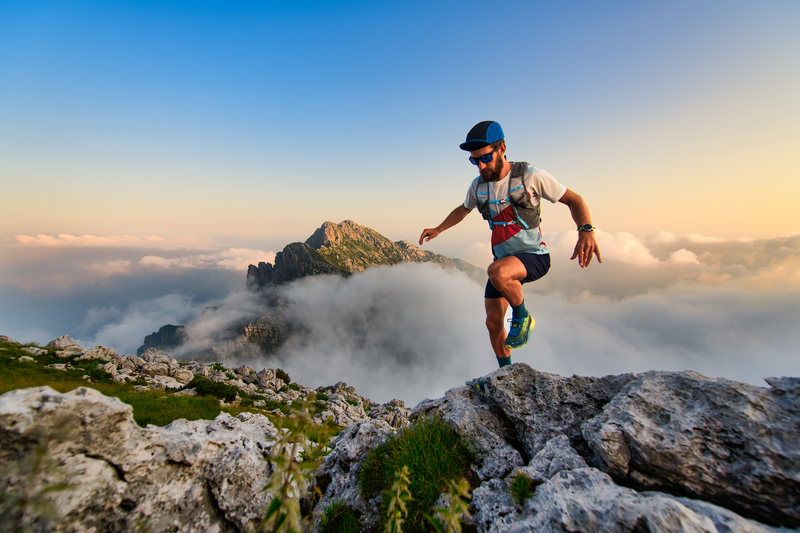
94% of researchers rate our articles as excellent or good
Learn more about the work of our research integrity team to safeguard the quality of each article we publish.
Find out more
ORIGINAL RESEARCH article
Front. Oncol. , 07 May 2021
Sec. Molecular and Cellular Oncology
Volume 11 - 2021 | https://doi.org/10.3389/fonc.2021.656172
Background: Mitochondrial ribosomal protein L15 (MRPL15), a member of mitochondrial ribosomal proteins whose abnormal expression is related to tumorigenesis. However, the prognostic value and regulatory mechanisms of MRPL15 in non-small-cell lung cancer (NSCLC) remain unclear.
Methods: GEPIA, ONCOMINE, Gene Expression Omnibus (GEO), UALCAN, Kaplan–Meier plotter, PrognoScan, LinkedOmics and GeneMANIA database were utilized to explore the expression and prognostic value of MRPL15 in NSCLC. Additionally, immune infiltration patterns were evaluated via ESTIMATE algorithm and TISIDB database. Furthermore, the expression and prognostic value of MRPL15 in lung cancer were validated via immunohistochemistry (IHC) assays.
Results: In NSCLC, multiple cohorts including GEPIA, ONCOMINE and 8 GEO series (GSE8569, GSE101929, GSE33532, GSE27262, GSE21933, GSE19804, GSE19188, GSE18842) described that MRPL15 was up-regulated. Moreover, MRPL15 was notably linked to gender, clinical stage, lymph node status and the TP53 mutation status. And patients with high MRPL15 expression showed poor overall survival (OS), progression-free survival (PFS), disease-free survival (DFS) and relapse-free survival (RFS) in NSCLC. Then, functional network analysis suggested that MRPL15 participated in metabolism-related pathways, DNA replication and cell cycle signaling via pathways involving several kinases, miRNAs and transcription factors. Additionally, it was found that MRPL15 expression was negatively related to immune infiltration, including immune scores, stromal scores and several tumor-infiltrating lymphocytes (TILs). Furthermore, IHC results further confirmed the high MRPL15 expression and its prognostic potential in lung cancer.
Conclusions: These findings demonstrate that high MRPL15 expression indicates poor prognosis in NSCLC and reveal potential regulatory networks as well as the negative relationship with immune infiltration. Thus, MRPL15 may be an attractive predictor and therapeutic strategy for NSCLC.
Lung cancer has been regarded as the leading cause of cancer deaths worldwide (1), in which NSCLC with aggressive clinical course and prominent association with tobacco use (2) is the main histological type (3). NSCLC includes several subtypes, among which lung adenocarcinoma (LUAD) and lung squamous cell carcinoma (LUSC) are the most prevalent. Due to a high proportion of patients with NSCLC are already metastatic at diagnosis, novel biomarkers that can reflect clinical status are urgently needed.
Alterations of mitochondrial DNA (mtDNA) possibly are related to carcinogenesis of lung cancer (4, 5). More and more data have revealed that tumorigenesis is partially dependent on the reprogramming of cellular metabolism as consequence of mitochondrial dysfunction (6, 7). MtDNA mutation may contribute to malignant transformation via ROS and mitochondrial metabolites (8, 9). Mitochondria not only can promote progression, but also functions in cell death signaling (10). Thus, mitochondria are supposed to be the regulators of cellular life and death in tumor cells. 13 proteins encoded by mtDNA (11), all of which are the mitochondrial respiratory chain enzymes. These proteins are synthesized in specialized mitochondrial ribosomes (mitoribosomes), which are composed of two rRNAs and mitochondrial ribosomal proteins (MRPs) (12).
Mutations in nuclear-encoded MRPs can lead to severe respiratory chain dysfunction (13) and multiple MRPs are important predictors of tumor diagnosis. Almost 80 MRP genes have been recognized, of which are divided into two main groups: components of the large subunit (MRPL) and components of the small subunit (MRPS) (14). Multiple MRPs abnormalities can be found in the same cancer. For instance, both MRPS30 and MRPL13 had been reported to be overexpressed and correlate to poor survival in breast cancer (15). Meanwhile, the same MRPs might work in various cancers. High MRPL13 promoted invasion in liver cancer cells (16). Apart from participating in mitochondrial oxidative phosphorylation (OXPHOS), the new roles for MRPs in cellular apoptosis and proliferation have been revealed (17). Actually, research has declared that MRPS29 promotes apoptosis through its interactions with Fas ligand (18).
MRPL15 is a member of MRPL, which provides energy in the form of ATP for cell growth. MRPL15 has been reported to have a predictive value in breast cancer metastasis (19) and can promote Burkitt lymphoma growth (20). Little research has reported the predictive value of MRPL15 in NSCLC. Only a recent study showed that MRPL15 was associated with progression in lung adenocarcinoma (21), but the prognostic value and regulatory mechanisms of MRPL15 in lung cancer are still unknown. In addition, immunological analysis of the tumor microenvironment shows great promise for better prognosis and immunotherapy benefit for NSCLC (22). However, few studies reveal the relationship between MRPs and immune infiltration patterns.
GEPIA (http://gepia.cancer-pku.cn/) is a web-based tool providing key interactive and customizable functions based on The Cancer Genome Atlas (TCGA) and the Genotype-Tissue Expression (GTEx) data (23). In this study, we explored the differential mRNA expression levels of MRPL15 in tumor and normal tissue datasets, especially LUAD and LUSC.
The mRNA expression of MRPL15 was assessed in lung carcinoma in the ONCOMINE database (www.oncomine.org), a cancer microarray database and integrated data-mining platform (24). This analysis drew on a study using the Hou Lung dataset (25).
Eight expression microarray series GSE8569, GSE101929, GSE33532, GSE27262, GSE21933, GSE19804, GSE19188 and GSE18842 containing NSCLC tumor and normal tissues with MRPL15 expression information were collected from the Gene Expression Omnibus dataset (GEO, https://www.ncbi.nlm.nih.gov/geo/). Information about selected GEO series is listed in Table 1. All the download datasets included NSCLC tumor and normal tissues, and the total number of samples in each dataset was more than 30.
UALCAN (http://ualcan.path.uab.edu) is a web portal to perform tumor subgroup gene expression and survival analyses based on TCGA data (26). Therefore, we analyzed the relative expression of MRPL15 in various tumor sub-groups based on gender, clinical stage, lymph node status and the TP53 mutation status of LUAD and LUSC.
The Kaplan–Meier plotter (http://kmplot.com/analysis/) (27) was applied for survival analysis of MRPL15 in lung cancer patients according to the hazard ratio (HR) with 95% confidence interval (CI) and log-rank P-values. GEPIA was also used for survival analysis. In addition, the prognostic potential of MRPL15 was validated in the PrognoScan database (http://www.abren.net/PrognoScan/) (28) with Cox P-value <0.05 as the threshold.
LinkedOmics (http://www.linkedomics.org/login.php) is a platform for analyzing cancer multi-omics data based on TCGA project (29). The LinkFinder module was applied to investigate the relationship of MRPL15 and differentially expressed genes in the LUAD cohort (n = 389, selected patients) and LUSC cohort (n = 319, selected patients) from TCGA, respectively. Moreover, the LinkInterpreter module was used to reveal potential regulatory mechanisms through pathway and network analysis. Gene set enrichment analysis (GSEA) tool was used to conduct KEGG pathways, kinase-target enrichment, miRNA-target enrichment and transcription factor-target enrichment. The rank standard was FDR <0.05 and 500 simulations.
GeneMANIA (http://www.genemania.org) is a website for generating hypotheses about gene function, analyzing gene lists and prioritizing genes for functional assays (30), such as information for protein and genetic interactions, enrichment analysis and so on. GeneMANIA was used to construct gene-gene functional interaction networks among the genes that GSEA identified as being enriched in LUAD and LUSC: kinase HCK and transcription factor ELK1.
The immune scores and stromal scores of each case were analyzed by Estimation of STromal and Immune cells in MAlignant Tumor tissues using Expression data (ESTIMATE) algorithm. Further, the different gene expression levels of human leukocyte antigen (HLA) family genes between the high-expression group and the low-expression group of MRPL15 were analyzed based on TCGA.
TISIDB (http://cis.hku.hk/TISIDB) is a web portal for investigation of tumor-immune interactions, which contains multiple types of data resources in oncoimmunology (31). TISIDB was employed to investigate correlations between MRPL15 expression and lymphocytes.
The tissue microarray containing 92 lung cancer tissues and 88 adjacent normal tissues was purchased from Shanghai Outdo Biotech (Shanghai, China). IHC staining was performed as previously described (32) with mouse monoclonal MRPL15 antibody (OriGene Technologies Inc., TA807480, 1:300 dilution). The staining results were identified by integrated scoring: the immunostaining intensity (negative, no staining of cells = 0; weak staining = 1; moderate staining = 2; strong staining = 3) and the percentage of the positively stained area (0–25% = 1, 26–50% = 2, 51–75% = 3, >75% = 4). The score was finally calculated according to the above staining criteria, and the scores over 6 were regarded as high expression.
Statistical analysis was carried out by SPSS 23.0 (IBM, Armonk, NY, USA) and GraphPad Prism 8.0 (La Jolla, CA, USA) software. The t-test was used for differential MRPL15 expression analysis and log-rank test was used to perform group comparison of survival correlation. Furthermore, the relationship between MRPL15 expression and clinicopathologic features was analyzed by Chi-square and independent prognostic factors of lung cancer patients were confirmed by the Cox regression analyses. The differences were considered significant when the probability value was less than 0.05.
We initially examined the level of MRPL15 expression in multiple tumor tissues in GEPIA. The analysis revealed that MRPL15 was up-regulated in pan-cancer (Figure 1A). Then, we further evaluated MRPL15 expression in multiple NSCLC cohorts from GEPIA, Oncomine and GEO databases. The expression of MRPL15 in LUAD or LUSC was obviously higher than in non-tumor tissues (Figure 1B). Meanwhile, MRPL15 ranked within the top 5% up-regulated genes in large cell lung carcinoma based on mRNA expression in Oncomine database (Figure 1C). That MRPL15 was also significantly overexpressed in GSE8569, GSE101929, GSE33532, GSE27262, GSE21933, GSE19804, GSE19188 and GSE18842 datasets (Figure 1D). The information of eight GEO series was shown in Table 1. The results demonstrated that MRPL15 was significantly elevated in NSCLC tissues.
Figure 1 Up-regulated expression of MRPL15 in NSCLC. (A) The expression levels of MRPL15 in pan-cancer. Data was obtained from the GEPIA. (B) The expression of MRPL15 in LUAD and LUSC in GEPIA. (C) The mRNA levels of MRPL15 in the Hou Lung dataset. (D) MRPL15 expression levels between tumor tissues and adjacent normal tissues in patients with NSCLC in eight GEO series (***P < 0.001).
Multiple clinic-pathological characters of LUAD and LUSC samples in the TCGA were further analyzed. The results of subgroup analyses claimed that MRPL15 was dramatically higher in LUAD or LUSC patients than in healthy people within different gender, clinical stage, lymph node status and the TP53 mutation status (Figures 2A–H). Furthermore, the up-regulated MRPL15 was notably correlated with poor OS (HR = 1.35, log rank P = 3.30E−06, Figure 2I), PFS (HR = 1.23, log rank P = 0.034, Figure 2J) and DFS (HR = 1.3, log rank P = 0.025, Supplementary Figure 1) in NSCLC patients. Interestingly, high MRPL15 expression was connected with worse 5-year OS in NSCLC patients with smoking history (Figure 2K), rather than in NSCLC without smoking history (Figure 2L). Additionally, data in NSCLC cohort (GSE8894, Figure 2M) and LUAD cohort (GSE31210, Figure 2N) ld> revealed that high MRPL15 expression was also associated with poor RFS, indicating an aggressive role for MRPL15. That high MRPL15 was positively linked with OS was also supported by two LUAD cohort (GSE13213, GSE31210, Figures 2O, P).
Figure 2 The sub-group analysis of multiple clinic-pathological features (UALCAN) and the prognostic value of MRPL15 in NSCLC. (A–D) Relative expression of MRPL15 in healthy and LUAD people with different (A) gender, (B) clinical stage, (C) lymph node status and (D) TP53 mutation status; (E–H) Relative expression of MRPL15 in healthy and LUSC people with different (E) gender, (F) clinical stage, (G) lymph node status and (H) TP53 mutation status. (I, J) Overall survival (OS) and progression-free survival (PFS) of MRPL15 in lung cancer cohort. (K, L) Subgroup analyses of 5-year OS comparison in (K) the group with smoking history and (L) the other group without smoking history of lung cancer patients. (M, N) Relapse-free survival (RFS) in the NSCLC cohort (GSE8894, n = 138, M) and LUAD cohort (GSE31210, n = 204, N). (O, P) OS in two LUAD cohorts [GSE13213 (n = 117, O) and GSE31210 (n = 204, P)].
To explore the biological function of MRPL15 in NSCLC, the LinkedOmics was applied to investigate co-expression genes associated with MRPL15 in LUAD (N = 389 patients) and LUSC cohort (N = 319 patients). In LUAD (Figure 3A), 3,987 genes were positively correlated with MRPL15, whereas 6,681 genes were negatively correlated (false discovery rate, FDR <0.01). And in LUSC (Figure 3D), 2,859 genes were positively correlated with MRPL15, whereas 4,286 genes were negatively correlated. The top 50 remarkable genes related to MRPL15 in LAUD (Figures 3B, C) and LUAD (Figures 3E, F) were displayed in the heat map, suggesting an extensive influence of MRPL15 on global transcriptome. Lysophospholipase1 (LYPLA1) was the most positively significant gene connected with MRPL15 expression in NSCLC (rank #2 in LUAD, R = 0.776, P = 2.44E−78; rank #1 in LUSC, R = 0.785, P = 3.12E−67). LYPLA1 was known as acyl−protein thioesterase1 (APT1), and its suppression inhibited proliferation and invasion in NSCLC cells (33).
Figure 3 Differentially expressed genes associated with MRPL15 in NSCLC (LinkedOmics). (A) Using Pearson test to identify significantly expressed genes related to MRPL15 in LUAD. (B, C) TOP 50 remarkable genes associated with MRPL15 in LUAD. (D) Using Pearson test to identify significantly expressed genes related to MRPL15 in LUSC. (E, F) TOP 50 remarkable genes associated with MRPL15 in LUSC. Red represents positively correlated genes and green represents negatively correlated genes.
In LUAD and LUSC, KEGG pathway analysis showed that MRPL15 co-expressed genes participate in metabolism function (like oxidative phosphorylation, carbon metabolism and pyrimidine metabolism) and DNA replication (Figures 4A, B). Higher expression of pyrimidine synthesis genes was shown to result in poor prognosis of patients with glioblastoma (34) and NSCLC (35, 36). As MRPL15 is important for mitochondrial metabolism, we have reason to suppose that MRPL15 is likely associated with pyrimidine metabolism. Moreover, some immune-related activities like Th1 and Th2 cell differentiation and Th17 cell differentiation were inhibited (Figures 4A, B). The above results indicate that MRPL15 may play a positive role in metabolism function, while a negative role in immune infiltration in NSCLC.
Figure 4 KEGG pathways networks of MRPL15 in NSCLC. (A) KEGG enrichments of MRPL15 in LUAD cohort. (B) KEGG enrichments of MRPL15 in LUSC cohort.
Next, we explored the regulators enrichment of MRPL15 co-expressed genes in NSCLC, including kinases, miRNAs and transcription factors’ (TF) enrichment. In LUAD, the kinases-target network was associated with hematopoietic cell kinase (HCK), polo like kinase 1 (PLK1), Aurora kinase B (AURKB), polo like kinase 3 (PLK3), and cyclin-dependent kinase 1 (CDK1) (Table 2 and Supplementary Table 1). In LUSC, the kinases-target network was mainly related to hematopoietic cell kinase (HCK) , mitogen-activated protein kinase 7 (MAPK7), protein kinase AMP-activated catalytic subunit alpha 2 (PRKAA2), mitogen-activated protein kinase 10 (MAPK10), mitogen-activated protein kinase 3 (MAPK3) (Table 2 and Supplementary Table 2). The miRNAs enriched in LUAD and LUSC were shown in Table 2 and Supplementary Tables 3, 4, while transcription factor enriched in LUAD and LUSC were listed in Table 2 and Supplementary Tables 5, 6.
Table 2 The Kinase, miRNA and transcription factor-target networks of MRPL15 in NSCLC (LinkedOmics).
We observed that HCK and ETS-like 1 transcription factor (ELK1) were in the top five regulators enriched in both LUAD and LUSC. Using protein- protein interaction (PPI) network, we revealed that kinase HCK regulating Fc receptor signaling pathway, immune response-regulating cell surface receptor signaling pathway and phosphatidylinositol 3-kinase complex were enriched in LUAD (Figure 5) or LUSC (Supplementary Figure 2). Inhibition of HCK has been reported to suppress macrophage polarization and impairs gastric tumor growth (37). Meanwhile, HCK knockdown can enhance the antitumor effects of immunotoxin (38). Taken together, the results indicate that MRPL15 may affect the process of immune through HCK signaling pathway. Additionally, the gene set enriched for transcription factor ELK1 (TF V$ELK1_02) included regulation of cellular amino acid metabolism, DNA damage response, signal transduction by p53 class mediator resulting in cell cycle arrest and signal transduction involved in mitotic DNA integrity checkpoint in LUAD (Figure 6) or LUSC (Supplementary Figure 3). ELK1 could promote epithelial to mesenchymal transition (EMT) in osteosarcoma tumor cell (39) and NSCLC (40). Moreover, MER/ERK/ELK1 pathway has been shown to promote amino acid metabolism (41, 42) and inactivation of ELK1 results in elevated apoptosis in response to viral infection (43). These findings confirm that HCK and ELK1 may participate in the metabolism function involved in the process that MRPL15 promotes tumorigenesis in NSCLC.
Figure 5 Protein–protein interaction network of kinases HCK-target networks in LUAD (GeneMANIA). PPI network and functional analysis indicating the gene set that was enriched in the target network of HCK. The network nodes’ colors represent the biological functions of the enrichment genes.
Figure 6 PPI network of transcription factor ELK1-target networks in LUAD (GeneMANIA). PPI network and functional analysis indicating the gene set that was enriched in the target network of ELK1-target. The network nodes’ colors represent the biological functions of the enrichment genes.
In lung cancer, immunological parameters were reported to better predict the clinical outcome than TNM stage (44). To investigate whether MRPL15 expression was correlated with immune infiltration patterns in NSCLC, we compared the degree of immune cell infiltration between the high-expression group and the low-expression group by ESTIMATE algorithm. The immune score and stromal score were lower in the high-expression group than the low-expression group in LUAD (Figures 7A, B) and LUSC (Figures 7C, D). Besides, the expression of MRPL15 was significantly negatively correlated with the immune score and stromal score in LUAD (Figures 7E, F) and LUSC (Figures 7G, H). Our results showed the correlation of MRPL15 expression level with poorer prognosis and low immune infiltration in NSCLC. Due to human leukocyte antigen (HLA) holding a predictive role in developing immune-related adverse events (irAEs) during therapy in NSCLC (45), we also investigated significantly lower expression level of the human leukocyte antigen (HLA) family genes in high-expression MRPL15 group in LUAD and LUSD (Supplementary Figures 4A, B). Moreover, Spearman’s correlations between MRPL15 expression and lymphocytes were analyzed using the TISIDB database. The top four TILs negatively associated with MRPL15 expression were eosinophils, natural killer cells, mast cells and immature B cells in LUAD (Figures 7I–L), while eosinophils, natural killer cells, activated B cell and immature B cells in LUSC (Figures 7M–P). Our findings suggest that MRPL15 may play a significant role in immune infiltration in NSCLC.
Figure 7 Association of MRPL15 expression with immune cell infiltration levels. (A, B) The high-expression group showed lower (A) immune score and (B) stromal score than the low-expression group in LUAD. (C, D) The high-expression group showed lower (C) immune score and (D) stromal score than the low-expression group in LUSC *P < 0.05, **P < 0.01, ***P < 0.001. (E, G) Association between immune score and the expression of MRPL15 in (E) LUAD and (G) LUSC. (F, H) Association between stromal score and the expression of MRPL15 in (F) LUAD and (H) LUSC. (I–L) Top four TILs displaying the greatest Spearman’s correlation with MRPL15 expression in LUAD. (M–P) Top four TILs displaying the greatest Spearman’s correlation with MRPL15 expression in LUSC.
In this study, 91 lung cancer patients with complete clinical information were included in the analysis and the clinical characteristics were shown in Table 3. Our results indicated that MRPL15 expression is strongly related to lymph node metastasis (P = 0.028). As shown in Figures 8A, B, the specific staining was observed mainly in the cytoplasm and the expression scores of MRPL15 were significantly higher in tumor tissues than in adjacent non-tumorous tissues. The lung cancer patients with high MRPL15 expression had a poor OS than those with low MRPL15 expression (Figure 8C). Furthermore, multivariate analysis revealed that MRPL15 expression level [hazard ratio (HR): 1.996; 95% CI: 1.121–3.554; P = 0.019] remained potential independent prognostic factors (Table 4). Taken together, MRPL15 can serve as an independent risk factor for survival and prognosis of lung cancer patients.
Table 3 Correlation between MRPL15 expression and clinicopathological characteristics in lung cancer patients.
Figure 8 Expression levels of MRPL15 in microarrays. (A) Representative pictures of MRPL15 IHC in lung cancer tissues and matched adjacent normal tissues. (B) Statistical analysis of IHC staining in lung cancer tissues and matched adjacent normal tissues. (C) Kaplan–Meier curves displaying OS for MRPL15 protein expression in the samples included in the analysis.
Here, we first declared that MRPL15 was up-regulated in tumor tissues in patients with NSCLC via multiple cohorts including GEPIA, ONCOMINE and eight GEO series (GSE8569, GSE101929, GSE33532, GSE27262, GSE21933, GSE19804, GSE19188, GSE18842). Moreover, the impact of abnormal MRPL15 expression on different clinical pathological characters was investigated in UALCAN. Kaplan–Meier analysis also demonstrated that high MRPL15 expression was associated with poor OS, PFS, DFS and RFS in NSCLC. Furthermore, using GeneMANIA, functional networks linked to MRPL15 were identified. Additionally, we found that MRPL15 expression was negatively associated with immune scores, stromal scores and several TILs, such as eosinophils and natural killer cells. IHC results further validated the high MRPL15 expression and its prognostic potential in lung cancer. Our results identify a possible biomarker for NSCLC and provide functional mechanisms to be further explored.
MRPL15 is a member of mitochondrial ribosomal proteins (MRPs) and it is connected to OXPHOS. MRPL15 has not been widely studied, but various MRPs play a prognosis and diagnosis role in multiple cancers. Here, we are the first to report that high MRPL15 expression indicates poor prognosis in NSCLC and reveal potential regulatory networks as well as the negative relationship with immune infiltration.
In our work, higher expression of MRPL15 was observed in pan-cancers tissues than adjacent normal tissues using online database, including LUAD and LUSC. Moreover, in NSCLC, MRPL15 expression was associated with different clinical pathological characters including gender, clinical stage, lymph node status and the TP53 mutation status. Kaplan–Meier Plotter also demonstrated a positive relationship between MRPL15 expression and poor clinical outcomes in NSCLC. IHC results further validated the findings: Firstly, MRPL15 expression is related to lymph node metastasis. Secondly, MRPL15 is overexpressed in lung cancer patients and predicts poor OS. Federica Sotgia et al. presented evidence revealing that MRPL15 has predictive value in breast cancer distant metastasis (19). Jandee Lee et al. illustrated that expression of MRPL44 (components of MRPs) was significantly related to lymph node metastasis and influenced OXPHOS in papillary thyroid cancers (PTCs) (46). Furthermore, reduced mitochondrial respiratory chain activity caused by mtDNA decreased promote metastasis and increased glycolysis in lung cancer cells (47, 48). Therefore, we suppose that MRPL15 might impact OXPHOS function and induce poor prognosis.
To explore the biological function of MRPL15 in NSCLC, we first identified co-expression genes associated with MRPL15 in LUAD and LUSD cohorts. Next, the KEGG pathway analysis was performed. The results showed that MRPL15 co-expressed genes were focused on OXPHOS and pyrimidine metabolism and DNA replication. Enrichment analysis of target gene sets through PPI network identify the significant networks of target kinases HCK and transcription factor ELK1 in LUCD and LUSD. These kinases regulate Fc receptor signaling pathway, immune response-regulating cell surface receptor and PI3K signaling pathway. Actually, HCK overexpression has also been observed in NSCLC (49) and HCK activation has been reported to force the recruitment of immune cells into tumors in many cancers (50, 51), which was consistent with the phenotype of up-regulated MRPL15 in our present study.
The network of transcription factors including ELK1 have been linked to amino acid metabolism, DNA damage response, signal transduction by p53 class mediator resulting in cell cycle arrest and signal transduction involved in mitotic DNA integrity checkpoint. In fact, ELK1 could improve LUAD progression (52). ELK1 was identified as a gene with a likely role in OXPHOS biogenesis similar to MRPL15 (53). Nevertheless, more researches are required to understand the function of MRPL15 in NSCLC. Based on our results and previous researches, we provide promising insights into the molecular mechanisms, which will be useful for clinical applications.
Another significant conclusion of our findings is that MRPL15 showed a negative correlation with immune infiltration in NSCLC (especially in LAUD). The high MRPL15 expression group displayed lower immune score and stromal score than the low MRPL15 expression group. Additionally, the results of HLA family genes are consistent with immune score and stromal score. TILs were associated with a positive clinical outcome in several cancers, including lung cancer (54, 55). MRPL15 also showed a negative correlation with immune cells, such as B cell, NK cell and eosinophils in NSCLC. Thus, this data provides evidence for the impact of MRPL15 on immune infiltration in NSCLC.
In conclusion, our study has identified MRPL15 as a novel predictor that contributes to poor prognosis for NSCLC. Furthermore, several kinases and transcription factors related to MRPL15 were recognized, indicating MRPL15 will be a therapeutic target. Overall, MRPL15 may be an attractive prognostic predictor for NSCLC and worthy to be deeply explored in NSCLC.
The original contributions presented in the study are included in the article/Supplementary Material. Further inquiries can be directed to the corresponding authors.
The studies involving human participants were reviewed and approved by The Ethics Committee of Shanghai Outdo Biotech Co., Ltd. Written informed consent for participation was not required for this study in accordance with the national legislation and the institutional requirements.
YanZ, YS, and LX participated in data analysis and wrote the manuscript, and they contributed equally to this work. YulZ and XC participated in verification and visualization of the manuscript. YW and NY contributed to carrying out additional analyses. YunZ and FZ conceived the idea and provided guidance. All authors contributed to the article and approved the submitted version.
This research was funded by the National Natural Science Foundation of China (81472799).
The authors declare that the research was conducted in the absence of any commercial or financial relationships that could be construed as a potential conflict of interest.
The Supplementary Material for this article can be found online at: https://www.frontiersin.org/articles/10.3389/fonc.2021.656172/full#supplementary-material
Supplementary Figure 1 | Disease-free survival (DFS) of MRPL15 in lung cancer cohort from GEPIA.
Supplementary Figure 2 | PPI network of kinases HCK-target networks in LUSC (GeneMANIA). PPI network and functional analysis indicating the gene set that was enriched in the target network of HCK. The network nodes’ colors represent the biological functions of the enrichment genes.
Supplementary Figure 3 | PPI network of transcription factor ELK1-target networks in LUSC (GeneMANIA). PPI network and functional analysis indicating the gene set that was enriched in the target network of ELK1-target. The network nodes’ colors represent the biological functions of the enrichment genes.
Supplementary Figure 4 | The expression of HLA family genes in high and low expression MRPL15 groups in NSCLC: (A) LUAD and (B) LUSC (P < 0.05).
1. Bade BC, Dela Cruz CS. Lung Cancer 2020: Epidemiology, Etiology, and Prevention. Clin Chest Med (2020) 41:1–24. doi: 10.1016/j.ccm.2019.10.001
2. Altan M, Chiang AC. Management of Small Cell Lung Cancer: Progress and Updates. Cancer J (2015) 21:425–33. doi: 10.1097/PPO.0000000000000148
3. Mitchell PL, John T. Lung Cancer in 2016: Immunotherapy Comes of Age. Lancet Respir Med (2016) 4:947–49. doi: 10.1016/S2213-2600(16)30379-4
4. Kazdal D, Harms A, Endris V, Penzel R, Kriegsmann M, Eichhorn F, et al. Prevalence of Somatic Mitochondrial Mutations and Spatial Distribution of Mitochondria in non-Small Cell Lung Cancer. Br J Cancer (2017) 117:220–26. doi: 10.1038/bjc.2017.155
5. Liu F, Sanin DE, Wang X. Mitochondrial DNA in Lung Cancer. Adv Exp Med Biol (2017) 1038:9–22. doi: 10.1007/978-981-10-6674-0_2
6. Zong WX, Rabinowitz JD, White E. Mitochondria and Cancer. Mol Cell (2016) 61:667–76. doi: 10.1016/j.molcel.2016.02.011
7. Porporato PE, Filigheddu N, Pedro JMB, Kroemer G, Galluzzi L. Mitochondrial Metabolism and Cancer. Cell Res (2018) 28:265–80. doi: 10.1038/cr.2017.155
8. Sabharwal SS, Schumacker PT. Mitochondrial ROS in Cancer: Initiators, Amplifiers or an Achilles’ Heel? Nat Rev Cancer (2014) 14:709–21. doi: 10.1038/nrc3803
9. Gaude E, Frezza C. Defects in Mitochondrial Metabolism and Cancer. Cancer Metab (2014) 2:10. doi: 10.1186/2049-3002-2-10
10. Lopez J, Tait SW. Mitochondrial Apoptosis: Killing Cancer Using the Enemy Within. Br J Cancer (2015) 112:957–62. doi: 10.1038/bjc.2015.85
11. Kim H-J, Maiti P, Barrientos A. Mitochondrial Ribosomes in Cancer. Semin Cancer Biol (2017) 47:67–81. doi: 10.1016/j.semcancer.2017.04.004
12. De Silva D, Tu YT, Amunts A, Fontanesi F, Barrientos A. Mitochondrial Ribosome Assembly in Health and Disease. Cell Cycle (2015) 14:2226–50. doi: 10.1080/15384101.2015.1053672
13. Emdadul Haque M, Grasso D, Miller C, Spremulli LL, Saada A. The Effect of Mutated Mitochondrial Ribosomal Proteins S16 and S22 on the Assembly of the Small and Large Ribosomal Subunits in Human Mitochondria. Mitochondrion (2008) 8:254–61. doi: 10.1016/j.mito.2008.04.004
14. Cheong A, Lingutla R, Mager J. Expression Analysis of Mammalian Mitochondrial Ribosomal Protein Genes. Gene Expr Patterns (2020) . 38:119147. doi: 10.1016/j.gep.2020.119147
15. Xu YH, Deng JL, Wang LP, Zhang HB, Tang L, Huang Y, et al. Identification of Candidate Genes Associated With Breast Cancer Prognosis. DNA Cell Biol (2020) 39:1205–27. doi: 10.1089/dna.2020.5482
16. Lee YK, Lim JJ, Jeoun UW, Min S, Lee EB, Kwon SM, et al. Lactate-Mediated Mitoribosomal Defects Impair Mitochondrial Oxidative Phosphorylation and Promote Hepatoma Cell Invasiveness. J Biol Chem (2017) 292:20208–17. doi: 10.1074/jbc.M117.809012
17. Huang G, Li H, Zhang H. Abnormal Expression of Mitochondrial Ribosomal Proteins and Their Encoding Genes With Cell Apoptosis and Diseases. Int J Mol Sci (2020) 21:8879. doi: 10.3390/ijms21228879
18. Cohen O, Inbal B, Kissil JL, Raveh T, Berissi H, Spivak-Kroizaman T, et al. DAP-Kinase Participates in TNF-Alpha- and Fas-Induced Apoptosis and its Function Requires the Death Domain. J Cell Biol (1999) 146:141–8. doi: 10.1083/jcb.146.1.141
19. Sotgia F, Fiorillo M, Lisanti MP. Mitochondrial Markers Predict Recurrence, Metastasis and Tamoxifen-Resistance in Breast Cancer Patients: Early Detection of Treatment Failure With Companion Diagnostics. Oncotarget (2017) 8:68730–45. doi: 10.18632/oncotarget.19612
20. Niu F, Kazimierska M, Nolte IM, Terpstra MM, de Jong D, Koerts J, et al. The Mir-26b-5p/KPNA2 Axis is an Important Regulator of Burkitt Lymphoma Cell Growth. Cancers (Basel) (2020) 12:1464. doi: 10.3390/cancers12061464
21. Deng F, Shen L, Wang H, Zhang L. Classify Multicategory Outcome in Patients With Lung Adenocarcinoma Using Clinical, Transcriptomic and Clinico-Transcriptomic Data: Machine Learning Versus Multinomial Models. Am J Cancer Res (2020) 10:4624–39. doi: 10.13140/RG.2.2.15071.48802/1
22. Stankovic B, Bjorhovde HAK, Skarshaug R, Aamodt H, Frafjord A, Muller E, et al. Immune Cell Composition in Human non-Small Cell Lung Cancer. Front Immunol (2018) 9:3101. doi: 10.3389/fimmu.2018.03101
23. Tang Z, Li C, Kang B, Gao G, Li C, Zhang Z. GEPIA: A Web Server for Cancer and Normal Gene Expression Profiling and Interactive Analyses. Nucleic Acids Res (2017) 45:W98–W102. doi: 10.1093/nar/gkx247
24. Rhodes DR, Kalyana-Sundaram S, Mahavisno V, Varambally R, Yu J, Briggs BB, et al. Oncomine 3.0: Genes, Pathways, and Networks in a Collection of 18,000 Cancer Gene Expression Profiles. Neoplasia (2007) 9:166–80. doi: 10.1593/neo.07112
25. Hou J, Aerts J, den Hamer B, van Ijcken W, den Bakker M, Riegman P, et al. Gene Expression-Based Classification of non-Small Cell Lung Carcinomas and Survival Prediction. PloS One (2010) 5:e10312. doi: 10.1371/journal.pone.0010312
26. Chandrashekar DS, Bashel B, Balasubramanya SAH, Creighton CJ, Ponce-Rodriguez I, Chakravarthi B, et al. UALCAN: A Portal for Facilitating Tumor Subgroup Gene Expression and Survival Analyses. Neoplasia (2017) 19:649–58. doi: 10.1016/j.neo.2017.05.002
27. Gyorffy B, Surowiak P, Budczies J, Lanczky A. Online Survival Analysis Software to Assess the Prognostic Value of Biomarkers Using Transcriptomic Data in non-Small-Cell Lung Cancer. PloS One (2013) 8:e82241. doi: 10.1371/journal.pone.0082241
28. Mizuno H, Kitada K, Nakai K, Sarai A. Prognoscan: A New Database for Meta-Analysis of the Prognostic Value of Genes. BMC Med Genomics (2009) 2:18. doi: 10.1186/1755-8794-2-18
29. Vasaikar SV, Straub P, Wang J, Zhang B. Linkedomics: Analyzing Multi-Omics Data Within and Across 32 Cancer Types. Nucleic Acids Res (2018) 46:D956–D63. doi: 10.1093/nar/gkx1090
30. Warde-Farley D, Donaldson SL, Comes O, Zuberi K, Badrawi R, Chao P, et al. The Genemania Prediction Server: Biological Network Integration for Gene Prioritization and Predicting Gene Function. Nucleic Acids Res (2010) 38:W214–20. doi: 10.1093/nar/gkq537
31. Ru B, Wong CN, Tong Y, Zhong JY, Zhong SSW, Wu WC, et al. TISIDB: An Integrated Repository Portal for Tumor-Immune System Interactions. Bioinformatics (2019) . 35:4200–02. doi: 10.1093/bioinformatics/btz210
32. Zeng Y, Jie X, Wu B, Wu G, Liu L, Xu S. IQGAP3 Interacts With Rad17 to Recruit the Mre11-Rad50-Nbs1 Complex and Contributes to Radioresistance in Lung Cancer. Cancer Lett (2020) 493:254–65. doi: 10.1016/j.canlet.2020.08.042
33. Mohammed A, Zhang C, Zhang S, Shen Q, Li J, Tang Z, et al. Inhibition of Cell Proliferation and Migration in Nonsmall Cell Lung Cancer Cells Through the Suppression of LYPLA1. Oncol Rep (2019) 41:973–80. doi: 10.3892/or.2018.6857
34. Wang X, Yang K, Wu Q, Kim LJY, Morton AR, Gimple RC, et al. Targeting Pyrimidine Synthesis Accentuates Molecular Therapy Response in Glioblastoma Stem Cells. Sci Transl Med (2019) 11:eaau4972. doi: 10.1126/scitranslmed.aau4972
35. Lu L, Li H, Wu X, Rao J, Zhou J, Fan S, et al. HJC0152 Suppresses Human non-Small-Cell Lung Cancer by Inhibiting STAT3 and Modulating Metabolism. Cell Prolif (2020) 53:e12777. doi: 10.1111/cpr.12777
36. Kim J, Hu Z, Cai L, Li K, Choi E, Faubert B, et al. CPS1 Maintains Pyrimidine Pools and DNA Synthesis in KRAS/LKB1-Mutant Lung Cancer Cells. Nature (2017) 546:168–72. doi: 10.1038/nature22359
37. Poh AR, Dwyer AR, Eissmann MF, Chand AL, Baloyan D, Boon L, et al. Inhibition of the SRC Kinase HCK Impairs STAT3-Dependent Gastric Tumor Growth in Mice. Cancer Immunol Res (2020) 8:428–35. doi: 10.1158/2326-6066.CIR-19-0623
38. Liu XF, Xiang L, FitzGerald DJ, Pastan I. Antitumor Effects of Immunotoxins are Enhanced by Lowering HCK or Treatment With SRC Kinase Inhibitors. Mol Cancer Ther (2014) . 13:82–9. doi: 10.1158/1535-7163.MCT-13-0726
39. Hou CH, Lin FL, Hou SM, Liu JF. Cyr61 Promotes Epithelial-Mesenchymal Transition and Tumor Metastasis of Osteosarcoma by Raf-1/MEK/ERK/Elk-1/TWIST-1 Signaling Pathway. Mol Cancer (2014) 13:236. doi: 10.1186/1476-4598-13-236
40. Wu DM, Deng SH, Zhou J, Han R, Liu T, Zhang T, et al. PLEK2 Mediates Metastasis and Vascular Invasion Via the Ubiquitin-Dependent Degradation of SHIP2 in non-Small Cell Lung Cancer. Int J Cancer (2020) 146:2563–75. doi: 10.1002/ijc.32675
41. Yang R, Li X, Wu Y, Zhang G, Liu X, Li Y, et al. EGFR Activates GDH1 Transcription to Promote Glutamine Metabolism Through MEK/ERK/ELK1 Pathway in Glioblastoma. Oncogene (2020) 39:2975–86. doi: 10.1038/s41388-020-1199-2
42. Shan J, Balasubramanian MN, Donelan W, Fu L, Hayner J, Lopez MC, et al. A Mitogen-Activated Protein Kinase/Extracellular Signal-Regulated Kinase Kinase (MEK)-Dependent Transcriptional Program Controls Activation of the Early Growth Response 1 (EGR1) Gene During Amino Acid Limitation. J Biol Chem (2014) . 289:24665–79. doi: 10.1074/jbc.M114.565028
43. Kew V, Wills M, Reeves M. HCMV Activation of ERK-MAPK Drives a Multi-Factorial Response Promoting the Survival of Infected Myeloid Progenitors. J Mol Biochem (2017) 6:13–25. doi: 10.17863/CAM.11285
44. Welsh TJ, Green RH, Richardson D, Waller DA, O’Byrne KJ, Bradding P. Macrophage and Mast-Cell Invasion of Tumor Cell Islets Confers a Marked Survival Advantage in non-Small-Cell Lung Cancer. J Clin Oncol (2005) 23:8959–67. doi: 10.1200/JCO.2005.01.4910
45. Hasan Ali O, Berner F, Bomze D, Fassler M, Diem S, Cozzio A, et al. Human Leukocyte Antigen Variation is Associated With Adverse Events of Checkpoint Inhibitors. Eur J Cancer (2019) 107:8–14. doi: 10.1016/j.ejca.2018.11.009
46. Lee J, Seol MY, Jeong S, Lee CR, Ku CR, Kang SW, et al. A Metabolic Phenotype Based on Mitochondrial Ribosomal Protein Expression as a Predictor of Lymph Node Metastasis in Papillary Thyroid Carcinoma. Med (Baltimore) (2015) 94:e380. doi: 10.1097/MD.0000000000000380
47. He K, Guo X, Liu Y, Li J, Hu Y, Wang D, et al. TUFM Downregulation Induces Epithelial-Mesenchymal Transition and Invasion in Lung Cancer Cells Via a Mechanism Involving AMPK-GSK3beta Signaling. Cell Mol Life Sci (2016) 73:2105–21. doi: 10.1007/s00018-015-2122-9
48. Zhang X, Han S, Zhou H, Cai L, Li J, Liu N, et al. TIMM50 Promotes Tumor Progression Via ERK Signaling and Predicts Poor Prognosis of non-Small Cell Lung Cancer Patients. Mol Carcinog (2019) 58:767–76. doi: 10.1002/mc.22969
49. Ding L, Getz G, Wheeler DA, Mardis ER, McLellan MD, Cibulskis K, et al. Somatic Mutations Affect Key Pathways in Lung Adenocarcinoma. Nature (2008) 455:1069–75. doi: 10.1038/nature07423
50. Poh AR, O’Donoghue RJ, Ernst M. Hematopoietic Cell Kinase (HCK) as a Therapeutic Target in Immune and Cancer Cells. Oncotarget (2015) 6:15752–71. doi: 10.18632/oncotarget.4199
51. Cougoule C, Le Cabec V, Poincloux R, Al Saati T, Mege JL, Tabouret G, et al. Three-Dimensional Migration of Macrophages Requires Hck for Podosome Organization and Extracellular Matrix Proteolysis. Blood (2010) 115:1444–52. doi: 10.1182/blood-2009-04-218735
52. Sheng K, Lu J, Zhao H. ELK1-Induced Upregulation of Lncrna HOXA10-as Promotes Lung Adenocarcinoma Progression by Increasing Wnt/Beta-Catenin Signaling. Biochem Biophys Res Commun (2018) 501:612–18. doi: 10.1016/j.bbrc.2018.04.224
53. van der Lee R, Szklarczyk R, Smeitink J, Smeets HJ, Huynen MA, Vogel R. Transcriptome Analysis of Complex I-Deficient Patients Reveals Distinct Expression Programs for Subunits and Assembly Factors of the Oxidative Phosphorylation System. BMC Genomics (2015) 16:691. doi: 10.1186/s12864-015-1883-8
54. Fridman WH, Pages F, Sautes-Fridman C, Galon J. The Immune Contexture in Human Tumours: Impact on Clinical Outcome. Nat Rev Cancer (2012) 12:298–306. doi: 10.1038/nrc3245
Keywords: MRPL15, non-small-cell lung cancer, prognostic value, functional network analysis, immune infiltration
Citation: Zeng Y, Shi Y, Xu L, Zeng Y, Cui X, Wang Y, Yang N, Zhou F and Zhou Y (2021) Prognostic Value and Related Regulatory Networks of MRPL15 in Non-Small-Cell Lung Cancer. Front. Oncol. 11:656172. doi: 10.3389/fonc.2021.656172
Received: 20 January 2021; Accepted: 09 April 2021;
Published: 07 May 2021.
Edited by:
Zhengfei Zhu, Fudan University, ChinaReviewed by:
Ignacio Matos, University College London, United KingdomCopyright © 2021 Zeng, Shi, Xu, Zeng, Cui, Wang, Yang, Zhou and Zhou. This is an open-access article distributed under the terms of the Creative Commons Attribution License (CC BY). The use, distribution or reproduction in other forums is permitted, provided the original author(s) and the copyright owner(s) are credited and that the original publication in this journal is cited, in accordance with accepted academic practice. No use, distribution or reproduction is permitted which does not comply with these terms.
*Correspondence: Yunfeng Zhou, eWZ6aG91d2h1QDE2My5jb20=; Fuxiang Zhou, aGFwcHl6aG91ZnhAc2luYS5jb20=
†These authors have contributed equally to this work and share first authorship
Disclaimer: All claims expressed in this article are solely those of the authors and do not necessarily represent those of their affiliated organizations, or those of the publisher, the editors and the reviewers. Any product that may be evaluated in this article or claim that may be made by its manufacturer is not guaranteed or endorsed by the publisher.
Research integrity at Frontiers
Learn more about the work of our research integrity team to safeguard the quality of each article we publish.