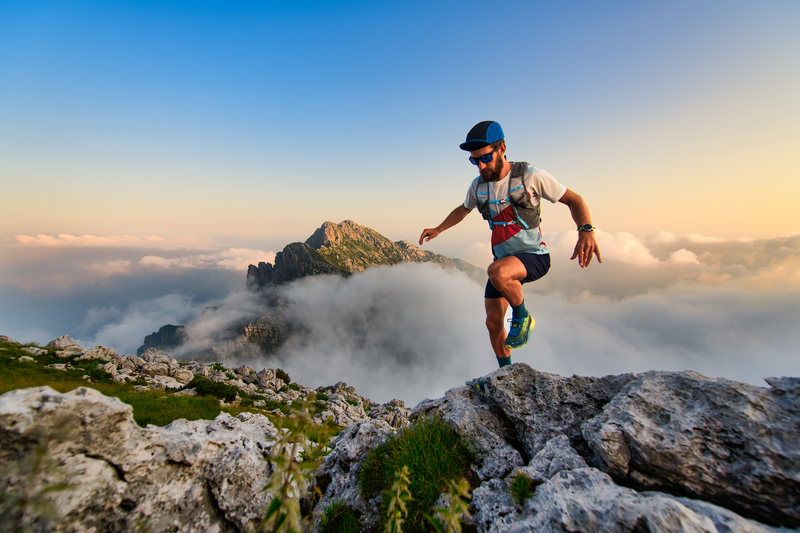
94% of researchers rate our articles as excellent or good
Learn more about the work of our research integrity team to safeguard the quality of each article we publish.
Find out more
SYSTEMATIC REVIEW article
Front. Oncol. , 24 June 2021
Sec. Thoracic Oncology
Volume 11 - 2021 | https://doi.org/10.3389/fonc.2021.670230
The lung immune prognostic index (LIPI) has been shown to be an important prognostic marker for various tumors. However, the prognostic value of LIPI among non-small cell lung cancer (NSCLC) patients treated with systemic therapy remains controversial. We aimed to evaluate survival status according to LIPI among NSCLC patients receiving different forms of systemic therapy at our institution. We also performed a meta-analysis of articles from PubMed and Embase to illustrate this question. For our cohort, we found that good LIPI was associated with better overall survival (OS) among 91 patients on immunotherapy, 329 patients on targeted therapy, and 570 patients on chemotherapy. For the meta-analysis, a total of eight studies with 8,721 patients were included. Pooled results showed that a higher LIPI (those with 1 or 2 factors) was associated with poor overall progression-free survival (PFS) (hazard ratio [HR], 1.57; 95% confidence interval [CI], 1.45−1.71) and OS (HR, 2.01; 95% CI, 1.75−2.31). Subgroup analyses showed that a higher LIPI was related to poor survival among patients prescribed different systemic therapies: immunotherapy (OS HR, 2.50; 95% CI, 1.99–3.13; PFS HR, 1.77; 95% CI, 1.56–2.01), chemotherapy (OS HR, 1.58; 95% CI, 1.34–1.86; PFS HR, 1.38; 95% CI, 1.23–1.55), and targeted therapy (OS HR; 2.15, 95% CI, 1.57–2.96; PFS HR, 1.60; 95% CI, 1.25–2.06). The study shows that the LIPI is a clinically significant prognostic factor for NSCLC patients receiving systemic therapy.
Systematic Review Registration: https://www.crd.york.ac.uk/PROSPERO/, identifier CRD420209009.
Lung cancer is a common malignancy with high morbidity and mortality (1). More than 80% of lung cancer patients present with non-small cell lung cancer (NSCLC), and the majority of NSCLC patients are initially ascertained at an advanced disease stage (2). In addition to traditional chemotherapy, precision medicine has brought new therapeutic strategies for the treatment of NSCLC, such as targeted therapy and immunotherapy; these treatment modalities have achieved significant survival benefits in NSCLC patients (3–5). However, a considerable proportion of patients do not benefit from systemic therapy, including chemotherapy, targeted therapy, and immunotherapy (6, 7). Thus, researchers have investigated effective prognostic factors of systemic therapies among NSCLC patients for the purpose of informing medical recommendations and taking effective medical decisions. There are many factors that can be used as an effective prognostic factor for immunotherapy, including smoking status, body mass index (BMI), a high-intensity statin regimen, and tumor cell expression of programmed death-1 (PD-L1) (8–11). In addition, inflammation and inflammatory processes have a substantial role in the development and progression of tumors, significantly affecting treatment effectiveness among patients with cancer (12). In recent years, researchers have developed various inflammation-related prognostic models, such as the neutrophil to lymphocyte ratio (NLR), the Glasgow prognostic score, the modified Glasgow prognostic score (GPS/mGPS), the tumor immune dysfunction and exclusion (TIDE) score, the Gustave Roussy immune (GRIm)-score, the lung immuno-oncology prognostic score (LIPS-3), and the lung immune prognostic index (LIPI). Wang et al. indicated that baseline NLR can be regarded as a prognostic biomarker for NSCLC patients taking systemic therapy (13), and a higher GPS has been shown to correlate with poor clinical outcomes (14). The TIDE dysfunction score, which is related to tumor immune evasion, is an effective indicator of immunotherapy response and resistance (15). The GRIm-score is based on patients’ NLR and albumin serum levels, and GRIm-score at 45 days since the patients’ first pembrolizumab injection has been shown to be an effective biomarker of clinical outcomes in NSCLC (16). LIPS-3 is a prognostic classification of patients receiving first-line PD-1 inhibitor for PD-L1 ≥ 50% NSCLC (17). Previous studies have indicated that LIPI can predict clinical outcome across many tumor types, such as renal cell carcinoma, melanoma, small-cell lung cancer, and especially NSCLC (18, 19).
The LIPI is defined according to a derived neutrophil to lymphocyte ratio (dNLR; absolute neutrophil count/[white blood cell (WBC) count − absolute neutrophil count]) greater than 3 as well as lactate dehydrogenase (LDH) levels greater than the upper limit of normal (ULN); LIPI is divided into three groups (good: 0 factors; intermediate: 1 factor; poor: 2 factors) (20), patients with 1 or 2 factors were considered as having a higher LIPI score compared to patients with 0 factors. The index is validated, easily derived from blood assays, and can be implemented to stratify patients based on their prognostic factor within clinical practice.
With regard to the LIPI, the number of relevant studies examining the prognostic value of this factor has increased since Mezquita et al. conducted a study exploring the prognostic value of the LIPI in NSCLC patients undergoing immunotherapy and chemotherapy (20). These authors investigated the associations between the LIPI and lung cancer survival outcomes among patients with different pathological types and according to different treatment regimens. However, the prognostic value of the LIPI in NSCLC patients remains a divisive issue. It was reported in a Japanese cohort that the LIPI was merely a valuable prognostic factor for specific subsets of NSCLC (21). Hence, this study aims to conduct a retrospective study at our center and perform a meta-analysis to provide the accurate prognostic value of the LIPI in NSCLC patients.
This retrospective study enrolled NSCLC patients presenting at the Department of Respiratory and Critical Care Medicine at the Affiliated Jinling Hospital who were treated with immune checkpoint inhibitors between May 2017 to April 2019. In total, 98 patients were enrolled. Of these, seven were excluded because of a lack of basal LDH measures that were necessary for the LIPI score calculation (Supplementary Figure 1).
Of the 1,510 patients initially diagnosed with advanced NSCLC at Jinling Hospital from January 2015 to December 2019, 899 were selected for our study. Exclusion criteria covered the following: insufficient data for compiling the LIPI (130 patients with no basal LDH measures and 16 patients with no basal dNLR measures), receiving other therapies such as anti-angiogenic therapy and radiotherapy, and loss to follow-up or incomplete follow-up. The final cohort included 329 patients receiving targeted therapy (36.6%) and 570 patients receiving chemotherapy (63.4%) (Supplementary Figure 2).
The LIPI is divided into three groups (good: 0 factors; intermediate: 1 factor; poor: 2 factors) (20), patients with 1 or 2 factors were considered as having a higher LIPI score compared to patients with 0 factors.
We examined progression-free survival (PFS) and overall survival (OS) to evaluate the clinical outcome of NSCLC patients. PFS is defined as the period from the start of anti-tumor treatment to the progression of disease (according to the Response Evaluation Criteria in Solid Tumors (RECIST) version 1.1). OS is delimited as the time from the beginning of the treatment to death for any reason. The study was conducted in accordance with the principles of the Declaration of Helsinki (as revised in 2013).
We conducted a systematic review of the PubMed and Embase databases, using the search terms “(((Lung Immune Prognostic Index[Text Word]) OR (LIPI[Text Word])) OR (LIPI score[Text Word])) AND (((((((((((((((((((Neoplasia[Title/Abstract]) OR (Neoplasias[Title/Abstract])) OR (Neoplasm[Title/Abstract])) OR (Tumors[Title/Abstract])) OR (Tumor[Title/Abstract])) OR (Cancer[Title/Abstract])) OR (Cancers[Title/Abstract])) OR (Malignancy[Title/Abstract])) OR (Malignancies[Title/Abstract])) OR (Malignant Neoplasms[Title/Abstract])) OR (Malignant Neoplasm[Title/Abstract])) OR (Neoplasm, Malignant[Title/Abstract])) OR (Neoplasms, Malignant[Title/Abstract])) OR (Benign Neoplasms[Title/Abstract])) OR (Neoplasms, Benign[Title/Abstract])) OR (Benign Neoplasm[Title/Abstract])) OR (Neoplasm, Benign[Title/Abstract]))) OR (“Neoplasms”[Mesh]))” and also included references from relevant articles (that were not identified in the database search). The database search was conducted on September 11, 2020. The included articles were subject to a dual review by two authors, and references in the identified manuscripts were reviewed manually for any additional publications. Our team also searched the PROSPERO database without restrictions, and no articles were found. The registration number is CRD42020209009.
All the included studies had to meet the following criteria: (1) Patients diagnosed with NSCLC by histopathological analysis; (2) baseline LIPI score was graded and recorded before systemic therapy; (3) endpoints including PFS or OS were reported; and (4) survival estimates were present in the form of HRs with 95% confidence intervals (CIs) or Kaplan–Meier curves. Data identified through the above criteria were independently collected by two authors (XC and LH). Any problems with data extraction were resolved through a team discussion. We collected the following data: author’s name, year of publication, country, therapy type, number of participants (i.e., number of patients with good, intermediate, and poor LIPI scores, respectively), as well as disease outcomes (PFS/OS and associated hazard ratios [HRs] and 95% confidence intervals [CI]). For studies that did not report HR values, we derived corresponding survival estimates from Kaplan-Meier curves using the Engauge Digitizer software.
χ2 or Fisher exact tests were used to compare the clinical characteristics between different LIPI groups. Survival estimates were calculated by the Kaplan-Meier method, and comparison between subgroups was performed by the log-rank test. Univariate Cox regression analysis was implemented to analyze HRs and associated confidence intervals. To compare survival estimates among the three groups, a pairwise comparison was conducted to correct for multiplicity.
For the meta-analysis, we computed the weighted average PFS or OS reported for patients with different LIPI groups. In addition, the team examined I² statistics as well as the p-values of each result to assess heterogeneity between articles. If I2 ≤ 50%, we used a random-effects model; otherwise, a fixed-effects model was chosen. A p-value less than 0.05 was considered to indicate statistically significant heterogeneity. A subgroup analysis was performed based on the distinction of treatment. Begger’s funnel plot was used to assess publication bias in this meta-analysis. Sensitivity analysis was performed to assess the robustness of the meta-analysis. SPSS 23.0 and STATA version 15 were used for all statistical analyses. All p-values were two-sided, and a p-value less than 0.05 was defined as the threshold of statistical significance.
Among all patients receiving immunotherapy, a total of 91 were included in the final analysis. Among these 91 patients, 61 patients (67.0%) had a good LIPI score, 23 patients (25.3%) had an intermediate LIPI score, and 7 patients (7.7%) had a poor LIPI score. Patient demographics and clinical data are shown in Table 1. Among patients receiving chemotherapy, a total of 59.6% (n = 340), 32.5% (n = 185), and 7.9% (n = 45) presented with good, intermediate, and poor LIPI scores, respectively. For patients receiving targeted therapy, 64.1% (n = 211), 28.3% (n = 93), and 7.6% (n = 25) had good, intermediate, and poor LIPI scores, respectively. Supplementary Table 1 illustrates the clinical baseline and demographic features of these patients.
Table 1 Baseline demographic and clinical characteristics of NSCLC patients receiving immunotherapy.
Among patients receiving immunotherapy, the median PFS for those with a good LIPI score was 11.7 months (95% CI, 2.6-20.7 months), while for those with a intermediate LIPI score was 5.0 months (95% CI, 2.1-7.8 months) and for those with a poor LIPI score was 13.4 months (95% CI, 0.8-25.9 months); hence, PFS differed significantly based on LIPI groups (P = 0.005). Univariate analyses showed that HRs were 2.5 (95% CI, 1.4−4.5) and 1.26 (95% CI, 0.4−3.5) for those with intermediate and poor LIPI scores, respectively, compared to those with a good LIPI score. As for patients receiving chemotherapy, median PFS for those with poor, intermediate, and good LIPI scores was 5.3 months, 9.4 months, and 10.6 months, respectively (P = 0.123). In the targeted therapy cohort, the median PFS was 8.3 months (95% CI, 5.6−10.9 months), 10.1 months (95% CI, 13.1−18.4 months), and 12.0 months (95% CI, 22.0−30.7 months) for those with poor, intermediate, and good LIPI scores, respectively (P = 0.252; Figure 1). Results of pairwise comparison of PFS among the three LIPI groups are shown in Supplementary Table 2.
Figure 1 Associations of the lung immune prognostic index (LIPI) score and progression-free survival (PFS) following systemic therapy among non-small cell lung carcinoma (NSCLC) patients. Kaplan-Meier curves of PFS, comparing patients receiving immunotherapy, chemotherapy, and targeted therapy, are presented in (A–C), respectively.
For patients receiving immunotherapy, median OS was 17.4 months, 26.2 months, and 66.7 months for those with poor, intermediate, and good LIPI scores, respectively (P = 0.044). Univariate analyses indicated that the HR was 2.2 (95% CI, 1.1−4.4) for the intermediate group and 3.1 (95% CI, 0.69−14.1) for the poor group. For patients receiving chemotherapy, median OS was 7.5 months, 12.1 months, and 13.3 months for those with poor, intermediate, and good LIPI scores, respectively (P = 0.023); we found HRs of 1.1 (95% CI, 0.9−1.4) and 1.6 (95% CI, 1.1−2.2) for the intermediate and poor LIPI group, respectively. Finally, for those receiving targeted therapy, the median OS for those with poor, intermediate, and good LIPI scores was 13.6 months, 15.8 months, and 26.4 months, respectively. Compared to those with a good LIPI score, a higher LIPI score was associated with worse OS, with associated HRs of 1.6 (95% CI, 1.2−2.2) and 1.8 (95% CI, 1.1−2.7), respectively (Figure 2). Results of pairwise comparison of OS among the three LIPI groups are shown in Supplementary Table 3.
Figure 2 Associations of the lung immune prognostic index (LIPI) score and overall survival (OS) following systemic therapy among non-small cell lung carcinoma (NSCLC) patients. Kaplan-Meier curves of OS, comparing patients receiving immunotherapy, chemotherapy, and targeted therapy, are presented in (A–C), respectively.
A total of 54 relevant reports were retrieved from the search databases, and eight additional studies were identified through reviewing the reference sections of these manuscripts. After a screening and eligibility assessment, 15 studies were examined with a full-text screening. Of these 15 studies, we excluded six reports, including one review, two letters, three incomplete studies, and one duplication. Thus, eight studies (including our cohort) were suitable for the quantitative analysis (Figure 3).
All reports were retrospective studies. Eight of these studies assessed immunotherapy, five articles reported on chemotherapy, and three articles reported targeted therapy. A total of 8,721 patients from the studies met our inclusion criteria. Among these patients, 45.2% (n = 3943) received immune checkpoint inhibitors, 36.8% (n = 3208) received chemotherapy, and 18.0% (n = 1570) received targeted therapy. The population distributions and characteristics of the included studies are presented in Table 2. HRs for PFS and OS were available within four articles, and HRs for the other four articles were extracted using Kaplan-Meier curves.
Based on this meta-analysis, we found that a higher LIPI score was associated with shorter PFS (HR, 1.57; 95% CI, 1.45−1.71). The forest plot showed that worse PFS was observed in patients with poor LIPI (HR, 1.95; 95% CI, 1.75−2.17) and intermediate LIPI scores (HR, 1.33; 95% CI, 1.24−1.41) compared to patients with good LIPI scores. Subgroup analyses on the basis of therapeutic strategy demonstrated the prognostic value of a higher LIPI score harbored in all systemic therapies, including immunotherapy (HR, 1.77; 95% CI, 1.56−2.01), chemotherapy (HR, 1.38; 95% CI, 1.23−1.55), and targeted therapy (HR, 1.60; 95% CI, 1.25−2.06). The pooled results of the meta-analysis are shown in Figure 4.
Figure 4 Forest plots of pooled studies examining associations between the lung immune prognostic index (LIPI) score and progression-free survival (PFS), with subgroup analyses categorized by treatment region (A) and LIPI scores (B); HR, hazard ratio; CI, confidence interval; LIPI score of 1 vs. 0: intermediate versus good; LIPI score of 2 vs. 0: poor versus good.
Forest plots of the pooled results showed that higher LIPI score (HR, 2.01; 95% CI, 1.75−2.31), as well as intermediate (HR, 1.52; 95% CI, 1.37−1.65) and poor LIPI scores (HR, 2.67; 95% CI, 2.23−3.18) were risk factors for poor OS in NSCLC patients receiving systemic therapy. Subgroup analyses showed that a higher LIPI score was associated with worse clinical outcomes with regard to all systemic therapies, including immunotherapy (HR, 2.50; 95% CI, 1.99−3.13), chemotherapy (HR, 1.58; 95% CI, 1.34−1.86), and targeted therapy (HR, 2.15; 95% CI, 1.57−2.96) (Figure 5).
Figure 5 Forest plots of pooled studies examining associations between the lung immune prognostic index (LIPI) score for overall survival (OS), with subgroup analyses categorized by treatment region (A) and LIPI scores (B); HR, hazard ratio; CI, confidence interval; LIPI score of 1 vs. 0: intermediate versus good; LIPI score of 2 vs. 0: poor versus good.
Funnel plots with regard to OS and PFS are shown in the Supplementary Materials. The results indicated no publication bias (all p-values > 0.05) (Supplementary Figure 3). We conducted sensitivity analysis for the OS and PFS outcomes, and the pooled results demonstrated that removing any one study in any order did not influence our results, which indicated that the results were stable and reliable (Supplementary Figure 4).
In our cohort, we found that a higher LIPI score was a prognostic factor for OS and PFS in NSCLC patients receiving immunotherapy and for OS in patients receiving chemotherapy or targeted therapy. However, we found no association between LIPI score and PFS in NSCLC patients receiving chemotherapy or targeted therapy.
With regard to the meta-analysis, the pooled results of eight studies (which included 8,721 patients) demonstrated that baseline LIPI score was associated with the survival outcomes of NSCLC patients receiving systemic therapy through various treatment regimens. Subgroup analyses indicated that a higher LIPI score was related to inferior efficacy of immunotherapy, chemotherapy, as well as targeted therapy.
An uncontrolled inflammatory reaction is one of the main mechanisms underlying the occurrence of malignant tumors. DNA damage caused by long-term chronic inflammation can lead to the development of cancer (26, 27). An excessive proliferation of lung epithelial cells can lead to the secretion of various inflammatory cytokines, chemokines, and other inflammatory mediators (28, 29). Proto-oncogene products activate inflammatory pathways (30), increase DNA damage, and accelerate cell aging, thereby further aggravating tumor inflammation; this in turn generates a cascade of immunosuppressive cells, such as bone marrow-derived immune inhibiting tumor cells and tumor-associated macrophages (31). Thus, inflammation is closely related to the occurrence and development of tumors. To date, researchers have investigated the relationship between WBCs, neutrophils, monocytes, platelets, dNLR, C-reaction protein (CRP), and other systemic inflammatory reaction indicators and the progression/treatment efficacy of NSCLC (32).
The dNLR is an effective indicator of systemic inflammation (33). Elevated dNLR levels may indicate an angiogenic or pro-inflammatory state in the tumor microenvironment, which reflects the balance between neutrophils and lymphocytes, as well as the patient’s overall immune status. LDH is a key enzyme involved in tumor metabolism and is a biomarker of poor survival in patients with cancer. It is capable of accelerating resistance to systemic therapy by promoting immune suppression and immune escape (34, 35).
Evaluating a combination of multiple prognostic factors has great advantages in clinical practice. At present, many researchers are committed to identifying valuable biomarkers to predict the efficacy of systemic treatment in NSCLC, especially with regard to immunotherapy (36). However, there is currently a lack of effective factors. The LIPI can be used to assess systemic inflammation among patients based on dNLR and LDH levels and can help to evaluate the level of systemic inflammation in patients with cancer. Our results indicate that a higher LIPI score is related to worse clinical outcome among NSCLC patients receiving systemic therapy. Both dNLR and LDH are routine and objective indicators that can be accessed without difficulty during clinical practice; each hospital has a uniform standard to define abnormal laboratory testing results.
Our study was the first to investigate the prognostic value of the LIPI among Chinese NSCLC patients receiving systemic therapy, and our results regarding target therapy were different from those of previous studies in Western countries. Of note, the most common mutation in our cohort was the epidermal growth factor receptor (EGFR) gene mutation, which is the most frequent driver gene mutation in NSCLC; this mutation has a prevalence of 10% in the Western NSCLC population, but the prevalence is as high as 50% in the Asian NSCLC population (37). The relationship between LIPI score and PFS of NSCLC patients with target therapy might be different between different populations and ethnic groups, so more data from Asian populations are needed in the future.
To our knowledge, only Xie et al. have published a meta-analysis about the relationship between the LIPI and efficacy of different treatments in NSCLC patients (38). In this article, there were only four studies included. Meanwhile, the article only included overall survival as outcome indicators. Our meta-analysis included more studies, especially the study performed in our institution, which is the first analysis about the association between LIPI score and PFS/OS of NSCLC patients in the Chinese cohort. Including our cohort of Chinese patients added patient diversity and improved the external interpretation of the meta-analysis. Therefore, we have provided stronger evidence concerning the prognostic value of LIPI score in advanced NSCLC patients.
This study presents limitations as well as strengths. Our study was a retrospective, single-center study. Although we conducted a meta-analysis, all of the included studies were retrospective studies. Moreover, baseline characteristics including pathological classification were not available in most of the literature, preventing further subgroup analysis in specific subsets of NSCLC patients. Specifically, research on targeted therapy cohorts mainly incorporated information on EGFR and anaplastic lymphoma kinase (ALK); other driver mutations should be considered in future studies. Additionally, in our immunotherapy cohort, the median PFS of the poor LIPI group were even longer than those of the good and intermedia group, and the intermediate group was the one with the worst prognosis. The reason may lie in that the sample size of the poor LIPI group was small (n=7). In fact, all the patients in the poor LIPI group had developed disease progression before the 14th month, whereas the other two groups had not. This phenomenon could be directly caused by limited patients in the poor group. Thus, these relevant results should be cautiously interpreted. Further studies with larger cohorts are required to verify our results. Besides, results from the meta-analysis with a large sample in the poor group (n=551) suggested that poor LIPI score was associated with worse prognoses in NSCLC patients. Moreover, some survival information was unavailable; therefore, digitizing and extracting Kaplan-Meier curve-related survival information through the Engauge Digitizer might have inevitably led to some bias.
In summary, among advanced NSCLC patients receiving systemic therapy, a higher baseline LIPI score (i.e., an intermediate or poor LIPI score) was associated with poorer survival outcomes. Additional randomized clinical trials are required to comprehensively inquire into the predictive value of the LIPI in NSCLC.
The original contributions presented in the study are included in the article/Supplementary Material. Further inquiries can be directed to the corresponding authors.
LH and LZ conceived the study. LH, XC, and QX authored the article. JX performed the literature review and extracted the signature models. LH, HH, PZ, and SC performed the analyses and wrote the first draft of the manuscript, supported by TL and YS. All authors contributed to the article and approved the submitted version.
This work was supported by the Jiangsu Provincial Social Development - Key Projects - Clinical Frontier Technologies (grant number BE2019719) and the Jiangsu Provincial Social Development - General Program (grant number BE2019718).
The authors declare that the research was conducted in the absence of any commercial or financial relationships that could be construed as a potential conflict of interest.
We are grateful to all the participants who made this research possible. All authors contributed equally to this article.
The Supplementary Material for this article can be found online at: https://www.frontiersin.org/articles/10.3389/fonc.2021.670230/full#supplementary-material
1. Siegel RL, Miller KD, Jemal A. Cancer Statistics, 2019. CA: Cancer J Clin (2019) 69(1):7–34. doi: 10.3322/caac.21551
2. Edwards AT. Tumours of the Lung. British Surgery (2010) 26(101):166–92. doi: 10.1002/bjs.18002610116
3. Kris MG, Johnson BE, Berry LD, Kwiatkowski DJ, Iafrate AJ, Wistuba II, et al. Using Multiplexed Assays of Oncogenic Drivers in Lung Cancers to Select Targeted Drugs. JAMA (2014) 311(19):1998–2006. doi: 10.1001/jama.2014.3741
4. Recondo G, Facchinetti F, Olaussen KA, Besse B, Friboulet L. Making the First Move in EGFR-Driven or ALK-Driven NSCLC: First-Generation or Next-Generation TKI? Nat Rev Clin Oncol (2018) 15(11):694–708. doi: 10.1038/s41571-018-0081-4
5. Reck M, Rodríguez-Abreu D, Robinson AG, Hui R, Csőszi T, Fülöp A, et al. Pembrolizumab Versus Chemotherapy for PD-L1-Positive Non-Small-Cell Lung Cancer. New Engl J Med (2016) 375(19):1823–33. doi: 10.1056/NEJMoa1606774
6. Kelderman S, Schumacher TN, Haanen JB. Acquired and Intrinsic Resistance in Cancer Immunotherapy. Mol Oncol (2014) 8(6):1132–9. doi: 10.1016/j.molonc.2014.07.011
7. Zhong J, Li L, Wang Z, Bai H, Gai F, Duan J, et al. Potential Resistance Mechanisms Revealed by Targeted Sequencing From Lung Adenocarcinoma Patients With Primary Resistance to Epidermal Growth Factor Receptor (Egfr) Tyrosine Kinase Inhibitors (Tkis). J Thoracic Oncol Off Publ Int Assoc Study Lung Cancer (2017) 12(12):1766–78. doi: 10.1016/j.jtho.2017.07.032
8. Cortellini A, Tiseo M, Banna GL, Cappuzzo F, Aerts J, Barbieri F, et al. Clinicopathologic Correlates of First-Line Pembrolizumab Effectiveness in Patients With Advanced NSCLC and a PD-L1 Expression of ≥ 50. Cancer Immunol Immunother CII (2020) 69(11):2209–21. doi: 10.1007/s00262-020-02613-9
9. Cortellini A, De Giglio A, Cannita K, Cortinovis DL, Cornelissen R, Baldessari C, et al. Smoking Status During First-Line Immunotherapy and Chemotherapy in NSCLC Patients: A Case-Control Matched Analysis From a Large Multicenter Study. Thorac Cancer (2021) 12(6):880–9. doi: 10.1111/1759-7714.13852
10. Cantini L, Pecci F, Hurkmans DP, Belderbos RA, Lanese A, Copparoni C, et al. High-Intensity Statins are Associated With Improved Clinical Activity of PD-1 Inhibitors in Malignant Pleural Mesothelioma and Advanced non-Small Cell Lung Cancer Patients. Eur J Cancer (Oxford Engl 1990) (2021) 144:41–8. doi: 10.1016/j.ejca.2020.10.031
11. Cortellini A, Ricciuti B, Tiseo M, Bria E, Banna GL, Aerts JG, et al. Baseline BMI and BMI Variation During First Line Pembrolizumab in NSCLC Patients With a PD-L1 Expression ≥ 50%: A Multicenter Study With External Validation. J Immunother Cancer (2020) 8(2):e001403. doi: 10.1136/jitc-2020-001403
12. Diakos CI, Charles KA, McMillan DC, Clarke SJ. Cancer-Related Inflammation and Treatment Effectiveness. Lancet Oncol (2014) 15(11):e493–503. doi: 10.1016/s1470-2045(14)70263-3
13. Wang Z, Zhan P, Lv Y, Shen K, Wei Y, Liu H, et al. Prognostic Role of Pretreatment Neutrophil-To-Lymphocyte Ratio in Non-Small Cell Lung Cancer Patients Treated With Systemic Therapy: A Meta-Analysis. Trans Lung Cancer Res (2019) 8(3):214–26. doi: 10.21037/tlcr.2019.06.10
14. Tong T, Guan Y, Xiong H, Wang L, Pang J. A Meta-Analysis of Glasgow Prognostic Score and Modified Glasgow Prognostic Score as Biomarkers for Predicting Survival Outcome in Renal Cell Carcinoma. Front Oncol (2020) 10:1541. doi: 10.3389/fonc.2020.01541
15. Jiang P, Gu S, Pan D, Fu J, Sahu A, Hu X, et al. Signatures of T Cell Dysfunction and Exclusion Predict Cancer Immunotherapy Response. Nat Med (2018) 24(10):1550–8. doi: 10.1038/s41591-018-0136-1
16. Lenci E, Cantini L, Pecci F, Cognigni V, Agostinelli V, Mentrasti G, et al. The Gustave Roussy Immune (Grim)-Score Variation is an Early-On-Treatment Biomarker of Outcome in Advanced Non-Small Cell Lung Cancer (Nsclc) Patients Treated With First-Line Pembrolizumab. J Clin Med (2021) 10(5):1005. doi: 10.3390/jcm10051005
17. Banna GL, Cortellini A, Cortinovis DL, Tiseo M, Aerts J, Barbieri F, et al. The Lung Immuno-Oncology Prognostic Score (LIPS-3): A Prognostic Classification of Patients Receiving First-Line Pembrolizumab for PD-L1 ≥ 50% Advanced Non-Small-Cell Lung Cancer. ESMO Open (2021) 6(2):100078. doi: 10.1016/j.esmoop.2021.100078
18. Benitez JC, Recondo G, Rassy E, Mezquita L. The LIPI Score and Inflammatory Biomarkers for Selection of Patients With Solid Tumors Treated With Checkpoint Inhibitors. Q J Nucl Med Mol Imaging Off Publ Ital Assoc Nucl Med (AIMN) [and] Int Assoc Radiopharmacol (IAR) [and] Section So (2020) 64(2):162–74. doi: 10.23736/s1824-4785.20.03250-1
19. Meyers DE, Stukalin I, Vallerand IA, Lewinson RT, Suo A, Dean M, et al. The Lung Immune Prognostic Index Discriminates Survival Outcomes in Patients With Solid Tumors Treated With Immune Checkpoint Inhibitors. Cancers (2019) 11(11):1713. doi: 10.3390/cancers11111713
20. Mezquita L, Auclin E, Ferrara R, Charrier M, Remon J, Planchard D, et al. Association of the Lung Immune Prognostic Index With Immune Checkpoint Inhibitor Outcomes in Patients With Advanced non-Small Cell Lung Cancer. JAMA Oncol (2018) 4(3):351–7. doi: 10.1001/jamaoncol.2017.4771
21. Minami S, Ihara S, Komuta K. Pretreatment Lung Immune Prognostic Index Is a Prognostic Marker of Chemotherapy and Epidermal Growth Factor Receptor Tyrosine Kinase Inhibitor. World J Oncol (2019) 10(1):35–45. doi: 10.14740/wjon1179
22. Kazandjian D, Gong Y, Keegan P, Pazdur R, Blumenthal GM. Prognostic Value of the Lung Immune Prognostic Index for Patients Treated for Metastatic Non-Small Cell Lung Cancer. JAMA Oncol (2019) 5(10):1481–5. doi: 10.1001/jamaoncol.2019.1747
23. Ruiz-Bañobre J, Areses-Manrique MC, Mosquera-Martínez J, Cortegoso A, Afonso-Afonso FJ, de Dios-Álvarez N, et al. Evaluation of the Lung Immune Prognostic Index in Advanced Non-Small Cell Lung Cancer Patients Under Nivolumab Monotherapy. Trans Lung Cancer Res (2019) 8(6):1078–85. doi: 10.21037/tlcr.2019.11.07
24. Sorich MJ, Rowland A, Karapetis CS, Hopkins AM. Evaluation of the Lung Immune Prognostic Index for Prediction of Survival and Response in Patients Treated With Atezolizumab for NSCLC: Pooled Analysis of Clinical Trials. J Thoracic Oncol Off Publ Int Assoc Study Lung Cancer (2019) 14(8):1440–6. doi: 10.1016/j.jtho.2019.04.006
25. Mazzaschi G, Minari R, Zecca A, Cavazzoni A, Ferri V, Mori C, et al. Soluble PD-L1 and Circulating Cd8+Pd-1+ and NK Cells Enclose a Prognostic and Predictive Immune Effector Score in Immunotherapy Treated NSCLC Patients. Lung Cancer (2020) 148:1–11. doi: 10.1016/j.lungcan.2020.07.028
26. Mantovani A, Allavena P, Sica A, Balkwill F. Cancer-Related Inflammation. Nature (2008) 454(7203):436–44. doi: 10.1038/nature07205
27. Crusz SM, Balkwill FR. Inflammation and Cancer: Advances and New Agents. Nat Rev Clin Oncol (2015) 12(10):584–96. doi: 10.1038/nrclinonc.2015.105
28. Grivennikov SI, Greten FR, Karin M. Immunity, Inflammation, and Cancer. Cell (2010) 140(6):883–99. doi: 10.1016/j.cell.2010.01.025
29. Chen DS, Mellman I. Elements of Cancer Immunity and the Cancer-Immune Set Point. Nature (2017) 541(7637):321–30. doi: 10.1038/nature21349
30. Borrello MG, Degl’Innocenti D, Pierotti MA. Inflammation and Cancer: The Oncogene-Driven Connection. Cancer Lett (2008) 267(2):262–70. doi: 10.1016/j.canlet.2008.03.060
31. Greten FR, Grivennikov SI. Inflammation and Cancer: Triggers, Mechanisms, and Consequences. Immunity (2019) 51(1):27–41. doi: 10.1016/j.immuni.2019.06.025
32. Takada K, Takamori S, Yoneshima Y, Tanaka K, Okamoto I, Shimokawa M, et al. Serum Markers Associated With Treatment Response and Survival in Non-Small Cell Lung Cancer Patients Treated With Anti-PD-1 Therapy. Lung Cancer (2020) 145:18–26. doi: 10.1016/j.lungcan.2020.04.034
33. Scilla KA, Bentzen SM, Lam VK, Mohindra P, Nichols EM, Vyfhuis MA, et al. Neutrophil-Lymphocyte Ratio is a Prognostic Marker in Patients With Locally Advanced (Stage IIIA and IIIB) non-Small Cell Lung Cancer Treated With Combined Modality Therapy. Oncologist (2017) 22(6):737–42. doi: 10.1634/theoncologist.2016-0443
34. Ding J, Karp JE, Emadi A. Elevated Lactate Dehydrogenase (LDH) can be a Marker of Immune Suppression in Cancer: Interplay Between Hematologic and Solid Neoplastic Clones and Their Microenvironments. Cancer Biomarkers Section A Dis Markers (2017) 19(4):353–63. doi: 10.3233/cbm-160336
35. Deme D, Telekes A. [Prognostic Importance of Lactate Dehydrogenase (LDH) in Oncology]. Orv Hetil (2017) 158(50):1977–88. doi: 10.1556/650.2017.30890
36. Havel JJ, Chowell D, Chan TA. The Evolving Landscape of Biomarkers for Checkpoint Inhibitor Immunotherapy. Nat Rev Cancer (2019) 19(3):133–50. doi: 10.1038/s41568-019-0116-x
37. Castellanos E, Feld E, Horn L. Driven by Mutations: The Predictive Value of Mutation Subtype in EGFR-Mutated Non-Small Cell Lung Cancer. J Thoracic Oncol Off Publ Int Assoc Study Lung Cancer (2017) 12(4):612–23. doi: 10.1016/j.jtho.2016.12.014
Keywords: non-small cell lung cancer, immunotherapy, chemotherapy, targeted therapy, lung immune prognostic index (LIPI)
Citation: Huang L, Han H, Zhou L, Chen X, Xu Q, Xie J, Zhan P, Chen S, Lv T and Song Y (2021) Evaluation of the Lung Immune Prognostic Index in Non-Small Cell Lung Cancer Patients Treated With Systemic Therapy: A Retrospective Study and Meta-Analysis. Front. Oncol. 11:670230. doi: 10.3389/fonc.2021.670230
Received: 02 March 2021; Accepted: 03 May 2021;
Published: 24 June 2021.
Edited by:
Rossana Berardi, Marche Polytechnic University, ItalyReviewed by:
Luca Cantini, Erasmus Medical Center, NetherlandsCopyright © 2021 Huang, Han, Zhou, Chen, Xu, Xie, Zhan, Chen, Lv and Song. This is an open-access article distributed under the terms of the Creative Commons Attribution License (CC BY). The use, distribution or reproduction in other forums is permitted, provided the original author(s) and the copyright owner(s) are credited and that the original publication in this journal is cited, in accordance with accepted academic practice. No use, distribution or reproduction is permitted which does not comply with these terms.
*Correspondence: Yong Song, eW9uZy5zb25nQG5qdS5lZHUuY24=; Tangfeng Lv, YmFpcm91c2h1aUAxNjMuY29t
†These authors share first authorship
Disclaimer: All claims expressed in this article are solely those of the authors and do not necessarily represent those of their affiliated organizations, or those of the publisher, the editors and the reviewers. Any product that may be evaluated in this article or claim that may be made by its manufacturer is not guaranteed or endorsed by the publisher.
Research integrity at Frontiers
Learn more about the work of our research integrity team to safeguard the quality of each article we publish.