- 1Institute of Genetic and Biomedical Research (IRGB), National Research Council (CNR), Sassari, Italy
- 2Institute of Biomolecular Chemistry (ICB), National Research Council (CNR), Sassari, Italy
- 3Department of Medical, Surgical, and Experimental Sciences, University of Sassari, Sassari, Italy
The improvement of the immunotherapeutic potential in most human cancers, including melanoma, requires the identification of increasingly detailed molecular features underlying the tumor immune responsiveness and acting as disease-associated biomarkers. In recent past years, the complexity of the immune landscape in cancer tissues is being steadily unveiled with a progressive better understanding of the plethora of actors playing in such a scenario, resulting in histopathology diversification, distinct molecular subtypes, and biological heterogeneity. Actually, it is widely recognized that the intracellular patterns of alterations in driver genes and loci may also concur to interfere with the homeostasis of the tumor microenvironment components, deeply affecting the immune response against the tumor. Among others, the different events linked to genetic instability—aneuploidy/somatic copy number alteration (SCNA) or microsatellite instability (MSI)—may exhibit opposite behaviors in terms of immune exclusion or responsiveness. In this review, we focused on both prevalence and impact of such different types of genetic instability in melanoma in order to evaluate whether their use as biomarkers in an integrated analysis of the molecular profile of such a malignancy may allow defining any potential predictive value for response/resistance to immunotherapy.
Introduction
The increasing efficacy of immunotherapy with immune checkpoint inhibitors (ICIs) has deeply changed life expectancy for different types of fatal cancer: melanoma, lung cancer, renal carcinoma, advanced squamous cell carcinoma of the head and neck or skin districts, some colorectal cancers, and refractory lymphomas (1–5). At the same time, it is widely recognized that the therapeutic indication of ICI cannot be extended to all subtypes of tumor histology since it has been observed that majority of patients are not responsive (6). Therefore, the identification of biomarkers able to accurately predict either response or resistance to the treatment represents a crucial need in cancer immunotherapy.
Although the introduction into clinical practice of validated immuno-oncological biomarkers is currently limited by the heterogeneity of the types of specimens analyzed, because of the diversity of the used methodologies and the absence of a real sharing of the produced data, it is necessary to continue to support the efforts in conducting biomarker-driven trials (7). In recent years, multidisciplinary approaches have significantly increased the quest for an even more accurate molecular classification through the assessment of the mutational status in multiple oncogenes and tumor suppressor genes; in the immuno-oncological field, such efforts have already produced some approved tests (PD-L1 expression and microsatellite instability rates) and other advanced tests yet to be fully proven for efficacy (tumor mutation load, neoantigen pattern, intratumor T-cell infiltration rate) (5, 8–10).
Toward a holistic approach aimed at implementing precision oncology for treatment of “difficult” human cancers, should evaluation of genetic instability be included into the patients’ molecular classification, probably even for the cancer types—like cutaneous melanoma—with a recognized low prevalence of such an alteration? In supporting a positive answer to this question, it has been recently demonstrated that a detailed tumor molecular profiling with identification of all low-frequency actionable alterations in pancreatic cancer—a definitely difficult-to-treat tumor—may produce a significant benefit from receiving a matched therapy (11). Before moving in this sense, we retain to firstly go through the features bringing to the classification of an unstable genome.
Genetic Instability
The accumulation and fixation of mutations into the genome, both in the transcribed or regulatory sequences and in those apparently inactive, is one of the most important ways through which evolution is carried out (12). Excluding mutations having deleterious effects with functional consequences, the great majority of sequence variants often display an undefined role (neither harmful nor beneficial) in disease pathogenesis (13). These apparently neutral genetic variants can spread and become fixed in a population, making a large contribution to the evolutionary change in genomes. Focusing on single individuals, the establishment of germinal mutations or the accumulation of somatic mutations can lead to serious cell dysfunctions. Figure 1 represents the main mechanisms inducing the increase of the mutations’ content in cancer cells.
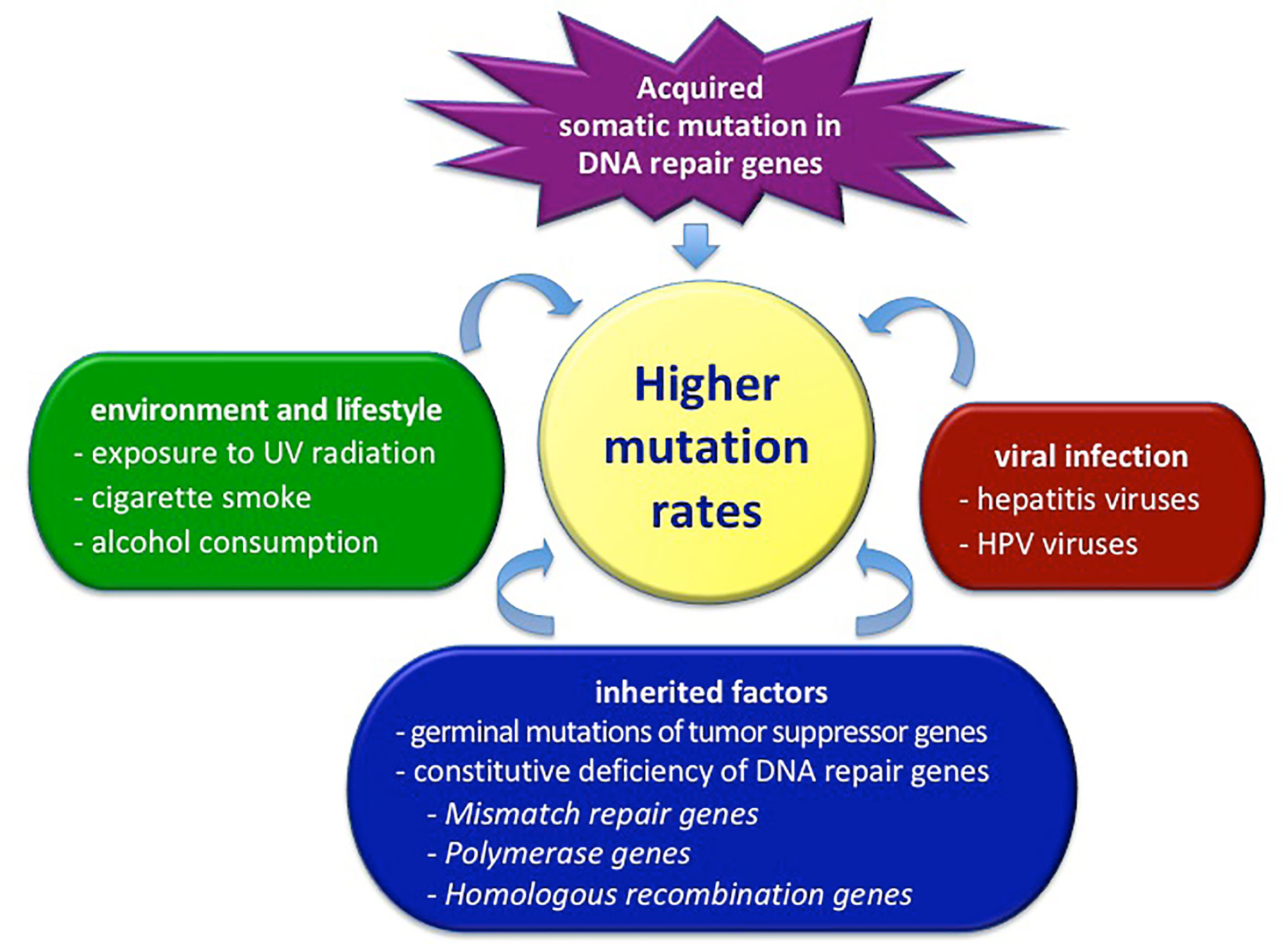
Figure 1 Factors determining the total level of mutations in cancer cells. HPV, human papilloma virus; UV, ultraviolet radiation.
An accurate and articulated system of control and repair of genomic DNA integrity has evolved into the cells (14, 15). The DNA damage can be caused by genetic instability that may exist at two distinct mechanistic levels. In most cases, genomic instability is observed at the chromosomal level as whole chromosome or segmental/focal aneuploidy; in a more limited fraction of tumors, instability is observed at the nucleotide level and is revealed by the presence of alterations in particular highly repeated DNA sequences with a uniform nucleotide composition, the satellite DNA loci (16, 17). Such satellite DNA regions are classified as minisatellite or microsatellite DNA, depending on the length of the repeated sequences (18–21). Minisatellites consist of repetitive motifs that range in length from 10 to over 100 base pairs. They are located mainly at the centromeres and at the sub-telomeric and telomeric chromosome regions (telomeres itself are constituted by tandem repeats). Minisatellites may play a role in modifying levels of transcription, alternative splicing, or imprinting changes; therefore, they can participate in cell functioning as regulators of gene expression (18, 19, 22). Microsatellites consist of tandem repeats of 1 to 6 base pairs, often organized in long strings, which are subject to mutational events such as insertions and deletions (18, 19, 21).
Aneuploidy—which is due to a genomic imbalance in terms of gain or loss of chromatid or chromosome regions—can be actually classified as a somatic copy number alteration (SCNA), being demonstrated to play a critical role during the process of tumorigenesis and prognosis (23). Occurrence of aneuploidy/SCNA seems to contribute to immune evasion through the reduction of a cytotoxic immune infiltrate into the tumor microenvironment (TME); on this regard, TME can be immunosuppressive per se, facilitating tumor progression through mobilization of cytokines, chemokines, and inhibitory factors (24). Moreover, the TME can also recruit immunosuppressive immune cells including regulatory T cells (TREGs), myeloid-derived suppressor cells (MDSCs), and tumor-associated macrophages (TAMs) to evade immune clearance (25). The aneuploid status may potentiate the immunosuppressive TME activity by also negatively interfering with the presentation of the antigens of the major histocompatibility complex (MHC), which represents a fundamental moment into the recognition of the tumor by the immune system (26). The content of peptide neoantigens seems to vary based on the levels of tumor SCNAs, with a relative concentration that is significantly lower in aneuploid tumors than diploid ones acting in an opposite way from the increased overall mutation load and correspondent tumor neoantigen expression levels, which are both positively correlated with the induction of cytotoxic immune infiltrates (27).
Microsatellite instability (MSI) seems to be usually due to deficient DNA damage repair; it has been associated with promotion of a higher load of tumor mutations (28, 29). The MSI occurrence (MSI+) is subsequent to impairment of at least one main gene regulating the different DNA repair mechanisms: homologous recombination (involving BLM, BRCA1/2, BRIP1, PALB2, RAD50/51, Fanconi Anemia genes), mismatch repair (MLH1, MSH2, MSH6, PMS2), cell cycle checkpoints (ATM, CHEK1/2), base excision repair (POLE) (30, 31). A high tumor mutation burden (TMB-high) is generally defined as the >10–20 mutations per megabase of genomic area (threshold is deeply varying according to the cancer type) and can somehow act as a surrogate marker of the neoantigen load (32–34). Tumor specific peptide epitopes, which are usually absent in the normal human genome, can be recognized and targeted by the immune system (33–35). Both MSI+ and TMB-high have been both associated with favorable outcome to ICI therapy in some cancer types (33, 34, 36), but their role in predicting overall survival is still controversial. Vast majority of MSI+ samples present with TMB-high (83%), but the converse is not true, since only 16% of samples with TMB-high are classified as MSI+ (37).
Overall, next-generation sequencing (NGS) analysis through a whole genome or exome screening is being used for detecting the high-level SCNAs, the MSI+ status, and the TMB-high in tumor tissues. The MSI+ and TMB-high conditions have been associated with the long-term response to ICI treatment in different human malignancies—including melanoma, lung and renal/bladder cancer, head and neck squamous cell carcinoma (38–45). Conversely, occurrence of aneuploidy/SCNA negatively correlates with the presence of a favorable immune signature, conferring resistance to ICI treatment (26). Figure 2 summarizes the effects exerted by the different conditions on the activity of the immune system.
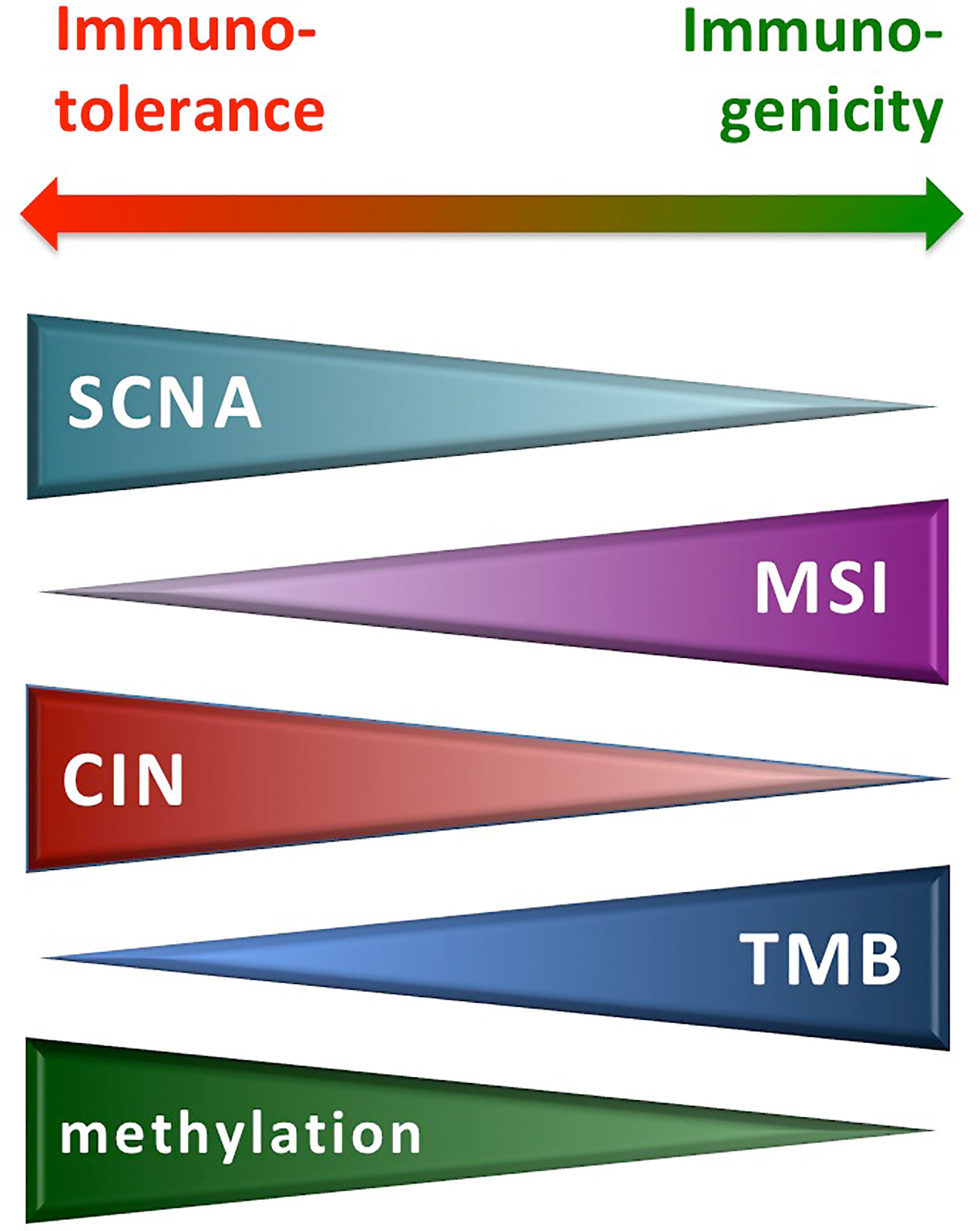
Figure 2 Molecular alterations from genetic instability and immune reactivity. CIN, chromosomal instability; MSI, microsatellite instability; SCNA, somatic copy number alteration; TMB, tumor mutation burden.
Although additional factors are involved in augmenting the adaptive immunity under ICI therapy—such as the histocompatibility leukocyte antigen (HLA) evolution pattern and tumor-infiltrating lymphocyte (TIL) reactivity (27), the simultaneous assessment of the SCNA burden and the rates of TMB and MSI in tumor tissue sections might be strongly useful for classifying patients who are more or less likely to respond to immunotherapies (46). Despite such recognized predictive values, the NGS-based test was not yet routinely included in clinics due to the required high level of technical expertise, the lack of standardization, the high cost, and the pretty-long time required to perform an extensive genomic screening (47, 48). Recently, the combination of reducing the costs of NGS technologies and developing large but manageable multi-gene panels has contributed to facilitate continuous implementations for the use of NGS-based assays in daily clinical practice (49). In other words, the aim of simplifying the sequencing of multiple genes per tumor sample, in order to detect targetable genomic alterations, is becoming a reality and NGS is presenting a really good analytical validity, with an increasingly favorable cost–benefit ratio. To achieve the most currently accurate molecular classification for guiding treatment decisions among cancer patients, recommendations on how multi-gene NGS assays should be used to profile human tumors for improving patients’ management are being provided by scientific societies (50).
Aneuploidy: Mechanism and Effects
Aneuploidy can be mostly considered as the result of the impairment of the cell cycle checkpoints, which consist of mechanisms that verify DNA replication accuracy and control the cell cycle progression, detecting errors in DNA repair, DNA synthesis, and chromosome segregation (51). Occurrence of structural alterations significantly affecting the genome integrity constitutes a signal sent to the replication/segregation machinery in order to repair the damage (52).
Several cyclin-dependent kinases (CDKs) physiologically drive cell division and regulate the different phases of the cell cycle through phosphorylation of a complex network of substrates and activation of cascades of transduction signals (53). In case of genomic DNA damage, the cell cycle checkpoints arrest the G1/G2 and G2/M transitions by repressing the CDK activity. Hyperactive CDKs, caused by mutations in genes controlling the DNA damage response pathway, lead to the progression into the cell cycle and cell survival (52). On this regard, inactivating mutations in TP53 gene have a permissive role, strongly contributing to the propagation of genetic errors in descendant daughter cells (54). As consequence, deregulation of the TP53-driven pathway—also including impairment of the activity of its downstream effectors (i.e., RB1)—contributes to aneuploidy (55). A number of cancers with mutated TP53 are chromosomal stable and show MSI+, whereas TP53 loss-of-function is predominant in non-hypermutated tumors (54–56). Indeed, the TP53 inactivation is mostly dependent on whether or not mutations in this gene affect the function of p53 on repressing the activity of the Cyclin D1–CDK2 system controlling centrosome duplication and preventing aneuploidy (53).
Activating mutations in oncogenes (such as CCND1, EGFR, PIK3CA, KRAS, BRAF) and inactivating changes in tumor suppressor genes—like RB1, APC, and WNT signaling pathway components (CHK1 and CHK2-BRCA1)—can dramatically enhance cell proliferation and increase the replication stress levels, causing double-strand breaks in the DNA, with consequent genomic instability that affects tumor progression (57). This seems due to the fact that the unbalanced activity of the driver genes involved in promotion of cell proliferation and survival leads to a sort of oncogene-induced mitotic stress status (58). The enormous variation of segregation errors among different malignancies is indeed a strong indicator that mitotic events act as important players in aneuploidy occurrence (59). Deregulation of the centrosome duplication may indeed promote the formation of multiple centrosomes, which in turn leads to multipolar spindles and aneuploidy (58, 59). Molecular alterations favoring instability of centromeres can thus lead to chromosome segregation defects.
Actually, assessment of aneuploidy is mostly based on measuring SCNA rates in malignancies through bioinformatics approach, the allele-specific copy number analysis of tumors (ASCAT), using data generated by whole-genome/exome sequencing strategies (60). The rates of intratumor karyotype heterogeneity can accurately be determined by simultaneous estimation of the allele-specific total copy number after adjusting for both tumor ploidy—including gains, losses, copy number-neutral events, and loss of heterozygosity (61).
Individual chromosome arm-level alterations were found to be related to expression changes in immune and cell-cycle markers, independent of aneuploidy level; however, increased arm- and chromosome-level SCNA burdens were associated with proliferation signatures and immune evasion profiles (62). Moreover, tumor aneuploidy is likely to increase intratumor heterogeneity, which may inhibit tumor immunity (63). Many solid cancers presenting with a high somatic copy alteration burden exhibit features of immune exclusion, whereas tumors displaying low rates of aneuploidy present an immune active profile (26, 27, 64). High-level SCNAs are classified through bioinformatic approaches as events where focal copy number gain (or loss) are higher (or lower) than the maximum (or minimum) median arm-level copy number gain (or loss), hence avoiding artifacts or false positives after comparison with low-level SCNAs linked to the ploidy of tumor samples and thus obtaining more reliable thresholds (65, 66). High-level SCNA profile in activating beta-catenin signaling pathway elements including CTNNB1, APC, and AXIN1-2 genes has been reported in metastatic melanoma but not in primary melanoma (67). A significantly higher concordance between mutated SCNA profiles in beta-catenin signaling pathway activated samples with a low level of T-cell tumor inflammation has been demonstrated, thus suggesting that SCNA signature may act as a progression marker in advanced melanoma (67). For its prediction of the T-cell-inflamed gene expression signature, the SCNA score is worth to be included in molecular tests aimed at somehow anticipating probabilities of resistance to immunotherapies. Further supporting this, the SCNA level has been found lower in lung cancer patients with a responsive disease than those with stable or progressive disease under ICI treatment (68).
Finally, SCNAs can be intrinsically linked to complex structural variants (CSVs) in affecting the efficacy of ICI treatment in melanoma. In particular, CSVs—which are represented by deletions, duplications, translocations, or inversions and arise through the breakage and fusion of one or two genomic locations—are particularly reported in acral melanoma (69). In bioinformatic analysis of NGS-generated data, SCNAs and CSVs are detected as changes in sequencing read depth and in junction-spanning read pairs across the candidate genomic loci (70).
Microsatellite Instability
MSI is characterized by small insertions or deletions within short tandem repeats in tumor DNA when compared with the corresponding normal DNA. In other words, regions that contain sequences of repeated nucleotides are intrinsically unstable and the insertion of inappropriate nucleotide(s) or the slippage events during DNA replication give rise to the insertion or deletion of single bases or small tandem DNA sequences (56). These alterations, which are normally recognized and repaired, in the absence of an efficient MMR function, are maintained giving origin to alleles of different sizes during the successive replication cycles. The accumulation of unpaired alleles is at the basis of such a genome-wide genetic instability, which is recognized as MSI+ phenotype and observed at higher prevalence in gastrointestinal and endometrial cancers (37, 44, 56, 71). Table 1 report frequencies of MSI+ in different tumor types, as inferred taking into the consideration the main published studies (72–76).
In colorectal carcinoma (CRC), the MSI+ phenotype has been long evaluated for its impacts on disease pathogenesis and behavior as well as for correlations with prognostic effects. While some distinct clinical and pathological features (proximal location, poor differentiation, mucinous histology) have been consistently associated with the occurrence of MSI, more controversial data have been produced on the prognostic role of this alteration (77). In early stage CRC, the MSI+ phenotype has been described in patients with a better prognosis; conversely, detection of unstable microsatellites seems to confer a negative prognosis in patients with metastatic disease (77–79).
MSI reflects a defect in genes involved in DNA replication fidelity and mostly, is due to inactivation of the mismatch repair (MMR) genes (29, 31). The MMR genes may be impaired by inactivating or down-regulating genetic mutations as well as by gene-silencing epigenetic changes (80). The result of such alterations is the expression of normal levels of functionally deficient MMR proteins or lack of the MMR protein expression, both conditions progressively inducing genetic instability and somehow providing a selective advantage during neoplastic transformation and progression (80). The important components of the DNA mismatch repair system are represented by seven specific ATP-binding proteins that work coordinately in sequential steps to initiate repair of DNA mismatches in genomic DNA: MLH1, MSH2, MLH3, MSH3, MSH6, PMS2, and PMS1 (81). Inactivation of MLH1 and MSH2 was detected in more than 85% of the MSI+ tumors (80, 81). Nearly all MMR genes contain a mononucleotide repeat and thus represent the first target of inactivating mutations when the MSI+ phenotype coexists (71).
The real breakthrough in defining a more impacting role of the MSI in the clinic practice for the management of neoplastic patients has been registered in 2017, when the U.S. Food and Drug Administration (FDA) granted approval of an immune checkpoint inhibitor (the anti-PD-1 pembrolizumab) for treatment of patients with cancers carrying MSI or deficient-MMR (82). The approval by FDA of the anti-PD-1 treatment for all advanced MSI+ solid tumors still represents the first regulatory authorization based exclusively on the use of a specific biomarker, regardless of the anatomic location in the body where the tumor originated (“tumor agnostic”) (83). The MSI and the mutation load underlie the response to PD-1 blockade immunotherapy in deficient-MMR human tumors; the extent of response seems to be particularly associated with the accumulation of insertion-deletion (indel) mutational load (84). In a recent meta-analysis of patients with MSI+ cancer, the ICI treatment was significantly confirmed to be associated with high activity independent of tumor type and drug used and MSI status assessment may have a predictive value for the selection of patients to be addressed to immunotherapy (85).
Epigenomic studies have shown that tumors with MSI exhibit hypermethylation of key genes implicated in tumor development (75, 86). The hypermethylated promoters were identified in some genes that regulate some main molecular signaling cascades (75, 76, 87): WNT (in the absence of WNT-signals, β-catenin—a key downstream effector of this pathway—is targeted for degradation through phosphorylation; the WNT signals thus stabilize the intracellular levels of β-catenin and subsequently increase transcription of downstream target genes in many human cancers), hedgehog (essential for embryonic and postnatal development, this pathway remains in the quiescent state in adult tissues but gets activated upon inflammation and injuries), and PTEN (its inactivation through mixed genetic/epigenetic mechanisms results in persistent activation of PI3K effectors, with an important impact on cell proliferation, apoptosis resistance, angiogenesis, metabolism regulation, genomic instability, cellular senescence, and cell migration). The hypermethylated status is also tightly correlated with the occurrence of somatic mutations in BRAF oncogene, overall causing a strong inhibition of the senescence mechanisms and a consequent promotion of an uncontrolled cell proliferation and survival (88, 89). Hypermethylation has also been related to the facilitation of tumor escape by repressing transcriptional expression of interferon (IFN) regulatory factors (90). Indeed, demethylating agents and histone deacetylases are being combined with ICI treatments in numerous clinical trials and types of malignancies (91, 92).
Several additional factors, other than those mainly underlying MSI, have been shown to be involved in determining a hyper-mutated status, such as inactivating mutations in the DNA polymerases as well as exposure to external (cigarette smoke, UV radiation, chemicals) and endogenous (reactive oxygen species) mutagens (93, 94). The hypermutated condition may be related to driver mutations in the DNA polymerase ϵ (POLE) and δ1 (POLD1) genes among different tumor types, including colorectal, endometrial, and other cancers such as melanoma and lung cancer (95, 96). Deleterious mutations in POLE/POLD1 genes compromise proofreading of genomic DNA during cell replication and the timing of their onset may vary, with constitutional defective MMR followed by acquired secondary POLE/POLD1 defects or vice versa (97). It has been shown that the presence of mutations in POLE may promote a high level of non-synonymous single-nucleotide variations (ns-SNVs), not tightly associated with the presence of a MSI+ phenotype (the highest mutation rates were observed in MSS tumors) (71). The POLD1 gene has been found silenced in several cancer types—mostly, in conjunction with a defective POLE gene—with increased genome instability and DNA damage effects (98–100). POLD1 is involved in different forms of DNA repair induced by exposure to mutagens, including nucleotide excision repair, double strand break repair, base excision repair, and mismatch repair (101). The coexistence of MSI+ and mutated POLE may be associated with higher densities of CD8+ TILs, PD-1-expressing CD8+ TILs, and tumor-infiltrating immune cells with a Th1 phenotype in the TME, strongly predicting response to checkpoint inhibitors (102).
As mentioned above, tumors with the hypermutated status present similar sensitivity to ICI. Indeed, a strong correlation was found between increased load of non-synonymous mutations and clinical benefits to PD-1 inhibition in non-small cell lung cancer (39) or to cytotoxic T-lymphocyte antigen T 4 (CTLA-4) blockade in melanoma (103). Considering such reported outcomes, one can speculate that increased production of neoepitopes predicting response to ICI might be even generated in cohorts of patients with low (<10% of case) or very low (<1%) prevalence of MSI (Table 1).
The hypermutated status can be actually defined with more extensively detailed approaches such as NGS or mass spectrometry assays (104). Among strategies not requiring to match normal DNA material, the single-molecule molecular inversion probe (smMIP) assay is able to detect the existence of an impaired intracellular capability of correcting smMIP-induced errors (105). All these screening strategies are useful in a research context, but technically difficult to translate into clinical practice for routine diagnostic application, since either requiring an extensive bioinformatics analysis of the obtained results either remaining still expensive methods (48—50). Conversely, a simple method to directly detect MSI on formalin-fixed paraffin embedded tumor tissue sections is represented by the Idylla™ test, a fully automated PCR-based assay including a high-resolution melting curve analysis. The Idylla™ MSI test is able to detect mutations in seven tumor-specific MSI loci (ACVR2A, BTBD7, DIDO1, MRE11, RYR3, SEC31A, and SULF2), not requiring the analysis of paired normal tissue samples. For more extensive and detailed information about the methodologies aimed at investigating the MSI status, one can refer to the recent report from our group (106, 107).
The contextual assessment of the MSI+ phenotype and the hypermutated status may be strongly indicative for the existence of a higher tumor immunogenicity, though none of the alterations described as immediate biological effects of the MSI+ phenotype and the hypermutated status—the mutation load, the neoantigen prediction, and the intratumor immune cell infiltration rate—may be considered as a reliable predictor of response to anti-PD-1 treatment (108). Several additional molecular factors are suggested to be involved in immune response. Occurrence of mutations inactivating JAK1—within the JAK-STAT pathway that regulates different cellular processes—has been reported to confer resistance to the anti-PD-1 treatment by reducing both the PD-L1 expression and the ability to promote the IFN-γ driven response (109, 110). The relationship between such JAK1 mutations and MSI status is however complex. In patients with tumors characterized by a low prevalence of MSI—including cutaneous melanoma, invasive breast cancer, and prostate adenocarcinoma—deleterious JAK1 mutations are associated with unfavorable prognosis (109, 110). In MSI+ tumors, JAK1 silencing seems to instead impair the tumor growth, playing a positive prognostic role (109, 110). This further confirms that often the same molecular alterations occurring in different tumor types have a distinct impact on biological behavior according to the different genetic backgrounds.
Classification of Melanoma Patients for Genetic Instability
According to their mutational status inferred by NGS analysis at somatic level, one could classify melanoma patients using:
-“qualitative” parameters, aimed at discriminating all classes of sequence changes or structural alterations (non-synonymous single-nucleotide variants/ns-SNVs, indels, copy number variations/CNVs, fusions, and splice variants) in tumor suppressor genes and/or oncogenes. These alterations occur at high frequency in melanoma samples. Research efforts should be aimed at defining the clinical role of the distinct mutational patterns of driver ns-SNVs as well as whether the increased load may rather represent the consequence of the sequential accumulation of “passenger” mutations in specific pathways during disease progression;
-“quantitative” parameters, aimed at defining the above described threshold-depending parameters representing the main immuno-oncology content (SCNA, MSI, and TMB). These alterations occur at low frequency in melanoma samples (Figure 3).
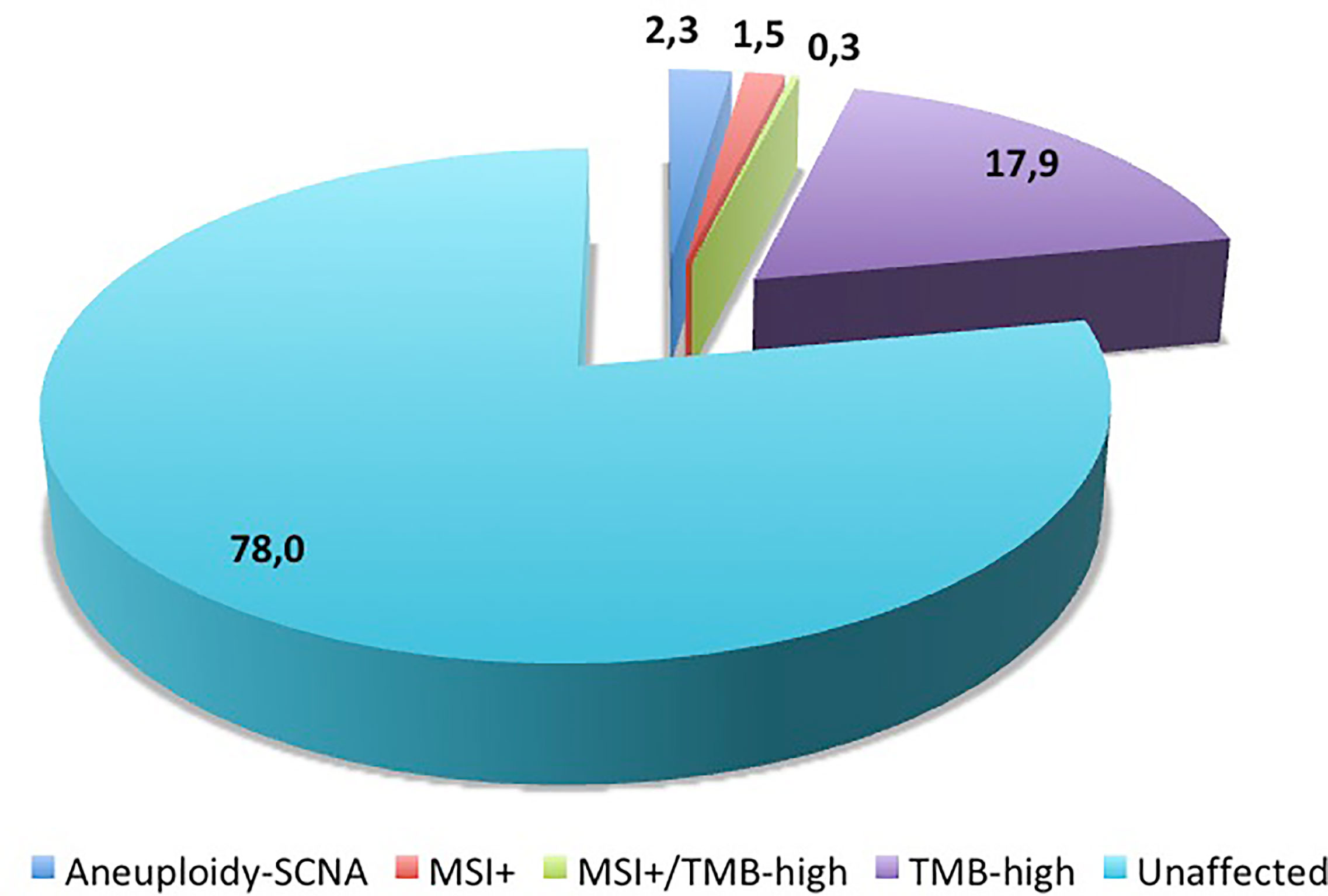
Figure 3 Distribution of molecular alterations linked to genetic instability in melanoma samples. Numbers indicate the percentages of cases reported in literature (see text for references).
Most of such key features are actually achieved using large NGS-based panels, which usually include over 400 unique driver genes in correspondent genomic loci for the achievement of a comprehensive and simultaneous genomic profiling (Table 2).
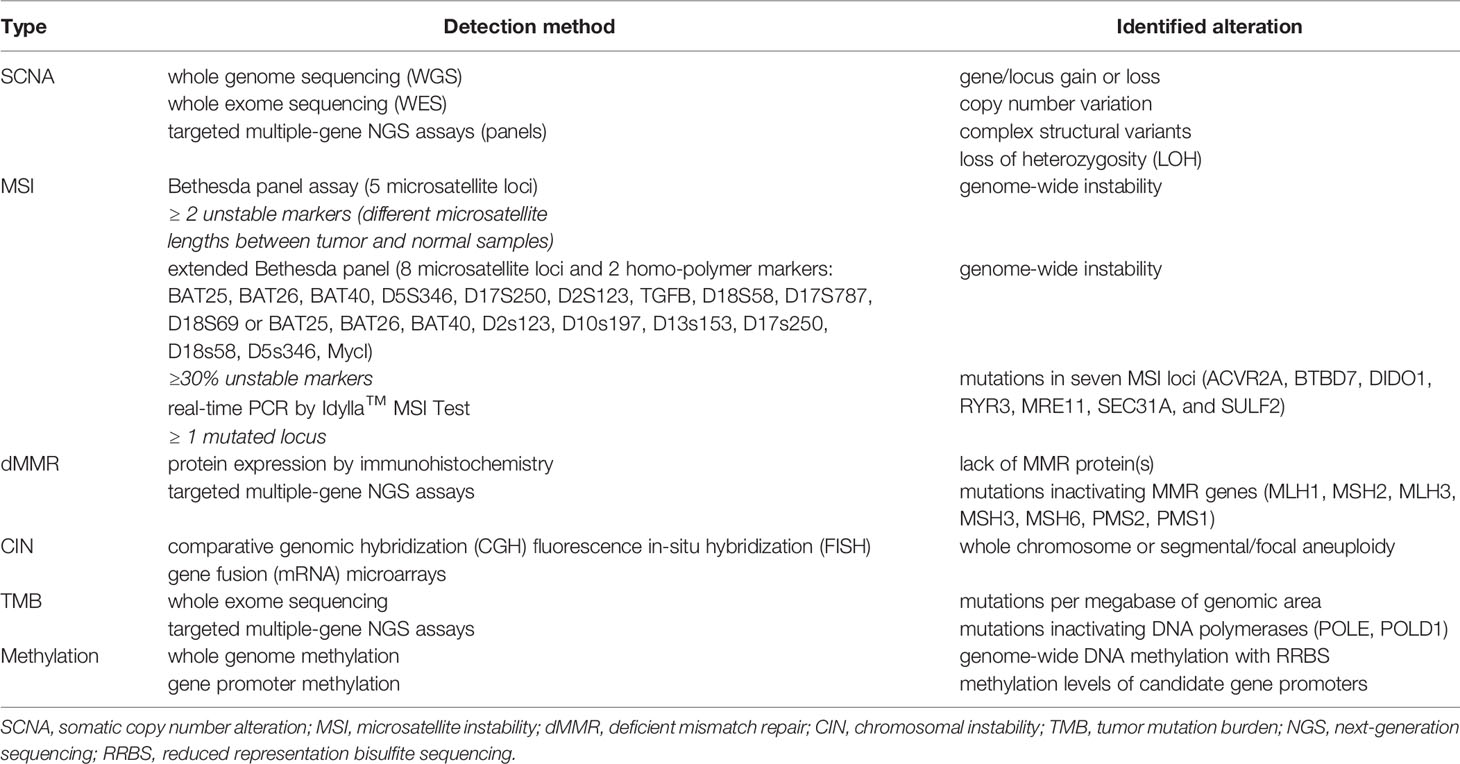
Table 2 Molecular alterations underlying genetic instability useful in cancer patients’ stratification for immunotherapy.
MSI Detection on Liquid Biopsies
In cancer patients, the assessment of PD-L1 status in circulating tumor cells (CTC) and the determination of specific somatic mutations in circulating tumor DNA (ctDNA) represent non-invasive tools acting as predictive markers of the efficacy of the therapeutic response to ICI. The technology for CTC isolation is not widely available, whereas genomic analyzes on ctDNA are methodologically feasible. In NSCLC, undetectable ctDNA levels after two months of ICI were demonstrated to be associated with a marked and lasting response to therapy, while an increase in ctDNA load after initiation of ICI was associated with poorer survival (111, 112). In melanoma, detectable ctDNA at baseline and post-surgical tumour removal may predict a shorter median disease-specific survival among stage III melanoma patients (113, 114) as well as detection of persistent or increasing ctDNA levels during follow-up was shown to predict worse prognosis when compared to patients with undetectable or falling ctDNA levels (115, 116). Currently, plasma-based commercially available assays (“liquid biopsies”) can be used to assess the MSI or the mismatch repair deficiency (dMMR) through genomic analysis by realt-time PCR or DNA sequencing assays in a large variety of cancer types (117–119). From the practical point of view, the real-time PCR is mainly based on the Idylla™ MSI assay (Biocartis, Bruxelles, Belgium; catalog n. A0101/6), which includes a set of seven MSI biomarkers consisting of short homo-polymers located in the above mentioned genes. The NGS tests on ctDNA are performed using complex multigene panels (i.e. the Oncomine Comprehensive Assay Plus panel, which provides highly multiplexed target selection of >400 genes implicated in cancer pathogenesis, carried out on the Ion GeneStudio S5 System) (120). These NGS-based tests are now feasible in clinical practice and they have very high concordance, sensitivity and specificity and a detection limit of 0.1% tumor content for MSI-H status. Moreover, such panels allow identification of further genomic alterations (i.e. the tumor mutation burden or TMB) with potential implications for predicting response to immunotherapy.
Conclusive Remarks
Considering the steadily increasing advances in the knowledge of the molecular mechanisms underlying the genetic instability at the chromosomal and nucleotide levels as well as the recognized ascertainment of their clinical impact on cancer management, selection of the subgroups of patients according to the type of instability (SCNA+ vs. SCNA−, MSI+ vs. MSI−) or mutational composition (TMB-high vs. TMB-low; neoantigen-high vs. neoantigen-low) present is becoming mandatory. Further advancements will be however achieved by increasing correlations between such molecular features—through a continuous dissemination of the methodologies to be used for their assessment into the clinical practice—and all disease-related and therapy-dependent parameters. These efforts should facilitate the development of innovative diagnostic, predictive, and/or prognostic tools for a better molecular classification of cancer patients, even in a malignancy like melanoma with lower rates of such alterations. Nevertheless, more extensive applications of the NGS technologies could improve the assessment of all driver alterations putatively acting as disease markers to be transferred into the daily clinical practice.
Author Contributions
All authors contributed to the conception, design, and writing of the manuscript. All authors contributed to the article and approved the submitted version.
Funding
This work was funded by the Fondazione AIRC “Programma di ricerca 5 per Mille 2018- ID#21073” to EPigenetic Immune-oncology Consortium Airc (EPICA) investigators.
Conflict of Interest
The authors declare that the research was conducted in the absence of any commercial or financial relationships that could be construed as a potential conflict of interest.
References
1. Fusi A, Dalgleish A. The Importance for Immunoregulation for Long-Term Cancer Control. Future Oncol (2017) 13(18):1619–32. doi: 10.2217/fon-2017-0085
2. Gelsomino F, Lamberti G, Parisi C, Casolari L, Melotti B, Sperandi F, et al. The Evolving Landscape of Immunotherapy in Small-Cell Lung Cancer: A Focus on Predictive Biomarkers. Cancer Treat Rev (2019) 79:101887. doi: 10.1016/j.ctrv.2019.08.003
3. Kok PS, Cho D, Yoon WH, Ritchie G, Marschner I, Lord S, et al. Validation of Progression-Free Survival Rate At 6 Months and Objective Response for Estimating Overall Survival in Immune Checkpoint Inhibitor Trials: A Systematic Review and Meta-Analysis. JAMA Netw Open (2020) 3(9):e2011809. doi: 10.1001/jamanetworkopen.2020.11809
4. Hu X, Yu H, Zheng Y, Zhang Q, Lin M, Wang J, et al. Immune Checkpoint Inhibitors and Survival Outcomes in Brain Metastasis: A Time Series-Based Meta-Analysis. Front Oncol (2020) 10:564382. doi: 10.3389/fonc.2020.564382
5. Wu X, Gu Z, Chen Y, Chen B, Chen W, Weng L, et al. Application of PD-1 Blockade in Cancer Immunotherapy. Comput Struct Biotechnol J (2019) 17:661–74. doi: 10.1016/j.csbj.2019.03.006
6. Datta M, Coussens LM, Nishikawa H, Hodi FS, Jain RK. Reprogramming the Tumor Microenvironment to Improve Immunotherapy: Emerging Strategies and Combination Therapies. Am Soc Clin Oncol Educ Book (2019) 39:165–74. doi: 10.1200/EDBK_237987
7. Ascierto PA, Bifulco C, Palmieri G, Peters S, Sidiropoulos N. Preanalytic Variables and Tissue Stewardship for Reliable Next-Generation Sequencing (NGS) Clinical Analysis. J Mol Diagn (2019) 21(5):756–67. doi: 10.1016/j.jmoldx.2019.05.004
8. Grasso CS, Giannakis M, Wells DK, Hamada T, Mu X, Quist M, et al. Genetic Mechanisms of Immune Evasion in Colorectal Cancer. Cancer Discovery (2018) 8:730–49. doi: 10.1158/2159-8290.CD-17-1327
9. Nava Rodrigues D, Rescigno P, Liu D, Yuan W, Carreira S, Lambros MB, et al. Immunogenomic Analyses Associate Immunological Alterations With Mismatch Repair Defects in Prostate Cancer. J Clin Invest (2018) 128:4441–53. doi: 10.1172/JCI121924
10. Travert C, Barlesi F, Greillier L, Tomasini P. Immune Oncology Biomarkers in Lung Cancer: An Overview. Curr Oncol Rep (2020) 22(11):107. doi: 10.1007/s11912-020-00970-3
11. Pishvaian MJ, Blais EM, Brody JR, Lyons E, DeArbeloa P, Hendifar A, et al. Overall Survival in Patients With Pancreatic Cancer Receiving Matched Therapies Following Molecular Profiling: A Retrospective Analysis of the Know Your Tumor Registry Trial. Lancet Oncol (2020) 21(4):508–18. doi: 10.1016/S1470-2045(20)30074-7
12. Xue C, Chen H, Yu F. Base-Biased Evolution of Disease-Associated Mutations in the Human Genome. Hum Mutat (2016) 37(11):1209–14. doi: 10.1002/humu.23065
13. Albert FW, Kruglyak L. The Role of Regulatory Variation in Complex Traits and Disease. Nat Rev Genet (2015) 16(4):197–212. doi: 10.1038/nrg3891
14. Scott SP, Pandita TK. The Cellular Control of DNA Double-Strand Breaks. J Cell Biochem (2006) 99(6):1463–75. doi: 10.1002/jcb.21067
15. Hustedt N, Durocher D. The Control of DNA Repair by the Cell Cycle. Nat Cell Biol (2016) 19(1):1–9. doi: 10.1038/ncb3452
16. Giam M, Rancati G. Aneuploidy and Chromosomal Instability in Cancer: A Jackpot to Chaos. Cell Div (2015) 10(3):1–12. doi: 10.1186/s13008-015-0009-7
17. Vodicka P, Musak L, Vodickova L, Vodenkova S, Catalano C, Kroupa M, et al. Genetic Variation of Acquired Structural Chromosomal Aberrations. Mutat Res (2018) 836(Pt A):13–21. doi: 10.1016/j.mrgentox.2018.05.014
18. Lengauer C, Kinzier KW, Volgestein B. Genetic Instabilities in Human Cancers. Nature (1998) 396:643–49. doi: 10.1038/25292
19. Catasti P, Chen X, Mariappan SV, Bradbury EM, Gupta G. DNA Repeats in the Human Genome. Genetics (1999) 106(1-2):15–36. doi: 10.1023/A:1003716509180
20. Schlotterer C. Evolutionary Dynamics of Microsatellite DNA. Chromosome (2000) 109:365–71. doi: 10.1007/s004120000089
21. Toth G, Gaspari Z, Jurka J. Microsatellites in Different Eukaryotic Genomes: Survey and Analysis. Genome Res (2000) 10:967–81. doi: 10.1101/gr.10.7.967
22. Bois P, Jeffreys AJ. Minisatellite Instability and Germline Mutation. Cell Mol Life Sci (1999) 55(12):1636–48. doi: 10.1007/s000180050402
23. Orr B, Godek KM, Compton D. Aneuploidy. Curr Biol (2015) 25:R538–42. doi: 10.1016/j.cub.2015.05.010
24. O’Donnell JS, Teng MWL, Smyth MJ. Cancer Immunoediting and Resistance to T Cell-Based Immunotherapy. Nat Rev Clin Oncol (2019) 16:151–67. doi: 10.1038/s41571-018-0142-8
25. Xiao Q, Nobre A, Piñeiro P, Berciano-Guerrero MÁ, Alba E, Cobo M, et al. Genetic and Epigenetic Biomarkers of Immune Checkpoint Blockade Response. J Clin Med (2020) 9(1):286. doi: 10.3390/jcm9010286
26. Davoli T, Uno H, Wooten EC, Elledge SJ. Tumor Aneuploidy Correlates With Markers of Immune Evasion and With Reduced Response to Immunotherapy. Science (2017) 355:eaaf8399. doi: 10.1126/science.aaf8399
27. Litchfield K, Reading JL, Puttick C, Thakkar K, Abbosh C, Bentham R, et al. Meta-Analysis of Tumor- and T Cell-Intrinsic Mechanisms of Sensitization to Checkpoint Inhibition. Cell (2021) 184(3):596–614. doi: 10.21203/rs.3.rs-76468/v1
28. Salem ME, Puccini A, Grothey A, Raghavan D, Goldberg RM, Xiu J, et al. Landscape of Tumor Mutation Load, Mismatch Repair Deficiency, and PD-L1 Expression in a Large Patient Cohort of Gastrointestinal Cancers. Mol Cancer Res (2018) 16(5):805–12. doi: 10.1158/1541-7786.MCR-17-0735
29. Ballhausen A, Przybilla MJ, Jendrusch M, Haupt S, Pfaffendorf E, Seidler F, et al. The Shared Frameshift Mutation Landscape of Microsatellite-Unstable Cancers Suggests Immunoediting During Tumor Evolution. Nat Commun (2020) 11(1):4740. doi: 10.1038/s41467-020-18514-5
30. Budczies J, Seidel A, Christopoulos P, Endris V, Kloor M, Győrffy B, et al. Integrated Analysis of the Immunological and Genetic Status in and Across Cancer Types: Impact of Mutational Signatures Beyond Tumor Mutational Burden. Oncoimmunology (2018) 7(12):e1526613. doi: 10.1080/2162402X.2018.1526613
31. Marmorino F, Boccaccino A, Germani MM, Falcone A, Cremolini C. Immune Checkpoint Inhibitors in pMMR Metastatic Colorectal Cancer: A Tough Challenge. Cancers (Basel) (2020) 12(8):2317. doi: 10.3390/cancers12082317
32. Chalmers ZR, Connelly CF, Fabrizio D, Gay L, Ali SM, Ennis R, et al. Analysis of 100,000 Human Cancer Genomes Reveals the Landscape of Tumor Mutational Burden. Genome Med (2017) 9(1). doi: 10.1186/s13073-017-0424-2
33. Samstein RM, Lee CH, Shoushtari AN, Hellmann MD, Shen R, Janjigian YY, et al. Tumor Mutational Load Predicts Survival After Immunotherapy Across Multiple Cancer Types. Nat Genet (2019) 51(2):202–6. doi: 10.1038/s41588-018-0312-8
34. Sha D, Jin Z, Budczies J, Kluck K, Stenzinger A, Sinicrope FA. Tumor Mutational Burden as a Predictive Biomarker in Solid Tumors. Cancer Discovery (2020) 10(12):1808–25. doi: 10.1158/2159-8290.CD-20-0522
35. Schumacher TN, Schreiber RD. Neoantigens in Cancer Immunotherapy. Science (2015) 348(6230):69–74. doi: 10.1126/science.aaa4971
36. Mei P, Freitag CE, Wei L, Zhang Y, Parwani AV, Li Z. High Tumor Mutation Burden is Associated With DNA Damage Repair Gene Mutation in Breast Carcinomas. Diagn Pathol (2020) 15(1):50. doi: 10.1186/s13000-020-00971-7
37. Schrock AB, Ouyang C, Sandhu J, Sokol E, Jin D, Ross JS, et al. Tumor Mutational Burden is Predictive of Response to Immune Checkpoint Inhibitors in MSI-high Metastatic Colorectal Cancer. Ann Oncol (2019) 30(7):1096–103. doi: 10.1093/annonc/mdz134
38. Le DT, Uram JN, Wang H, Bartlett BR, Kemberling H, Eyring AD, et al. PD-1 Blockade in Tumors With Mismatch-Repair Deficiency. N Engl J Med (2015) 372:2509–20. doi: 10.1056/NEJMoa1500596
39. Rizvi NA, Hellmann M, Snyder A, Kvistborg P, Makarov V, Havel J. Cancer Immunology. Mutational Landscape Determines Sensitivity to PD-1 Blockade in non-Small Cell Lung Cancer. Science (2015) 348:124–8. doi: 10.1126/science.aaa1348
40. Goodman AM, Kato S, Bazhenova L, Patel SP, Frampton GM, Miller V, et al. Tumor Mutational Burden as an Independent Predictor of Response to Immunotherapy in Diverse Cancers. Mol Cancer Ther (2017) 16(11):2598–608. doi: 10.1158/1535-7163.MCT-17-0386
41. Hellmann MD, Nathanson T, Rizvi H, Creelan BC, Sanchez-Vega F, Ahuja A, et al. Genomic Features of Response to Combination Immunotherapy in Patients With Advanced non-Small-Cell Lung Cancer. Cancer Cell (2018) 33:843–52.e4. doi: 10.1016/j.ccell.2018.03.018
42. Rizvi H, Sanchez-Vega F, La K, Chatila W, Jonsson P, Halpenny D, et al. Molecular Determinants of Response to Anti-Programmed Cell Death (PD)-1 and Anti-Programmed Death-Ligand 1 (PD-L1) Blockade in Patients With non-Small-Cell Lung Cancer Profiled With Targeted Next-Generation Sequencing. J Clin Oncol (2018) 36(7):633–41. doi: 10.1200/JCO.2017.75.3384
43. Macherla S, Laks S, Naqash AR, Bulumulle A, Zervos E, Muzaffar M. Emerging Role of Immune Checkpoint Blockade in Pancreatic Cancer. Int J Mol Sci (2018) 19:3505. doi: 10.3390/ijms19113505
44. Abida W, Cheng ML, Armenia J, Middha S, Autio KA, Vargas HA, et al. Analysis of the Prevalence of Microsatellite Instability in Prostate Cancer and Response to Immune Checkpoint Blockade. JAMA Oncol (2019) 5:471–8. doi: 10.1001/jamaoncol.2018.5801
45. Priestley P, Baber J, Lolkema MP, Steeghs N, de Bruijn E, Shale C, et al. Pan-cancer Whole-Genome Analyses of Metastatic Solid Tumours. Nature (2019) 575(7781):210–6. doi: 10.1038/s41586-019-1689-y
46. Xiang L, Fu X, Wang X, Li W, Zheng X, Nan K, et al. A Potential Biomarker of Combination of Tumor Mutation Burden and Copy Number Alteration for Efficacy of Immunotherapy in KRAS-mutant Advanced Lung Adenocarcinoma. Front Oncol (2020) 10:559896. doi: 10.3389/fonc.2020.559896
47. van Nimwegen KJ, van Soest RA, Veltman JA, Nelen MR, van der Wilt GJ, Vissers LE, et al. Is the $1000 Genome as Near as We Think? A Cost Analysis of Next-Generation Sequencing. Clin Chem (2016) 62(11):1458–64. doi: 10.1373/clinchem.2016.258632
48. Weymann D, Laskin J, Roscoe R, Schrader KA, Chia S, Yip S, et al. The Cost and Cost Trajectory of Whole-Genome Analysis Guiding Treatment of Patients With Advanced Cancers. Mol Genet Genom Med (2017) 5(3):251–60. doi: 10.1002/mgg3.281
49. Marino P, Touzani R, Perrier L, Rouleau E, Kossi DS, Zhaomin Z, et al. Cost of Cancer Diagnosis Using Next-Generation Sequencing Targeted Gene Panels in Routine Practice: A Nationwide French Study. Eur J Hum Genet (2018) 26(3):314–23. doi: 10.1038/s41431-017-0081-3
50. Mosele F, Remon J, Mateo J, Westphalen CB, Barlesi F, Lolkema MP, et al. Recommendations for the Use of Next-Generation Sequencing (NGS) for Patients With Metastatic Cancers: A Report From the ESMO Precision Medicine Working Group. Ann Oncol (2020) 31(11):1491–505. doi: 10.1016/j.annonc.2020.07.014
51. Kaushal S, Freudenreich CH. The Role of Fork Stalling and DNA Structures in Causing Chromosome Fragility. Genes Chromosomes Cancer (2019) 58(5):270–83. doi: 10.1002/gcc.22721
52. Sansregret L, Vanhaesebroeck B, Swanton C. Determinants and Clinical Implications of Chromosomal Instability in Cancer. Nat Rev Clin Oncol (2018) 15(3):139–50. doi: 10.1038/nrclinonc.2017.198
53. Ingham M, Schwartz GK. Cell-cycle Therapeutics Come of Age. J Clin Oncol (2017) 35(25):2949–59. doi: 10.1200/JCO.2016.69.0032
54. Merkel O, Taylor N, Prutsch N, Staber PB, Moriggl R, Turner SD, et al. When the Guardian Sleeps: Reactivation of the p53 Pathway in Cancer. Mutat Res (2017) 773:1–13. doi: 10.1016/j.mrrev.2017.02.003
55. Manning AL, Benes C, Dyson NJ. Whole Chromosome Instability Resulting From the Synergistic Effects of pRB and p53 Inactivation. Oncogene (2014) 33(19):2487–94. doi: 10.1038/onc.2013.201
56. Dariya B, Aliya S, Merchant N, Alam A, Nagaraju GP. Colorectal Cancer Biology, Diagnosis, and Therapeutic Approaches. Crit Rev Oncog (2020) 25(2):71–94. doi: 10.1615/CritRevOncog.2020035067
57. Orr B, Compton DA. A Double-Edged Sword: How Oncogenes and Tumor Suppressor Genes can Contribute to Chromosomal Instability. Front Oncol (2013) 3:164. doi: 10.3389/fonc.2013.00164
58. Duijf PH, Benezra R. The Cancer Biology of Whole-Chromosome Instability. Oncogene (2013) 32(40):4727–36. doi: 10.1038/onc.2012.616
59. Cuomo M, Knebel A, Morrice N, Paterson H, Cohen P, Mittnacht S. P53-Driven Apoptosis Limits Centrosome Amplification and Genomic Instability Downstream of NPM1 Phosphorylation. Nat Cell Biol (2008) 10(6):723–30. doi: 10.1038/ncb1735
60. Van Loo P, Nordgard SH, Lingjærde OC, Russnes HG, Rye IH, Sun W, et al. Allele-Specific Copy Number Analysis of Tumors. Proc Natl Acad Sci USA (2010) 107(39):16910–5. doi: 10.1073/pnas.1009843107
61. Camacho N, Van Loo P, Edwards S, Kay JD, Matthews L, Haase K, et al. Appraising the Relevance of DNA Copy Number Loss and Gain in Prostate Cancer Using Whole Genome DNA Sequence Data. PloS Genet (2017) 13(9):e1007001. doi: 10.1371/journal.pgen.1007001
62. Taylor AM, Shih J, Ha G, Gao GF, Zhang X, Berger AC, et al. Genomic and Functional Approaches to Understanding Cancer Aneuploidy. Cancer Cell (2018) 33(4):676–89.e3. doi: 10.1016/j.ccell.2018.03.007
63. Anichini A, Tassi E, Grazia G, Mortarini R. The non-Small Cell Lung Cancer Immune Landscape: Emerging Complexity, Prognostic Relevance and Prospective Significance in the Context of Immunotherapy. Cancer Immunol Immunother (2018) 67(6):1011–22. doi: 10.1007/s00262-018-2147-7
64. Bassaganyas L, Pinyol R, Esteban-Fabró R, Torrens L, Torrecilla S, Willoughby CE, et al. Copy-number Alteration Burden Differentially Impacts Immune Profiles and Molecular Features of Hepatocellular Carcinoma. Clin Cancer Res (2020) 26(23):6350–61. doi: 10.1158/1078-0432.CCR-20-1497
65. Mermel CH, Schumacher SE, Hill B, Meyerson ML, Beroukhim R, Getz G. GISTIC2.0 Facilitates Sensitive and Confident Localization of the Targets of Focal Somatic Copy-Number Alteration in Human Cancers. Genome Biol (2011) 12(4). doi: 10.1186/gb-2011-12-4-r41
66. The Cancer Genome Atlas (TCGA). (2021). Available at: https://gdac.broadinstitute.org/ (Accessed 28 January 2021).
67. Luke JJ, Bao R, Sweis RF, Spranger S, Gajewski TF. Wnt/β-Catenin Pathway Activation Correlates With Immune Exclusion Across Human Cancers. Clin Cancer Res (2019) 25(10):3074–83. doi: 10.1158/1078-0432.CCR-18-1942
68. Kim HS, Cha H, Kim J, Park WY, Choi YL, Sun JM, et al. Genomic Scoring to Determine Clinical Benefit of Immunotherapy by Targeted Sequencing. Eur J Cancer (2019) 120:65–74. doi: 10.1016/j.ejca.2019.08.001
69. Hadi K, Yao X, Behr JM, Deshpande A, Xanthopoulakis C, Tian H, et al. Distinct Classes of Complex Structural Variation Uncovered Across Thousands of Cancer Genome Graphs. Cell (2020) 183(1):197–210.e32. doi: 10.1016/j.cell.2020.08.006
70. Cameron DL, Schroder J, Penington JS, Do H, Molania R, Dobrovic A, et al. GRIDSS: Sensitive and Specific Genomic Rearrangement Detection Using Positional De Bruijn Graph Assembly. Genome Res (2017) 27(12):2050–60. doi: 10.1101/gr.222109.117
71. Kim TM, Laird PW, Park PJ. The Landscape of Microsatellite Instability in Colorectal and Endometrial Cancer Genomes. Cell (2013) 155(4):858–68. doi: 10.1016/j.cell.2013.10.015
72. Palmieri G, Ascierto PA, Cossu A, Colombino M, Casula M, Botti G, et al. Assessment of Genetic Instability in Melanocytic Skin Lesions Through Microsatellite Analysis of Benign Nevi, Dysplastic Nevi, and Primary Melanomas Along With Their Metastases. Melanoma Res (2003) 13(2):167–70. doi: 10.1097/00008390-200304000-00009
73. Hause RJ, Pritchard CC, Shendure J, Salipante SJ. Classification and Characterization of Microsatellite Instability Across 18 Cancer Types. Nat Med (2016) 22(11):1342–50. doi: 10.1038/nm.4191
74. Yan L, Zhang W. Precision Medicine Becomes Reality-Tumor Type-Agnostic Therapy. Cancer Commun (Lond) (2018) 38(1):6. doi: 10.1186/s40880-018-0274-3
75. Dudley JC, Lin MT, Le DT, Eshleman JR. Microsatellite Instability as a Biomarker for PD-1 Blockade. Clin Cancer Res (2016) 22(4):813–20. doi: 10.1158/1078-0432.CCR-15-1678
76. Le DT, Durham JN, Smith KN, Wang H, Bartlett BR, Aulakh LK, et al. Mismatch Repair Deficiency Predicts Response of Solid Tumors to PD-1 Blockade. Science (2017) 357(6349):409–13. doi: 10.1126/science.aan6733
77. Gelsomino F, Barbolini M, Spallanzani A, Pugliese G, Cascinu S. The Evolving Role of Microsatellite Instability in Colorectal Cancer: A Review. Cancer Treat Rev (2016) 51:19–26. doi: 10.1016/j.ctrv.2016.10.005
78. Yang Y, Wang D, Jin L, Wu G, Bai Z, Wang J, et al. Prognostic Value of the Combination of Microsatellite Instability and BRAF Mutation in Colorectal Cancer. Cancer Manag Res (2018) 10:3911–29. doi: 10.2147/CMAR.S169649
79. Murcia O, Juárez M, Rodríguez-Soler M, Hernández-Illán E, Giner-Calabuig M, Alustiza M, et al. Colorectal Cancer Molecular Classification Using BRAF, KRAS, Microsatellite Instability and CIMP Status: Prognostic Implications and Response to Chemotherapy. PloS One (2018) 13(9):e0203051. doi: 10.1371/journal.pone.0203051
80. Zhao H, Thienpont B, Yesilyurt BT, Moisse M, Reumers J, Coenegrachts L, et al. Mismatch Repair Deficiency Endows Tumors With a Unique Mutation Signature and Sensitivity to DNA Double-Strand Breaks. Elife (2014) 3:e02725. doi: 10.7554/eLife.02725
81. Richman S. Deficient Mismatch Repair: Read All About it. Int J Oncol (2015) 47(4):1189–202. doi: 10.3892/ijo.2015.3119
82. U.S. Food and Drug Administration. (2017). Available at: https://www.fda.gov/newsevents/newsroom/pressannouncements/ucm560167.htm.
83. Yoshino T, Pentheroudakis G, Mishima S, Overman MJ, Yeh KH, Baba E, et al. Jsco-ESMO-ASCO-JSMO-TOS: International Expert Consensus Recommendations for Tumor-Agnostic Treatments in Patients With Solid Tumors With Microsatellite Instability or NTRK Fusions. Ann Oncol (2020) 31:861–72. doi: 10.1016/j.annonc.2020.03.299
84. Mandal R, Samstein RM, Lee KW, Havel JJ, Wang H, Krishna C, et al. Genetic Diversity of Tumors With Mismatch Repair Deficiency Influences anti-PD-1 Immunotherapy Response. Science (2019) 364(6439):485–91. doi: 10.1126/science.aau0447
85. Petrelli F, Ghidini M, Ghidini A, Tomasello G. Outcomes Following Immune Checkpoint Inhibitor Treatment of Patients With Microsatellite Instability-High Cancers: A Systematic Review and Meta-Analysis. JAMA Oncol (2020) 6(7):1068–71. doi: 10.1001/jamaoncol.2020.1046
86. Hu X, Estecio MR, Chen R, Reuben A, Wang L, Fujimoto J, et al. Evolution of DNA Methylome From Precancerous Lesions to Invasive Lung Adenocarcinomas. Nat Commun (2021) 12(1):687. doi: 10.1038/s41467-021-20907-z
87. Thorstensen L, Lind GE, Løvig T, Diep CB, Meling GI, Rognum TO, et al. Genetic and Epigenetic Changes of Components Affecting the WNT Pathway in Colorectal Carcinomas Stratified by Microsatellite Instability. Neoplasia (2005) 7(2):99–108. doi: 10.1593/neo.04448
88. Molinari F, Signoroni S, Lampis A, Bertan C, Perrone F, Sala P, et al. BRAF Mutation Analysis is a Valid Tool to Implement in Lynch Syndrome Diagnosis in Patients Classified According to the Bethesda Guidelines. Tumori (2014) 100(3):315–20. doi: 10.1700/1578.17214
89. Guinney J, Dienstmann R, Wang X, de Reyniès A, Schlicker A, Soneson C, et al. The Consensus Molecular Subtypes of Colorectal Cancer. Nat Med (2015) 21(11):1350–6. doi: 10.1038/nm.3967
90. Yang D, Thangaraju M, Greeneltch K, Browning DD, Schoenlein PV, Tamura T, et al. Repression of IFN Regulatory Factor 8 by DNA Methylation is a Molecular Determinant of Apoptotic Resistance and Metastatic Phenotype in Metastatic Tumor Cells. Cancer Res (2007) 67:3301–9. doi: 10.1158/0008-5472.CAN-06-4068
91. Covre A, Coral S, Nicolay H, Parisi G, Fazio C, Colizzi F, et al. Antitumor Activity of Epigenetic Immunomodulation Combined With CTLA-4 Blockade in Syngeneic Mouse Models. Oncoimmunology (2015) 4(8):e1019978. doi: 10.1080/2162402X.2015.1019978
92. Jones PA, Ohtani H, Chakravarthy A, De Carvalho DD. Epigenetic Therapy in Immune-Oncology. Nat Rev Cancer (2019) 19(3):151–61. doi: 10.1038/s41568-019-0109-9
93. Alexandrov LB, Nik-Zainal S, Wedge DC, Aparicio SA, Behjati S, Biankin AV, et al. Signatures of Mutational Processes in Human Cancer. Nature (2013) 500:415–21. doi: 10.1038/nature12477
94. Roberts SA, Gordenin DA. Hypermutation in Human Cancer Genomes: Footprints and Mechanisms. Nat Rev Cancer (2014) 14:786–800. doi: 10.1038/nrc3816
95. Kandoth C, McLellan MD, Vandin F, Ye K, Niu B, Lu C, et al. Mutational Landscape and Significance Across 12 Major Cancer Types. Nature (2013) 502(7471):333–9. doi: 10.1038/nature12634
96. Kane DP, Shcherbakova PV. A Common Cancer-Associated DNA Polymerase ϵ Mutation Causes an Exceptionally Strong Mutator Phenotype, Indicating Fidelity Defects Distinct From Loss of Proofreading. Cancer Res (2014) 74(7):1895–901. doi: 10.1158/0008-5472.CAN-13-2892
97. Campbell BB, Light N, Fabrizio D, Zatzman M, Fuligni F, de Borja R, et al. Comprehensive Analysis of Hypermutation in Human Cancer. Cell (2017) 171:1042–1056.e10. doi: 10.1016/j.cell.2017.09.048
98. Tumini E, Barroso S, Calero CP, Aguilera A. Roles of Human POLD1 and POLD3 in Genome Stability. Sci Rep (2016) 6:38873. doi: 10.1038/srep38873
99. Esteban-Jurado C, Giménez-Zaragoza D, Muñoz J, Franch-Expósito S, Álvarez-Barona M, Ocaña T, et al. POLE and POLD1 Screening in 155 Patients With Multiple Polyps and Early-Onset Colorectal Cancer. Oncotarget (2017) 8(16):26732–43. doi: 10.18632/oncotarget.15810
100. Rosner G, Gluck N, Carmi S, Bercovich D, Fliss-Issakov N, Ben-Yehoyada M, et al. POLD1 and POLE Gene Mutations in Jewish Cohorts of Early-Onset Colorectal Cancer and of Multiple Colorectal Adenomas. Dis Colon Rectum (2018) 61(9):1073–9. doi: 10.1097/DCR.0000000000001150
101. Nicolas E, Golemis EA, Arora S. Pold1: Central Mediator of DNA Replication and Repair, and Implication in Cancer and Other Pathologies. Gene (2016) 590(1):128–41. doi: 10.1016/j.gene.2016.06.031
102. Wang C, Gong J, Tu TY, Lee PP, Fakih M. Immune Profiling of Microsatellite Instability-High and Polymerase ϵ (POLE)-Mutated Metastatic Colorectal Tumors Identifies Predictors of Response to anti-PD-1 Therapy. J Gastrointest Oncol (2018) 9:404–15. doi: 10.21037/jgo.2018.01.09
103. Snyder A, Makarov V, Merghoub T, Yuan J, Zaretsky JM, Desrichard A, et al. Genetic Basis for Clinical Response to CTLA-4 Blockade in Melanoma. N Engl J Med (2014) 371:2189–99. doi: 10.1056/NEJMoa1406498
104. Yadav M, Jhunjhunwala S, Phung QT, Lupardus P, Tanguay J, Bumbaca S, et al. Predicting Immunogenic Tumor Mutations by Combining Mass Spectrometry and Exome Sequencing. Nature (2014) 515:572–6. doi: 10.1038/nature14001
105. Waalkes A, Smith N, Penewit K, Hempelmann J, Konnick EQ, Hause RJ, et al. Accurate Pan-Cancer Molecular Diagnosis of Microsatellite Instability by Single-Molecule Molecular Inversion Probe Capture and High-Throughput Sequencing. Clin Chem (2018) 64(6):950–8. doi: 10.1373/clinchem.2017.285981
106. Palmieri G, Colombino M, Cossu A, Marchetti A, Botti G, Ascierto PA. Genetic Instability and Increased Mutational Load: Which Diagnostic Tool Best Direct Patients With Cancer to Immunotherapy? J Transl Med (2017) 15(1):17. doi: 10.1186/s12967-017-1119-6
107. Palmieri G, Casula M, Manca A, Palomba G, Sini MC, Doneddu V, et al. Genetic Instability Markers in Cancer. Methods Mol Biol (2020) 2055:133–54. doi: 10.1007/978-1-4939-9773-2_6
108. Hugo W, Zaretsky JM, Sun L, Song C, Moreno BH, Hu-Lieskovan S, et al. Genomic and Transcriptomic Features of Response to anti-PD-1 Therapy in Metastatic Melanoma. Cell (2016) 165:35–44. doi: 10.1016/j.cell.2016.02.065
109. Zaretsky JM, Garcia-Diaz A, Shin DS, Escuin-Ordinas H, Hugo W, Hu-Lieskovan S, et al. Mutations Associated With Acquired Resistance to PD-1 Blockade in Melanoma. N Engl J Med (2016) 375:819–29. doi: 10.1056/NEJMoa1604958
110. Shin DS, Zaretsky JM, Escuin-Ordinas H, Garcia-Diaz A, Hu-Lieskovan S, Kalbasi A, et al. Primary Resistance to PD-1 Blockade Mediated by JAK1/2 Mutations. Cancer Discovery (2017) 7(2):188–201. doi: 10.1158/2159-8290.CD-16-1223
111. Passiglia F, Galvano A, Castiglia M, Incorvaia L, Calò V, Listì A, et al. Monitoring Blood Biomarkers to Predict Nivolumab Effectiveness in NSCLC Patients. Ther Adv Med Oncol (2019) 11. doi: 10.1177/1758835919839928
112. Guibert N, Jones G, Beeler JF, Plagnol V, Morris C, Mourlanette J, et al. Targeted Sequencing of Plasma Cell-Free DNA to Predict Response to PD1 Inhibitors in Advanced non-Small Cell Lung Cancer. Lung Cancer (2019) 137:1–6. doi: 10.1016/j.lungcan.2019.09.005
113. Tan L, Sandhu S, Lee RJ, Li J, Callahan J, Ftouni S, et al. Prediction and Monitoring of Relapse in Stage III Melanoma Using Circulating Tumor DNA. Ann Oncol (2019) 30(5):804–14. doi: 10.1093/annonc/mdz048
114. Lee JH, Saw RPM, Thompson JF, Lo S, Spillane AJ, Shannon KF, et al. Pre-Operative ctDNA Predicts Survival in High Risk Stage III Cutaneous Melanoma Patients. Ann Oncol (2019) 30(5):815–22. doi: 10.1093/annonc/mdz075
115. Santiago-Walker A, Gagnon R, Mazumdar J, Casey M, Long GV, Schadendorf D, et al. Correlation of BRAF Mutation Status in Circulating-Free DNA and Tumor and Association With Clinical Outcome Across Four BRAFi and MEKi Clinical Trials. Clin Cancer Res (2016) 22(3):567–74. doi: 10.1158/1078-0432.CCR-15-0321
116. Palmieri G. Circulating Driver Gene Mutations: What is the Impact on Melanoma Patients’ Management? Ann Oncol (2019) 30:669–71. doi: 10.1093/annonc/mdz090
117. Kasi PM. Mutational Burden on Circulating Cell-Free tumor-DNA Testing as a Surrogate Marker of Mismatch Repair Deficiency or Microsatellite Instability in Patients With Colorectal Cancers. J Gastrointest Oncol (2017) 8:747–8. doi: 10.21037/jgo.2017.06.05
118. Barata P, Agarwal N, Nussenzveig R, Gerendash B, Jaeger E, Hatton W, et al. Clinical Activity of Pembrolizumab in Metastatic Prostate Cancer With Microsatellite Instability High (MSI-H) Detected by Circulating Tumor DNA. J Immunother Cancer (2020) 8(2):e001065. doi: 10.1136/jitc-2020-001065
119. Chen EX, Jonker DJ, Loree JM, Kennecke HF, Berry SR, Couture F, et al. Effect of Combined Immune Checkpoint Inhibition vs Best Supportive Care Alone in Patients With Advanced Colorectal Cancer: The Canadian Cancer Trials Group Co.26 Study. JAMA Oncol (2020) 6(6):831–8. doi: 10.1001/jamaoncol.2020.0910
Keywords: melanoma, microsatellite instability, aneuploidy, tumor mutation burden, immunotherapy response
Citation: Palmieri G, Rozzo CM, Colombino M, Casula M, Sini MC, Manca A, Pisano M, Doneddu V, Paliogiannis P and Cossu A (2021) Are Molecular Alterations Linked to Genetic Instability Worth to Be Included as Biomarkers for Directing or Excluding Melanoma Patients to Immunotherapy? Front. Oncol. 11:666624. doi: 10.3389/fonc.2021.666624
Received: 10 February 2021; Accepted: 15 April 2021;
Published: 05 May 2021.
Edited by:
Renato Franco, University of Campania Luigi Vanvitelli, ItalyReviewed by:
Monica Cantile, Int fondazione pascale, ItalyMassimiliano Berretta, University of Messina, Italy
Copyright © 2021 Palmieri, Rozzo, Colombino, Casula, Sini, Manca, Pisano, Doneddu, Paliogiannis and Cossu. This is an open-access article distributed under the terms of the Creative Commons Attribution License (CC BY). The use, distribution or reproduction in other forums is permitted, provided the original author(s) and the copyright owner(s) are credited and that the original publication in this journal is cited, in accordance with accepted academic practice. No use, distribution or reproduction is permitted which does not comply with these terms.
*Correspondence: Antonio Cossu, Y29zc3VAdW5pc3MuaXQ=
†These authors have contributed equally to this work