- 1Department of Emergency, The First Affiliated Hospital of Guangxi Medical University, Nanning, China
- 2Guangxi Clinical Research Center for CRC, Department of Gastrointestinal Surgery, Guangxi Medical University Cancer Hospital, Nanning, China
- 3Department of Anorectal Surgery, Zhuzhou Center Hospital, Zhuzhou, China
- 4Department of Medical Oncology, The First Affiliated Hospital of Guangxi Medical University, Nanning, China
- 5Department of Hepatological Surgery, Guangxi Medical University Cancer Hospital, Nanning, China
Background: Lymph node metastasis (LNM) is a well-established prognostic factor for colon cancer. Preoperative LNM evaluation is relevant for planning colon cancer treatment. The aim of this study was to construct and evaluate a nomogram for predicting LNM in primary colon cancer according to pathological features.
Patients and Methods: Six-hundred patients with clinicopathologically confirmed colon cancer (481 cases in the training set and 119 cases in the validation set) were enrolled in the Affiliated Cancer Hospital of Guangxi Medical University from January 2010 to December 2019. The expression of molecular markers (p53 and β-catenin) was determined by immunohistochemistry. Multivariate logistic regression was used to screen out independent risk factors, and a nomogram was established. The accuracy and discriminability of the nomogram were evaluated by consistency index and calibration curve.
Results: Univariate logistic analysis revealed that LNM in colon cancer is significantly correlated (P <0.05) with tumor size, grading, stage, preoperative carcinoembryonic antigen (CEA) level, and peripheral nerve infiltration (PNI). Multivariate logistic regression analysis confirmed that CEA, grading, and PNI were independent prognostic factors of LNM (P <0.05). The nomogram for predicting LNM risk showed acceptable consistency and calibration capability in the training and validation sets.
Conclusions: Preoperative CEA level, grading, and PNI were independent risk factor for LNM. Based on the present parameters, the constructed prediction model of LNM has potential application value.
Introduction
Colorectal cancer (CRC) is one of the most common malignancies with the second highest death rate in 2018 (1, 2). Doctors can formulate a variety of treatment plans for CRC because of the continuous enrichment of treatment options. However, surgical treatment remains the primary treatment for CRC. The rate of lymph node metastasis (LNM) in early CRC is 6.9–19.6% (3–6). The scope of surgery can be adopted for patients with CRC to avoid excessive treatment. Preoperative evaluation of LNM risk in colon cancer may help i) to provide information on an important prognostic factor (7, 8) and ii) to plan the most appropriate therapeutic and staging strategies, particularly in the neoadjuvant setting (9).
LNM in colon cancer can be predicted by histopathological markers. Tumor stage and grade are well-recognized predictors (10–12). However, in clinical practice, statistical results cannot be directly applied to individual clinical diagnosis and treatment. Although a nomogram model for colon cancer LNM has been developed for clinical use, the validation of the model with external data sets is still lacking (8, 10). Therefore, we retrospectively analyzed the data of 600 patients with primary colon cancer admitted in 2010 and 2019 and attempted to establish a nomogram prediction model for colon cancer.
Methods
Study Selection
This study retrospectively collected 600 clinical cases of colon cancer confirmed by postoperative pathology in the Affiliated Cancer Hospital of Guangxi Medical University from January 2010 to December 2019. The cases included 369 males and 231 females (age range, 19–87 years; mean age, 60 years old). We defined the training and the validation groups by time in the study. The training set was used to establish the model, and the validation set was used to verify the performance of the model. The training group was composed of 481 patients who were admitted between January 2013 and December 2019, and the validation group consisted of 119 patients who were hospitalized between January 2010 and December 2012. The inclusion criteria were as follows (1): colon cancer was confirmed by pathological examination (2); lymph node dissection was performed, and the number of lymph nodes detected was at least 12 (3); radical surgery was performed (4); complete clinical data and pathological information were available (5); all patients underwent lymph node D3 dissection (D3 lymph node dissection refers to the dissection of parenteral, intermediate, and central lymph nodes); and (6) the postoperative pathological diagnosis was colorectal adenocarcinoma. The exclusion criteria were as follows (1): preoperative neoadjuvant chemotherapy was performed; and (2) the co-occurrence of other tumor diseases at the time of diagnosis (Figure 1). The surgery was performed by an experienced associate chief physician or a chief physician who is able to perform laparoscopic surgery independently.
Variable Analysis
The variables selected in this study included the following clinicopathologic data and biomarkers: gender, age, BMI, drinking status, smoking status, tumor site, tumor size, carcinoembryonic antigen (CEA) level, platelet–lymphocyte ratio (PLR), neutrophil–lymphocyte ratio (NLR), LNM, pathological tumor (pT) stage, tumor differentiation, peripheral nerve infiltration (PNI), p53 expression, vascular infiltration, and β-catenin expression. The optimal cutoff value (PLR = 279, NLR = 4.24, tumor diameter = 3.35), sensitivity, and specificity were calculated according to the receiver operating characteristic (ROC) curve. The preoperative CEA value was 5 U/ml. Immunohistochemical staining score is based on the proportion of stained tumor cells. The protein expression levels of p53 and β-catenin were divided into high expression (>20%) and low expression (≤20%).
Statistical Analysis
In this study, SPSS 26.0 and R software (version 3.6.1, www.r-project.org) were used in statistical analyses. P values were calculated by Chi-Square test for categorical variables. P <0.05 was considered statistically significant. We used Cox proportional risk model to determine the independent factors that affect LNM based on the variables selected in the univariate analysis. We used logistic regression model in multivariate analysis to predict LNM. Consistency index (C-index) was calculated, and the calibration results were evaluated by calibration curves. The nomogram was drawn by referring to the step-by-step method provided by Zhang et al. (13). We used a series of software packages in R, including rmda, proc, foreign, nrichens, rms, and survival, to build the nomogram.
Results
Patient Characteristics
The parameters of the training and verification sets are shown in Table 1. The training set consisted of 302 males and 179 females. The average age was 60 years. Among them, 398 cases had high P53 expression and 83 cases had low P53 expression. The validation group consisted of 78 men and 41 women. Among them, 95 cases had high p53 expression and 24 cases had low p53 expression. LNM was associated with the following clinicopathological parameters: tumor differentiation (P = 0.004), CEA (P ≤0.01), pT stage (P = 0.004), PNI (P ≤0.01), vascular infiltration (P ≤0.01), and tumor diameter (P = 0.033, Table 2).
Univariate and Multivariate Analyses of Clinical Variables
Univariate logistic regression analysis showed that colon cancer LNM was correlated with tumor size, grading, pT stage, preoperative CEA level, and PNI (P <0.05, Table 2). Multivariate logistic regression analysis showed that preoperative CEA, grading, and PNI were correlated with LNM in colon cancer (P <0.05). PNI was an independent predictor of LNM in colon cancer (P <0.05, Table 3).
Construction and Validation of the Nomogram
A nomogram was established to predict the risk of LNM in colon cancer (Figure 2). The C-index was 0.686 in the training set and 0.644 in the validation set. The bootstrap method was internally and externally validated to show the good fit of the model. The prediction of LNM risk highly fits the actual metastasis (Figures 3, 4). Declining curve analysis (DCA) shows that the net benefit rate of the model is in the range of 0.2–0.8 for pT, which is higher than that in the limit curve (Figure 5), using the nomogram. The corresponding score was determined according to the clinicopathological data of the patient and the tumor. All the points were added to obtain the total. Finally, the risk value that corresponds to the total score was determined. For example, the following results were obtained: preoperative CEA >5 U/ml; the total score of moderate differentiation with PNI in patients with colon cancer was 90 + 42 + 75 = 207; then, the corresponding risk for LNM was 65%. Physicians and patients can use the nomogram to predict the risk of LNM and individually assess patients more accurately to help them choose a more appropriate treatment plan.
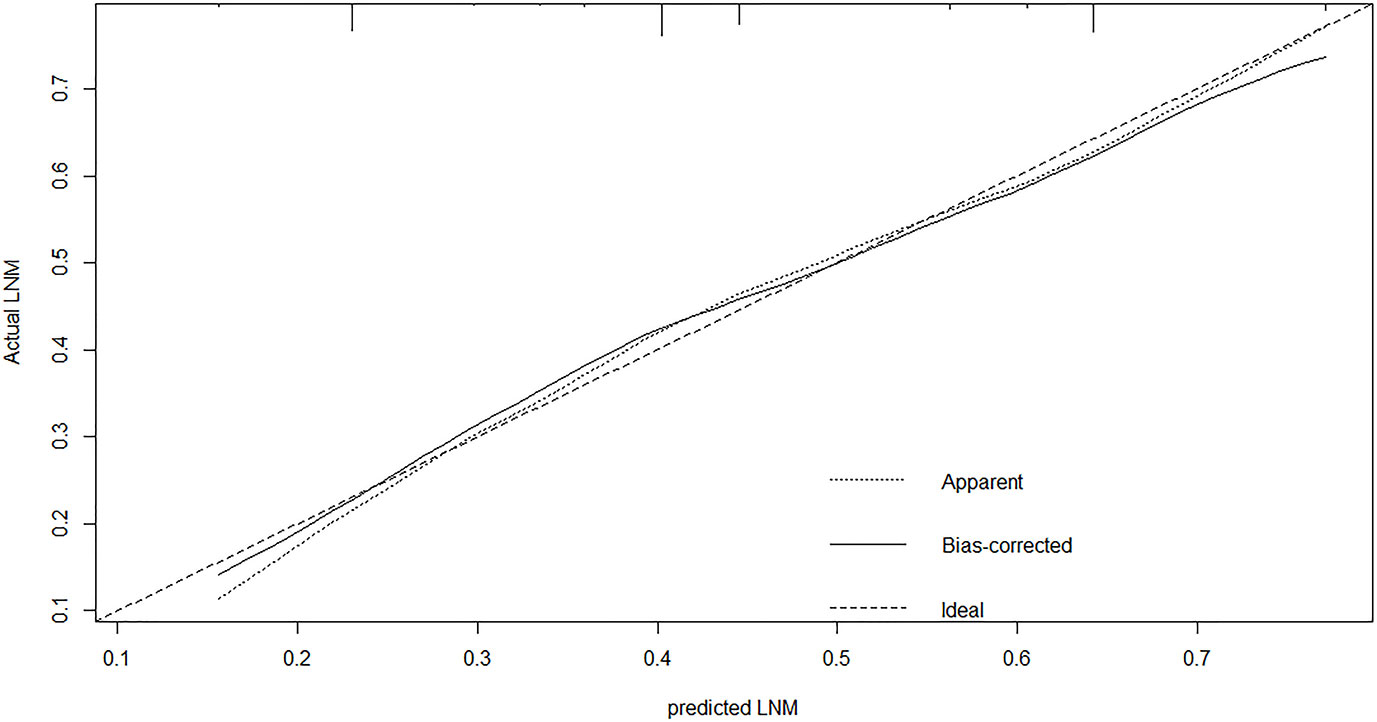
Figure 3 The calibration plot showed a high fit between actual and predicted lymph node metastases in training set.
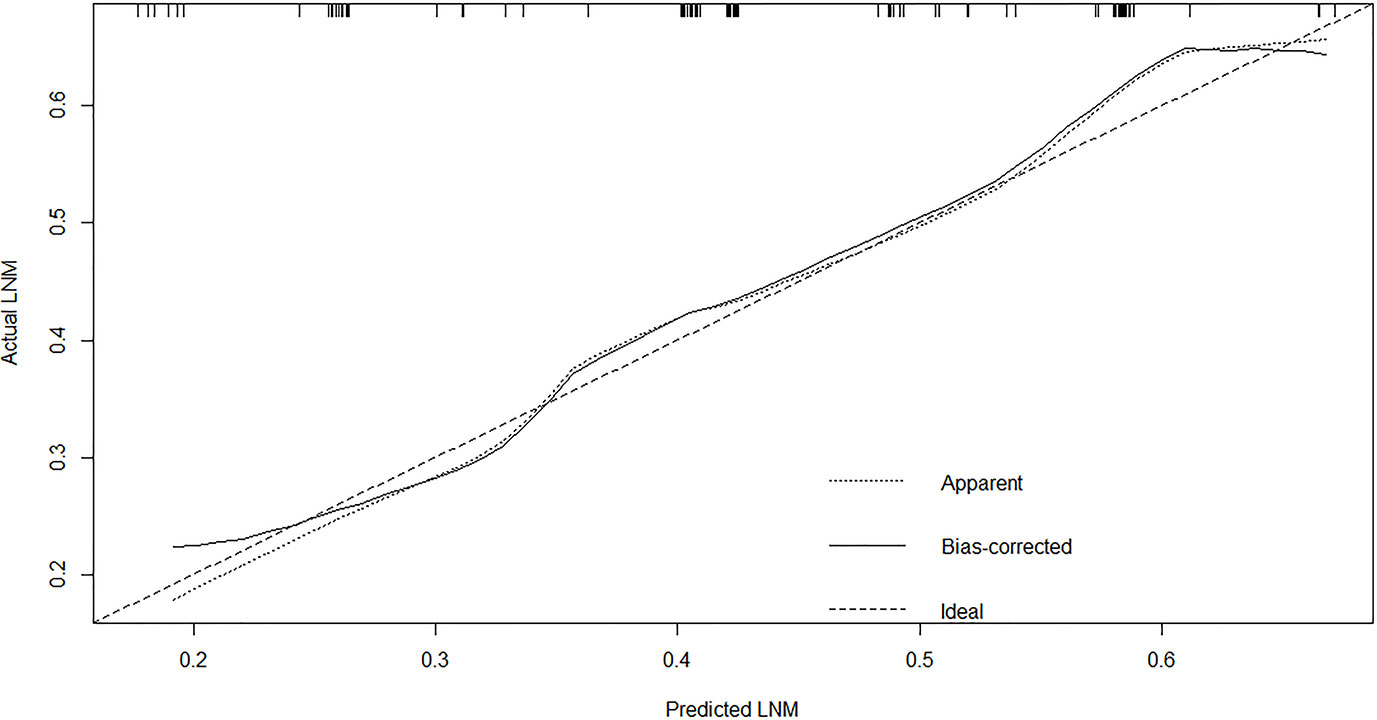
Figure 4 The calibration plot showed a high fit between actual and predicted lymph node metastases in external set.
Discussion
In this study, a clinical model for the individualized prediction of LNM in colon cancer was established. The model consists of basic data and clinical risk factors. Sixteen clinicopathological features were analyzed by univariate regression. Three clinical and pathological risk indicators were selected as independent risk factors for multivariate logistic analysis. Finally, the independent risk factors were applied to establish a visual prediction model. We analyzed an external validation set, which did not involve those enrolled in the internal data set, to further validate the predictive performance of the model. The model has promising clinical value in predicting LNM. The results of this study indicated that the LNM-associated nomogram has a favorable application prospect in patients with colon cancer. Similar to previous reports (3, 14–17), this study found that tumor differentiation, preoperative CEA level, and PNI were independent risk factors associated with LNM in colon cancer.
Among the three potential clinical risk factors, CEA level is the earliest clinical indicator and closely related to LNM in colon cancer (14). Several studies have examined the number, distribution, size, and percentage of lymph nodes involved to assess colon cancer survival (18–20). A multicenter study found that higher CEA level and worse tumor differentiation leads to more LNM (16). Han et al. also suggested that LNM and even the distant metastasis of colon cancer are positively correlated with CEA level (21). We found by analyzing the nomogram constructed in this study that, except for tissue differentiation, preoperative CEA level had the greatest influence on the model among all the potential risk factors. The results of this study further support the idea that patients with a higher preoperative CEA level should be considered at high risk for colon cancer LNM. Martin R. Weiser and colleagues developed a colon cancer recurrence nomogram to predict relapse based on the number of positive and negative lymph nodes, lymphovascular invasion, and other risk factors (22). Compared with the vascular infiltration of CRC, few studies have been conducted on the correlation between PNI and LNM in colon cancer. Although PNI can be considered a way of local diffusion, it can also be the only way to determine the range of distant metastasis (23). The number of LNM in patients with PNI is twice than that in patients without PNI (24). Compared with the 12.6–30% incidence of PNI reported in other studies (10, 24, 25), the present study found that the event rate was nearly 50%, which may lead to a high incidence that differed from the inclusion criteria. The study included patients who underwent radical resection of colon cancer. The rate of LNM in patients with PNI was 53.3% (145/272), which was remarkably higher than that in patients without PNI (69/209). Studies have found statistically substantial differences in the expression of P53 and β-catenin in CRC LNM. P53 overexpression or decreased β-catenin expression is more common in patients with LNM from CRC (26, 27). In addition, disease-free survival is reduced in patients with p53 overexpression (28). Although the above relationship was not found in the present study, we still cannot ignore the potential role of P53 and β-catenin in LNM.
Regional LNM prediction models for CRC had been proposed to better apply the research results to clinical work. However, these models were developed for CRC. Specific prediction models for LNM in colon cancer are lacking. Two scholars constructed a risk model of LNM in T1 stage CRC using clinical and pathological parameters (11, 29). Clinical imaging nomograms based on radiomics and clinical risk factors were constructed to improve the predictive power of preoperative LNM (30, 31). The accuracy of the model is better than that of simple clinical or pathological parameters. Many scholars agree that the model can be used for the preoperative prediction of LNM in patients with CRC and other tumors (11, 32, 33). The nomogram’s area under the ROC curve (AUC) for predicting LNM of non-digestive tract tumors using clinicopathological parameters was above 0.75, which was remarkably higher than that for digestive tract tumors, including colon cancer (34–36). Nomogram is widely used in CRC because of its remarkability for individual treatment and prognostic prediction. The C-index showed good discrimination in the internal (C = 0.687) and external validation sets (C = 0.644). The nomogram’s calibration curve is very close to the optimal curve. Doctors can add scores corresponding to each index state to obtain the total score in clinical application and then obtain the corresponding LNM probability because of the model’s convenience, economy, and practicality. The application of this nomogram will contribute to an accurate understanding of the disease and help doctors and patients choose a personalized treatment. After treatment, the nomogram could help doctors to distinguish high- and low-risk patients and formulate follow-up for high-risk patients. Some adjuvant treatments, such as chemical drugs and targeted drugs, should be given to consolidate therapeutic effects and help postoperative patients, especially high-risk patients. The nomogram is a useful clinical tool that can reduce colectomy after endoscopic resection among patients with T1 colon cancer. The effectiveness of conventional diagnostic methods is usually determined by creating ROC curves and calculating the AUC. However, ROC only considers the specificity and sensitivity of the method and pursues accuracy. We speculated whether the nomogram is clinically accurate enough and whether patients benefit from the use of the nomogram. Therefore, in addition to building the model, we used DCA to determine whether patients would benefit from the clinical prediction model. The DCA results show that the net benefit rate of the model was in the range of 0.2–0.8 for pT, which was higher than that in the extreme curve.
This study has the following limitations. 1. This study is a retrospective study, and selectivity bias is inevitable. 2. Data from a single center at different periods were used for the external validation of the model. Thus, the promotion and use of the model should be performed cautiously. 3. Regional lymph nodes were not subdivided and compared. 4. The model reflects the basic situation of the patient at a specific time. The use span of the model may show certain limitations with changes in time. 5. Validation of external data from multiple medical centers is also needed to promote the model. Therefore, the next research focus is to test the model with external data. In addition, we considered the influence of PNI and tissue differentiation degree on CRC when setting possible influencing factors. Although the grading and PNI can be obtained preoperatively through a puncture, this procedure often requires an adequate amount of tissue and therefore needs to be performed with caution.
Conclusion
Based on tumor differentiation, preoperative CEA level and PNI, a nomogram model was established for predicting the incidence of LNM in colon cancer patients. The predicted model of LNM risk of colon cancer has potential application value. However, further prospective studies with large samples are still necessary.
Data Availability Statement
The raw data supporting the conclusions of this article will be made available by the authors, without undue reservation.
Ethics Statement
The studies involving human participants were reviewed and approved by The First Affiliated Hospital of Guangxi Medical University. The patients/participants provided their written informed consent to participate in this study.
Author Contributions
YX, YC, and CL contributed equally to this study. YX designed the experiments and wrote the manuscript. L-xH, CW, and SL collected the date. YC and SL analyzed the data. WT funded the project. YC, CL, FL, and HZ checked and revised the manuscript and confirmed all the data in the manuscript. All authors contributed to the article and approved the submitted version.
Funding
This work was supported by (1): National Natural Science Foundation of China (81973533) (2), 2019 Guangxi University High-level Innovation Team and the Project of Outstanding Scholars Program, and (3) Guangxi Science and Technology Project (2019AC03004 and AD19245197).
Conflict of Interest
The authors declare that the research was conducted in the absence of any commercial or financial relationships that could be construed as a potential conflict of interest.
References
1. Freddie Bray BMP, Jacques Ferlay M, Isabelle Soerjomataram MMP, Rebecca L, Siegel M, Lindsey A. Torre M, et al. Global Cancer Statistics: 2018 GLOBOCAN Estimates of Incidence and Mortality Worldwide for 36 Cancers in 185 Countries. CA Cancer J Clin (2018) 0:1–31. doi: 10.3322/caac.21492
2. Eric E, Low MMJD, RBMC MPH, Provenzale MTKM, Elena Martinez PSGM. Risk Factors for Early-Onset Colorectal Cancer. Gastroenterology (2020) 159:492–501. doi: 10.1053/j.gastro.2020.01.004
3. Yasue C, Chino A, Takamatsu M, Namikawa K, Ide D, Saito S, et al. Pathological Risk Factors and Predictive Endoscopic Factors for Lymph Node Metastasis of T1 Colorectal Cancer: A Single-Center Study of 846 Lesions. J Gastroenterol (2019) 54:708–17. doi: 10.1007/s00535-019-01564-y
4. Watanabe T, Muro K, Ajioka Y, Hashiguchi Y, Ito Y, Saito Y, et al. Japanese Society for Cancer of the Colon and Rectum (JSCCR) Guidelines 2016 for the Treatment of Colorectal Cancer. Int J Clin Oncol (2018) 23:1–34. doi: 10.1007/s10147-017-1101-6
5. Liebig-Hörl G PCGM. Therapeutische Strategie Bei Frühkarzinomen Im Rektum (T1-Karzinom. Chirurg (2018) 89:358–64. doi: 10.1007/s00104-018-0603-8
6. Lee YJ, Huh JW, Shin JK, Park YA, Cho YB, Kim HC, et al. Risk Factors for Lymph Node Metastasis in Early Colon Cancer. Int J Colorectal Dis (2020) 35:1607–13. doi: 10.1007/s00384-020-03618-7
7. Xie Y, Huang Y, Ruan Q, Wang H, Liang X, Hu Z, et al. Impact of Tumor Site on Lymph Node Status and Survival in Colon Cancer. J Cancer (2019) 10:2376–83. doi: 10.7150/jca.32038
8. Dolan RD, McSorley ST, Horgan PG, McMillan DC. Determinants of Lymph Node Count and Positivity in Patients Undergoing Surgery for Colon Cancer. Medicine (2018) 97:e0185. doi: 10.1097/MD.0000000000010185
9. Foxtrot CG. Feasibility of Preoperative Chemotherapy for Locally Advanced, Operable Colon Cancer: The Pilot Phase of a Randomised Controlled Trial. Lancet Oncol (2012) 13:1152–60. doi: 10.1016/S1470-2045(12)70348-0
10. Huh JW, Lee WY, Shin JK, Park YA, Cho YB, Kim HC, et al. A Novel Histologic Grading System Based on Lymphovascular Invasion, Perineural Invasion, and Tumor Budding in Colorectal Cancer. J Cancer Res Clin (2019) 145:471–7. doi: 10.1007/s00432-018-2804-4
11. Oh PBLS Jr. Nomogram Development and External Validation for Predicting the Risk of Lymph Node Metastasis in T1 Colorectal Cancer. Cancer Res Treat (2019) 4:1275–84. doi: 10.4143/crt.2018.569
12. Vilcea I, Romain B, Rohr S, Burcoş T. Prognostic Factors Analysis for Colon Cancer With Lymph Node Negative. Chirurgia (2018) 113:503–15. doi: 10.21614/chirurgia.113.4.503
13. Zhang Z, Geskus RB, Kattan MW, Zhang H, Liu T. Nomogram for Survival Analysis in the Presence of Competing Risks. Ann Trans Med (2017) 5:403–3. doi: 10.21037/atm.2017.07.27
14. Zhai H, Huang J, Yang C, Fu Y, Yang B. Serum CEA and CA19-9 Levels are Associated With the Presence and Severity of Colorectal Neoplasia. Clin Lab (Heidelberg) (2018) 64:351–6. doi: 10.7754/Clin.Lab.2017.170914
15. Catal O, Ozer B, Sit M. Prediction of Lymph Node Metastasis in Colon Cancer Via Platelet to Lymphocyte Ratio and Platelet Count. J Coll Physicians Surg Pak (2020) 30:250–3. doi: 10.29271/jcpsp.2020.03.250
16. Sun Z, Ma S, Zhou Q, Yang S, Chang Y, Zeng X, et al. Prognostic Value of Lymph Node Metastasis In Patients With T1-stage Colorectal Cancer From Multiple Centers in China. World J Gastroentero (2017) 23:8582–90. doi: 10.3748/wjg.v23.i48.8582
17. Leijssen LGJ, Dinaux AM, Taylor MS, Deshpande V, Kunitake H, Bordeianou LG, et al. Perineural Invasion Is a Prognostic But Not a Predictive Factor in Nonmetastatic Colon Cancer. Dis Colon Rectum (2019) 62:1212–21. doi: 10.1097/DCR.0000000000001450
18. Berger AC, Sigurdson ER, LeVoyer T, Hanlon A, Mayer RJ, Macdonald JS, et al. Colon Cancer Survival Is Associated With Decreasing Ratio of Metastatic to Examined Lymph Nodes. J Clin Oncol (2005) 23:8706–12. doi: 10.1200/JCO.2005.02.8852
19. Wolmark N, FISHER ER. Tumor Size and Regional Lymph Node Metastasis in Colorectal Cancer. Cancer-Am Cancer Soc (1983) 51:1315–22. doi: 10.1002/1097-0142(19830401)51:7<1315::AID-CNCR2820510723>3.0.CO;2-0
20. Chang GJ, Rodriguez-Bigas MA, Skibber JM, Moyer VA. Lymph Node Evaluation and Survival After Curative Resection of Colon Cancer: Systematic Review. JNCI J Natl Cancer Institute (2007) 99:433–41. doi: 10.1093/jnci/djk092
21. Han EC, Kwon Y, Park KJ, Jeong S, Kang S, Oh JH, et al. Significance of Lymph Node Metastasis in the Survival of Stage IV Colorectal Cancer by Hematogenous Metastasis. Ann Surg Treat Res (2018) 95:201–12. doi: 10.4174/astr.2018.95.4.201
22. Weiser MR, Landmann RG, Kattan MW, Gonen M, Shia J, Chou J, et al. Individualized Prediction of Colon Cancer Recurrence Using a Nomogram. J Clin Oncol (2008) 26:380–5. doi: 10.1200/JCO.2007.14.1291
23. Song JH, Yu M, Kang KM, Lee JH, Kim SH, Nam TK, et al. Significance of Perineural and Lymphovascular Invasion in Locally Advanced Rectal Cancer Treated by Preoperative Chemoradiotherapy and Radical Surgery: Can Perineural Invasion Be an Indication of Adjuvant Chemotherapy? Radiother Oncol (2019) 133:125–31. doi: 10.1016/j.radonc.2019.01.002
24. Hu G, Li L, Hu K. Clinical Implications of Perineural Invasion in Patients With Colorectal Cancer. Med (Baltimore) (2020) 99:e19860–0. doi: 10.1097/MD.0000000000019860
25. Alotaibi AM, Lee JL, Kim J, Lim S, Yu CS, Kim TW, et al. Prognostic and Oncologic Significance of Perineural Invasion in Sporadic Colorectal Cancer. Ann Surg Oncol (2017) 24:1626–34. doi: 10.1245/s10434-016-5748-4
26. Ozguven BY, Karacetin D, Kabukcuoglu F, Taskin T, Yener S. Immunohistochemical Study of E-Cadherin and Beta-Catenin Expression in Colorectal Carcinomas. Pol J Pathol (2011) 62:19–24.
27. Meteoglu I, Erdogdu IH, Tuncyurek P, Coskun A, Culhaci N, Erkus M, et al. Matrix Metalloproteinase-1, p53, and Ki-67 Expressions in the Primary Tumors and the Lymph Node Metastases of Colorectal Cancer Cases. GASTROENT Res Pract (2015) 2015:1–9. doi: 10.1155/2015/945392
28. Zeng ZS, Sarkis AS, Zhang ZF, Klimstra DS, Charytonowicz E, Guillem JG, et al. p53 Nuclear Overexpression: An Independent Predictor of Survival in Lymph Node–Positive Colorectal Cancer Patients. J Clin Oncol (1994) 12:2043–50. doi: 10.1200/JCO.1994.12.10.2043
29. Brunner W, Widmann B, Marti L, Tarantino I, Schmied BM, Warschkow R. Predictors for Regional Lymph Node Metastasis in T1 Rectal Cancer: A Population-Based SEER Analysis. Surg Endoscopy (2016) 30:4405–15. doi: 10.1007/s00464-016-4759-3
30. Choi J. Computed Tomography and Magnetic Resonance Imaging Evaluation of Lymph Node Metastasis in Early Colorectal Cancer. World J Gastroentero (2015) 21:556. doi: 10.3748/wjg.v21.i2.556
31. Li M, Zhang J, Dan Y, Yang G, Yao Y, Tong T. Establishment and Validation of Clinical - Imaging Omics Histograms for Preoperative Prediction of Lymph Node Metastasis From Colorectal Cancer. Chin J Cancer (2020) 030:49–56. doi: 10.19401/21j.cnki.1007-3639.2020.01.006
32. Dong D, Fang MJ, Tang L. Deep Learning Radiomic Nomogram can Predict the Number of Lymph Node Metastasis. Ann Oncol (2020) 31:912–20. doi: 10.3748/wjg.v21.i2.556
33. Wu S, Zheng J, Li Y, Wu Z, Shi S, Huang M, et al. Development and Validation of an MRI-Based Radiomics Signature for the Preoperative Prediction of Lymph Node Metastasis in Bladder Cancer. Ebiomedicine (2018) 34:76–84. doi: 10.1016/j.ebiom.2018.07.029
34. Lu X, Wang Y, Jiang L, Gao J, Zhu Y, Hu W, et al. A Pre-Operative Nomogram for Prediction of Lymph Node Metastasis in Bladder Urothelial Carcinoma. Front Oncol (2019) 9:488–500. doi: 10.3389/fonc.2019.00488
35. Zhu M, Cao B, Li X, Li P, Wen Z, Ji J, et al. Risk Factors and a Predictive Nomogram for Lymph Node Metastasis of Superficial Esophagogastric Junction Cancer. J Gastroen Hepatol (2020) 35:1524–31. doi: 10.1111/jgh.15004
Keywords: colon cancer, biomarkers, lymph node metastasis, nomogram, diagnosis
Citation: Xu Y, Chen Y, Long C, Zhong H, Liang F, Huang L-x, Wei C, Lu S and Tang W (2021) Preoperative Predictors of Lymph Node Metastasis in Colon Cancer. Front. Oncol. 11:667477. doi: 10.3389/fonc.2021.667477
Received: 13 February 2021; Accepted: 07 May 2021;
Published: 31 May 2021.
Edited by:
Alessandro Ottaiano, Istituto Nazionale Tumori Fondazione G. Pascale (IRCCS), ItalyReviewed by:
Daniela Rega, Istituto Nazionale Tumori Fondazione G. Pascale (IRCCS), ItalyAndrea Belli, Istituto Nazionale Tumori Fondazione G. Pascale (IRCCS), Italy
Copyright © 2021 Xu, Chen, Long, Zhong, Liang, Huang, Wei, Lu and Tang. This is an open-access article distributed under the terms of the Creative Commons Attribution License (CC BY). The use, distribution or reproduction in other forums is permitted, provided the original author(s) and the copyright owner(s) are credited and that the original publication in this journal is cited, in accordance with accepted academic practice. No use, distribution or reproduction is permitted which does not comply with these terms.
*Correspondence: Yansong Xu, hbxys81@163.com; Weizhong Tang, tangweizhong0771@163.com