs). (C) A forest map showed 12 DEirlncRNA pairs identified by Multivariate Cox proportional hazard regression in the stepwise method.
Construction and Validation of Prognostic Model Based on DEirlncRNAs Pairs
A total of 457 cancer cases with survival time >30 days and matched clinicopathological features (except for M stage for 26.5% of them were missing or unknown) from the TCGA was included for the following analysis. Using an iteration loop and a 0-or-1 matrix screening among 160 DEirlncRNAs, 9,931 valid DEirlncRNA pairs were identified. After a Univariate Cox proportional hazard analysis, 260 DEirlncRNA pairs were extracted. Then a modified Lasso regression analysis was utilized to prevent overfitting and screened to 45 DEirlncRNA pairs, followed by a Multivariate Cox proportional hazards analysis, and 15 of them were incorporated into the prognostic model based on step-by-step approach. (Figure 2C). We drew the ROC curves of the model for 1, 3, and 5 years with all AUC values more than 0.77 and the greatest AUC value—0.828 for 3 years (Figure 3A). Additionally, we compared the ROC curves of the model and other clinicopathologic factors for 3 years, which showed the risk score possessed the maximum AUC value (Figure 3B). These results validated the optimality of the signature. We calculated the AIC value to identify the cut-off point of the ROC curve for 3 years. (Figure 3C). Included 457 cases were classified into 259 high-risk and 198 low-risk cases based on the above cut-off point. Figures 3D, E showed their risk score and survival condition. These results manifested better clinical outcome of low-risk patients than that of high-risk. A Kaplan–Meier analysis showed that patients with low risk lived longer than patients with high risk (p <0.001) (Figure 3F).
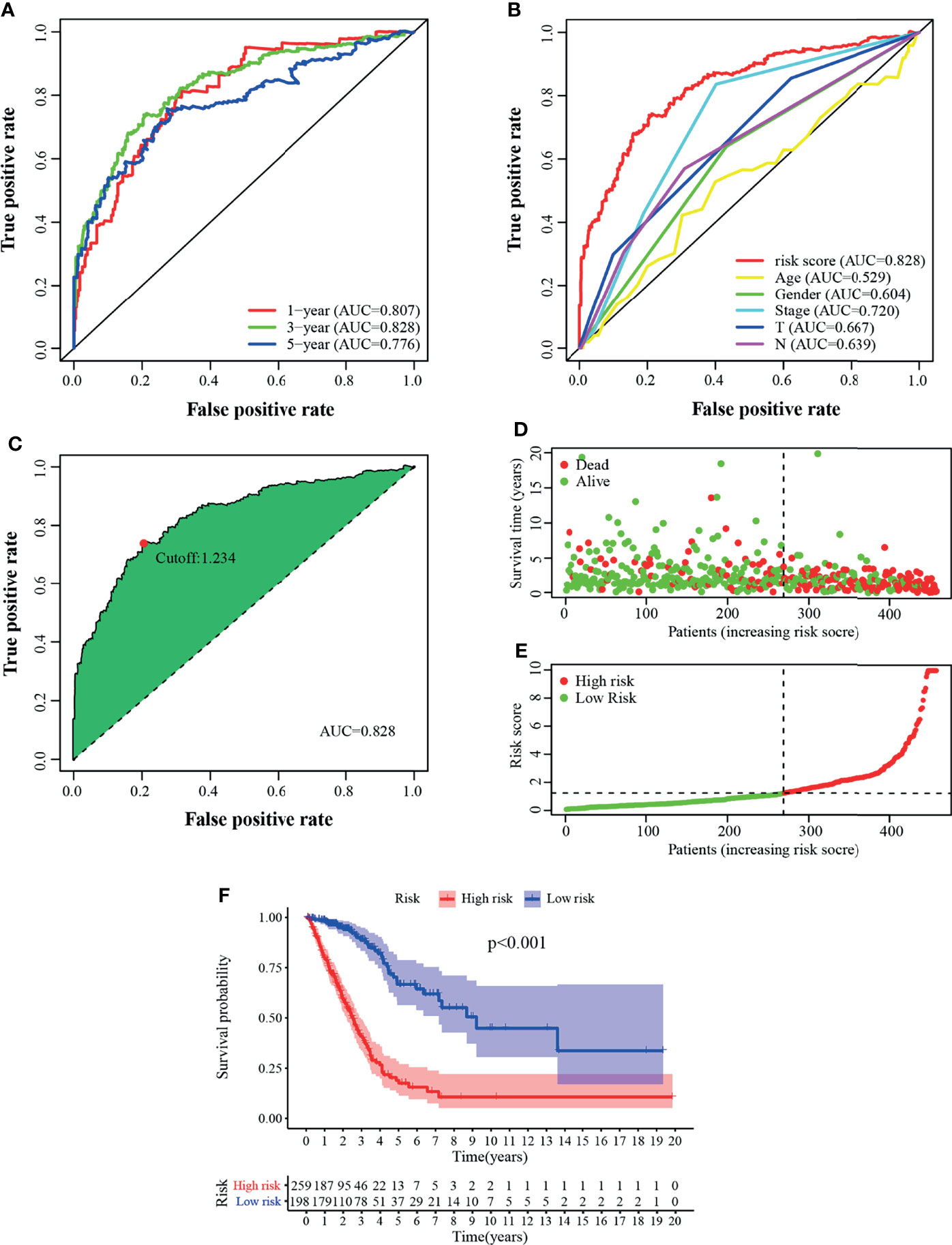
Figure 3 Validation of the prognostic model. (A) The 1-, 3-, and 5-year ROC of the model suggested that all AUC values were over 0.77. (B) A comparison of ROC curves of 3-year with other common clinical factors showed the superiority of the riskscore. (C) Riskscore for 457 patients with LUAD; the maximum inflection point is the cut-off point obtained by the AIC. Risk scores (D) and survival outcome (E) of each case are shown. (F) Patients in the low-risk group experienced a longer survival time tested by the Kaplan–Meier test.
Correlation Between Risk Score and the Clinical Variables
The strip illustration (Figure 4A) and scatter drawing showed that the T stage (Figure 4B), N stage (Figure 4C), and clinical stage (Figure 4D) were significantly related to the risk score. Next, Univariate Cox regression analysis indicated that the clinical stage (p <0.001, HR = 1.608, 95% CI [1.390–1.860]), T stage (p <0.001, HR = 1.528, 95% CI [1.270–1.840]), N stage (p <0.001, HR = 1.643, 95% CI [1.378–1.958]), and risk score (p <0.001, HR = 1.215, 95% CI [1.178–1.253]) were associated with overall survival (Figure 4E), however, only clinical stage (p = 0.004, HR = 1.390, 95% CI [1.112–1.738]) and risk score (p <0.001, HR = 1.205, 95% CI [1.166–1.246]) illustrated independent correlation by Multivariate Cox regression analysis (Figure 4F). Overall, the risk score was an independent factor associated with survival of the patients.
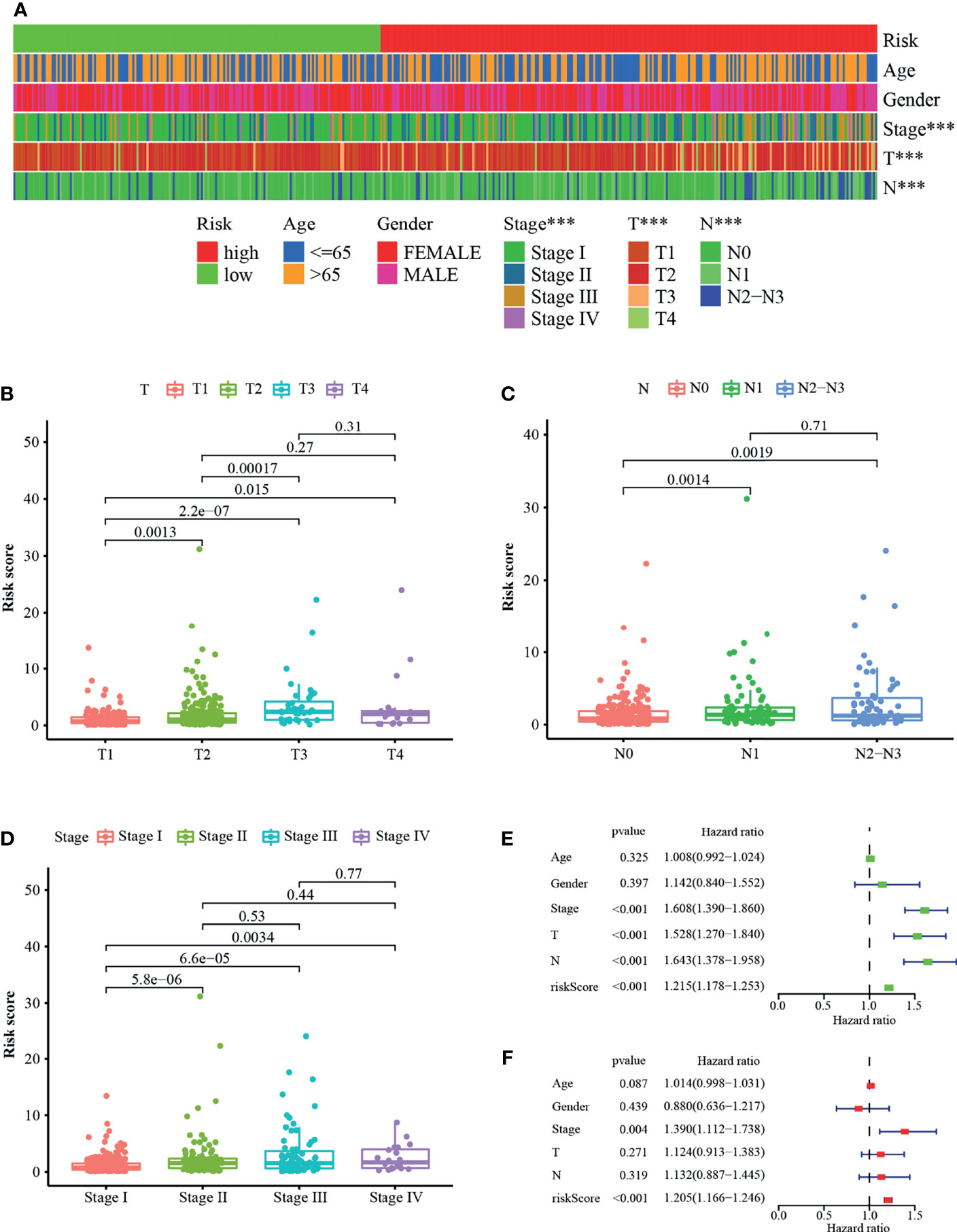
Figure 4 Correlation between riskscore and the clinical variables. (A) A strip illustration and scatter drawing showed that the (B) T stage, (C) N stage and (D) clinical stage were significantly related to the riskscore. (E) Univariate Cox hazard ratio analysis and (F) Multivariate Cox regression analysis of riskscore and other common clinical factors. ***p < 0.001.
Relevance of the Prognostic Signature to Immune Landscape
Studies have shown that the suppressive tumor immune microenvironment is a hallmark of tumors (including lung cancer). A breakthrough has been made in immunotherapy (cell adoptive therapy and immune checkpoint blocking therapy). We subsequently explored whether the prognostic signature based on irlncRNAs pairs had a relation to the tumor immune landscape. Results showed that most immune cells in tumor microenvironment including CD8+ T cells, CD4+ T cells, monocytes, B cells, dendritic cells, and NKT cells were negatively associated with the high-risk scores, whereas fibroblasts hold distinct results in different algorithms (Figures 5A–I, 6A and Tables S3, S4). Tumor environment score, immune, and stromal score (Figures 5J–L) calculated by xCELL algorithm were higher in the low-risk group than the high-risk group. Besides, we explored whether the prognostic signature was correlated with immune checkpoint-related gene expression, and found that the high-risk group showed a higher level of ICOS (p <0.01, Figure 6B), although CTLA4, CD274, and PDCD1 (Figures 6C–E) showed no significant association.
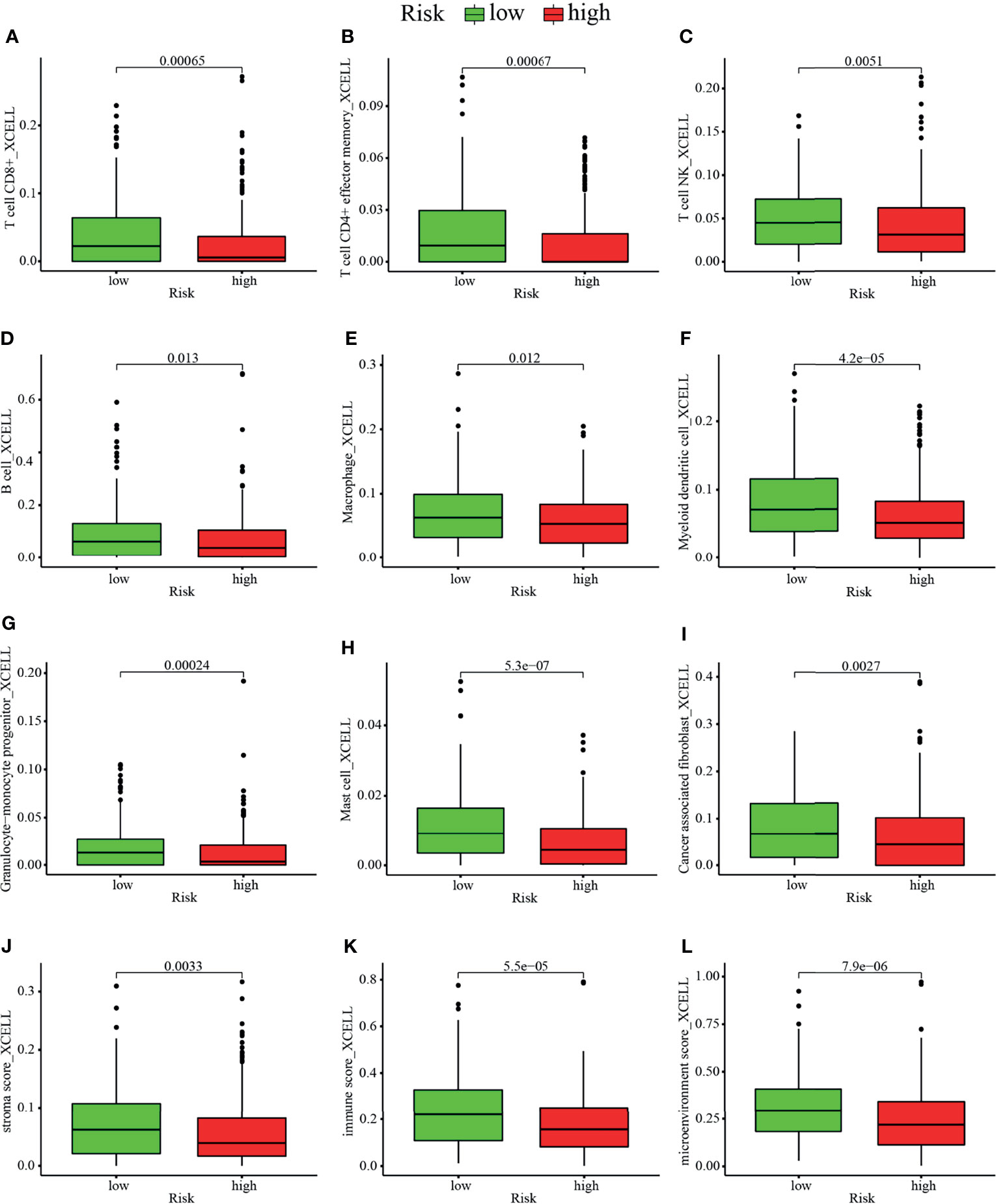
Figure 5 Estimation of tumor-infiltrating cells by the prognostic model. Comparison of composition of (A–H) immune cells, namely, (A) CD8+ T cell, (B) CD4+ effector memory T cell, (C) NKT cells, (D) B cell, (E) macrophage, (F) myeloid dendritic cell, (G) granulocyte–monocyte progenitor cell, and (H) mast cell and (I) cancer associated fibroblast cell between the high risk and low-risk group. (J–L) Comparison of (J) stromal score, (K) immune score, and (L) microenvironment score between the high risk and low-risk groups.
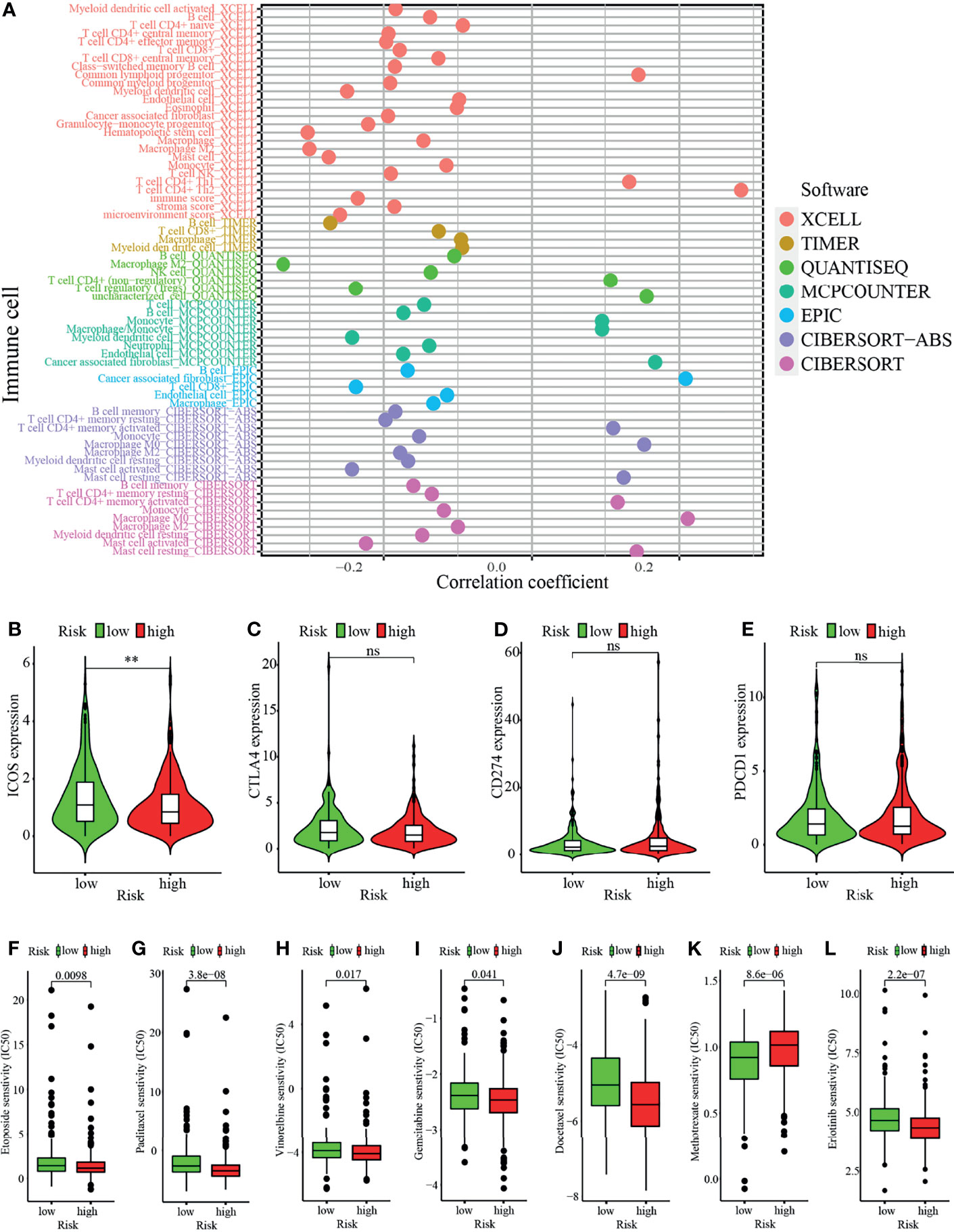
Figure 6 Analysis of immune landscape between the high-risk and low-risk groups. (A) Overview of association among riskscore and immune cells and stromal cells shown by Spearman correlation analysis. (B–E) Comparison of expression level of (B) ICOS, (C) CTLA4, (D) CD274, and (E) PDCD1 levels. (F–L) Prediction of drug sensitivity (IC50) for chemotherapeutics such as (F) etoposide, (G) paclitaxel (H) vinorelbine, (I) gemcitabine, (J) docetaxel, (K) methotrexate, and (L) targeted therapy—erlotinib. **p < 0.01; ns, not significant.
Correlation Analysis Between the Prognostic Signature and Chemotherapeutics
In addition to immune checkpoint blockades therapy, we tried to explore whether there were associations between risk score and the sensibility of LUAD patients to the common chemotherapeutics and molecular targeted therapy. Results showed that risk score was negatively related to IC50 of chemotherapy drugs such as etoposide (p = 0.0098), paclitaxel (p <0.0001), vinorelbine (p = 0.017), gemcitabine (p = 0.041), and docetaxel (p <0.0001), whereas it was positively associated with IC50 of methotrexate (p <0.0001), which suggested that the model possessed great potential in predicting chemotherapeutic sensitivity (Figures 6F–K). In addition, the risk score was suggested to be negatively associated with the IC50 of erlotinib (p <0.0001) (Figure 6L) though there was no significant association between other targeted drugs (such as gefitinib or afatinib) (data not shown).
Discussion
Lung cancer is still the most afflicting cancer in the world and the 5-year survival rate of lung cancer is only 10–20% in many countries (1). Comprehensive screening with low-dose computed tomography (CT) and advances in therapeutic strategies such as targeted therapy and immunotherapy had improved the survival of lung cancer patients. However, individual heterogeneity (e.g., immune heterogeneity) of patients results in differential responses to immunotherapy (7) and chemotherapy (8) and targeted therapy (9). Discovering immune-related biomarkers that can predict the prognosis and treatment sensitivity of LUAD patients for adjusting the optimum treatment regimens in advance was urgently needed. Recent studies have shown immune-associated lncRNAs signature has a prognostic (overall survival) value (11–14) or immunotherapeutic effect (15) for LUAD patients. However, these prognostic signatures are restricted by the normalization processing of lncRNA expression data from different platforms. In this study, we took a strategy using irlncRNA pairs, inspired by the research of Li (16), to establish and validate an individual and reliable model to predict prognosis and provide references for selection of therapeutic drugs of patients with LUAD. The founding of the prognostic model in our study is the comparative ranking of irlncRNA expression in a tumor sample, which can utilize irlncRNA expression data from various sources such as microarray, RNA-Seq, or quantitative PCR.
Prognostic signatures associated with the tumor immune landscape possess great potential in recognizing new molecular biomarkers and ameliorating patient management (28). Our prognostic model based on 15 irlncRNA pairs showed excellent performance in distinguishing high and low-risk groups. Moreover, it was an independent predictive factor for the prognosis of LUAD patients. A total of 12 of 27 irlncRNAs (15 irlncRNA pairs) in the model have been identified as biomarkers or been found to take a crucial part in the pathogenesis of cancer or other diseases. AC022784.1 (12), TDRKH-AS1 (29), and LINC00941 (29) had been reported to be associated with the prognosis of LUAD. LINC00942, LINC01116, SNHG4, MIR31HG, and LINC00460 had been known to be associated with tumor development and progression and drug resistance in various cancers including lung cancer (30–39). AC107959.3 (40) and LINC02154 (41) were reported to be associated with the prognosis of hepatocellular carcinoma and laryngeal cancer respectively. LINC01977 (42) and HIF1A-AS3 (43) might be related to the pathogenesis of thyroid carcinoma multiple sclerosis respectively, whereas other 15 irlncRNAs were revealed for the first time. Whether these new irlncRNAs are novel biomarkers and play crucial roles in LUAD progress needs further research.
The composition of tumor-infiltrating immune cells and immune checkpoints have related to the responses to immune checkpoint inhibitors (44, 45). Lung cancer patients with higher PD-L1 expression possessed a better effect of pembrolizumab therapy than those with lower expression (6). In our study, the low-risk group possessed a higher composition of most immune cells, namely, CD4+ T cells, CD8+ T cells, B cells, and dendritic cells, which was consistent with previous studies (45–47). The low-risk group had a higher microenvironment score, immune and stromal score indicated that they possessed lower tumor purity and superior responses from immunotherapy (48). The low-risk group had a higher level of ICOS expression though there was no significant relation between riskscore and expression of CTLA4, CD274 or PDCD1. These results suggested that patients of low risk might have superior responses to immunotherapy such as immune checkpoint blockade and cancer vaccines. Nevertheless, the high-risk group in our study was more sensitive to chemotherapeutics such as etoposide, paclitaxel, vinorelbine, gemcitabine and docetaxel and targeted therapeutic drug-erlotinib. Therefore, the prognostic signature in our study has great potential in guiding treatment strategies for LUAD in clinical practice.
There are several limitations to our study. First, this was a retrospective study. Second, the dataset was simply downloaded from TCGA and further experimental data is needed to support these findings. Third, we had not done external validation for the constructed model to improve its applicability, which is restricted to the potential selection bias of patients. We utilized relative ranking of irlncRNA expression values within each sample to minimize errors caused by differential expression and diverse detection platforms, and the individualized prognostic signature possessed certain applicability for its ability to integrating various data sources from microarray, RNA-Seq or real-time PCR. Overall, we supposed that the prognostic model in this study was acceptable. Furthermore, we are planning to collect clinical samples for further verification.
In conclusion, the proposed irlncRNA pair-based signature has promising value in the prognostic prediction of LUAD. Furthermore, this prognostic model has great potential in the evaluation of tumor immune microenvironment and guiding individualized treatment regimens. Prospective evidence to further assess its accuracy and applicability are necessary in the future.
Data Availability Statement
Publicly available datasets were analyzed in this study. This data can be found here: TCGA database, ImmPort database.
Author Contributions
YJ designed the study. ZY download data from the TCGA and ImmPort database and did all the data analysis. MZ and TL drew all the figures. The tables were produced by JF and JD. The manuscript was drafted by ZY, MZ, TL, and JX. YJ supervised the overall workflow and critically revised the manuscript. YJ is the guarantor of this paper, taking responsibility for the integrity of the work as a whole, from inception to published article. All authors contributed to the article and approved the submitted version.
Funding
This work was supported by the National Major Science and Technology Projects of China (CN):2019ZX09301001, and the Ministry of Science and Technology of the People’s Republic of China (CN):2020YFC0844300. The research sponsors did not participate in the study design, data collection, analysis and interpretation, and they were not involved in the writing of the manuscript and the decision to submit the manuscript for publication.
Conflict of Interest
The authors declare that the research was conducted in the absence of any commercial or financial relationships that could be construed as a potential conflict of interest.
Publisher’s Note
All claims expressed in this article are solely those of the authors and do not necessarily represent those of their affiliated organizations, or those of the publisher, the editors and the reviewers. Any product that may be evaluated in this article, or claim that may be made by its manufacturer, is not guaranteed or endorsed by the publisher.
Acknowledgments
ZY wants to thank the inspiration, accompany and care from Mengqin Yuan. You are the silver shining behind the clouds in my life.
Supplementary Material
The Supplementary Material for this article can be found online at: https://www.frontiersin.org/articles/10.3389/fonc.2021.673567/full#supplementary-material
References
1. Sung H, Ferlay J, Siegel RL, Laversanne M, Soerjomataram I, Jemal A, et al. Global Cancer Statistics 2020: GLOBOCAN Estimates of Incidence and Mortality Worldwide for 36 Cancers in 185 Countries. CA Cancer J Clin (2021) 71(3):209–49. doi: 10.3322/caac.21660
2. Siegel RL, Miller KD, Fuchs HE, Jemal A. Cancer Statistics, 2021. CA Cancer J Clin (2021) 71(1):7–33. doi: 10.3322/caac.21654
3. Network National Comprehensive Cancer. Nccn Guidelines Version 2.2021 - Non-Small Cell Lung Cancer 2020. Available at: https://www.nccn.org/professionals/physician_gls/default_nojava.aspx.
4. Carbone DP, Gandara DR, Antonia SJ, Zielinski C, Paz-Ares L. Non-Small-Cell Lung Cancer: Role of the Immune System and Potential for Immunotherapy. J Thorac Oncol (2015) 10(7):974–84. doi: 10.1097/JTO.0000000000000551
5. Topalian SL, Hodi FS, Brahmer JR, Gettinger SN, Smith DC, McDermott DF, et al. Safety, Activity, and Immune Correlates of Anti-PD-1 Antibody in Cancer. N Engl J Med (2012) 366(26):2443–54. doi: 10.1056/NEJMoa1200690
6. Garon EB, Hellmann MD, Rizvi NA, Carcereny E, Leighl NB, Ahn MJ, et al. Five-Year Overall Survival for Patients With Advanced Non‒Small-Cell Lung Cancer Treated With Pembrolizumab: Results From the Phase I KEYNOTE-001 Study. J Clin Oncol (2019) 37(28):2518–27. doi: 10.1200/JCO.19.00934
7. Jia Q, Wu W, Wang Y, Alexander PB, Sun C, Gong Z, et al. Local Mutational Diversity Drives Intratumoral Immune Heterogeneity in non-Small Cell Lung Cancer. Nat Commun (2018) 9(1):5361. doi: 10.1038/s41467-018-07767-w
8. Zhou Y, Chen C, Zhang X, Fu S, Xue C, Ma Y, et al. Immune-Checkpoint Inhibitor Plus Chemotherapy Versus Conventional Chemotherapy for First-Line Treatment in Advanced Non-Small Cell Lung Carcinoma: A Systematic Review and Meta-Analysis. J Immunother Cancer (2018) 6(1):155. doi: 10.1186/s40425-018-0477-9
9. Hastings K, Yu HA, Wei W, Sanchez-Vega F, DeVeaux M, Choi J, et al. EGFR Mutation Subtypes and Response to Immune Checkpoint Blockade Treatment in Non-Small-Cell Lung Cancer. Ann Oncol (2019) 30(8):1311–20. doi: 10.1093/annonc/mdz141
10. Bhan A, Soleimani M, Mandal SS. Long Noncoding RNA and Cancer: A New Paradigm. Cancer Res (2017) 77(15):3965–81. doi: 10.1158/0008-5472.CAN-16-2634
11. Jin D, Song Y, Chen Y, Zhang P. Identification of a Seven-Lncrna Immune Risk Signature and Construction of a Predictive Nomogram for Lung Adenocarcinoma. BioMed Res Int (2020) 2020:7929132. doi: 10.1155/2020/7929132
12. Li JP, Li R, Liu X, Huo C, Liu TT, Yao J, et al. A Seven Immune-Related Lncrnas Model to Increase the Predicted Value of Lung Adenocarcinoma. Front Oncol (2020) 10:560779. doi: 10.3389/fonc.2020.560779
13. Miao H, Chen D, Li R, Hu J, Chen Y, Xu C, et al. Identification of an Immune-Related Six-Long Noncoding RNA Signature as a Novel Prognosis Biomarker for Adenocarcinoma of Lung. Biosci Rep (2021) 41(1):BSR20202444. doi: 10.1042/BSR20202444
14. Li J, Li X, Zhang C, Zhang C, Wang H. A Signature of Tumor Immune Microenvironment Genes Associated With the Prognosis of Non−Small Cell Lung Cancer. Oncol Rep (2020) 43(3):795–806. doi: 10.3892/or.2020.7464
15. Sun J, Zhang Z, Bao S, Yan C, Hou P, Wu N, et al. Identification of Tumor Immune Infiltration-Associated Lncrnas for Improving Prognosis and Immunotherapy Response of Patients With Non-Small Cell Lung Cancer. J Immunother Cancer (2020) 8(1):e000110. doi: 10.1136/jitc-2019-000110
16. Li B, Cui Y, Diehn M, Li R. Development and Validation of an Individualized Immune Prognostic Signature in Early-Stage Nonsquamous Non-Small Cell Lung Cancer. JAMA Oncol (2017) 3(11):1529–37. doi: 10.1001/jamaoncol.2017.1609
17. Kim S, Lin CW, Tseng GC. Metaktsp: A Meta-Analytic Top Scoring Pair Method for Robust Cross-Study Validation of Omics Prediction Analysis. Bioinformatics (2016) 32(13):1966–73. doi: 10.1093/bioinformatics/btw115
18. Hong W, Liang L, Gu Y, Qi Z, Qiu H, Yang X, et al. Immune-Related Lncrna to Construct Novel Signature and Predict the Immune Landscape of Human Hepatocellular Carcinoma. Mol Ther Nucleic Acids (2020) 22:937–47. doi: 10.1016/j.omtn.2020.10.002
19. Chen B, Khodadoust MS, Liu CL, Newman AM, Alizadeh AA. Profiling Tumor Infiltrating Immune Cells With CIBERSORT. Methods Mol Biol (2018) 1711:243–59. doi: 10.1007/978-1-4939-7493-1_12
20. Tamminga M, Hiltermann TJN, Schuuring E, Timens W, Fehrmann RS, Groen HJ. Immune Microenvironment Composition in Non-Small Cell Lung Cancer and its Association With Survival. Clin Trans Immunol (2020) 9(6):e1142. doi: 10.1002/cti2.1142
21. Li T, Fu J, Zeng Z, Cohen D, Li J, Chen Q, et al. TIMER2.0 for Analysis of Tumor-Infiltrating Immune Cells. Nucleic Acids Res (2020) 48(W1):W509–14. doi: 10.1093/nar/gkaa407
22. Aran D, Hu Z, Butte AJ. Xcell: Digitally Portraying the Tissue Cellular Heterogeneity Landscape. Genome Biol (2017) 18(1):220. doi: 10.1186/s13059-017-1349-1
23. Aran D. Cell-Type Enrichment Analysis of Bulk Transcriptomes Using Xcell. Methods Mol Biol (2020) 2120:263–76. doi: 10.1007/978-1-0716-0327-7_19
24. Dienstmann R, Villacampa G, Sveen A, Mason MJ, Niedzwiecki D, Nesbakken A, et al. Relative Contribution of Clinicopathological Variables, Genomic Markers, Transcriptomic Subtyping and Microenvironment Features for Outcome Prediction in Stage II/III Colorectal Cancer. Ann Oncol (2019) 30(10):1622–9. doi: 10.1093/annonc/mdz287
25. Finotello F, Mayer C, Plattner C, Laschober G, Rieder D, Hackl H, et al. Molecular and Pharmacological Modulators of the Tumor Immune Contexture Revealed by Deconvolution of RNA-Seq Data. Genome Med (2019) 11(1):34. doi: 10.1186/s13073-019-0638-6
26. Racle J, de Jonge K, Baumgaertner P, Speiser DE, Gfeller D. Simultaneous Enumeration of Cancer and Immune Cell Types From Bulk Tumor Gene Expression Data. eLife (2017) 6:e26476. doi: 10.7554/eLife.26476
27. Geeleher P, Cox N, Huang RS. Prrophetic: An R Package for Prediction of Clinical Chemotherapeutic Response From Tumor Gene Expression Levels. PloS One (2014) 9(9):e107468. doi: 10.1371/journal.pone.0107468
28. Vargas AJ, Harris CC. Biomarker Development in the Precision Medicine Era: Lung Cancer as a Case Study. Nat Rev Cancer (2016) 16(8):525–37. doi: 10.1038/nrc.2016.56
29. Wang L, Zhao H, Xu Y, Li J, Deng C, Deng Y, et al. Systematic Identification of Lincrna-Based Prognostic Biomarkers by Integrating Lincrna Expression and Copy Number Variation in Lung Adenocarcinoma. Int J Cancer (2019) 144(7):1723–34. doi: 10.1002/ijc.31865
30. Wang H, Lu B, Ren S, Wu F, Wang X, Yan C, et al. Long Noncoding RNA LINC01116 Contributes to Gefitinib Resistance in Non-Small Cell Lung Cancer Through Regulating IFI44. Mol Ther Nucleic Acids (2020) 19:218–27. doi: 10.1016/j.omtn.2019.10.039
31. Xing H, Sun H, Du W. LINC01116 Accelerates Nasopharyngeal Carcinoma Progression Based on Its Enhancement on MYC Transcription Activity. Cancer Med (2020) 9(1):269–77. doi: 10.1002/cam4.2624
32. Bi C, Cui H, Fan H, Li L. Lncrna LINC01116 Promotes the Development of Colorectal Cancer by Targeting Mir-9-5p/STMN1. Onco Targets Ther (2020) 13:10547–58. doi: 10.2147/OTT.S253532
33. Zeng L, Lyu X, Yuan J, Wang W, Zhao N, Liu B, et al. Long Non-Coding RNA LINC01116 Is Overexpressed in Lung Adenocarcinoma and Promotes Tumor Proliferation and Metastasis. Am J Trans Res (2020) 12(8):4302–13.
34. Tang Y, Wu L, Zhao M, Zhao G, Mao S, Wang L, et al. Lncrna SNHG4 Promotes the Proliferation, Migration, Invasiveness, and Epithelial-Mesenchymal Transition of Lung Cancer Cells by Regulating Mir-98-5p. Biochem Cell Biol (2019) 97(6):767–76. doi: 10.1139/bcb-2019-0065
35. Wang ZY, Duan Y, Wang P. SP1-Mediated Upregulation of Lncrna SNHG4 Functions as a Cerna for Mir-377 to Facilitate Prostate Cancer Progression Through Regulation of ZIC5. J Cell Physiol (2020) 235(4):3916–27. doi: 10.1002/jcp.29285
36. Wang R, Ma Z, Feng L, Yang Y, Tan C, Shi Q, et al. Lncrna MIR31HG Targets HIF1A and P21 to Facilitate Head and Neck Cancer Cell Proliferation and Tumorigenesis by Promoting Cell-Cycle Progression. Mol Cancer (2018) 17(1):162. doi: 10.1186/s12943-018-0916-8
37. Qin J, Ning H, Zhou Y, Hu Y, Yang L, Huang R. Lncrna MIR31HG Overexpression Serves as Poor Prognostic Biomarker and Promotes Cells Proliferation in Lung Adenocarcinoma. BioMed Pharmacother (2018) 99:363–8. doi: 10.1016/j.biopha.2018.01.037
38. Wang HX, Kang LJ, Qin X, Xu J, Fei JW. LINC00460 Promotes Proliferation and Inhibits Apoptosis of Non-Small Cell Lung Cancer Cells Through Targeted Regulation of Mir-539. Eur Rev Med Pharmacol Sci (2020) 24(12):6752–8. doi: 10.26355/eurrev_202006_21663
39. Hu H, Xu H, Lu F, Zhang J, Xu L, Xu S, et al. Exploring the Effect of Differentially Expressed Long non-Coding Rnas Driven by Copy Number Variation on Competing Endogenous RNA Network by Mining Lung Adenocarcinoma Data. Front Cell Dev Biol (2020) 8:627436. doi: 10.3389/fcell.2020.627436
40. Huang ZL, Li W, Chen QF, Wu PH, Shen LJ. Eight Key Long non-Coding Rnas Predict Hepatitis Virus Positive Hepatocellular Carcinoma as Prognostic Targets. World J Gastrointest Oncol (2019) 11(11):983–97. doi: 10.4251/wjgo.v11.i11.983
41. Gong S, Xu M, Zhang Y, Shan Y, Zhang H. The Prognostic Signature and Potential Target Genes of Six Long non-Coding RNA in Laryngeal Squamous Cell Carcinoma. Front Genet (2020) 11:413. doi: 10.3389/fgene.2020.00413
42. Guo K, Chen L, Wang Y, Qian K, Zheng X, Sun W, et al. Long Noncoding RNA RP11-547D24.1 Regulates Proliferation and Migration in Papillary Thyroid Carcinoma: Identification and Validation of a Novel Long Noncoding RNA Through Integrated Analysis of TCGA Database. Cancer Med (2019) 8(6):3105–19. doi: 10.1002/cam4.2150
43. Rodríguez-Lorenzo S, Ferreira Francisco DM, Vos R, van Het Hof B, Rijnsburger M, Schroten H, et al. Altered Secretory and Neuroprotective Function of the Choroid Plexus in Progressive Multiple Sclerosis. Acta Neuropathologica Commun (2020) 8(1):35. doi: 10.1186/s40478-020-00903-y
44. Hamid O, Schmidt H, Nissan A, Ridolfi L, Aamdal S, Hansson J, et al. A Prospective Phase II Trial Exploring the Association Between Tumor Microenvironment Biomarkers and Clinical Activity of Ipilimumab in Advanced Melanoma. J Transl Med (2011) 9:204. doi: 10.1186/1479-5876-9-204
45. Budczies J, Kirchner M, Kluck K, Kazdal D, Glade J, Allgäuer M, et al. A Gene Expression Signature Associated With B Cells Predicts Benefit From Immune Checkpoint Blockade in Lung Adenocarcinoma. Oncoimmunology (2021) 10(1):1860586. doi: 10.1080/2162402X.2020.1860586
46. Hiraoka K, Miyamoto M, Cho Y, Suzuoki M, Oshikiri T, Nakakubo Y, et al. Concurrent Infiltration by CD8+ T Cells and CD4+ T Cells Is a Favourable Prognostic Factor in Non-Small-Cell Lung Carcinoma. Br J Cancer (2006) 94(2):275–80. doi: 10.1038/sj.bjc.6602934
47. Djenidi F, Adam J, Goubar A, Durgeau A, Meurice G, de Montpréville V, et al. CD8+CD103+ Tumor-Infiltrating Lymphocytes Are Tumor-Specific Tissue-Resident Memory T Cells and a Prognostic Factor for Survival in Lung Cancer Patients. J Immunol (2015) 194(7):3475–86. doi: 10.4049/jimmunol.1402711
Keywords: immune-related lncRNA pair, lung adenocarcinoma, signature, immune landscape, drug sensitivity
Citation: Yin Z, Zhou M, Liao T, Xu J, Fan J, Deng J and Jin Y (2022) Immune-Related lncRNA Pairs as Prognostic Signature and Immune-Landscape Predictor in Lung Adenocarcinoma. Front. Oncol. 11:673567. doi: 10.3389/fonc.2021.673567
Received: 27 February 2021; Accepted: 14 December 2021;
Published: 10 January 2022.
Edited by:
Luciano Mutti, Temple University, United StatesReviewed by:
Nikolaj Frost, Charité University Medicine Berlin, GermanyTongpeng Xu, Nanjing Medical University, China
Copyright © 2022 Yin, Zhou, Liao, Xu, Fan, Deng and Jin. This is an open-access article distributed under the terms of the Creative Commons Attribution License (CC BY). The use, distribution or reproduction in other forums is permitted, provided the original author(s) and the copyright owner(s) are credited and that the original publication in this journal is cited, in accordance with accepted academic practice. No use, distribution or reproduction is permitted which does not comply with these terms.
*Correspondence: Yang Jin, d2h1aGp5QDEyNi5jb20=
†These authors have contributed equally to this work