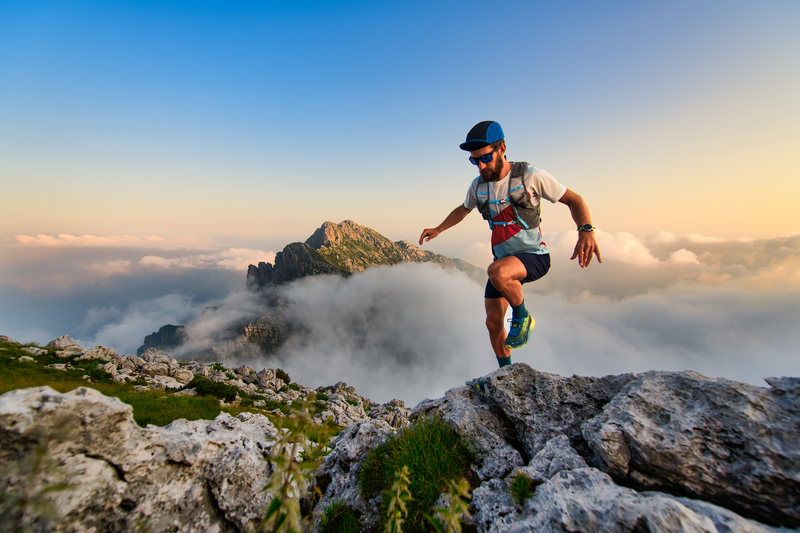
95% of researchers rate our articles as excellent or good
Learn more about the work of our research integrity team to safeguard the quality of each article we publish.
Find out more
ORIGINAL RESEARCH article
Front. Oncol. , 10 August 2021
Sec. Gastrointestinal Cancers
Volume 11 - 2021 | https://doi.org/10.3389/fonc.2021.664321
This article is part of the Research Topic Advanced Molecular Targets in the Diagnosis and Treatment of Gastrointestinal Cancers View all 55 articles
Colorectal adenocarcinoma (CRC) ranks one of the five most lethal malignant tumors both in China and worldwide. Early diagnosis and treatment of CRC could substantially increase the survival rate. Emerging evidence has revealed the importance of gut microbiome on CRC, thus fecal microbial community could be termed as a potential screen for non-invasive diagnosis. Importantly, few numbers of bacteria genus as non-invasive biomarkers with high sensitivity and specificity causing less cost would be benefitted more in clinical compared with the whole microbial community analysis. Here we analyzed the gut microbiome between CRC patients and healthy people using 16s rRNA sequencing showing the divergence of microbial composition between case and control. Furthermore, ExtraTrees classifier was performed for the classification of CRC gut microbiome and heathy control, and 13 bacteria were screened as biomarkers for CRC. In addition, 13 biomarkers including 12 bacteria genera and FOBT showed an outstanding sensitivity and specificity for discrimination of CRC patients from healthy controls. This method could be used as a non-invasive method for CRC early diagnosis.
As one of the most common gastrointestinal tumors worldwide, colorectal cancer (CRC) ranks third in the world among men and second among women, affecting more than 1.36 million people every year (1). Most of the CRC patients display no symptoms at early stages; in addition, the majority of CRCs develop slowly from adenomatous precursors (2). It has been estimated that >95% of colorectal cancer (CRC) would benefit from curative surgery if diagnosed at earlier or intermediate stages (3–6). Thus, early detection is of vital importance for improving the survival of CRC patients. Conventional screening methods including barium enema, colonoscopy, and sigmoidoscopy are uncomfortable, invasive, time consuming and expensive (7, 8). Fecal occult-blood testing (FOBT) and serum carcinoembryonic antigen (CEA) test are non-invasive methods; however, they are compromised by its low specificity (9–13). More non-invasive screening methods with high specificity and high sensitivity should be established for early detection of CRC.
Massive efforts in whole-genome sequencing and genome-wide association studies show that genetic factors only explain a small proportion of disease variance (14), and only about 5% cancers occur in the setting of a known genetic predisposition syndrome (15). It has been established that epigenetic regulation altering gene expression alone or in combination with inherited or somatic mutation plays important contribution to CRC (16). As a result, an intensive effort has been undertaken on CRC early diagnosis, which largely focuses on the methylation detection of tumor DNA or combined with the mutation deletion on certain genes (17–19). More importantly, the epigenetic alteration can be strongly affected by some environmental aspect, including diet habits or chronic alcohol consumption, which also affect human gut microbiota (20).
The gut microbiota maintains survival and metabolism with nutrients in the human body and works with the human body to respond to external environmental factors, carry out metabolic and immune activities, and maintain human health (21). Studying the intestinal microbiome composition of colorectal cancer patients can open new inspection methods for tumor screening. Recent studies, including ours, have suggested that microbiota profiles determined by high-throughput sequencing may be effective in predicting CRCs (22). It has been reported that peptostreptococcus anaerobius, an anaerobic bacterium enriched in the fecal and mucosal microbiota from CRC patients, promotes CRC (23). In addition, a number of bacteria, including Bacteroides fragilis and a strain of Escherichia coli (24–29), Streptococcus bovis (30, 31), Clostridium septicumand (32), and Fusobacterium nucleatum (33, 34) have been reported to be associated with CRC. Furthermore, metagenomic analysis of fecal microbiome has been performed and a couple of gene markers have been identified and validated as biomarkers for early diagnosis of CRC (35). Difference in gut microbiota between colorectal cancer patients and healthy people combined with other methods such as fecal immunochemical test (FIT), CEA, or other risks factors such as age and BMI index is required for improving accuracy (22, 36).
We evaluated differences in bacterial communities in stool samples of colorectal cancers and non-cancer controls through 16S rRNA high-throughput sequencing. In addition, 12 microbial biomarkers combined with FOBT have been identified for non-invasive early diagnosis of CRC.
Stool samples were collected from 382 individuals undergoing colonoscopy at the endoscopy center of Liaoning Cancer Hospital and Dongfang Hospital Affiliated to Tongji University, including 226 CRCs and 156 healthy controls. To avoid potential alternation of the gut microbiota, the exclusion criteria were: (1) past history of any cancer; (2) use of antibiotics within the past 3 months; (3) had surgery or invasive procedure within the past 3 months; and (4) had an inflammatory bowel disease. All enrolled subjects were asked to keep a steady dietary schedule and lifestyle and leave fecal sample over 1.0 g in a special containment before bowel preparation for any endoscopy or surgery. After stool collection from the patients, samples were stored at −80°C immediately for further analysis.
DNA from stool samples was extracted using Qiagen QIAamp DNA Stool Mini Kit (Qiagen) according to instructions of the manufacturer. Quality and quantity of extracted DNA were examined by electrophoretic separation in a 0.8% (wt/vol) agarose gel and NanoDrop 2000 spectrophotometer, respectively.
The hypervariable V3–V4 regions of the 16S rRNA gene were amplified using the primer set of 338F (5′-ACTCCTACGGGAGGCAGCA-3′) and 806R (5′-GGACTACHVGGGTWTCTAAT-3′). PCR amplification uses Pfu high-fidelity DNA polymerase from TransGen Biotech and strictly controls the number of amplification cycles to keep the number of cycles as low as possible while ensuring the same amplification conditions for the same batch of samples. PCR amplification, purification of amplified product, sequencing library preparation, and pyrosequencing were performed at paired-end 250 bp on the Illumina MiSeq platform by Personal Biotechnology, Co., Ltd. (Shanghai, China).
Raw sequencing data were processed using Quantitative Insights into Microbial Ecology (QIIME) v1.8.0 (37) and filtered by removing tags and primers. A quality cut-off was applied to discarding the reads (1) that are shorter than 150 bp, with (2) an average Phred score lower than 20, (3) with ambiguous bases. After that, the filtered reads were assembled using FLASH software v1.2.7 with overlapping between the paired-end reads >10. Chimeric sequences were filtered using USEARCH v5.2.236 (http://www.drive5.com/usearch/). After quality filtering and chimera removal, clean reads were then clustered into operational taxonomic units (OTUs) at 97% sequence identity using UCLUST. The taxonomic classification was performed with Greengenes database release 13.8. Alpha diversity indices of Chao1, ACE, Simpson and Shannon were estimated. Beta diversity analysis was performed with UniFrac in QIIME. Non-metric multi-dimensional scaling (NMDS) was generated by R language release package for analysis based on distance.
All enrolled subjects were asked to offer a valid fecal occult blood test report from a community hospital or a general hospital in recent 6 months. Stool samples with blank FIT result would have to be examined using Fecal Occult Blood Diagnostic Kit (Colloidal Gold) (Chemtrue@) which had been approved by the Chinese Food and Drug Administration Bureau. The cut-off value for positive FOBT was 200 ng/ml according to the instructions of the manufacturer.
Significant differences among treatments were identified through one-way analysis of variance (ANOVA) followed by Tukey’s test. Typically, homogeneity of variance for the obtained data was tested, and data of the test values >0.05 were adopted for the ANOVA analysis. All statistical analyses were performed using SPSS 19.0 (IBM, New York, USA), and significant levels were reported at p <0.05 and p <0.01.
FOBT test results were recorded as positive or negative.
We used an SVM (support vector machine) (R 3.6.1; the e1071 R package) to build the classifier for colorectal cancer with genera abundances as features. All the genera were normalized, and rare genera with less than 20% occurrences in all samples were removed. To filter out redundant features from the resulting 107 genera, the mRMR algorithm (38) was performed to 10 data sets with 50 features, each using the R package “mRMRe”. Leave-one-out cross-validation LDA (linear discriminant analysis) was applied to determine how many features to be used. We tuned both radial basis function (RBF) kernel (Gaussian kernel) and a linear kernel function of SVM with a tolerance of 0.001 to get a better performance using 10-fold cross-validation. For RBF kernel, the penalty parameter C was varied as {1e-4, 1e-3…, 1e4} and the gamma parameter G as {1e-5, 1e-3…, 1e3}. For linear kernel, the penalty parameter C was varied as {1e-4, 1e-3…, 1e4}. FOBT test from stool sample has been widely used in diagnosis; genera data together with FOBT test result were selected as feature set as well. Matthews correlation coefficient (MCC) was chosen as indicator of the performance. Receiver operating characteristic (ROC) figures were drawn using R package “pROC”.
After quality filtering and primer trimming, a total of 5,153 usable high-quality sequence reads were generated from 382 samples, the length of which was about 468 bp. In this study, a total of 4,728 OTUs were obtained from the colon cancer group and 4,331 OTUs from the healthy control group. A total of 3,906 OTUs were shared among the two groups. Compared with 423 unique OTUs from the healthy group, CRC group contained 822 unique OTUs (Supplemental Figure S1). Rarefaction curves of CRC and control samples almost plateaued, suggesting the sequencing was sufficient (Supplemental Figure S2).
Based on the total OTU statistical sequence, fecal microbial richness, as estimated by ACE and Chao1 (P-values <0.001, respectively), was significantly decreased in CRC (Figures 1A, B). The fecal microbial diversity, estimated by Shannon and Simpson, did not show significance between control and CRCs (Supplemental Figure S3). When microbiota composition between CRC and healthy gut was compared, beta-diversity exhibited difference between two groups (p = 0.001) (Figures 1C, D). These results suggested dysbiosis in the gut microbiome of CRC patients.
Figure 1 Comparison of gut microbiome between CRC and healthy gut. (A) Alpha diversity communities based on observed OTUs by richness (Chao1, ACE). (B) Beta diversity measured by unweighted unifrac. (C) Beta diversity measured by unweighted unifrac. (D) Beta diversity measured by weighted unifrac. Overall differences in the microbiome composition among groups were assessed by ANOSIM. *** means biological significance.
After quality filtering, sequences at a 97% sequence similarity were selected for taxonomic composition analysis; 21 bacterial phyla, 34 microbial class, 56 microbial orders, 107 microbial families, 209 microbial genera, and 268 microbial species have been identified (Supplemental Table S1).
The LEfSe (linear discriminant analysis effect size) analysis was performed to determine differences in bacterial taxonomy. The histogram with cladogram showed that overall phylum Bacteroidetes was highly accumulated in CRC while overall phylum Actinobacteria was overall less accumulated in health samples. Divergent alteration was observed at lower taxonomic levels from phylum Firmicutes and Proteobacteria (Figure 2).
Figure 2 Linear discriminant analysis effect size (LEfSe) analysis of gut microbiota composition between two groups.
We further compared the difference between control and CRC microbiome at different levels. At the phylum level, four phyla were detected with relatively high abundance. CRC samples showed increased abundance of phylum Bacteroidetes while healthy samples showed increased abundance of Firmicutes, Proteobacteria, and Actinobacteria (Supplemental Figure S4A). At the family level, for the family with relatively high abundance, CRC samples had higher abundances of Bacteroidaceae, Veillonellaceae and Prevotellaceae whereas healthy samples had higher abundances of Ruminococcaceae, Lachnospiraceae, Enterobacteriaceae, and Bifidobacteriaceae (Supplemental Figure S4B). At the genus level, the most abundant genera identified in healthy samples were Faecalibacterium (10.49%), Bifidobacterium (7.65%), and Bacteroides (7.33%), while in CRC gut, the most abundant genera were Faecalibacterium (6.37%), Bacteroides (21.79%), and Prevotella (5.14%) (Figure 3A). The most abundant gut microbe, Fecalibacterium, termed as the marker of healthy gut (39), decreased by 60%. Beneficial intestinal bacteria Bifidobacterium (40) decreased by 35% in the CRC gut (Figure 3B). Importantly, the role of several genera the role in CRC, such as Peptostreptococcus (23), Fusobacterium (33, 34), Porphyromonas, Parvimonas, Gemella, and Prevotella (41) had been reported; they were extremely upregulated in the CRC gut (Figure 3C and Supplemental Table S2). The abundance of all gut microbes was listed as Supplemental Table S1. These results strongly suggested the dysbiosis in the CRC gut.
Figure 3 Analysis of genus level and selected genus between CRC and control. (A) The distribution of two groups at genus level. (B) Abundance of benefit intestinal bacteria between two groups. (C) Abundance of CRC related intestinal bacteria between two groups.
We further compared the functional capacity of the gut microbiota between CRC and healthy subjects; the transporter pathway, especially the ABC transporter pathway, is significantly increased in CRC gut, and a large number of metabolism related pathways, such as vitamin B6 metabolism, energy metabolism, amino sugar and nucleotide sugar metabolism, fructose and mannose metabolism, phosphonate and phosphinate metabolism, pyruvate metabolism, phenylalanine metabolism, D-Glutamine and D-glutamate metabolism, sphingolipid metabolism, and nitrogen metabolism decreased in CRC gut, with the exception of glycerophospholipid metabolism. These results suggested the disorder of metabolism in CRC patients (Figure 4).
The changes in the bacterial community between the two groups could be screened as biomarkers for colorectal cancer detection to assist in its diagnosis. To select the most relevant feature which could be term as biomarkers for CRC, the ExtraTrees classifier calculating feature importance score was performed. Forty significantly different features showing different abundances were selected for further analysis (Supplemental Table S3).
To illustrate the diagnostic value of the selected biomarkers in the gut microbiome for colorectal cancer and find out a smaller number of biomarkers for diagnosis, we constructed a classifier established by SVM (Support Vector Machine) model to detect cancerous samples. We selected 267 samples as training set, including 141 from Liaoning and 126 from Shanghai; in addition, the rest 115 samples were selected as verification set, including 61 from Liaoning and 54 from Shanghai. The SVM model used 13 genera (Supplemental Table S4) from bacteria as features to distinguish CRC patients from healthy controls with a sensitivity of 82.6%, specificity of 78.3%, precision of 85.1%, and accuracy of 80.9% under the para1 conditions (‘C’: 1,000, ‘gamma’: 1e-5, ‘kernel’: ‘radial’) (Supplemental Table S5 and Supplemental Figure S5). We also constructed the classifier from bacteria together with FOBT; 13 features including 12 bacteria genera and FOBT (Table 1) could distinguish CRC and healthy people. The sensitivity, specificity, precision, and accuracy increased into 91.3, 93.5, 95.4, and 92.2% under the para2 conditions (‘C’: 10, ‘kernel’: ‘linear’) (Table 2 and Figure 5). The 12 fecal bacteria and FOBT could be termed as non-invasive biomarkers for colorectal adenocarcinoma.
CRC is a high risk cancer in China and all over the world. Early detection and treatment of CRC are important for improving the late survival rate and reducing the cost of late treatment. Colonoscopy and FOBT are widely used in CRC screening; however, for their low compliance or sensitivity, more non-invasive and painless methods with high sensitivity and specificity are required (7–13). Circulating tumor DNA (ctDNA) is an extracellular DNA that originates from tumor cells and circulates in several bodily fluids, including blood, synovial fluid, and cerebrospinal fluid (42). For the similarity of genetic and epigenetic information provided by ctDNA to that of invasive tumor biopsies, ctDNA has been widely used to detect the gene mutation and is termed as a non-invasive diagnostic tool for several cancers (43). In many tumors, increased methylation of tumor suppressor genes occurs at an early stage, thus, ctDNA methylation profiling detection can be used as an alternative non-invasive diagnostic tool (44–46). Some specific DNA methylation sites, such as SEPT9, have been identified as biomarkers of CRC (47, 48). However, the extremely low level in the blood and the non-organ information of ctDNA present a great challenge to early diagnosis.
The gut microbiome plays a major role in protecting the host against the overgrowth of pathogens and in sustaining the health of colon. There is intensive evidence revealing the close relationship between gut microbiome and colorectal cancer (49–51). In clinical application, changes of gut microbiome can be regularly monitored, thus, revealing gut microbiome divergence between CRC and healthy control would help to find out the weighted bacteria for distinguishing them, and the bacteria can be termed biomarkers for CRC early diagnosis.
We have performed high-throughput sequencing on the v3–v4 regions of intestinal bacteria 16S rRNA gene in stool and described the patterns of gut microbiome relative to healthy control and CRC patients. Fecal richness from colorectal cancer patients decreased; in addition, the proportion of various beneficial bacteria decreased, and the proportion of harmful bacteria significantly increased. A dozen of opportunistic pathogens including Bacteroides and Prevotella were significantly increased in patients with colorectal cancer (Figure 3). A couple of pathogens, including Fusobacterium nucleatum (33, 34), Peptostreptococcus anaerobius, and enterotoxigenic Bacteroides fragilis (52), whose roles have been established in CRC induction, were highly accumulated in CRC patients (Figure 3 and Supplemental Table S1). The identification of dysbiosis characteristics could be facilitated, and they can be considered as taxonomic biomarkers for CRC screening.
We performed machine learning using SVM model between pairs of cohorts to conduct binary classification for classifying CRC patients and control. A variety of features including taxonomic, functional (53), and k-mer-based (54) classification schemes have been used for machine learning approaches. Here, we used 13 genera of the gut bacteria to show their great contribution in differentiating the CRC state versus control for machine learning. In addition, FOBT test results were selected as a their importance on CRC diagnosis in clinical practice. Our machine learning results showed high performances in CRC versus control models (Table 1 and Figure 5). Parvimonas, Prevotella (41), Clostridium (32), Dorea (55), and Bacteroides (56) have been reported to have higher accumulation in CRC gut. On the other side, Blautia and Faecalibacterium have been reported to have lower accumulation in CRC gut (57). Notably, Blautia, Lachnospira, and Roseburia belong to Lachnospiraceae, whose lower levels were associated with CRC (58). In our results, the genera beneficial for health were increased, and the genera harmful for health were reduced in the CRC gut. In addition, the association of Odoribacter and SMB53 with CRC was reported for the first time. The high performance of fecal bacteria and FOBT test from stool sample facilitates the establishment of a new non-invasive method for an examination of colorectal cancer.
In addition to causing intestinal diseases, gut microbiome also contributes to obesity, diabetes, allergic asthma, and neuropsychiatric diseases (59–61); thus, clinical monitoring of fecal bacteria can assist in the diagnosis of other diseases related to gut microbiome. Furthermore, gut status could be improved by artificially guiding the intervention of diet or the intake of beneficial bacteria according to the changes of gut microbiome (62), and the improvement could be easily detected from the fecal bacteria. Thus, gut microbiome has become a hot spot in clinical research.
In summary, we monitored the gut microbiome and took 12 bacteria genus and FOBT displaying high weight for classification between CRC and healthy gut as biomarkers for CRC early diagnosis. The method benefits those who cannot receive colonoscopy in a short time and those who are not willing to use colonoscopy. Compared with the existing methods of CRC diagnosis, our method is non-invasive and painless; not only does it not require complex examination and preparation before sampling, but also improves the sensitivity and specificity of the test compared with the FOBT alone.
The original contributions presented in the study are publicly available. This data can be found here: NCBI repository, accession number: PRJNA706061.
The studies involving human participants were reviewed and approved by Ethics Committee of Liaoning Cancer Hospital and Dongfang Hospital Affiliated to Tongji University. The patients/participants provided their written informed consent to participate in this study.
BY and BM prepared the samples, extracted the DNA for 16s rRNA sequencing and revised the manuscript. JY analyzed the 16s rRNA sequencing data and wrote the manuscript. QM helped to prepare the samples. TD helped to perform the data analysis and organize the data. HL and YZ performed the machine learning analysis. ZS revised the manuscript. SM and CS designed and leaded the project. All authors contributed to the article and approved the submitted version.
This study was supported by Innovation Fund of Science and Technology Committee in Shanghai Pudong New Area (Nos. PKJ2016-Y60), National Natural Science Foundation of China (Nos. 81871953) and Jiangxi Youth Science Fund (Nos. 20171BAB215043) National Science Foundation of China, Grant/Award Number: 81902383, Revitalizing Liaoning Talents Program, Grant/Award Number: XLYC1907004
JY, YZ, ZS and SM were employed by Shanghai Personal Biotechnology Co., Ltd.
The remaining authors declare that the research was conducted in the absence of any commercial or financial relationships that could be construed as a potential conflict of interest.
All claims expressed in this article are solely those of the authors and do not necessarily represent those of their affiliated organizations, or those of the publisher, the editors and the reviewers. Any product that may be evaluated in this article, or claim that may be made by its manufacturer, is not guaranteed or endorsed by the publisher.
The Supplementary Material for this article can be found online at: https://www.frontiersin.org/articles/10.3389/fonc.2021.664321/full#supplementary-material
Supplementary Figure 1 | Venn diagram based on OTU. Each ellipse represents a group of samples. The overlapping area between the ellipses represents the shared OTU among the sample groups, and number of each block indicates the number of common or unique OTU of the sample groups contained in the block.
Supplementary Figure 2 | Rarefaction curves of CRC and control samples.
Supplementary Figure 3 | Alpha diversity communities based on observed OTUs by diversity (Simpson, Shannon).
Supplementary Figure 4 | The distribution of two groups at phylum level (A) and family level (B).
1. Ferlay J, Soerjomataram I, Dikshit R, Eser S, Mathers C, Rebelo M, et al. Cancer Incidence and Mortality Worldwide: Sources, Methods and Major Patterns in GLOBOCAN 2012. Int J Cancer (2015) 136(5):E359–86.
2. Lieberman DA, Weiss DG, Bond JH, Ahnen DJ, Garewal H, Chejfec G. Use of Colonoscopy to Screen Asymptomatic Adults for Colorectal Cancer. N Engl J Med (2000) 343:162–8. doi: 10.1056/NEJM200007203430301
3. Gumaste VV. CT Colonography Can Be an Adjunct to Optical Colonoscopy in CRC Screening. Dig Dis Sci (2009) 54:212–7. doi: 10.1007/s10620-008-0360-5
4. Pawa N, Arulampalam T, Norton JD. Screening for Colorectal Cancer: Established and Emerging Modalities. Nat Rev Gastroenterol Hepatol (2011) 8:711–22. doi: 10.1038/nrgastro.2011.205
5. Siegel RL, Miller KD, Fedewa SA, Ahnen DJ, Meester RGS, Barzi A. Colorectal Cancer Statistics. CA Cancer J Clin (2017) 67:177–93. doi: 10.3322/caac.21395
6. Feng RM, Zong YN, Cao SM, Xu RH. Current Cancer Situation in China: Good or Bad News from the 2018 Global Cancer Statistics? Cancer Commun (Lond) (2019) 39:22. doi: 10.1186/s40880-019-0368-6
7. Sung JJ, Ng SC, Chan FK, Chiu HM, Kim HS, Matsuda T, et al. An Updated Asia Pacific Consensus Recommendations on Colorectal Cancer Screening. Gut (2015) 64:121–32. doi: 10.1136/gutjnl-2013-306503
8. Rex DK, Johnson DA, Anderson JC, Schoenfeld PS, Burke CA, Inadomi JM, et al. American College of Gastroenterology Guidelines for Colorectal Cancer Screening. Am J Gastroenterol (2009) 104:739–50. doi: 10.1038/ajg.2009.104
9. Hewitson P, Glasziou P, Irwig L, Towler B, Watson E. Screening for Colorectal Cancer Using the Fecal Occult Blood Test, Hemoccult. Cochrane Database Syst Rev (2007) 1:CD001216. doi: 10.1002/14651858.CD001216.pub2
10. Raginel T, Puvinel J, Ferrand O, Bouvier V, Levillain R, Ruiz A, et al. A Population-Based Comparison of Immunochemical Fecal Occult Blood Tests for Colorectal Cancer Screening. Gastroenterology (2013) 144:918–25. doi: 10.1053/j.gastro.2013.01.042
11. Nicholson BD, Shinkins B, Pathiraja I, Roberts NW, James TJ, Mallett S, et al. Blood CEA Levels for Detecting Recurrent Colorectal Cancer. Cochrane Database Syst Rev (2015) 12:CD011134. doi: 10.1002/14651858.CD011134.pub2
12. Palmqvist R, Engarås B, Lindmark G, Hallmans G, Tavelin B, Nilsson O, et al. Prediagnostic Levels of Carcinoembryonic Antigen and CA 242 in Colorectal Cancer: A Matched Case-Control Study. Dis Colon rectum (2003) 46:1538–44. doi: 10.1007/s10350-004-6810-z
14. Galvan A, Ioannidis JP, Dragani TA. Beyond Genome-Wide Association Studies: Genetic Heterogeneity and Individual Predisposition to Cancer. Trends Genet (2010) 26:132–41. doi: 10.1016/j.tig.2009.12.008
15. Foulkes WD. Inherited Susceptibility to Common Cancers. N Engl J Med (2008) 359:2143–53. doi: 10.1056/NEJMra0802968
16. Yang T, Owen JL, Lightfoot YL, Kladde MP, Mohamadzadeh M. Microbiota Impact on the Epigenetic Regulation of Colorectal Cancer. Trends Mol Med (2013) 19:714–25. doi: 10.1016/j.molmed.2013.08.005
17. Muller HM, Oberwalder M, Fiegl H, Morandell M, Goebel G, Zitt M, et al. Methylation Changes in Fecal DNA: A Marker for Colorectal Cancer Screening? Lancet (2004) 363:1283–5. doi: 10.1016/S0140-6736(04)16002-9
18. Mitchell SM, Ho T, Brown GS, Baker RT, Thomas ML, McEvoy A, et al. Evaluation of Methylation Biomarkers for Detection of Circulating Tumor DNA and Application to Colorectal Cancer. Genes (Basel) (2016) 15:125. doi: 10.3390/genes7120125
19. Carmona FJ, Azuara D, Berenguer-Llergo A, Fernandez AF, Biondo S, de Oca J, et al. DNA Methylation Biomarkers for Noninvasive Diagnosis of Colorectal Cancer. Cancer Prev Res (Phila) (2013) 6:656–65. doi: 10.1158/1940-6207.CAPR-12-0501
20. Kim BC, Shin A, Hong CW, Sohn DK, Han KS, Ryu KH, et al. Association of Colorectal Adenoma with Components of Metabolic Syndrome. Cancer Causes Control (2012) 23:727–35. doi: 10.1007/s10552-012-9942-9
21. Jie Z, Xia H, Zhong SL, Feng Q, Li S, Liang S, et al. The Gut Microbiome in Atherosclerotic Cardiovascular Disease. Nat Commun (2017) 8:845. doi: 10.1038/s41467-017-00900-1
22. Zeller G, Tap J, Voigt AY, Sunagawa S, Kultima JR, Costea PI, et al. Potential of Fecal Microbiota for Early-Stage Detection of Colorectal Cancer. Mol Syst Biol (2014) 10:766. doi: 10.15252/msb.20145645
23. Long X, Wong CC, Tong L, Chu ESH, Ho Szeto C, Go MYY, et al. Peptostreptococcus Anaerobius Promotes Colorectal Carcinogenesis and Modulates Tumour Immunity. Nat Microbiol (2019) 4:2319–30. doi: 10.1038/s41564-019-0541-3
24. Arthur JC, Perez-Chanona E, Muhlbauer M, Tomkovich S, Uronis JM, Fan TJ, et al. Intestinal Inflammation Targets Cancer-Inducing Activity of the Microbiota. Science (2012) 338:120–23. doi: 10.1126/science.1224820
25. Cuevas-Ramos G, Petit CR, Marcq I, Boury M, Oswald E, Nougayrede JP. Escherichia Coli Induces DNA Damage In Vivo and Triggers Genomic Instability in Mammalian Cells. Proc Natl Acad Sci USA (2010) 107:11537–42. doi: 10.1073/pnas.1001261107
26. Grivennikov SI, Wang K, Mucida D, Stewart CA, Schnabl B, Jauch D, et al. Adenoma-Linked Barrier Defects and Microbial Products Drive IL-23/IL-17-Mediated Tumour Growth. Nature (2012) 91:254–58. doi: 10.1038/nature11465
27. Toprak NU, Yagci A, Gulluoglu BM, Akin ML, Demirkalem P, Celenk T, et al. A Possible Role of Bacteroides Fragilis Enterotoxin in the Aetiology of Colorectal Cancer. Clin Microbio Infect (2006) 12:782–86. doi: 10.1111/j.1469-0691.2006
28. Uronis JM, Muhlbauer M, Herfarth HH, Rubinas TC, Jones GS, Jobin C. Modulation of the Intestinal Microbiota Alters Colitis-Associated Colorectal Cancer Susceptibility. PloS One (2009) 4:e6026. doi: 10.1371/journal.pone.0006026
29. Wu S, Rhee KJ, Albesiano E, Rabizadeh S, Wu X, Yen HR, et al. A Human Colonic Commensal Promotes Colon Tumorigenesis via Activation of T Helper Type 17 T Cell Responses. Nat Med (2009) 15:1016–22. doi: 10.1038/nm.2015
30. Boleij A, Schaeps RM, Tjalsma H. Association Between Streptococcus Bovis and Colon Cancer. J Clin Microbiol (2009) 47:516. doi: 10.1128/JCM.01755-08
31. Burton JP, Wescombe PA, Moore CJ, Chilcott CN, Tagg JR. Safety Assessment of the Oral Cavity Probiotic Streptococcus Salivarius K12. Appl Environ Microb (2006) 72:3050–53. doi: 10.1128/AEM.72.4.3050-3053.2006
32. Seder CW, Kramer M, Long G, Uzieblo MR, Shanley CJ, Bove P. Clostridium Septicum Aortitis: Report of Two Cases and Review of the Literature. J Vasc Surg (2009) 49:1304–09. doi: 10.1016/j.jvs.2008.11.058
33. Castellarin M, Warren RL, Freeman JD, Dreolini L, Krzywinski M, Strauss J, et al. Fusobacterium Nucleatum Infection Is Prevalent in Human Colorectal Carcinoma. Genome Res (2012) 22:299–306. doi: 10.1101/gr.126516.111
34. Kostic AD, Gevers D, Pedamallu CS, Michaud M, Duke F, Earl AM, et al. Genomic Analysis Identifies Association of Fusobacterium with Colorectal Carcinoma. Genome Res (2012) 22:292–98. doi: 10.1101/gr.126573.111
35. Yu J, Feng Q, Wong SH, Zhang D, Liang QY, Qin Y, et al. Metagenomic Analysis of Fecal Microbiome as a Tool Towards Rargeted Non-Invasive Biomarkers for Colorectal Cancer. Gut (2017) 66:70–8. doi: 10.1136/gutjnl-2015-309800
36. Zackular JP, Rogers MA, Ruffin MT, Schloss PD. The Human Gut Microbiome as a Screening Tool for Colorectal Cancer. Cancer Prev Res (Phila) (2014) 7:1112–21. doi: 10.1158/1940-6207.CAPR-14-0129
37. Caporaso JG, Kuczynski J, Stombaugh J, Bittinger K, Bushman FD, Costello EK, et al. QIIME Allows Analysis of High-Throughput Community Sequencing Data. Nat Methods (2010) 7:335–36. doi: 10.1038/nmeth.f.303
38. De Jay N, Papillon-Cavanagh S, Olsen C, El-Hachem N, Bontempi G, Haibe-Kains B. mRMRe: An R Package for Parallelized mRMR Ensemble Feature Selection. Bioinformatics (2013) 29:2365–68. doi: 10.1093/bioinformatics/btt383
39. Qin J, Li R, Raes J, Arumugam M, Burgdorf KS, Manichanh C, et al. A Human Gut Microbial Gene Catalogue Established by Metagenomic Sequencing. Nature (2010) 464:59–65. doi: 10.1038/nature08821
40. Gibson GR, Beatty ER, Wang X, Cummings JH. Selective Stimulation of Bifidobacteria in the Human Colon by Oligofructose and Inulin. Gastroenterology (1995) 108:975–82. doi: 10.1016/0016-5085(95)90192-2
41. Baxter NT, Ruffin MT, Rogers MAM, Schloss PD. Microbiota-Based Model Improves the Sensitivity of Fecal Immunochemical Test for Detecting Colonic Lesions. Genome Med (2016) 8:37. doi: 10.1186/s13073-016-0290-3
42. Cheng F, Su L, Qian C. Circulating Tumor DNA: A Promising Biomarker in the Liquid Biopsy of Cancer. Oncotarget (2016) 7:4883248841. doi: 10.18632/oncotarget.9453
43. Berger AW, Schwerdel D, Welz H, Marienfeld R, Schmidt SA, Kleger A. Treatment Monitoring in Metastatic Colorectal Cancer Patients by Quantification and KRAS Genotyping of Circulating Cell-Free DNA. PloS One (2017) 12:e0174308. doi: 10.1371/journal.pone.0174308
44. Baylin SB, Jones PAA. Decade of Exploring the Cancer Epigenome - Biological and Rranslational Implications. Nat Rev Cancer (2011) 11:726–34. doi: 10.1038/nrc3130
45. Baylin SB, Jones PA. Epigenetic Determinants of Cancer. Cold Spring Harb Perspect Biol (2016) 8:a019505. doi: 10.1101/cshperspect a019505.
46. Irizarry RA, Ladd-Acosta C, Wen B, Wu Z, Montano C, Onyango P, et al. The Human Colon Cancer Methylome Dhows Similar Hypo- and Hypermethylation at Conserved Tissue Specific CpG Island Shores. Nat Genet (2009) 41:178–86. doi: 10.1038/ng.298
47. Warren JD, Xiong W, Bunker AM, Vaughn CP, Furtado LV, Roberts WL, et al. Septin 9 Methylated DNA Is a Sensitive and Specific Blood Test for Colorectal Cancer. BMC Med (2011) 9:133. doi: 10.1186/1741-7015-9-133
48. Church TR, Wandell M, Lofton-Day C, Mongin SJ, Burger M, Payne SR, et al. Prospective Evaluation of Methylated SEPT9 in Plasma for Detection of Asymptomatic Colorectal Cancer. Gut (2014) 63:317–25. doi: 10.1136/gutjnl-2012-304149
49. Fong W, Li Q, Yu J. Gut Microbiota Modulation: A Novel Strategy for Prevention and Treatment of Colorectal Cancer. Oncogene (2020) 39:4925–43. doi: 10.1038/s41388-020-1341-1
50. Hold GL, Garrett WS. Gut Microbiota. Microbiota Organization–A Key to Understanding CRC Development. Nat Rev Gastroenterol Hepatol (2015) 12:128–29. doi: 10.1038/nrgastro.2015.25
51. Ray K. Gut microbiota: Oral Microbiome Could Provide Clues to CRC. Nat Rev Gastroenterol Hepatol (2017) 14:690. doi: 10.1038/nrgastro.2017.158
52. Rubinstein MR, Wang X, Liu W, Hao Y, Cai G, Han YW. Fusobacterium Nucleatum Promotes Colorectal Carcinogenesis by Modulating E-Cadherin/Beta-Catenin Signaling via Its FadA Adhesin. Cell Host Microbe (2013) 14:195–206. doi: 10.1016/j.chom.2013.07.012
53. Ning J, Beiko RG. Phylogenetic Approaches to Microbial Community Classification. Microbiome (2015) 3:47. doi: 10.1186/s40168-015-0114-5
54. Duvallet C, Gibbons SM, Gurry T, Irizarry RA, Alm EJ. Meta-Analysis of Gut Microbiome Studies Identifies Disease-Specific and Shared Responses. Nat Commun (2017) 8:1784. doi: 10.1038/s41467-017-01973-8
55. Yang J, Mcdowell A, Kim EK, Seo H, Lee WH, Moon C-M. Development of a Colorectal Cancer Diagnostic Model and Dietary Risk Assessment through Gut Microbiome Analysis. Exp Mol Med (2019) 51:117. doi: 10.1038/s12276-019-0313-4
56. Chen WG, Liu FL, Ling ZX, Tong XJ, Xiang C. Human Intestinal Lumen and Mucosa-Associated Microbiota in Patients with Colorectal Cancer. PloS One (2012) 7(6):e39743. doi: 10.1371/journal.pone.0039743
57. Sinha R, Ahn JY, Sampson JN, Shi JX, Yu GQ, Xiong XQ, et al. Fecal Microbiota, Fecal Metabolome, and Colorectal Cancer Interrelations. PloS One (2016) 11:e0152126. doi: 10.1371/journal.pone.0152126
58. Wang TT, Cai GX, Qiu YP, Zhang MH, Pang XY, Jia W, et al. Structural Segregation of Gut Microbiota between Colorectal Cancer Patients and Healthy Volunteers. ISME Journal (2011) 6:320–9. doi: 10.1038/ismej.2011.109
59. Nielsen DS, Krych L, Buschard K, Hansen CH, Hansen AK. Beyond Genetics. Influence of Dietary Factors and Gut Microbiota on Type 1 Diabetes. FEBS Lett (2014) 588:4234–43. doi: 10.1016/j.febslet.2014.04.010
60. Serino M, Blasco-Baque V, Nicolas S, Burcelin R. Far From the Eyes, Close to the Heart: Dysbiosis of Gut Microbiota and Cardiovascular Consequences. Curr Cardiol Rep (2014) 16:540. doi: 10.1007/s11886-014-0540-1
61. Arrieta MC, Finlay B. The Intestinal Microbiota and Allergic Asthma. J Infect (2014) 69:53–5. doi: 10.1016/j.jinf.2014.07.015
Keywords: colorectal adenocarcinoma, non-invasive diagnosis, biomarker, gut microbiome, 16s rRNA sequencing, machine learning
Citation: Yuan B, Ma B, Yu J, Meng Q, Du T, Li H, Zhu Y, Sun Z, Ma S and Song C (2021) Fecal Bacteria as Non-Invasive Biomarkers for Colorectal Adenocarcinoma. Front. Oncol. 11:664321. doi: 10.3389/fonc.2021.664321
Received: 05 February 2021; Accepted: 07 June 2021;
Published: 10 August 2021.
Edited by:
Simona Gurzu, George Emil Palade University of Medicine, Pharmacy, Sciences and Technology of Târgu Mureş, RomaniaReviewed by:
Ying Yuan, Zhejiang University, ChinaCopyright © 2021 Yuan, Ma, Yu, Meng, Du, Li, Zhu, Sun, Ma and Song. This is an open-access article distributed under the terms of the Creative Commons Attribution License (CC BY). The use, distribution or reproduction in other forums is permitted, provided the original author(s) and the copyright owner(s) are credited and that the original publication in this journal is cited, in accordance with accepted academic practice. No use, distribution or reproduction is permitted which does not comply with these terms.
*Correspondence: Siping Ma, YnJ1Y2VtYTVAMTYzLmNvbQ==; Chun Song, Y2h1bnNvbmcxNjNAMTYzLmNvbQ==
†These authors have contributed equally to this work
Disclaimer: All claims expressed in this article are solely those of the authors and do not necessarily represent those of their affiliated organizations, or those of the publisher, the editors and the reviewers. Any product that may be evaluated in this article or claim that may be made by its manufacturer is not guaranteed or endorsed by the publisher.
Research integrity at Frontiers
Learn more about the work of our research integrity team to safeguard the quality of each article we publish.