- 1Institute of Translational Medicine, Pirogov Russian National Research Medical University, Moscow, Russia
- 2Laboratory of Clinical Biochemistry, N.N. Blokhin Cancer Research Medical Center of Oncology, Moscow, Russia
Nucleic acid fragments found in blood circulation originate mostly from dying cells and carry signs pointing to specific features of the parental cell types. Deciphering these clues may be transformative for numerous research and clinical applications but strongly depends on the development and implementation of robust analytical methods. Remarkable progress has been achieved in the reliable detection of sequence alterations in cell-free DNA while decoding epigenetic information from methylation and fragmentation patterns requires more sophisticated approaches. This review discusses the currently available strategies for detecting and analyzing the epigenetic marks in the liquid biopsies.
Introduction
The pools of circulating nucleic acids found in biological fluids have been extensively studied in recent decades, primarily due to the minimally invasive sampling procedures that promise a number of apparent practical benefits. First, an opportunity to identify molecular changes that underlie pathological processes associated with cell death occurring in distant tissues from a simple blood draw. Second, real-time monitoring of the alterations through a sequential sampling series without disturbing the pathological foci. Third, cell-free nucleic acids in blood circulation are believed to represent a cumulative pool of fragments originating from different sources in the body, allowing for a snapshot of alterations that occur at various locations with increased cell turnover or active release (1–3), a key feature for cancer investigation in the light of tumor heterogeneity.
Currently, these fundamental properties of cell-free DNA (cfDNA) analysis are translated to several applications in clinical oncology, generally termed as liquid biopsy, namely, molecular tumor profiling (4–6), therapy response monitoring (7–9), minimal residual disease (MRD) and recurrence detection (10–12), as well as early cancer diagnostics (representing a highly desirable but still elusive output) (13). Most of these clinical aims can be partially achieved through careful analysis of somatic mutations present in the tumor fraction of cfDNA (circulating tumor DNA, ctDNA) (14–16). The recent technological advances in the field fostered ultra-sensitive variant detection methods that nevertheless should be thoroughly validated in extensive independent studies before being fully adopted for reliable clinical use (17–20).
Aside from sequence alterations, tumorigenesis is characterized by early occurring and further extensive genome-wide epigenetic changes that tune expression programs in favor of tumor-specific phenotypes (21–23). Variations in CpG methylation, histone modifications, and chromatin remodeling occur mainly in a patterned and cell-type-specific fashion, making these processes vast sources of attractive cancer biomarkers. However, in contrast to sequence alterations, these changes are not encoded in DNA sequence and have to be extracted from cell-free DNA with indirect methods. Here we are going to briefly summarize currently available approaches and future prospects of cfDNA epigenetic analysis for cancer diagnostics.
Biology of Circulating DNA
The current understanding of cfDNA biology (3, 24, 25) and particularly epigenetics (26) has been recently reviewed elsewhere. However, to further discuss analytical methods, we have to emphasize some critical points. It is believed that the bulk of cell-free nucleic acids is formed as a byproduct during the course of cell death scenarios (27). The contribution of different cell types is still a matter of debate, as is the balance between active and passive release mechanisms and the possible functional roles being attributed to cfDNA subfractions by some researchers (3, 24, 25). Nevertheless, there seems to be a consensus on the high complexity of cfDNA pools found in biological fluids, which makes the tracing of sequence alterations and epigenetic marks found in cfDNA back to the cells of origin a challenging task.
The exact paths that DNA molecules may follow on their ways from the nucleus and mitochondria to the blood are not fully understood. The median cfDNA length of only ~165 bp reported in most studies (28–30) suggests that high molecular weight genomic DNA encounters nucleases during the shedding to the bloodstream. Surprisingly, despite being present in higher copy numbers in cells, mitochondrial DNA seems less accessible in plasma (31). This could be explained by the circular structure of mtDNA or its even higher fragmentation (32) due to the lack of nucleosomal structure and histone-mediated protection from nucleases in contrast to nuclear DNA. At the same time, mtDNA levels in plasma may provide clues on some pathological conditions, including cancer (33–37). Recent research highlights the roles of extrachromosomal DNA (ecDNA) in tumor progression (38–40). Often highly amplified and oncogene-enriched ecDNA molecules are detectable in blood plasma and may serve as an additional source of novel biomarkers after the development of appropriate analytical methods (41–43).
The turnover of cfDNA in blood seems to be rapid, with a half-life range of approximately 0,5-3 hours and a bias towards slower elimination of protein-bound DNA (44–46). If these estimates are true, any cfDNA test represents a nearly real-time snapshot of the cellular genomes. The sequence alterations and aberrant methylation that occurred in tumors seem to be apparently stable in ctDNA, while the inconsistency in alteration detection between tissue specimens and circulating DNA is most likely explained by the biological complexity of cancers and technical constraints (47–50). However, further studies are required to support the stability of tumor-specific alterations in cfDNA, especially aberrant methylation.
Principles of Detection and Challenges
The detection of tumor-specific changes is the key for most cfDNA applications in oncology, but it is complicated by individual variability of ctDNA fraction, heterogeneity of cancer genomes, and the limited amount of cfDNA usually available for analysis. Our simulations suggest that the reliable detection of a single point mutation is theoretically limited by a ctDNA fraction of 0,1% for typically sampled cfDNA amounts (Figure 1). In contrast, the detection of aberrant cytosine methylation may result in higher overall sensitivity due to the tendency of the densely clustered CpG sites to share the same methylation state at least at the distances of up to 50-100 nucleotides (2, 54–57). Consequently, tumor-derived DNA fragments from differentially methylated regions (DMRs) technically carry a number of point epimutations in contrast to single nucleotide substitutions. It increases the theoretical probability of tumor DNA detection with epigenetic methylation-based assays (Figure 1). Moreover, the ensemble nature of epigenetic changes in cancer leads to a patterned structure of DMRs across the genome that further multiplicates the number of tumor-specific markers available for detection (22, 58).
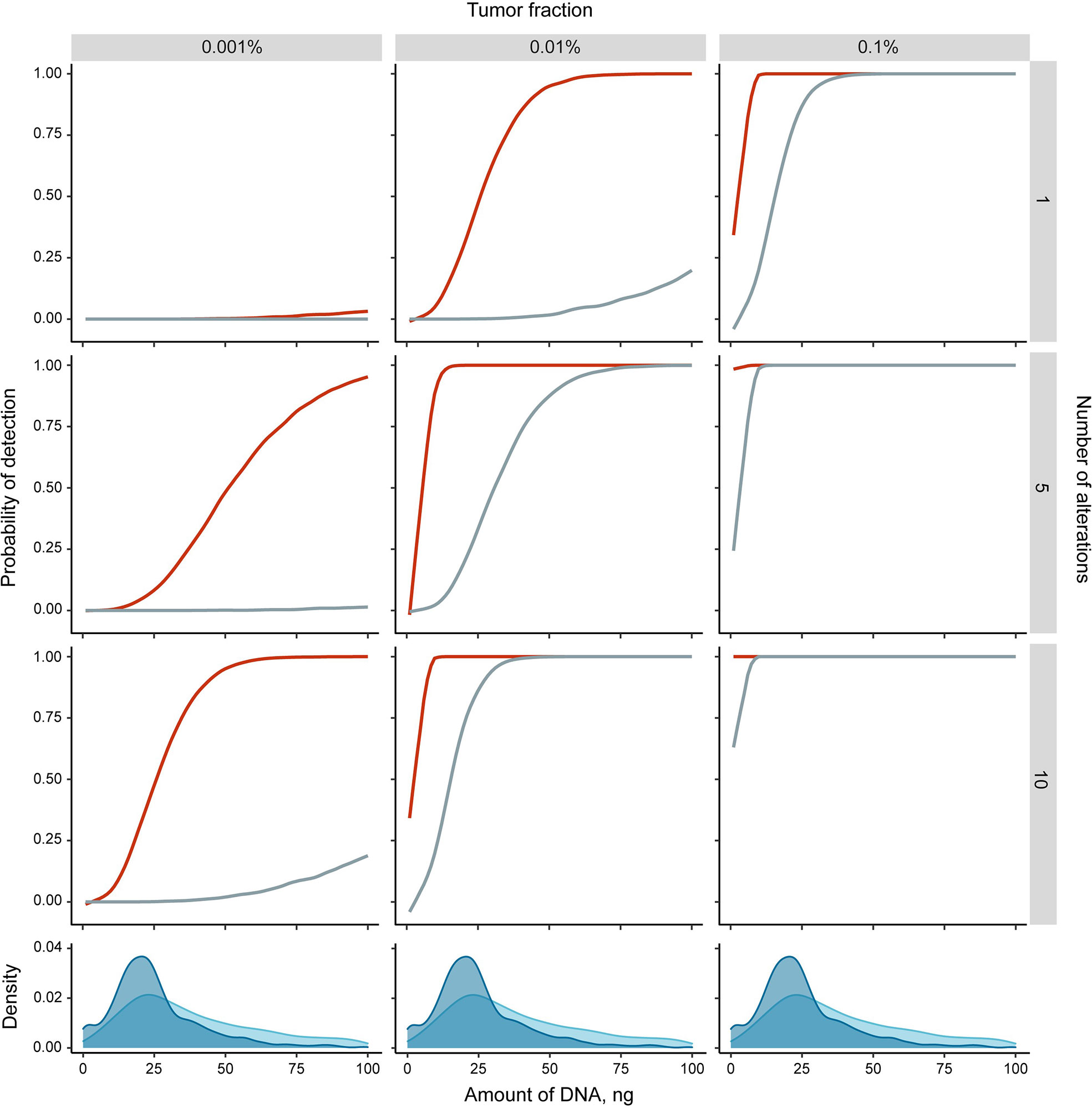
Figure 1 Theoretical simulations of the detection probability of single tumor-specific alteration (point mutation (grey) or differentially methylated region (DMR, red)) in cfDNA. Monte Carlo simulations were performed in R (see Supplementary Text for details) with the following assumptions: a DMR is represented by 6 co-methylated CpG sites (51); at least 5 observations of any alteration is required to classify the sample as cancer positive (adjustment for DNA loss during sample preparation and detection errors intrinsic to analytical methods); alterations are independent. Density plots at the bottom panels show the distributions of sampled cfDNA amounts in the four recent studies (16, 30, 52, 53) [3286 samples total, of which 1716 were from healthy individuals (blue) and 1570 from cancer patients (light blue)].
The rate of cfDNA release from the tumor, or cfDNA shedding, is another crucial factor for successful cancer detection. The ability to release cfDNA varies significantly among individuals and cancer types (16). The proposed explanations of the variability in ctDNA shedding kinetics among tumor types include distinct anatomical features (blood-brain barrier, organ capsules, vascularization), mitotic/cell death rates, metabolic characteristics, and the predominant cell death mechanisms (59, 60). Tumor clinicopathological parameters significantly associated with the increased ctDNA shedding are higher tumor stage, nodal metastases, solid adenocarcinoma pattern, tumor necrosis, larger primary tumor diameter or volume, and frequent mitosis in tissue specimens (61). The detection of ctDNA was considerably higher in certain malignancies like pancreatic, ovarian, colorectal, bladder, gastroesophageal, breast, melanoma, hepatocellular, and head and neck cancers. In contrast, ctDNA was detected in only <50% of the primary brain, renal, prostate, or thyroid cancers (62). A recently proposed mathematical model of cfDNA shedding suggests that the probability of a false negative for a particular actionable mutation clonally present in tumors with diameters of 1 and 2 cm is 82 and 9.3%, respectively (at 99% specificity) (63).
Taking the above-mentioned into consideration, minimizing DNA loss and suppressing analytical errors during all steps of analysis is crucial for robust identification of lower tumor fractions. The recovery and purity of cfDNA during the preanalytical step are strongly dependent on careful protocol selection and validation (64–67). For instance, undesirable lymphocyte gDNA contamination can be avoided by either minimizing time before plasma separation to less than 4 hours or storage in stabilizer-containing tubes (68). It has been shown that size-selection in favor of shorter fragments (90-150 bp range) can enrich tumor fraction and consequently increase the sensitivity of upstream variant detection (28), which logically implies that the opposite is also true and suggests that the DNA size distribution should be controlled during sample processing.
Methylation-Based Approach
Methylation of cytosines serves as an additional layer of instructive annotations for hard-coded genetic information in a cell-type-specific manner (69). Genome-wide cataloging of methylation patterns typical for normal and pathological tissues may result in a reference atlas that could be used for tissue-of-origin deconvolution from cfDNA methylation and revealing tumor localization (1, 2, 51, 70–72). However, the heterogeneity of cell types comprising most tissues increases noise and complicates identifying common specific patterns (1). An opposite idea, finding a universal methylation signature shared by multiple cancer types, may result in the development of pan-cancer early detection tests (52). Notably, the methylation profiles of cfDNA fraction derived from normal tissues must be taken into account as an inevitable background when determining DMRs. Despite the experimental wide-range approaches driving the field of liquid biopsy research, the analysis of limited marker sets is still more feasible in a practical setting due to lower costs and more straightforward interpretation. The genome-wide analysis of tumor-specific methylation, however, may yield novel candidates for designing narrow assays based on PCR or targeted sequencing of a small number of DMRs (73), thus expanding the selection of epigenetic cancer biomarkers such as methylation of SHOX2 and SEPT9 loci among others (74–77).
The two primary forms of modified cytosine in the human genome are 5-methylcytosine (5mC) and 5-hydroxymethylcytosine (5hmC). The latter can be considered not only as a product of 5mC oxidation by TET dioxygenases during demethylation but also as an independent epigenetic mark of the loci being activated (78–81). Nevertheless, these modifications are not directly detectable by most of the widespread sequencing or PCR-based methods, which makes some type of 5mC/5hmC-discriminating modification or enrichment a necessary step in the protocols. Chemical bisulfite conversion of unmethylated C to U underlies most PCR-based methods, methylation arrays, and sequencing approaches (82). Further development resulted in bisulfite-based oxBS-seq and TAB-seq protocols that differentiate between 5mC and 5hmC (83, 84). However, the related DNA loss of up to 90% (85–87) is a crucial obstacle for the analysis of low-input cfDNA samples. Another drawback intrinsic to the tactic of unmodified cytosine to uracil conversion is the reduced complexity of the output DNA sequence, which perplexes probe design and bioinformatic analysis (88, 89). The recently proposed conversion methods rely on enzymatic or combined treatments, which are reported to be less disruptive for DNA integrity. Particularly, TET dioxygenases can convert 5mC and 5hmC further to 5-carboxylcytosine (90), which can be either converted to dihydrouracils in TAPS protocol or protected from APOBEC-mediated deamination of C to U in EM-seq (91–93). Both methods with some modifications allow for 5mC and 5hmC discrimination.
Alternative approaches to methylation analysis rely on affinity enrichment, for instance, with 5mC-antibodies as proposed in cfMeDIP-seq (94, 95). Notably, the direct comparison suggests higher sensitivity of this method compared to sequence variant analysis (96), and further studies confirmed its utility for the detection of low-shedding renal (97) and intracranial tumors (98). In the 5hmC-Seal hydroxymethylcytosine, residues are selectively labeled with biotin and further captured on avidin beads (99, 100). The feasibility of this method for cancer detection was demonstrated in several studies (101–103). Moreover, a combination of cfMeDIP-seq and 5hm-Seal for simultaneous 5mC and 5hmC profiling in pancreatic cancer improved the prediction accuracy (104). MBD-seq takes advantage of the methyl-binding proteins such as MBD2 to capture methylated DNA (105–107). The protocol has been modified for low DNA input and showed performance similar to bisulfite sequencing (108), but its utility for cfDNA analysis has not been thoroughly evaluated. In contrast to most conversion strategies, affinity-based enrichment for methylated sequences may be more cost-effective at a whole-genome scale in applications where single-base resolution is not required since it allows to sequence predominantly methylated regions. On the other hand, it does not selectively target regions of interest and requires specific statistical tools capable of analyzing enrichment data.
Fragmentation-Based Approach
Genome-wide cfDNA sequencing revealed a biased fragmentation pattern that correlates with chromatin organization levels from nucleosomal occupancy to high order 3D structure (109–111). These observations can be explained by more efficient cleavage of accessible DNA in open-chromatin regions in contrast to better-protected protein-bound DNA in a closed inactive conformation. In turn, the changes in chromatin accessibility reflect shifts in transcription regulation (112), thus indirectly connecting cfDNA fragmentation features to gene expression programs in parental cells. Moreover, for reasons that are still unclear, circulating tumor DNA fragments tend to be shorter than cfDNA originating from normal tissues (28–30, 113, 114). It can be related to globally altered methylation and histone modifications [epigenetic changes that may alter the tightness of DNA wrapping around the nucleosomes (24, 115, 116)] or to aberrant mechanisms of DNA fragmentation in tumors and their microenvironments. Moreover, some researchers associate the observed difference with immune activity (114). Either way, cfDNA fragmentation features reflect massive epigenetic changes in tumor cells and may be considered as novel types of tumor markers (117, 118).
Several enzymes that are likely responsible for cfDNA fragmentation have been recently extensively studied in murine models (119–121). Generally, they could be attributed to three types based on localization (24). The first ones act in the cells of cfDNA origin during active cell death (e.g., apoptosis), with the caspase-activated DNase being one of the most widely known. Other nucleases cleave DNA during phagocytosis or in the extracellular space (e.g., deoxyribonuclease 1, deoxyribonuclease 1 like 3), and some secreted enzymes retain activity in the blood. Evidence suggests moderate sequence specificity of the enzymes acting in blood and apoptotic cells (119, 120), while tissue and macrophage-localized nucleases seem to introduce additional diversity to preferred ctDNA end sequences in cancer (122). As a result, cfDNA sampled from the bloodstream may bear the signs of consecutive exposure to a number of nucleases.
To date, several strategies have been proposed to apply these concepts for tumor detection. Cristiano et al. investigated cfDNA fragmentation in cancer patients at a whole genome scale (30). Their classifier predicted tumor types based on the ratios of longer and shorter fragments in the bins across the genome sequenced with low coverage. Analysis of cfDNA fragmentation focused on tumor-specific transcription factor binding sites revealed patterns that may reflect critical changes in tumor cells’ epigenetic regulation (117). Another consequence of non-random cfDNA fragmentation is an uneven distribution of the ends of fragments across the genome. It has been shown that the preferred DNA end coordinates may be characteristic of the tissue of origin (123). Moreover, accounting for the orientation of fragments may facilitate the detection of tissue-specific cfDNA fraction (124). The sequence specificity of nucleases involved in cfDNA formation can also be exploited to detect tumor presence. For instance, a biased distribution and increased diversity of sequence motifs were described in the ends of cfDNA fragments in patients with liver cancer (122). The technical loss of short (<100 bp) and degraded (nicked, partially single-stranded) DNA fragments during sample preparation for next-generation sequencing results in their underrepresentation in the final library. Similar issues in handling ancient DNA are addressed mostly by certain enzymes’ ability to ligate single-stranded templates (125, 126). Based on these developments and original ideas, novel methods are constantly proposed to increase the recovery of shorter cfDNA fragments enabling precise profiling of fragment size distributions (127–130).
Conclusions and Prospects
The presence of tumor-derived DNA molecules in the plasma of cancer patients allows tumor detection and profiling with non-invasive blood tests. In practice, it is complicated by several biological factors that affect reproducibility and require ultrasensitive assays for reliable detection. Various ctDNA analysis strategies are optimal in different clinical scenarios due to the diversity of underlying biological and methodological foundations. Despite only a fraction of liquid biopsy capabilities being utilized in clinical cancer care by now, some prospects may be extrapolated. The detection of somatic mutations in ctDNA may reveal the genomic profile of the tumor, facilitating prognosis, response monitoring, and targeted therapy selection, making mutation-based ctDNA analysis techniques arguably the most widely adopted to date (131). MRD detection may also be based on tumor-specific mutations detection (132). With the development of novel targeted therapies and accumulation of the knowledge interconnecting clinical outcomes and genomic biomarkers, the practice of ctDNA mutation-based analysis will expand, supporting clinical decisions for more cancer types beyond lung, breast, gastric, and colon cancers. At the same time, rigorous attention should be given to interpreting mutations that may occur in normal tissues (133), especially during clonal hematopoiesis (134). Beyond the analysis of tumor-specific sequence alterations in ctDNA, epigenetic marks may be favorable for many applications due to their cell-type specificity and patterned nature. The landscape of available epigenetic-based cfDNA assays is represented mainly by cytosine methylation tests targeting a narrow set of well-established differentially methylated loci or more complex wide-range approaches that infer from ensembles of individual methylation markers [Figure 2 and Supplementary Table 1; recently reviewed in detail in (135, 136)]. Recent extensive early detection efforts are based on the analysis of the broad panels of differentially methylated regions (51, 52), and this strategy may result in reliable screening tests. Analysis of methylation markers in cfDNA can also facilitate prognosis, recurrence monitoring, and management of cancers of unknown primary (137–140). The rising field of cfDNA fragmentomics has already yielded some promising approaches with comparable overall performance. Further developments in the field may include targeted fragmentation assays focused on the differentially fragmented regions and novel methods of deciphering epigenetic marks from fragment size distributions, end motifs, or new fragmentomic features. Although the fragmentation-based ctDNA analysis is still far from adoption for routine clinical use itself, it may be incorporated as an additional dimension to mutation-based or methylation-based liquid biopsy assays (28, 141). Furthermore, the possibility to improve tumor detection and characterization may lie in the simultaneous analysis of multiple marker types available from liquid biopsies, including proteins, circulating RNAs, tumor cells, and vesicles.
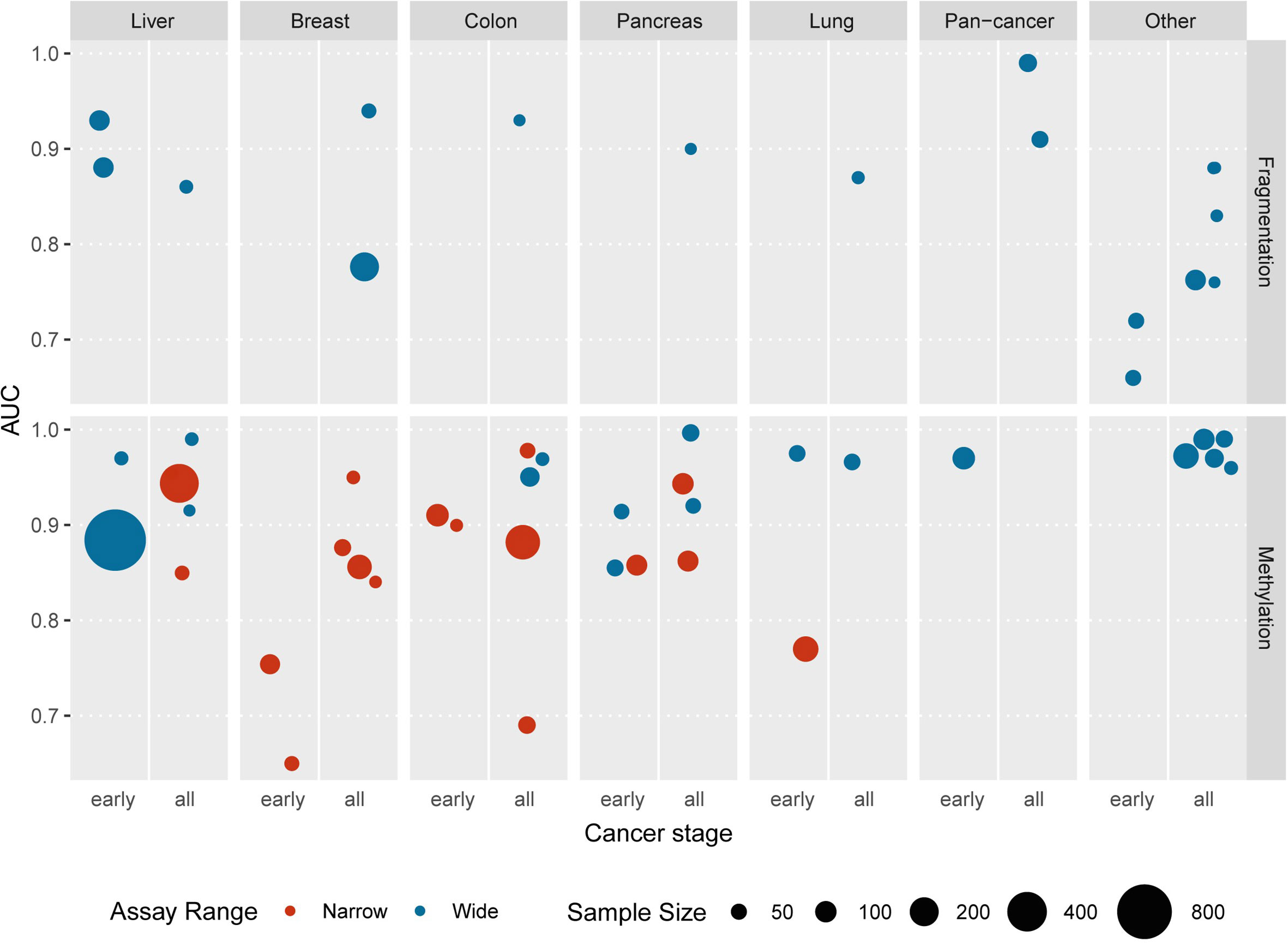
Figure 2 The recent studies reporting the application of epigenetic cfDNA assays for the detection of various tumor types are represented as areas under the ROC curves (AUCs) indicated in the respective publications. “Pan-cancer” category includes unified tests aimed at the detection of several cancer types; “other” category includes esophagus, bile duct, blood, central nervous system, gastric, kidney, ovarian, and urothelial cancers. The reported stages of cancers are grouped into two categories: “early” — pre-diagnosis or stage I or stage II and “all” — any other combination of stages or unspecified. The number of cancer patients involved in each study is plotted as the areas of the circles. Color represents the number of genomic loci included into each assay: red denotes narrow assays involving 20 loci or less, blue indicates whole-genome assays or larger targeted panels (more than 20 regions). Underlying data summarized in Supplementary Table 1.
Cancer diagnostics may greatly benefit from the comprehensive characterization of hallmark events occurring in the early stages of tumorigenesis. As our understanding of these processes expands, future research in liquid biopsy may focus on identifying signs of premalignant growths’ progression in cell-free DNA. The interception of metastases is another crucial component of improving cancer management that can be further enhanced by liquid biopsy. We suppose that epigenetic-based approaches to the analysis of cfDNA features will play an increasingly important role in translating fundamental findings to clinical settings.
Author Contributions
AK and DS wrote the main text. DS and NK conceptualized, planned, and supervised the preparation of the manuscript. KB, AK, and DS gathered data. DS and KB designed and prepared figures. NK and DS critically revised the manuscript. All authors contributed to the article and approved the submitted version.
Funding
This study was supported by a grant from the Russian Science Foundation (project #20-75-10008).
Conflict of Interest
The authors declare that the research was conducted in the absence of any commercial or financial relationships that could be construed as a potential conflict of interest.
Supplementary Material
The Supplementary Material for this article can be found online at: https://www.frontiersin.org/articles/10.3389/fonc.2021.662094/full#supplementary-material
References
1. Moss J, Magenheim J, Neiman D, Zemmour H, Loyfer N, Korach A, et al. Comprehensive Human Cell-Type Methylation Atlas Reveals Origins of Circulating Cell-Free DNA in Health and Disease. Nat Commun (2018) 9:5068. doi: 10.1038/s41467-018-07466-6
2. Guo S, Diep D, Plongthongkum N, Fung H-L, Zhang K, Zhang K. Identification of Methylation Haplotype Blocks Aids in Deconvolution of Heterogeneous Tissue Samples and Tumor Tissue-of-Origin Mapping From Plasma DNA. Nat Genet (2017) 49:635–42. doi: 10.1038/ng.3805
3. Aucamp J, Bronkhorst AJ, Badenhorst CPS, Pretorius PJ. The Diverse Origins of Circulating Cell-Free DNA in the Human Body: A Critical Re-Evaluation of the Literature. Biol Rev Camb Philos Soc (2018) 93:1649–83. doi: 10.1111/brv.12413
4. Kleftogiannis D, Ho D, Liew JX, Poon PSY, Gan A, Ng RC-H, et al. Detection of Genomic Alterations in Breast Cancer With Circulating Tumour DNA Sequencing. Sci Rep (2020) 10:16774. doi: 10.1038/s41598-020-72818-6
5. Garcia-Gisbert N, Fernández-Ibarrondo L, Fernández-Rodríguez C, Gibert J, Andrade-Campos M, Arenillas L, et al. Circulating Cell-Free DNA Improves the Molecular Characterisation of Ph-negative Myeloproliferative Neoplasms. Br J Haematol (2020) 192:300–9. doi: 10.1111/bjh.17087
6. Kang J-K, Heo S, Kim H-P, Song S-H, Yun H, Han S-W, et al. Liquid Biopsy-Based Tumor Profiling for Metastatic Colorectal Cancer Patients With Ultra-Deep Targeted Sequencing. PloS One (2020) 15:e0232754. doi: 10.1371/journal.pone.0232754
7. Welter L, Xu L, McKinley D, Dago AE, Prabakar RK, Restrepo-Vassalli S, et al. Treatment Response and Tumor Evolution: Lessons From an Extended Series of Multianalyte Liquid Biopsies in a Metastatic Breast Cancer Patient. Cold Spring Harb Mol Case Stud (2020) 6(6):a005819. doi: 10.1101/mcs.a005819
8. Weiss GJ, Beck J, Braun DP, Bornemann-Kolatzki K, Barilla H, Cubello R, et al. Tumor Cell-Free Dna Copy Number Instability Predicts Therapeutic Response to Immunotherapy. Clin Cancer Res (2017) 23:5074–81. doi: 10.1158/1078-0432.CCR-17-0231
9. Oellerich M, Schütz E, Beck J, Kanzow P, Plowman PN, Weiss GJ, et al. Using Circulating Cell-Free DNA to Monitor Personalized Cancer Therapy. Crit Rev Clin Lab Sci (2017) 54:205–18. doi: 10.1080/10408363.2017.1299683
10. Leal A, van Grieken NCT, Palsgrove DN, Phallen J, Medina JE, Hruban C, et al. White Blood Cell and Cell-Free DNA Analyses for Detection of Residual Disease in Gastric Cancer. Nat Commun (2020) 11:525. doi: 10.1038/s41467-020-14310-3
11. Abbosh C, Birkbak NJ, Swanton C. Early Stage NSCLC — Challenges to Implementing ctDNA-based Screening and MRD Detection. Nat Rev Clin Oncol (2018) 15:577–86. doi: 10.1038/s41571-018-0058-3
12. Reinert T, Schøler LV, Thomsen R, Tobiasen H, Vang S, Nordentoft I, et al. Analysis of Circulating Tumour DNA to Monitor Disease Burden Following Colorectal Cancer Surgery. Gut (2016) 65:625–34. doi: 10.1136/gutjnl-2014-308859
13. Chabon JJ, Hamilton EG, Kurtz DM, Esfahani MS, Moding EJ, Stehr H, et al. Integrating Genomic Features for non-Invasive Early Lung Cancer Detection. Nature (2020) 580:245–51. doi: 10.1038/s41586-020-2140-0
14. Wan JCM, Heider K, Gale D, Murphy S, Fisher E, Mouliere F, et al. ctDNA Monitoring Using Patient-Specific Sequencing and Integration of Variant Reads. Sci Transl Med (2020) 12:eaaz8084. doi: 10.1126/scitranslmed.aaz8084
15. Parsons HA, Rhoades J, Reed SC, Gydush G, Ram P, Exman P, et al. Sensitive Detection of Minimal Residual Disease in Patients Treated for Early-Stage Breast Cancer. Clin Cancer Res (2020) 26:2556–64. doi: 10.1158/1078-0432.CCR-19-3005
16. Cohen JD, Li L, Wang Y, Thoburn C, Afsari B, Danilova L, et al. Detection and Localization of Surgically Resectable Cancers With a Multi-Analyte Blood Test. Science (2018) 359:926–30. doi: 10.1126/science.aar3247
17. Weber S, Spiegl B, Perakis SO, Ulz CM, Abuja PM, Kashofer K, et al. Technical Evaluation of Commercial Mutation Analysis Platforms and Reference Materials for Liquid Biopsy Profiling. Cancers (2020) 12(6):1588. doi: 10.3390/cancers12061588
18. Gale D, Lawson ARJ, Howarth K, Madi M, Durham B, Smalley S, et al. Development of a Highly Sensitive Liquid Biopsy Platform to Detect Clinically-Relevant Cancer Mutations At Low Allele Fractions in Cell-Free DNA. PloS One (2018) 13:e0194630. doi: 10.1371/journal.pone.0194630
19. Reckamp KL, Melnikova VO, Karlovich C, Sequist LV, Camidge DR, Wakelee H, et al. A Highly Sensitive and Quantitative Test Platform for Detection of NSCLC EGFR Mutations in Urine and Plasma. J Thorac Oncol (2016) 11:1690–700. doi: 10.1016/j.jtho.2016.05.035
20. Newman AM, Bratman SV, To J, Wynne JF, Eclov NCW, Modlin LA, et al. An Ultrasensitive Method for Quantitating Circulating Tumor DNA With Broad Patient Coverage. Nat Med (2014) 20:548–54. doi: 10.1038/nm.3519
21. Dawson MA, Kouzarides T. Cancer Epigenetics: From Mechanism to Therapy. Cell (2012) 150:12–27. doi: 10.1016/j.cell.2012.06.013
22. Jones PA, Baylin SB. The Epigenomics of Cancer. Cell (2007) 128:683–92. doi: 10.1016/j.cell.2007.01.029
23. Sharma S, Kelly TK, Jones PA. Epigenetics in Cancer. Carcinogenesis (2010) 31:27–36. doi: 10.1093/carcin/bgp220
24. Heitzer E, Auinger L, Speicher MR. Cell-Free DNA and Apoptosis: How Dead Cells Inform About the Living. Trends Mol Med (2020) 26:519–28. doi: 10.1016/j.molmed.2020.01.012
25. Kustanovich A, Schwartz R, Peretz T, Grinshpun A. Life and Death of Circulating Cell-Free DNA. Cancer Biol Ther (2019) 20:1057–67. doi: 10.1080/15384047.2019.1598759
26. van der Pol Y, Mouliere F. Toward the Early Detection of Cancer by Decoding the Epigenetic and Environmental Fingerprints of Cell-Free Dna. Cancer Cell (2019) 36:350–68. doi: 10.1016/j.ccell.2019.09.003
27. Jahr S, Hentze H, Englisch S, Hardt D, Fackelmayer FO, Hesch RD, et al. DNA Fragments in the Blood Plasma of Cancer Patients: Quantitations and Evidence for Their Origin From Apoptotic and Necrotic Cells. Cancer Res (2001) 61:1659–65.
28. Mouliere F, Chandrananda D, Piskorz AM, Moore EK, Morris J, Ahlborn LB, et al. Enhanced Detection of Circulating Tumor DNA by Fragment Size Analysis. Sci Transl Med (2018) 10:eaat4921. doi: 10.1126/scitranslmed.aat4921
29. Underhill HR, Kitzman JO, Hellwig S, Welker NC, Daza R, Baker DN, et al. Fragment Length of Circulating Tumor Dna. PloS Genet (2016) 12:1–24. doi: 10.1371/journal.pgen.1006162
30. Cristiano S, Leal A, Phallen J, Fiksel J, Adleff V, Bruhm DC, et al. Genome-Wide Cell-Free DNA Fragmentation in Patients With Cancer. Nature (2019) 570:385–9. doi: 10.1038/s41586-019-1272-6
31. Weerts MJA, Timmermans EC, van de Stolpe A, Vossen RHAM, Anvar SY, Foekens JA, et al. Tumor-Specific Mitochondrial Dna Variants are Rarely Detected in Cell-Free Dna. Neoplasia (2018) 20:687–96. doi: 10.1016/j.neo.2018.05.003
32. Zhang R, Nakahira K, Guo X, Choi AMK, Gu Z. Very Short Mitochondrial DNA Fragments and Heteroplasmy in Human Plasma. Sci Rep (2016) 6:36097. doi: 10.1038/srep36097
33. An Q, Hu Y, Li Q, Chen X, Huang J, Pellegrini M, et al. The Size of Cell-Free Mitochondrial DNA in Blood is Inversely Correlated With Tumor Burden in Cancer Patients. Precis Clin Med (2019) 2:131–9. doi: 10.1093/pcmedi/pbz014
34. Ellinger J, Müller DC, Müller SC, Hauser S, Heukamp LC, von Ruecker A, et al. Circulating Mitochondrial DNA in Serum: A Universal Diagnostic Biomarker for Patients With Urological Malignancies. Urol Oncol (2012) 30:509–15. doi: 10.1016/j.urolonc.2010.03.004
35. Kageyama Y, Kasahara T, Kato M, Sakai S, Deguchi Y, Tani M, et al. The Relationship Between Circulating Mitochondrial DNA and Inflammatory Cytokines in Patients With Major Depression. J Affect Disord (2018) 233:15–20. doi: 10.1016/j.jad.2017.06.001
36. Lowes H, Pyle A, Santibanez-Koref M, Hudson G. Circulating Cell-Free Mitochondrial DNA Levels in Parkinson’s Disease are Influenced by Treatment. Mol Neurodegeneration (2020) 15:10. doi: 10.1186/s13024-020-00362-y
37. Lindqvist D, Wolkowitz OM, Picard M, Ohlsson L, Bersani FS, Fernström J, et al. Circulating Cell-Free Mitochondrial DNA, But Not Leukocyte Mitochondrial DNA Copy Number, is Elevated in Major Depressive Disorder. Neuropsychopharmacology (2018) 43:1557–64. doi: 10.1038/s41386-017-0001-9
38. Kumar P, Kiran S, Saha S, Su Z, Paulsen T, Chatrath A, et al. ATAC-Seq Identifies Thousands of Extrachromosomal Circular DNA in Cancer and Cell Lines. Sci Adv (2020) 6:eaba2489. doi: 10.1126/sciadv.aba2489
39. Wu S, Turner KM, Nguyen N, Raviram R, Erb M, Santini J, et al. Circular ecDNA Promotes Accessible Chromatin and High Oncogene Expression. Nature (2019) 575:699–703. doi: 10.1038/s41586-019-1763-5
40. Koche RP, Rodriguez-Fos E, Helmsauer K, Burkert M, MacArthur IC, Maag J, et al. Extrachromosomal Circular DNA Drives Oncogenic Genome Remodeling in Neuroblastoma. Nat Genet (2020) 52:29–34. doi: 10.1038/s41588-019-0547-z
41. Zhu J, Zhang F, Du M, Zhang P, Fu S, Wang L. Molecular Characterization of Cell-Free eccDNAs in Human Plasma. Sci Rep (2017) 7:10968. doi: 10.1038/s41598-017-11368-w
42. Kumar P, Dillon LW, Shibata Y, Jazaeri AA, Jones DR, Dutta A. Normal and Cancerous Tissues Release Extrachromosomal Circular Dna (eccDNA) Into the Circulation. Mol Cancer Res (2017) 15:1197–205. doi: 10.1158/1541-7786.MCR-17-0095
43. Sin STK, Jiang P, Deng J, Ji L, Cheng SH, Dutta A, et al. Identification and Characterization of Extrachromosomal Circular DNA in Maternal Plasma. Proc Natl Acad Sci U S A (2020) 117:1658–65. doi: 10.1073/pnas.1914949117
44. Yao W, Mei C, Nan X, Hui L. Evaluation and Comparison of In Vitro Degradation Kinetics of DNA in Serum, Urine and Saliva: A Qualitative Study. Gene (2016) 590:142–8. doi: 10.1016/j.gene.2016.06.033
45. Khier S, Lohan L. Kinetics of Circulating Cell-Free DNA for Biomedical Applications: Critical Appraisal of the Literature. Future Sci OA (2018) 4:FSO295. doi: 10.4155/fsoa-2017-0140
46. Muhanna N, Di Grappa MA, Chan HHL, Khan T, Jin CS, Zheng Y, et al. Cell-Free DNA Kinetics in a Pre-Clinical Model of Head and Neck Cancer. Sci Rep (2017) 7:16723. doi: 10.1038/s41598-017-17079-6
47. Danese E, Minicozzi AM, Benati M, Montagnana M, Paviati E, Salvagno GL, et al. Comparison of Genetic and Epigenetic Alterations of Primary Tumors and Matched Plasma Samples in Patients With Colorectal Cancer. PloS One (2015) 10:e0126417. doi: 10.1371/journal.pone.0126417
48. Beltran H, Romanel A, Conteduca V, Casiraghi N, Sigouros M, Franceschini GM, et al. Circulating Tumor DNA Profile Recognizes Transformation to Castration-Resistant Neuroendocrine Prostate Cancer. J Clin Invest (2020) 130:1653–68. doi: 10.1172/JCI131041
49. Danese E, Minicozzi AM, Benati M, Montagnana M, Paviati E, Salvagno GL, et al. Epigenetic Alteration: New Insights Moving From Tissue to Plasma - the Example of PCDH10 Promoter Methylation in Colorectal Cancer. Br J Cancer (2013) 109:807–13. doi: 10.1038/bjc.2013.351
50. Jensen SØ, Øgaard N, Ørntoft M-BW, Rasmussen MH, Bramsen JB, Kristensen H, et al. Novel DNA Methylation Biomarkers Show High Sensitivity and Specificity for Blood-Based Detection of Colorectal Cancer-a Clinical Biomarker Discovery and Validation Study. Clin Epigen (2019) 11:158. doi: 10.1158/1538-7445.AM2018-5604
51. Liu MC, Oxnard GR, Klein EA, Swanton C, Seiden MV, Liu MC, et al. Sensitive and Specific Multi-Cancer Detection and Localization Using Methylation Signatures in Cell-Free DNA. Ann Oncol (2020) 31:745–59. doi: 10.1016/j.annonc.2020.04.013
52. Chen X, Gole J, Gore A, He Q, Lu M, Min J, et al. Non-Invasive Early Detection of Cancer Four Years Before Conventional Diagnosis Using a Blood Test. Nat Commun (2020) 11:3475. doi: 10.1038/s41467-020-17316-z
53. Zviran A, Schulman RC, Shah M, Hill STK, Deochand S, Khamnei CC, et al. Genome-Wide Cell-Free DNA Mutational Integration Enables Ultra-Sensitive Cancer Monitoring. Nat Med (2020) 26:1114–24. doi: 10.1038/s41591-020-0915-3
54. Affinito O, Palumbo D, Fierro A, Cuomo M, De Riso G, Monticelli A, et al. Nucleotide Distance Influences Co-Methylation Between Nearby CpG Sites. Genomics (2020) 112:144–50. doi: 10.1016/j.ygeno.2019.05.007
55. Lövkvist C, Dodd IB, Sneppen K, Haerter JO. DNA Methylation in Human Epigenomes Depends on Local Topology of CpG Sites. Nucleic Acids Res (2016) 44:5123–32. doi: 10.1093/nar/gkw124
56. Eckhardt F, Lewin J, Cortese R, Rakyan VK, Attwood J, Burger M, et al. DNA Methylation Profiling of Human Chromosomes 6, 20 and 22. Nat Genet (2006) 38:1378–85. doi: 10.1038/ng1909
57. Gaspar JM, Hart RP. Dmrfinder: Efficiently Identifying Differentially Methylated Regions From MethylC-seq Data. BMC Bioinf (2017) 18:528. doi: 10.1186/s12859-017-1909-0
58. Hanahan D, Weinberg RA. Hallmarks of Cancer: The Next Generation. Cell (2011) 144:646–74. doi: 10.1016/j.cell.2011.02.013
59. Rostami A, Lambie M, Yu CW, Stambolic V, Waldron JN, Bratman SV. Senescence, Necrosis, and Apoptosis Govern Circulating Cell-Free DNA Release Kinetics. Cell Rep (2020) 31:107830. doi: 10.1016/j.celrep.2020.107830
60. Aucamp J, Bronkhorst AJ, Peters DL, Van Dyk HC, Van der Westhuizen FH, Pretorius PJ. Kinetic Analysis, Size Profiling, and Bioenergetic Association of DNA Released by Selected Cell Lines In Vitro. Cell Mol Life Sci (2017) 74:2689–707. doi: 10.1007/s00018-017-2495-z
61. Cho M-S, Park CH, Lee S, Park HS. Clinicopathological Parameters for Circulating Tumor DNA Shedding in Surgically Resected non-Small Cell Lung Cancer With EGFR or KRAS Mutation. PloS One (2020) 15:e0230622. doi: 10.1371/journal.pone.0230622
62. Bettegowda C, Sausen M, Leary RJ, Kinde I, Wang Y, Agrawal N, et al. Detection of Circulating Tumor DNA in Early- and Late-Stage Human Malignancies. Sci Transl Med (2014) 6:224ra24. doi: 10.1158/1538-7445.AM2014-5606
63. Avanzini S, Kurtz DM, Chabon JJ, Moding EJ, Hori SS, Gambhir SS, et al. A Mathematical Model of ctDNA Shedding Predicts Tumor Detection Size. Sci Adv (2020) 6:eabc4308. doi: 10.1126/sciadv.abc4308
64. Bronkhorst AJ, Ungerer V, Holdenrieder S. The Emerging Role of Cell-Free DNA as a Molecular Marker for Cancer Management. Biomol Detection Quantification (2019) 17:100087. doi: 10.1016/j.bdq.2019.100087
65. Rikkert LG, van der Pol E, van Leeuwen TG, Nieuwland R, Coumans FAW. Centrifugation Affects the Purity of Liquid Biopsy-Based Tumor Biomarkers. Cytometry A (2018) 93:1207–12. doi: 10.1002/cyto.a.23641
66. Meddeb R, Pisareva E, Thierry AR. Guidelines for the Preanalytical Conditions for Analyzing Circulating Cell-Free Dna. Clin Chem (2019) 65:623–33. doi: 10.1373/clinchem.2018.298323
67. Barták BK, Kalmár A, Galamb O, Wichmann B, Nagy ZB, Tulassay Z, et al. Blood Collection and Cell-Free Dna Isolation Methods Influence the Sensitivity of Liquid Biopsy Analysis for Colorectal Cancer Detection. Pathol Oncol Res (2019) 25:915–23. doi: 10.1007/s12253-018-0382-z
68. Alidousty C, Brandes D, Heydt C, Wagener S, Wittersheim M, Schäfer SC, et al. Comparison of Blood Collection Tubes From Three Different Manufacturers for the Collection of Cell-Free DNA for Liquid Biopsy Mutation Testing. J Mol Diagn (2017) 19:801–4. doi: 10.1016/j.jmoldx.2017.06.004
69. Dor Y, Cedar H. Principles of DNA Methylation and Their Implications for Biology and Medicine. Lancet (2018) 392:777–86. doi: 10.1016/S0140-6736(18)31268-6
70. Sun K, Jiang P, Chan KCA, Wong J, Cheng YKY, Liang RHS, et al. Plasma DNA Tissue Mapping by Genome-Wide Methylation Sequencing for Noninvasive Prenatal, Cancer, and Transplantation Assessments. Proc Natl Acad Sci U S A (2015) 112:E5503–12. doi: 10.1073/pnas.1508736112
71. Liu X, Ren J, Luo N, Guo H, Zheng Y, Li J, et al. Comprehensive DNA Methylation Analysis of Tissue of Origin of Plasma Cell-Free DNA by Methylated CpG Tandem Amplification and Sequencing (MCTA-Seq). Clin Epigen (2019) 11:93. doi: 10.1186/s13148-019-0689-y
72. Kang S, Li Q, Chen Q, Zhou Y, Park S, Lee G, et al. CancerLocator: non-Invasive Cancer Diagnosis and Tissue-of-Origin Prediction Using Methylation Profiles of Cell-Free DNA. Genome Biol (2017) 18:53. doi: 10.1186/s13059-017-1191-5
73. Luo H, Zhao Q, Wei W, Zheng L, Yi S, Li G, et al. Circulating Tumor DNA Methylation Profiles Enable Early Diagnosis, Prognosis Prediction, and Screening for Colorectal Cancer. Sci Transl Med (2020) 12:1–12. doi: 10.1126/scitranslmed.aax7533
74. Grützmann R, Molnar B, Pilarsky C, Habermann JK, Schlag PM, Saeger HD, et al. Sensitive Detection of Colorectal Cancer in Peripheral Blood by Septin 9 DNA Methylation Assay. PloS One (2008) 3:e3759. doi: 10.1371/journal.pone.0003759
75. deVos T, Tetzner R, Model F, Weiss G, Schuster M, Distler J, et al. Circulating Methylated SEPT9 DNA in Plasma is a Biomarker for Colorectal Cancer. Clin Chem (2009) 55:1337–46. doi: 10.1373/clinchem.2008.115808
76. Toyota M, Ho C, Ahuja N, Jair KW, Li Q, Ohe-Toyota M, et al. Identification of Differentially Methylated Sequences in Colorectal Cancer by Methylated CpG Island Amplification. Cancer Res (1999) 59:2307–12.
77. Schmidt B, Liebenberg V, Dietrich D, Schlegel T, Kneip C, Seegebarth A, et al. Shox2 DNA Methylation is a Biomarker for the Diagnosis of Lung Cancer Based on Bronchial Aspirates. BMC Cancer (2010) 10:600. doi: 10.1186/1471-2407-10-600
78. Bachman M, Uribe-Lewis S, Yang X, Williams M, Murrell A, Balasubramanian S. 5-Hydroxymethylcytosine is a Predominantly Stable DNA Modification. Nat Chem (2014) 6:1049–55. doi: 10.1038/nchem.2064
79. Jeschke J, Collignon E, Fuks F. Portraits of TET-mediated DNA Hydroxymethylation in Cancer. Curr Opin Genet Dev (2016) 36:16–26. doi: 10.1016/j.gde.2016.01.004
80. Wang J, Su Y, Tian Y, Ding Y, Wang X. Characterization of DNA Hydroxymethylation Profile in Cervical Cancer. Artif Cells Nanomed Biotechnol (2019) 47:2706–14. doi: 10.1080/21691401.2019.1634578
81. Branco MR, Ficz G, Reik W. Uncovering the Role of 5-Hydroxymethylcytosine in the Epigenome. Nat Rev Genet (2011) 13:7–13. doi: 10.1038/nrg3080
82. Berney M, McGouran JF. Methods for Detection of Cytosine and Thymine Modifications in DNA. Nat Rev Chem (2018) 2:332–48. doi: 10.1038/s41570-018-0044-4
83. Yu M, Hon GC, Szulwach KE, Song C-X, Jin P, Ren B, et al. Tet-Assisted Bisulfite Sequencing of 5-Hydroxymethylcytosine. Nat Protoc (2012) 7:2159–70. doi: 10.1038/nprot.2012.137
84. Booth MJ, Ost TWB, Beraldi D, Bell NM, Branco MR, Reik W, et al. Oxidative Bisulfite Sequencing of 5-Methylcytosine and 5-Hydroxymethylcytosine. Nat Protoc (2013) 8:1841–51. doi: 10.1038/nprot.2013.115
85. Tanaka K, Okamoto A. Degradation of DNA by Bisulfite Treatment. Bioorg Med Chem Lett (2007) 17:1912–5. doi: 10.1016/j.bmcl.2007.01.040
86. Grunau C, Clark SJ, Rosenthal A. Bisulfite Genomic Sequencing: Systematic Investigation of Critical Experimental Parameters. Nucleic Acids Res (2001) 29:E65–5. doi: 10.1093/nar/29.13.e65
87. Worm Ørntoft M-B, Jensen SØ, Hansen TB, Bramsen JB, Andersen CL. Comparative Analysis of 12 Different Kits for Bisulfite Conversion of Circulating Cell-Free DNA. Epigenetics (2017) 12:626–36. doi: 10.1080/15592294.2017.1334024
88. Bibikova M. “Chapter 2 - DNA Methylation Microarrays”. In: Fraga MF, Fernández AF, editors. Epigenomics in Health and Disease. Boston: Academic Press (2016). p. 19–46. doi: 10.1016/B978-0-12-800140-0.00002-9
89. Krueger F, Kreck B, Franke A, Andrews SR. DNA Methylome Analysis Using Short Bisulfite Sequencing Data. Nat Methods (2012) 9:145–51. doi: 10.1038/nmeth.1828
90. Ito S, Shen L, Dai Q, Wu SC, Collins LB, Swenberg JA, et al. Tet Proteins can Convert 5-Methylcytosine to 5-Formylcytosine and 5-Carboxylcytosine. Science (2011) 333:1300–3. doi: 10.1126/science.1210597
91. Liu Y, Siejka-Zielińska P, Velikova G, Bi Y, Yuan F, Tomkova M, et al. Bisulfite-Free Direct Detection of 5-Methylcytosine and 5-Hydroxymethylcytosine At Base Resolution. Nat Biotechnol (2019) 37:424–9. doi: 10.1038/s41587-019-0041-2
92. Hoppers A, Williams L, Chaithanya Ponnaluri VK, Sexton B, Saleh L, Campbell M, et al. Enzymatic Methyl-seq: Next Generation Methylomes. J Biomol Tech (2020) 31:S15.
93. Schutsky EK, Nabel CS, Davis AKF, DeNizio JE, Kohli RM. APOBEC3A Efficiently Deaminates Methylated, But Not TET-oxidized, Cytosine Bases in DNA. Nucleic Acids Res (2017) 45:7655–65. doi: 10.1093/nar/gkx345
94. Shen SY, Singhania R, Fehringer G, Chakravarthy A, Roehrl MHA, Chadwick D, et al. Sensitive Tumour Detection and Classification Using Plasma Cell-Free DNA Methylomes. Nature (2018) 563:579–83. doi: 10.1038/s41586-018-0703-0
95. Shen SY, Burgener JM, Bratman SV, De Carvalho DD. Preparation of cfMeDIP-seq Libraries for Methylome Profiling of Plasma Cell-Free DNA. Nat Protoc (2019) 14:2749–80. doi: 10.1038/s41596-019-0202-2
96. Lasseter K, Nassar AH, Hamieh L, Berchuck JE, Nuzzo PV, Korthauer K, et al. Plasma Cell-Free DNA Variant Analysis Compared With Methylated DNA Analysis in Renal Cell Carcinoma. Genet Med (2020) 22:1366–73. doi: 10.1038/s41436-020-0801-x
97. Nuzzo PV, Berchuck JE, Korthauer K, Spisak S, Nassar AH, Abou Alaiwi S, et al. Detection of Renal Cell Carcinoma Using Plasma and Urine Cell-Free DNA Methylomes. Nat Med (2020) 26:1041–3. doi: 10.1038/s41591-020-0933-1
98. Nassiri F, Chakravarthy A, Feng S, Shen SY, Nejad R, Zuccato JA, et al. Detection and Discrimination of Intracranial Tumors Using Plasma Cell-Free DNA Methylomes. Nat Med (2020) 26:1044–7. doi: 10.1038/s41591-020-0932-2
99. Li W, Zhang X, Lu X, You L, Song Y, Luo Z, et al. 5-Hydroxymethylcytosine Signatures in Circulating Cell-Free DNA as Diagnostic Biomarkers for Human Cancers. Cell Res (2017) 27:1243–57. doi: 10.1038/cr.2017.121
100. Song C-X, Szulwach KE, Fu Y, Dai Q, Yi C, Li X, et al. Selective Chemical Labeling Reveals the Genome-Wide Distribution of 5-Hydroxymethylcytosine. Nat Biotechnol (2011) 29:68–72. doi: 10.1038/nbt.1732
101. Gao P, Lin S, Cai M, Zhu Y, Song Y, Sui Y, et al. 5-Hydroxymethylcytosine Profiling From Genomic and Cell-Free DNA for Colorectal Cancers Patients. J Cell Mol Med (2019) 23:3530–7. doi: 10.1111/jcmm.14252
102. Cai J, Chen L, Zhang Z, Zhang X, Lu X, Liu W, et al. Genome-Wide Mapping of 5-Hydroxymethylcytosines in Circulating Cell-Free DNA as a non-Invasive Approach for Early Detection of Hepatocellular Carcinoma. Gut (2019) 68:2195–205. doi: 10.1136/gutjnl-2019-318882
103. Tian X, Sun B, Chen C, Gao C, Zhang J, Lu X, et al. Circulating Tumor DNA 5-Hydroxymethylcytosine as a Novel Diagnostic Biomarker for Esophageal Cancer. Cell Res (2018) 28:597–600. doi: 10.1038/s41422-018-0014-x
104. Cao F, Wei A, Hu X, He Y, Zhang J, Xia L, et al. Integrated Epigenetic Biomarkers in Circulating Cell-Free DNA as a Robust Classifier for Pancreatic Cancer. Clin Epigen (2020) 12:112. doi: 10.1186/s13148-020-00898-2
105. Brinkman AB, Simmer F, Ma K, Kaan A, Zhu J, Stunnenberg HG. Whole-Genome DNA Methylation Profiling Using Methylcap-Seq. Methods (2010) 52:232–6. doi: 10.1016/j.ymeth.2010.06.012
106. Serre D, Lee BH, Ting AH. MBD-Isolated Genome Sequencing Provides a High-Throughput and Comprehensive Survey of DNA Methylation in the Human Genome. Nucleic Acids Res (2010) 38:391–9. doi: 10.1093/nar/gkp992
107. Aberg KA, Chan RF, Xie L, Shabalin AA, van den Oord EJCG. Methyl-Cpg-Binding Domain Sequencing: MBD-Seq. Methods Mol Biol (2018) 1708:171–89. doi: 10.1007/978-1-4939-7481-8_10
108. Aberg KA, Chan RF, Shabalin AA, Zhao M, Turecki G, Staunstrup NH, et al. A MBD-seq Protocol for Large-Scale Methylome-Wide Studies With (Very) Low Amounts of DNA. Epigenetics (2017) 12:743–50. doi: 10.1080/15592294.2017.1335849
109. Snyder MW, Kircher M, Hill AJ, Daza RM, Shendure J. Cell-Free DNA Comprises an In Vivo Nucleosome Footprint That Informs its Tissues-of-Origin. Cell (2016) 164:57–68. doi: 10.1016/j.cell.2015.11.050
110. Ivanov M, Baranova A, Butler T, Spellman P, Mileyko V. Non-Random Fragmentation Patterns in Circulating Cell-Free DNA Reflect Epigenetic Regulation. BMC Genomics (2015) 16(Suppl 13):S1. doi: 10.1186/1471-2164-16-S13-S1
111. Teo YV, Capri M, Morsiani C, Pizza G, Faria AMC, Franceschi C, et al. Cell-Free DNA as a Biomarker of Aging. Aging Cell (2019) 18:e12890. doi: 10.1111/acel.12890
112. Klemm SL, Shipony Z, Greenleaf WJ. Chromatin Accessibility and the Regulatory Epigenome. Nat Rev Genet (2019) 20:207–20. doi: 10.1038/s41576-018-0089-8
113. Lo YMD, Chan KCA, Sun H, Chen EZ, Jiang P, Lun FMF, et al. Maternal Plasma DNA Sequencing Reveals the Genome-Wide Genetic and Mutational Profile of the Fetus. Sci Transl Med (2010) 2:61ra91. doi: 10.1126/scitranslmed.3001720
114. Guo J, Ma K, Bao H, Ma X, Xu Y, Wu X, et al. Quantitative Characterization of Tumor Cell-Free DNA Shortening. BMC Genomics (2020) 21:473. doi: 10.1186/s12864-020-06848-9
115. Otterstrom J, Castells-Garcia A, Vicario C, Gomez-Garcia PA, Cosma MP, Lakadamyali M. Super-Resolution Microscopy Reveals How Histone Tail Acetylation Affects DNA Compaction Within Nucleosomes In Vivo. Nucleic Acids Res (2019) 47:8470–84. doi: 10.1093/nar/gkz593
116. Shogren-Knaak M, Ishii H, Sun J-M, Pazin MJ, Davie JR, Peterson CL. Histone H4-K16 Acetylation Controls Chromatin Structure and Protein Interactions. Science (2006) 311:844–7. doi: 10.1126/science.1124000
117. Ulz P, Perakis S, Zhou Q, Moser T, Belic J, Lazzeri I, et al. Inference of Transcription Factor Binding From Cell-Free DNA Enables Tumor Subtype Prediction and Early Detection. Nat Commun (2019) 10:4666. doi: 10.1038/s41467-019-12714-4
118. Ulz P, Thallinger GG, Auer M, Graf R, Kashofer K, Jahn SW, et al. Inferring Expressed Genes by Whole-Genome Sequencing of Plasma DNA. Nat Genet (2016) 48:1273–8. doi: 10.1038/ng.3648
119. Han DSC, Ni M, Chan RWY, Chan VWH, Lui KO, Chiu RWK, et al. The Biology of Cell-free Dna Fragmentation and the Roles of DNASE1, DNASE1L3, and DFFB. Am J Hum Genet (2020) 106:202–14. doi: 10.1016/j.ajhg.2020.01.008
120. Serpas L, Chan RWY, Jiang P, Ni M, Sun K, Rashidfarrokhi A, et al. Dnase1l3 Deletion Causes Aberrations in Length and End-Motif Frequencies in Plasma DNA. Proc Natl Acad Sci U S A (2019) 116:641–9. doi: 10.1073/pnas.1815031116
121. Watanabe T, Takada S, Mizuta R. Cell-Free DNA in Blood Circulation is Generated by DNase1L3 and Caspase-Activated Dnase. Biochem Biophys Res Commun (2019) 516:790–5. doi: 10.1016/j.bbrc.2019.06.069
122. Jiang P, Sun K, Peng W, Cheng SH, Ni M, Yeung PC, et al. Plasma DNA End Motif Profiling as a Fragmentomic Marker in Cancer, Pregnancy and Transplantation. Cancer Discovery (2020) 10:664–73, CD–19–0622. doi: 10.1158/2159-8290.CD-19-0622
123. Jiang P, Sun K, Tong YK, Cheng SH, Cheng THT, Heung MMS, et al. Preferred End Coordinates and Somatic Variants as Signatures of Circulating Tumor DNA Associated With Hepatocellular Carcinoma. Proc Natl Acad Sci U S A (2018) 115:E10925–33. doi: 10.1073/pnas.1814616115
124. Sun K, Jiang P, Cheng SH, Cheng THT, Wong J, Wong VWS, et al. Orientation-Aware Plasma Cell-Free DNA Fragmentation Analysis in Open Chromatin Regions Informs Tissue of Origin. Genome Res (2019) 29:418–27. doi: 10.1101/gr.242719.118
125. Kuhn H, Frank-Kamenetskii MD. Template-Independent Ligation of Single-Stranded DNA by T4 DNA Ligase. FEBS J (2005) 272:5991–6000. doi: 10.1111/j.1742-4658.2005.04954.x
126. Gansauge M-T, Meyer M. Single-Stranded DNA Library Preparation for the Sequencing of Ancient or Damaged DNA. Nat Protoc (2013) 8:737–48. doi: 10.1038/nprot.2013.038
127. Wu J, Dai W, Wu L, Wang J. SALP, a New Single-Stranded DNA Library Preparation Method Especially Useful for the High-Throughput Characterization of Chromatin Openness States. BMC Genomics (2018) 19:143. doi: 10.1186/s12864-018-4688-8
128. Wu J, Dai W, Wu L, Li W, Xia X, Wang J. Decoding Genetic and Epigenetic Information Embedded in Cell Free DNA With Adapted SALP-Seq. Int J Cancer (2019) 145:2395–406. doi: 10.1002/ijc.32206
129. Burnham P, Kim MS, Agbor-Enoh S, Luikart H, Valantine HA, Khush KK, et al. Single-Stranded DNA Library Preparation Uncovers the Origin and Diversity of Ultrashort Cell-Free DNA in Plasma. Sci Rep (2016) 6:27859. doi: 10.1038/srep27859
130. Troll CJ, Kapp J, Rao V, Harkins KM, Cole C, Naughton C, et al. A Ligation-Based Single-Stranded Library Preparation Method to Analyze Cell-Free DNA and Synthetic Oligos. BMC Genomics (2019) 20:1023. doi: 10.1186/s12864-019-6355-0
131. Cheng ML, Pectasides E, Hanna GJ, Parsons HA, Choudhury AD, Oxnard GR. Circulating Tumor DNA in Advanced Solid Tumors: Clinical Relevance and Future Directions. CA Cancer J Clin (2021) 71:176–90. doi: 10.3322/caac.21650
132. Chaudhuri AA, Chabon JJ, Lovejoy AF, Newman AM, Stehr H, Azad TD, et al. Early Detection of Molecular Residual Disease in Localized Lung Cancer by Circulating Tumor DNA Profiling. Cancer Discovery (2017) 7:1394–403. doi: 10.1158/2159-8290.CD-17-0716
133. Yizhak K, Aguet F, Kim J, Hess JM, Kübler K, Grimsby J, et al. RNA Sequence Analysis Reveals Macroscopic Somatic Clonal Expansion Across Normal Tissues. Science (2019) 364:eaaw0726. doi: 10.1126/science.aaw0726
134. Zink F, Stacey SN, Norddahl GL, Frigge ML, Magnusson OT, Jonsdottir I, et al. Clonal Hematopoiesis, With and Without Candidate Driver Mutations, is Common in the Elderly. Blood (2017) 130:742–52. doi: 10.1182/blood-2017-02-769869
135. Galardi F, Luca FD, Romagnoli D, Biagioni C, Moretti E, Biganzoli L, et al. Cell-Free DNA-Methylation-Based Methods and Applications in Oncology. Biomolecules (2020) 10:1677. doi: 10.3390/biom10121677
136. Beltrán-García J, Osca-Verdegal R, Mena-Mollá S, García-Giménez JL. Epigenetic IVD Tests for Personalized Precision Medicine in Cancer. Front Genet (2019) 10:621. doi: 10.3389/fgene.2019.00621
137. Warton K, Mahon KL, Samimi G. Methylated Circulating Tumor DNA in Blood: Power in Cancer Prognosis and Response. Endocr Relat Cancer (2016) 23:R157–71. doi: 10.1530/ERC-15-0369
138. Jin S, Zhu D, Shao F, Chen S, Guo Y, Li K, et al. Efficient Detection and Post-Surgical Monitoring of Colon Cancer With a Multi-Marker DNA Methylation Liquid Biopsy. Proc Natl Acad Sci U S A (2021) 118:e2017421118. doi: 10.1073/pnas.2017421118
139. Conway A-M, Mitchell C, Kilgour E, Brady G, Dive C, Cook N. Molecular Characterisation and Liquid Biomarkers in Carcinoma of Unknown Primary (CUP): Taking the “U” Out of “Cup.” Br J Cancer (2019) 120:141–53. doi: 10.1038/s41416-018-0332-2
140. Laprovitera N, Riefolo M, Ambrosini E, Klec C, Pichler M, Ferracin M. Cancer of Unknown Primary: Challenges and Progress in Clinical Management. Cancers (2021) 13:451. doi: 10.3390/cancers13030451
Keywords: cell-free DNA, liquid biopsy, epigenetics, biomarkers, cancer diagnostics and screening
Citation: Koval AP, Blagodatskikh KA, Kushlinskii NE and Shcherbo DS (2021) The Detection of Cancer Epigenetic Traces in Cell-Free DNA. Front. Oncol. 11:662094. doi: 10.3389/fonc.2021.662094
Received: 31 January 2021; Accepted: 12 April 2021;
Published: 29 April 2021.
Edited by:
Pasquale Simeone, University of Studies G. d’Annunzio Chieti and Pescara, ItalyReviewed by:
Jiaqi Liu, National Cancer Center of China, ChinaYinan Zheng, Northwestern University, United States
Copyright © 2021 Koval, Blagodatskikh, Kushlinskii and Shcherbo. This is an open-access article distributed under the terms of the Creative Commons Attribution License (CC BY). The use, distribution or reproduction in other forums is permitted, provided the original author(s) and the copyright owner(s) are credited and that the original publication in this journal is cited, in accordance with accepted academic practice. No use, distribution or reproduction is permitted which does not comply with these terms.
*Correspondence: Dmitry S. Shcherbo, dshcherbo@gmail.com