- 1Department of Obstetrics and Gynecology, Shengjing Hospital of China Medical University, Shenyang, China
- 2Department of Health Management, Shengjing Hospital of China Medical University, Shenyang, China
Background: Ovarian cancer is a common gynecological malignancy, most of which is epithelial ovarian cancer (EOC). Advanced EOC is linked with a higher incidence of premature death. To date, no effective prognostic tools are available to evaluate the possibility of early death in patients with advanced EOC.
Methods: Advanced (FIGO stage III and IV) EOC patients who were enrolled in the Surveillance, Epidemiology, and End Results database between 2004 and 2015 were regarded as subjects and studied. We aimed to construct a nomogram that can deliver early death prognosis in patients with advanced EOC by identifying crucial independent factors using univariate and multivariate logistic regression analyses to help deliver accurate prognoses.
Results: In total, 13,403 patients with advanced EOC were included in this study. Three hundred ninety-seven out of a total of 9,379 FIGO stage III patients died early. There were 4,024 patients with FIGO stage IV, 414 of whom died early. Nomograms based on independent prognostic factors have the satisfactory predictive capability and clinical pragmatism. The internal validation feature of the nomogram demonstrated a high level of accuracy of the predicted death.
Conclusions: By analyzing data from a large cohort, a clinically convenient nomogram was established to predict premature death in advanced EOC. This tool can aid clinicians in screening patients who are at higher risk for tailoring treatment plans.
Introduction
Ovarian tumor is the second most common malignancy in females, with a 5-year survival rate of only 48.6% (1, 2). Epithelial ovarian cancer (EOC) is the most prevalent type of ovarian tumor, which accounts for ~90% of all tumors (3). The four most common subtypes of EOCs are serous, endometrioid, clear cell, and mucous carcinoma (2). EOC is diagnosed with advanced disease in ~70% of the patients (4). Although debulking surgery combined with neoadjuvant or adjuvant platinum- or purple gold-based chemotherapy has become the standard treatment approach for patients with advanced EOC, survival rates for advanced ovarian cancer are still low, patients who are classified as stage III and IV under the Federation International of Gynecology and Obstetrics (FIGO) have the 5-year survival rates of only 42 and 26%, respectively (3, 5). In advanced EOC, a subset of patients dies within 3 months of diagnosis. For these patients, aggressive treatment did not significantly delay the survival of the patients, but reduced the quality of life of the patients. Exploring the related factors of early death can benefit clinicians as it distinguishes high-risk patients promptly and targeted palliative care, such as relieve cancer pain and other adverse symptoms, to improve the quality of life. To the best of our knowledge, very little attention has been paid to inspect factors associated with early death in advanced EOC. A premature mortality prediction model has become essential for patients with advanced EOC in order to guide oncologists to personalize treatments for patients.
The Surveillance, Epidemiology, and the End Results (SEER) database (https://seer.Cancer.gov/) have been widely used in the study of tumor pathogenesis and survival (6, 7). The SEER database registers ~34.6% of U.S. cancer patients and contains a wealth of clinical information. The SEER database had a larger number of patients compared to the single-center study. The present study evaluated the frequency of early death from advanced EOC recorded in the SEER database between 2004 and 2015 and established a prognostic model for early identification of high-risk patients.
Materials and Methods
Ethics Statement
The SEER database data does not need informed patient consent, and cancer is a reportable disease in every state in the United States. This study was consistent with the 1964 Helsinki Declaration and subsequent amendments or similar ethical standards.
Patients
Patient information was obtained using SEER*Stat (version 8.3.6.1). The inclusion criteria were as follows: (1) advanced ovarian epithelial neoplasm registered between 2004 and 2015 (FIGO stage III and IV), (2) Site code: C56.9, and (3) Histological code: 838/3-8482/3 (in accordance with the International Classification of Tumor Diseases, Third Edition (ICD-O-3). The excluding principles were: (1) lack of tumor size information; (2) no survival months recorded; (3) no cause of death; (4) no ethnicity; (5) no marital status; and (6) no surgical information. Figure 1 illustrates a flowchart of patient selection. According to past research (8, 9), death within 3 months of initial diagnosis was defined as early death.
Data Collection
Information on patients with advanced ovarian epithelial tumors was extracted from the SEER database. (1) Demographic information including race, age, insurance status, and marital status (2) Clinical features: histological subclassification, histological grading, laterality, tumor size, metastasis location, surgical information, radiotherapy information, and chemotherapy information. (3) Main results: early death tends to occur in FIGO stage III and IV patients (≤ 3 months).
Statistical Analysis
Using the X-tile software, the optimal cutoff values for age and tumor size were examined (10). The best cutoff values were 63 years and 76 years of age, and 27 mm for tumor size (Figure 2). All data was using Rsize. R Version 4.0.2 (The R Development Core Team, Vienna, Austria, http://www.r-project.org) to analyze in the RStudio environment. The statistical significance level was set at P < 0.05. Univariate and multivariate logistic regression analyses were both completed on the data gathered in order to examine factors linked with premature mortality. Constructed a nomogram based on the significant variables in multivariate logistic regression model. The receiver operating characteristic (ROC) curve was plotted to evaluate the performance of the nomogram (11). The R-language DCA package was utilized to assess the clinical impact of the nomogram by the decision curve analysis (DCA) (12). Bootstrapping (1,000 re-samplings) was used for internal validation, comparison between the validation model and original data was made using consistency statistics (C statistics) (13) and Brier score (14) to estimate the accuracy of the nomogram.
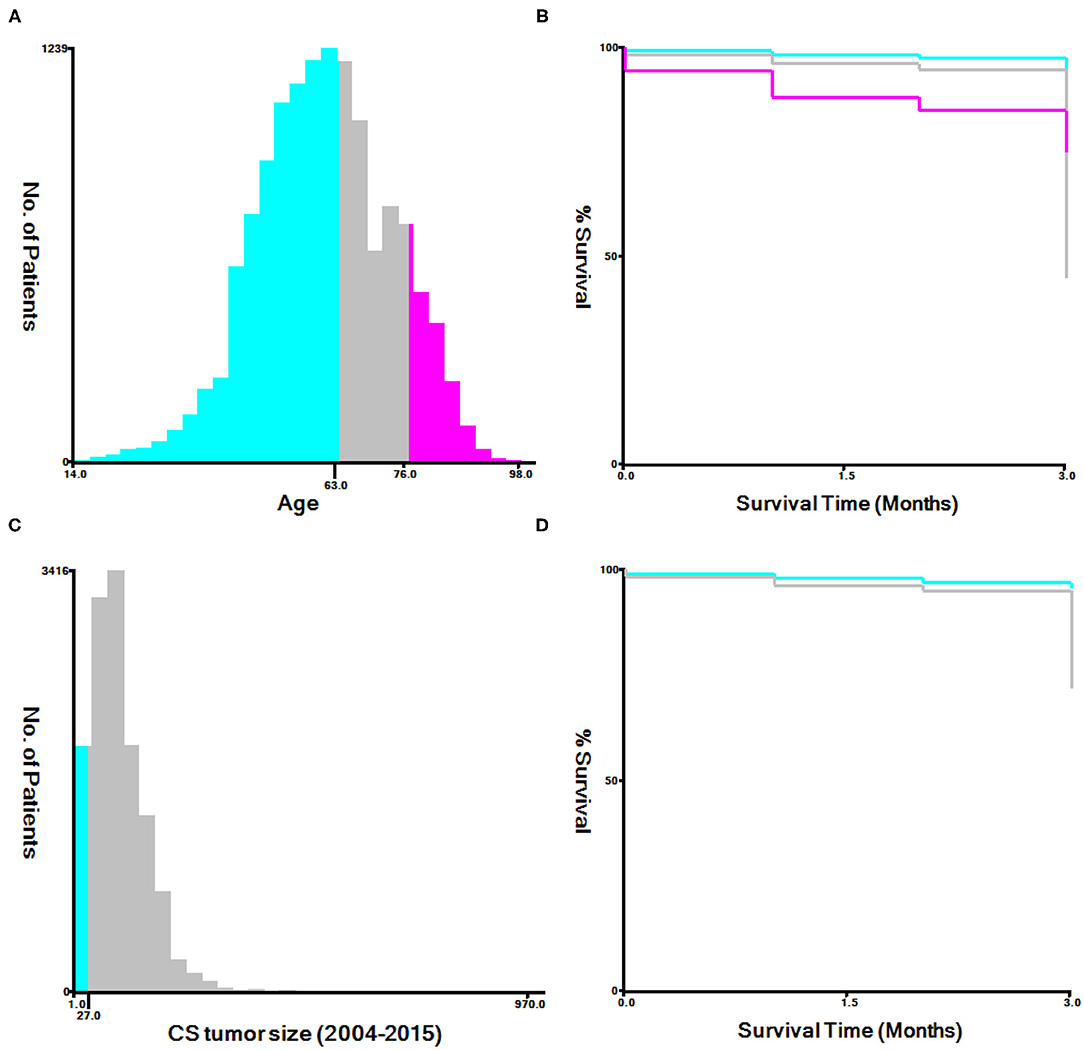
Figure 2. The appropriate age and tumor size cutoff values. (A,B): The appropriate cutoff values of age were 63 and 76 years; (C,D): The appropriate cutoff values of tumor size were 27 mm.
Results
Characteristics of Patients
A total of 23,599 patients with advanced ovarian epithelial tumors met the inclusion criteria, and 13,403 patients were eventually included in this study. There were 9,379 patients who were FIGO stage III, including 397 early deaths and 4,024 FIGO stage IV patients, including 414 early deaths. Table 1 demonstrates the patients characteristics.
Risk Factor Analysis for Early Death
The univariate and multivariate logistic regression analyses of early mortality in patients with advanced EOC are depicted in Tables 2, 3. Univariate analysis indicated that FIGO stage III patients and those who were older, of black race, widowed, and those who had mucinous cystadenocarcinoma, unilateral, large tumor, CA125 positive, non-operative, or non-chemotherapy patients had were at increased risk of premature mortality. In addition to the aforementioned risk factors, except for CA125 positivity, liver and lung metastases were also associated with increased risk of early death in FIGO stage IV patients. It was demonstrated through multivariate analysis that FIGO stage III, older, divorced/separated patients, as well as those with mucinous cystadenocarcinoma, CA125 positive, non-operative, or non-chemotherapy had an elevated risk of premature death. With regards to FIGO stage IV patients, elderly patients, and those with mucinous cystadenocarcinoma, liver metastases, lung metastases, and non-operative or non-chemotherapy, an increased risk of premature mortality was also observed.
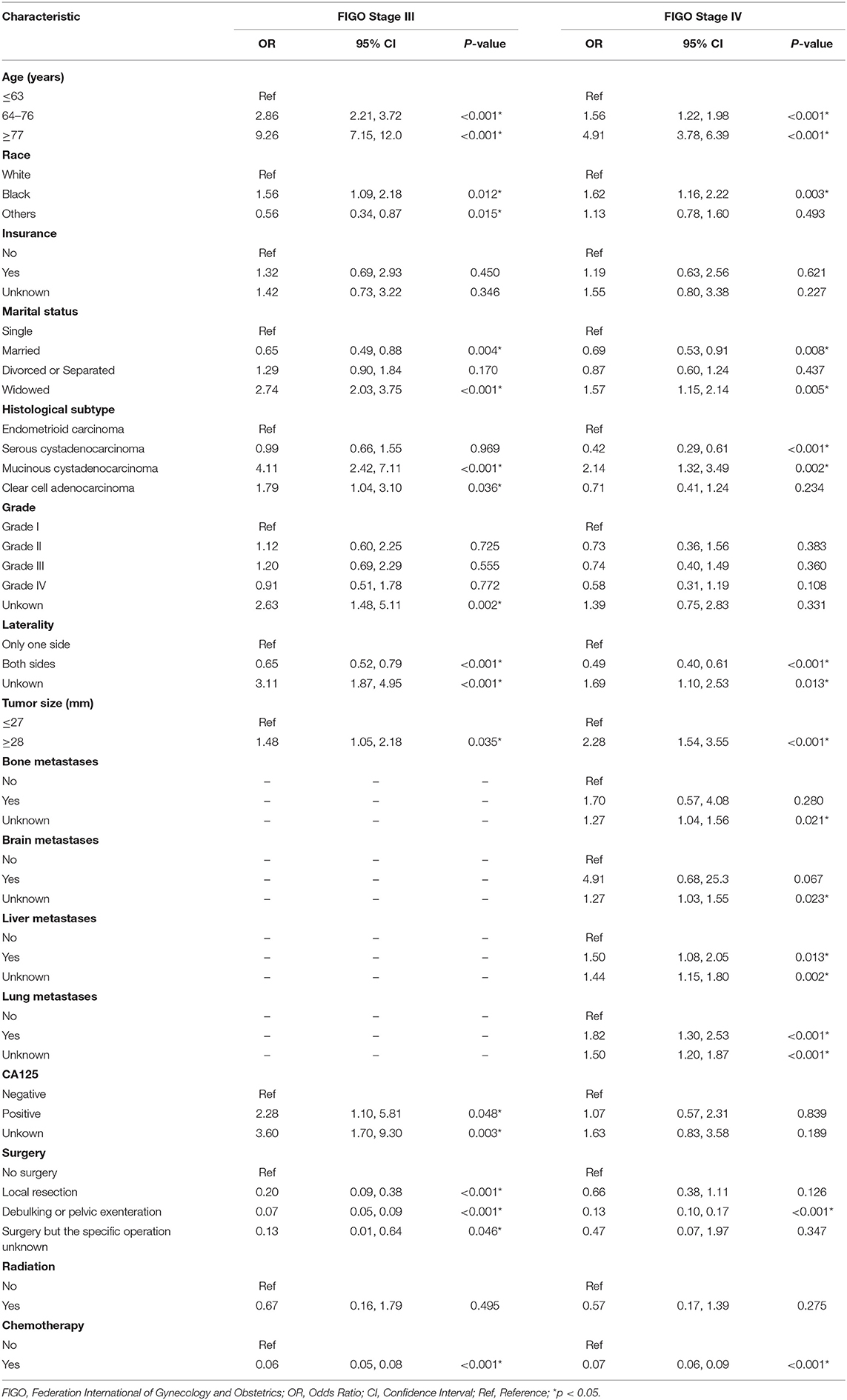
Table 2. The univariable logistic regression analysis of early death in patients with advanced epithelial ovarian cancer.
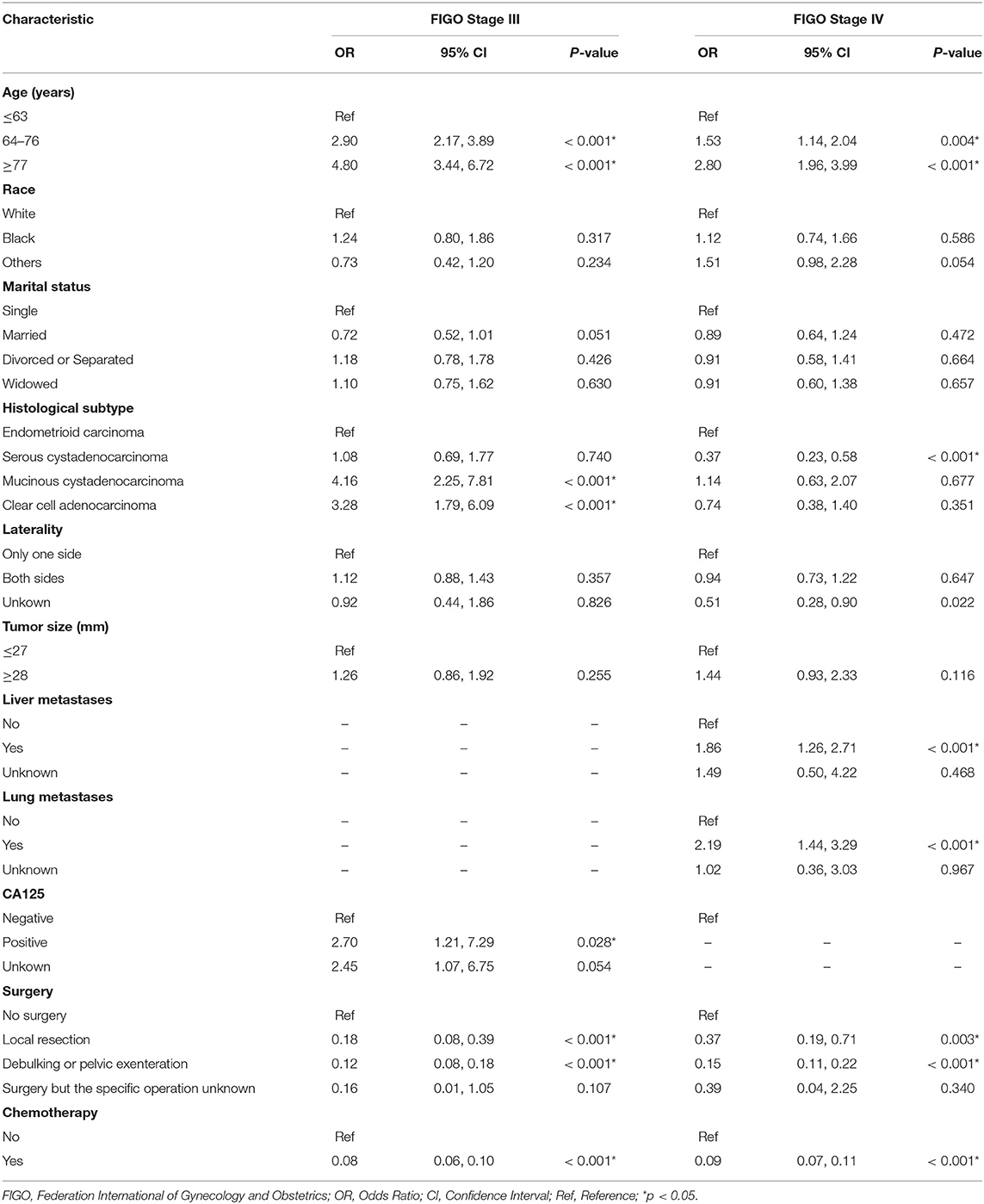
Table 3. The multivariate logistic regression analysis of early death in patients with advanced epithelial ovarian cancer.
Construct Nomogram
Several crucial variables from multiple logistic regression were selected, which include age, histological subtypes, liver metastasis, lung metastasis, surgical treatment, and chemotherapy. A nomogram of premature mortality in FIGO stage III and IV ovarian epithelial tumor patients was constructed (Figure 3).
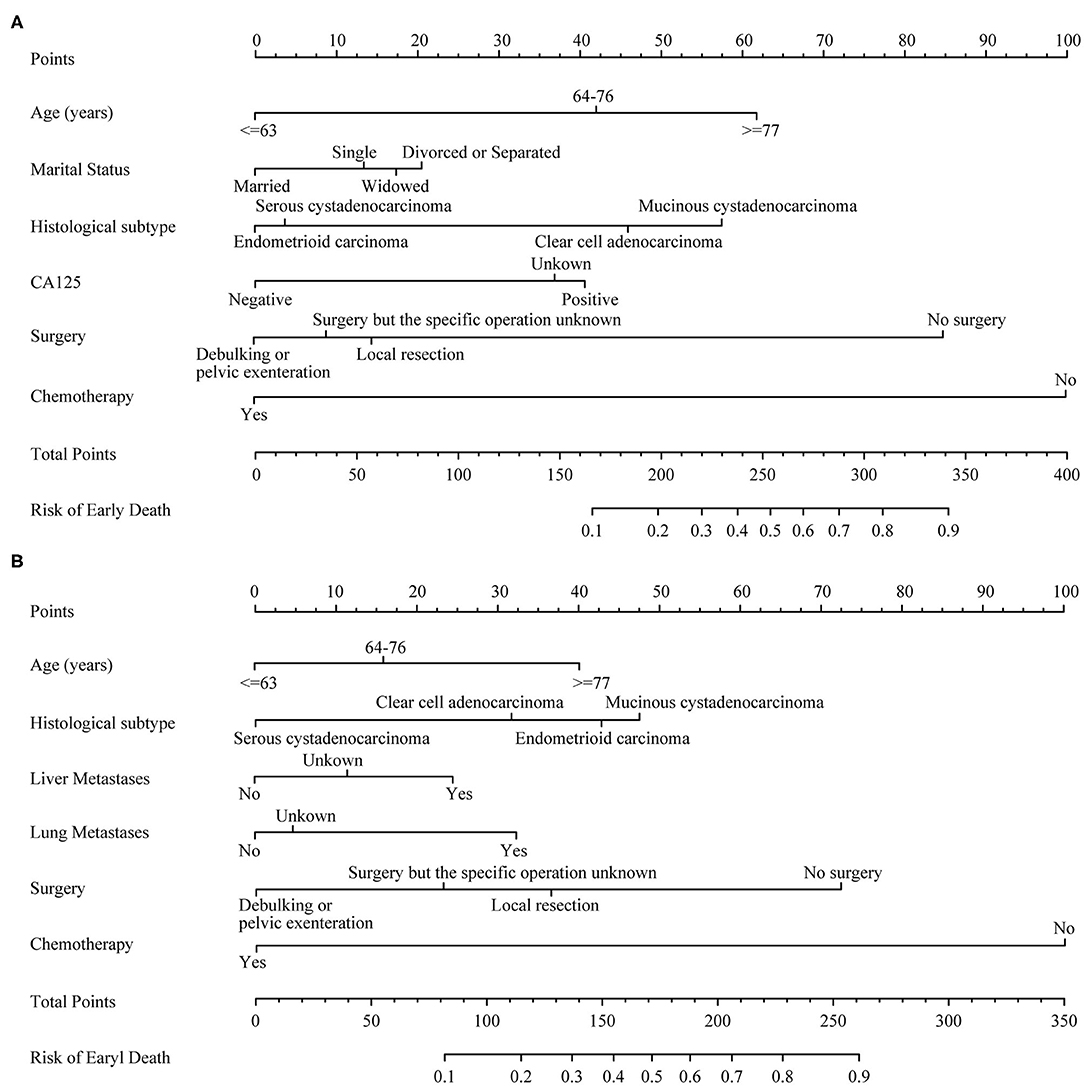
Figure 3. The nomograms of early death in patients with advanced epithelial ovarian cancer. (A) FIGO stage III; (B) FIGO stage IV.
Performance Evaluation of Nomograms
Figure 4 illustrates the ROC curve of nomograms used to evaluate FIGO stage III and IV EOC patients. The area under the ROC curve (AUC) of nomograms exceeds 85%, implying that nomograms have satisfactory predictive ability. Moreover, DCA results (Figure 5) show that the predictive model is clinically beneficial. All calibration curves have been internally verified and are close to the 45° line (Figure 6). The C-statistics and Brier scores before and after bootstrapping (1,000 resamplings) are displayed in Table 4. The internal validation demonstrates that the predicted value is consistent with the actual value.
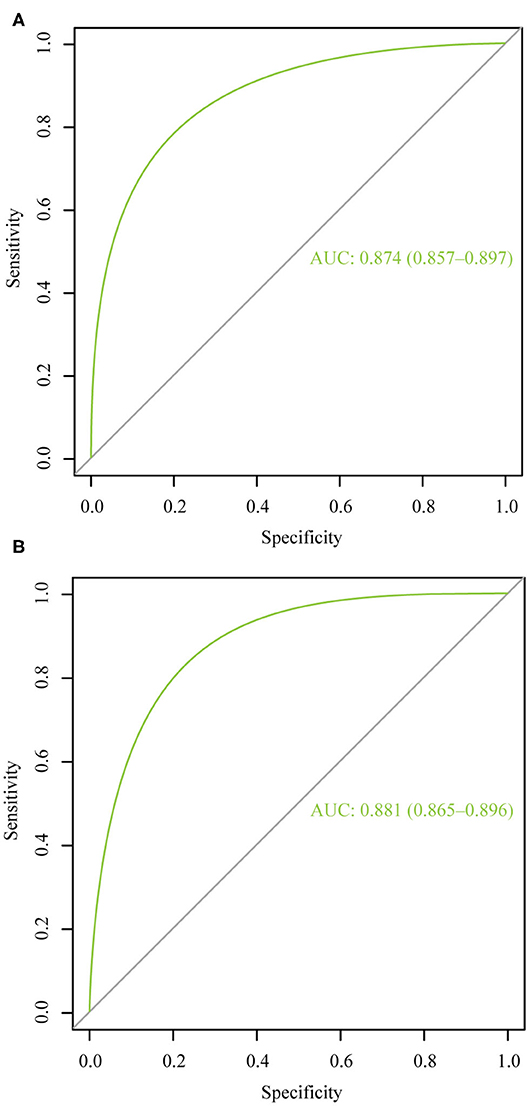
Figure 4. The receiver operating characteristic curve for nomogram. (A) FIGO stage III; (B) FIGO stage IV. AUC, area under the curve.
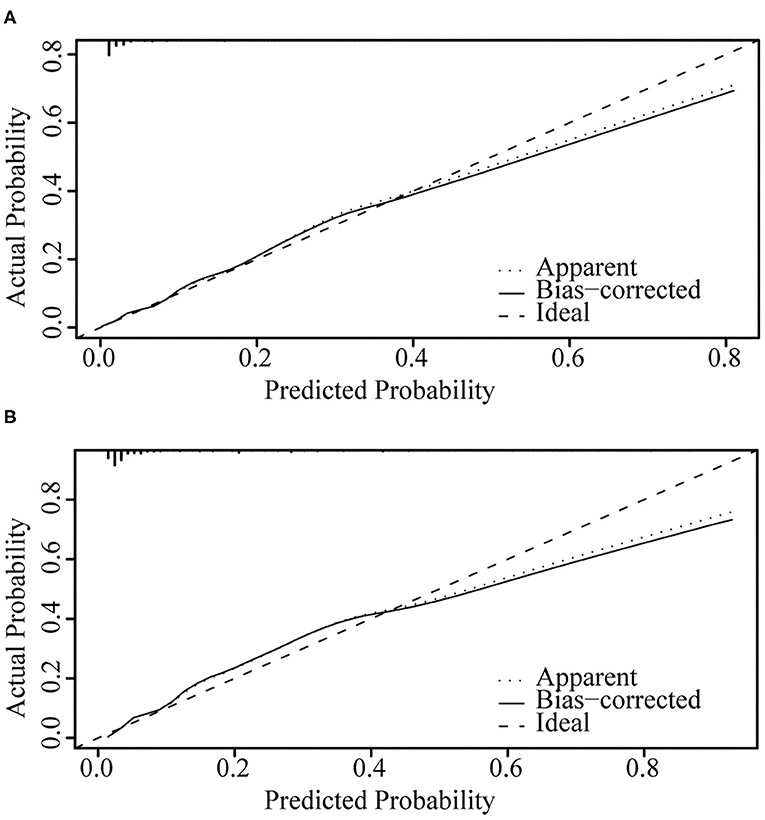
Figure 6. Internal verification plots of nomogram calibration curves by bootstrapping with 1,000 resamples. (A) FIGO stage III; (B) FIGO stage IV.
Discussion
Advanced EOC has a higher early death rate. In the present study, the premature mortality rate is 4.23% for FIGO stage III EOC and 10.29% for FIGO stage IV EOC. A set of predictive tools was necessary to identify high-risk patients early death and to provide personalized treatment. A nomogram is a commonly used prognostic tool. In recent years, nomograms have been used extensively to predict the risk and prognosis of malignant tumors (15, 16). Nomograms based on the SEER database have a larger population sample, so nomograms are more accurate and stable (17, 18). The innovation of our study is that, for the first time, the SEER database was used to construct a nomogram to predict the risk of early death in patients with advanced EOC. Our study showed that for FIGO stage III patients, those who were older, divorced/ separated, mucinous cystadenocarcinoma, CA125 positive, and those without surgery or chemotherapy had an elevated risk of premature mortality. For FIGO stage IV patients, those who were more elderly, have mucinous cystadenocarcinoma, liver metastasis, lung metastasis, who had not undergone surgery and chemotherapy have an increased likelihood of premature mortality.
Previous studies have elucidated that age is associated with the prognosis of EOC. Hanatani et al. have shown that younger patients with EOC or borderline EOC under the age of 40 are more likely to survive (19). Cress et al. also showed that younger age was an important predictor of long-term survival in patients with EOC (20). In our study, older age was an important predictor of early death in advanced EOC, consistent with the above findings.
In our study, the histological staging of advanced epithelial ovarian tumors was associated with premature mortality. For FIGO stage III epithelial ovarian tumors, the risk of premature death ranged from low to high for endometrioid carcinoma, serous cystadenocarcinoma, clear cell adenocarcinoma, and mucinous cystadenocarcinoma. For FIGO stage IV epithelial ovarian tumors, the risk of premature death also ranged from low to high for clear cell adenocarcinoma, serous cystadenocarcinoma, mucinous cystadenocarcinoma, and endometrioid carcinoma. Therefore, patients included in the present study with mucinous cystadenocarcinoma had the highest risk of early death, whether they were FIGO stage III or IV epithelial ovarian tumors. Previous studies have shown that mucinous ovarian cancer is usually diagnosed at a low level and early stage, therefore, regardless of the influence of FIGO staging, mucinous ovarian cancer generally has a better prognosis (21, 22). Hess et al. showed that compared with other histological subtypes of EOC, patients with advanced mucinous ovarian cancer had a poorer response to platinum-type first-line chemotherapy and a poorer survival rate (23). Winter et al. studied stage III epithelial ovarian tumors and found that compared with serous tumors, mucinous ovarian cancer and clear cell cancer had inferior progression-free survival (PFS) and overall survival (OS) rate (24). Although it is an epithelial ovarian tumor, the histological molecular structure of the ovarian mucinous tumor is different from other subtypes. Serous tumors are mainly caused by P53 mutations, whereas ovarian mucinous cancer is mainly caused by K-ras mutation (25). With regards to the effectiveness of platinum-based chemotherapy, better results are observed in advanced ovarian serous carcinoma compared with ovarian mucinous carcinoma (26). These may explain why different histological subtypes influence the risk of early death.
CA125 is a specific serum marker used to detect EOC. In the advanced stage of FIGO, CA125 levels are elevated (>35 U/mL) in about 90% of patients (27). However, the effect of CA125 on the prognosis of advanced EOC remains controversial. Studies have shown that PFS and OS are significantly better in advanced FIGO stage EOC when CA125 is <500 U/mL than when CA125 is >500 U/mL (28). However, there are studies that argue against it. Morales-Vasquez et al. described that a high level of CA125 (>500 U/mL) was associated with higher survival rates (29). In our study, for FIGO stage III patients, positive CA125 was associated with early death, while for FIGO stage IV patients, CA125 was not associated with early death. In the SEER database, CA125 only distinguishes between “negative,” “positive” and “unknown.” Unfortunately, due to the limitations of the SEER database, our study was unable to obtain the specific value of CA125 in patients and find the optimal truncation value of CA125 for predicting early death, contributing to one of the major limitations of this study.
The results of the present study are subjected to other limitations. Firstly, as a retrospective study, selection bias was unavoidable. A poor performance status also increase the risk of major complications after the surgery (30). Patients' performance status, comorbidities, Eastern Cooperative Oncology Group (ECOG) score, and other factors were not taken into account, therefore there was also a bias. Secondly, certain factors that might induce premature mortality were not incorporated in the study. For example, EOC has a large number of potential tumor biomarkers, including CA-125, WFDC2 (HE4) protein, and serum mesothelin (31). This study merely included CA125, lacking other important biomarkers. The tumor dissemination can play a role in determining prognosis in ovarian cancer patients (32). Due to the limitations of SEER database, the tumor dissemination, the residual tumor after the surgery, the type of chemotherapy and the maintenance therapy and other information with important significance for the prognosis of EOC were not included in this study, so the above information was not included. Thirdly, the SEER database contains a large amount of unidentified data, which could interfere the results of the model. Moreover, the model was not validated by external clinical data. Future studies could verify the present model with clinical data to assess its credibility.
In conclusion, the nomogram created in this study can effectively predict early death from advanced EOC. Thus, it can help clinicians screen patients at high risk and provide individualized treatment and improved survival.
Data Availability Statement
Publicly available datasets were analyzed in this study. This data can be found here: https://seer.Cancer.gov/.
Author Contributions
ZS: research ideas and drafting drafts. YZ: statistical analysis and manuscript writing. XB: data extraction. DZ: conception of research and quality control. All authors contributed to the article and approved the submitted version.
Funding
This research was supported by internal funding of Shengjing Hospital affiliated to China Medical University (SJ-M0133).
Conflict of Interest
The authors declare that the research was conducted in the absence of any commercial or financial relationships that could be construed as a potential conflict of interest.
References
1. Brooks SE, Zhan M, Cote T, Baquet CR. Surveillance, epidemiology, and end results analysis of 2677 cases of uterine sarcoma 1989-1999. Gynecol Oncol. (2004) 93:204–8. doi: 10.1016/j.ygyno.2003.12.029
2. Ramalingam P. Morphologic, immunophenotypic, and molecular features of epithelial ovarian cancer. Oncology (Williston Park). (2016) 30:166–76.
3. Torre LA, Trabert B, DeSantis CE, Miller KD, Samimi G, Runowicz CD, et al. Ovarian cancer statistics, 2018. CA Cancer J Clin. (2018) 68:284–96. doi: 10.3322/caac.21456
4. Lucidi A, Buca D, Ronsini C, Tinari S, Bologna G, Buca D, et al. Role of extracellular vesicles in epithelial ovarian cancer: a systematic review. Int J Mol Sci. (2020) 21:8762. doi: 10.3390/ijms21228762
5. Wu SG, Wang J, Sun JY, He ZY, Zhang WW, Zhou J. Real-world impact of survival by period of diagnosis in epithelial ovarian cancer between 1990 and 2014. Front Oncol. (2019) 9:639. doi: 10.3389/fonc.2019.00639
6. Ilcisin LA, Ma C, Janeway KA, DuBois SG, Shulman DS. Derivation and validation of risk groups in patients with osteosarcoma utilizing regression tree analysis. Pediatr Blood Cancer. (2020) 68:e28834. doi: 10.1002/pbc.28834
7. Zuo Z, Zhang G, Song P, Yang J, Li S, Zhong Z, et al. Survival nomogram for stage IB non-small-cell lung cancer patients, based on the SEER database and an external validation cohort. Ann Surg Oncol. (2020). doi: 10.1245/s10434-020-09362-0. [Epub ahead of print].
8. Wang X, Mao M, Xu G, Lin F, Sun P, Baklaushev VP, et al. The incidence, associated factors, and predictive nomogram for early death in stage IV colorectal cancer. Int J Colorectal Dis. (2019) 34:1189–201. doi: 10.1007/s00384-019-03306-1
9. Song Z, Wang Y, Zhang D, Zhou Y. A novel tool to predict early death in uterine sarcoma patients: a surveillance, epidemiology, and end results-based study. Front Oncol. (2020) 10:2670. doi: 10.3389/fonc.2020.608548
10. Camp RL, Dolled-Filhart M, Rimm DL. X-tile: a new bio-informatics tool for biomarker assessment and outcome-based cut-point optimization. Clin Cancer Res. (2004) 10:7252–9. doi: 10.1158/1078-0432.CCR-04-0713
11. Janssens A, Martens FK. Reflection on modern methods: revisiting the area under the ROC Curve. Int J Epidemiol. (2020) 49:1397–403. doi: 10.1093/ije/dyz274
12. Van Calster B, Wynants L, Verbeek JFM, Verbakel JY, Christodoulou E, Vickers AJ, et al. Reporting and interpreting decision curve analysis: a guide for investigators. Eur Urol. (2018) 74:796–804. doi: 10.1016/j.eururo.2018.08.038
13. Pencina MJ, D'Agostino RB. Overall C as a measure of discrimination in survival analysis: model specific population value and confidence interval estimation. Stat Med. (2004) 23:2109–23. doi: 10.1002/sim.1802
14. Roulston M. Performance targets and the brier score. Meteorol Appl. (2007) 14:185–94. doi: 10.1002/met.21
15. Sun L, Wang D, Zhang M, Jin Y, Jin B, Xu H, et al. Preoperative immune prognostic index can predict the clinical outcomes of patients with gallbladder cancer: single-center experience. Cancer Manag Res. (2020) 12:12137–50. doi: 10.2147/CMAR.S271044
16. Wei FZ, Mei SW, Chen JN, Wang ZJ, Shen HY, Li J, et al. Nomograms and risk score models for predicting survival in rectal cancer patients with neoadjuvant therapy. World J Gastroenterol. (2020) 26:6638–57. doi: 10.3748/wjg.v26.i42.6638
17. Huang Z, Hu C, Liu K, Yuan L, Li Y, Zhao C, et al. Risk factors, prognostic factors, and nomograms for bone metastasis in patients with newly diagnosed infiltrating duct carcinoma of the breast: a population-based study. BMC Cancer. (2020) 20:1145. doi: 10.1186/s12885-020-07635-1
18. Zhou G, Xiao K, Gong G, Wu J, Zhang Y, Liu X, et al. A novel nomogram for predicting liver metastasis in patients with gastrointestinal stromal tumor: a SEER-based study. BMC Surg. (2020) 20:298. doi: 10.1186/s12893-020-00969-4
19. Hanatani M, Yoshikawa N, Yoshida K, Tamauchi S, Ikeda Y, Nishino K, et al. Impact of age on clinicopathological features and survival of epithelial ovarian neoplasms in reproductive age. Int J Clin Oncol. (2020) 25:187–94. doi: 10.1007/s10147-019-01550-7
20. Cress RD, Chen YS, Morris CR, Petersen M, Leiserowitz GS. Characteristics of long-term survivors of epithelial ovarian cancer. Obstet Gynecol. (2015) 126:491–7. doi: 10.1097/AOG.0000000000000981
21. Heintz AP, Odicino F, Maisonneuve P, Quinn MA, Benedet JL, Creasman WT, et al. Carcinoma of the ovary. FIGO 26th annual report on the results of treatment in gynecological cancer. Int J Gynaecol Obstet. (2006) 95(Suppl. 1):S161–92. doi: 10.1016/S0020-7292(06)60033-7
22. Shimada M, Kigawa J, Ohishi Y, Yasuda M, Suzuki M, Hiura M, et al. Clinicopathological characteristics of mucinous adenocarcinoma of the ovary. Gynecol Oncol. (2009) 113:331–4. doi: 10.1016/j.ygyno.2009.02.010
23. Hess V, A'Hern R, Nasiri N, King DM, Blake PR, Barton DP, et al. Mucinous epithelial ovarian cancer: a separate entity requiring specific treatment. J Clin Oncol. (2004) 22:1040–4. doi: 10.1200/JCO.2004.08.078
24. Winter WE III, Maxwell GL, Tian C, Carlson JW, Ozols RF, Rose PG, et al. Prognostic factors for stage III epithelial ovarian cancer: a gynecologic oncology group study. J Clin Oncol. (2007) 25:3621–7. doi: 10.1200/JCO.2006.10.2517
25. Fujita M, Enomoto T, Murata Y. Genetic alterations in ovarian carcinoma: with specific reference to histological subtypes. Mol Cell Endocrinol. (2003) 202:97–9. doi: 10.1016/S0303-7207(03)00069-8
26. Pectasides D, Fountzilas G, Aravantinos G, Kalofonos HP, Efstathiou E, Salamalekis E, et al. Advanced stage mucinous epithelial ovarian cancer: the Hellenic cooperative oncology group experience. Gynecol Oncol. (2005) 97:436–41. doi: 10.1016/j.ygyno.2004.12.056
27. Soletormos G, Duffy MJ, Othman Abu Hassan S, Verheijen RH, Tholander B, Bast RC Jr, et al. Clinical use of cancer biomarkers in epithelial ovarian cancer: updated guidelines from the European group on tumor markers. Int J Gynecol Cancer. (2016) 26:43–51. doi: 10.1097/IGC.0000000000000586
28. Bachmann R, Brucker S, Stabler A, Kramer B, Ladurner R, Konigsrainer A, et al. Prognostic relevance of high pretreatment CA125 levels in primary serous ovarian cancer. Mol Clin Oncol. (2021) 14:8. doi: 10.3892/mco.2021.2247
29. Morales-Vasquez F, Pedernera E, Reynaga-Obregon J, Lopez-Basave HN, Gomora MJ, Carlon E, et al. High levels of pretreatment CA125 are associated to improved survival in high grade serous ovarian carcinoma. J Ovarian Res. (2016) 9:41. doi: 10.1186/s13048-016-0247-6
30. Vizzielli G, Costantini B, Tortorella L, Pitruzzella I, Gallotta V, Fanfani F, et al. A laparoscopic risk-adjusted model to predict major complications after primary debulking surgery in ovarian cancer: a single-institution assessment. Gynecol Oncol. (2016) 142:19–24. doi: 10.1016/j.ygyno.2016.04.020
31. Shah CA, Lowe KA, Paley P, Wallace E, Anderson GL, McIntosh MW, et al. Influence of ovarian cancer risk status on the diagnostic performance of the serum biomarkers mesothelin, HE4, and CA125. Cancer Epidemiol Biomarkers Prev. (2009) 18:1365–72. doi: 10.1158/1055-9965.EPI-08-1034
32. Vizzielli G, Costantini B, Tortorella L, Petrillo M, Fanfani F, Chiantera V, et al. Influence of intraperitoneal dissemination assessed by laparoscopy on prognosis of advanced ovarian cancer: an exploratory analysis of a single-institution experience. Ann Surg Oncol. (2014) 21:3970–7. doi: 10.1245/s10434-014-3783-6
Keywords: advanced epithelial ovarian cancer, nomograms, early death, SEER database, prognosis
Citation: Song Z, Zhou Y, Bai X and Zhang D (2021) A Practical Nomogram to Predict Early Death in Advanced Epithelial Ovarian Cancer. Front. Oncol. 11:655826. doi: 10.3389/fonc.2021.655826
Received: 19 January 2021; Accepted: 22 February 2021;
Published: 19 March 2021.
Edited by:
Giuseppe Vizzielli, Catholic University of the Sacred Heart, ItalyReviewed by:
Rita Trozzi, Agostino Gemelli University Polyclinic, ItalyMartina Arcieri, University of Messina, Italy
Elena Teodorico, Agostino Gemelli University Polyclinic, Italy
Copyright © 2021 Song, Zhou, Bai and Zhang. This is an open-access article distributed under the terms of the Creative Commons Attribution License (CC BY). The use, distribution or reproduction in other forums is permitted, provided the original author(s) and the copyright owner(s) are credited and that the original publication in this journal is cited, in accordance with accepted academic practice. No use, distribution or reproduction is permitted which does not comply with these terms.
*Correspondence: Dandan Zhang, zhangdd@sj-hospital.org