- School of Traditional Chinese Medicine, Beijing University of Chinese Medicine, Beijing, China
Background: Molecular characteristics can be good indicators of tumor prognosis and have been introduced into the classification of gliomas. The prognosis of patients with newly classified lower-grade gliomas (LGGs, including grade 2 and grade 3 gliomas) is highly heterogeneous, and new molecular markers are urgently needed.
Methods: Autophagy related genes (ATGs) were obtained from Human Autophagy Database (HADb). From the Cancer Genome Atlas (TCGA) and the Chinese Glioma Genome Atlas (CGGA), gene expression profiles including ATG expression information and patient clinical data were downloaded. Cox regression analysis, receiver operating characteristic (ROC) analysis, Kaplan–Meier analysis, random survival forest algorithm (RSFVH) and stratification analysis were performed.
Results: Through univariate Cox regression analysis, we found a total of 127 ATGs associated with the prognosis of LGG patients from TCGA dataset and a total of 131 survival-related ATGs from CGGA dataset. Using TCGA dataset as the training group (n = 524), we constructed a five-ATG signature (including BAG1, BID, MAP1LC3C, NRG3, PTK6), which could divide LGG patients into two risk groups with significantly different overall survival (Log Rank P < 0.001). Then we confirmed in the independent CGGA dataset that the five-ATG signature had the ability to predict prognosis (n = 431, Log Rank P < 0.001). We further discovered that the predictive ability of the five-ATG signature was better than the existing clinical indicators and IDH mutation status. In addition, the five-ATG signature could further classify patients after receiving radiotherapy or chemotherapy into groups with different prognosis.
Conclusions: We identified a five-ATG signature that could be a reliable prognostic marker and might be therapeutic targets for autophagy therapy for LGG patients.
Introduction
Glioma is a malignant tumor that seriously threatens human health worldwide. It is characterized by complex histopathological types, large differences in patient prognosis, and limited treatment options. By convention, the histopathological classification (World Health Organization classification) divides patients with glioma into grades 1 to 4, and is widely used by clinicians to evaluate prognosis and guide treatment. However, due to the wide range of patients' survival within each grade, the evaluation results of the classic TNM classification are not entirely satisfactory. In recent years, with the in-depth research in molecular biology, scientists have found that IDH 1 and 2 mutations and 1p/19q codeletion play a more important role than TNM stage in the prognosis of glioma patients (1, 2). In 2016, the WHO made a major change in the classification of gliomas, incorporating molecular characteristics into the classification (3). Subsequently, in the field of glioma, deep mining of gene expression data and the discovery of more effective molecular markers have become research hotspots.
Lower-grade gliomas (LGGs, WHO grades II and III gliomas) are more likely to occur in young people aged 20–40 (4). LGGs are highly malignant and have a high mortality rate, which cause a huge burden on society, families and individuals. In the past decades, treatment methods such as surgery, radiotherapy and chemotherapy have continued to develop, and the survival rate of LGG patients has been greatly improved. However, the prognosis of patients is highly heterogeneous. Some patients survive for only 1 year, while others can survive for 15 years (5). Therefore, LGG currently is currently facing two major problems, that is, the molecular markers that have been discovered (such as IDH mutation and 1p/19q coding status) cannot completely accurately distinguish the prognosis of LGG, and the existing treatment methods cannot further improve the survival rate of patients.
Autophagy is a highly regulated catabolic process that can maintain cell homeostasis under basal and stress conditions. There is accumulating evidence showing that autophagy plays a complex and contradictory role in the occurrence and development of tumors, and has the dual effects of promoting and inhibiting tumorigenesis (6, 7). When gliomas are involved, the dual function of autophagy also appears. Shukla et al. found that ULK1/2 in glioblastoma was down-regulated, while overexpression of ULK2 increased autophagy and inhibited tumor cell growth (8). Pallichankandy et al. reported that the up-regulation of reactive oxygen species (ROS) in gliomas can activate the ERK1/2 pathway and trigger autophagic cell death (9). Therefore, some scientists believe that autophagy, as a tumor suppressor, can degrade damaged proteins and mitochondria, and prevent the activation of oncogenic signaling pathways and accumulation of p62 aggregates, thereby inhibiting the malignant behavior of tumors such as cell proliferation, migration and invasion (10). Since autophagy degradation produces a large number of amino acids, fatty acids and metabolic substrates, which provide abundant nutrients and convenient conditions for the growth of tumor cells, other researchers believe that autophagy plays a pro-tumoral role in gliomas (11). In short, autophagy is of great significance in gliomas. At present, new autophagy inducers or inhibitors that promote the autophagic death of glioma cells are being developed and are expected to become a new therapy for glioma (12–14).
Autophagy related genes (ATGs) are a group of evolutionarily highly conserved genes, which are essential molecules and participate in all stages of autophagy. In recent years, a growing number of ATGs and the etiological association between ATGs and tumors (15) have been discovered. Therefore, ATGs may have potential value in the prognosis of LGG patients. Our study aims to find the prognostic biomarkers of LGG through exploring the association between ATG and the survival of LGG patients and constructing prognostic gene signature.
Materials and Methods
Gene Expression Data and Clinical Characteristics of LGG Patients
The gene expression profile of patients with LGG in The Cancer Genome Atlas Program (TCGA, August 17, 2018) was tested experimentally using the Illumina HiSeq 2000 RNA Sequencing platform and obtained from the UCSC Xena browser (https://xenabrowser.net/). Another dataset was downloaded from Chinese Glioma Genome Atlas (CGGA) database (http://www.cgga.org.cn/). The BIGD accession number of LGG dataset in China National Genomics Data Center is PRJCA001747 (https://bigd.big.ac.cn/bioproject/browse/PRJCA001747). TCGA dataset was used as the training group, and CGGA dataset was used as test group. All clinical information of the LGG patients is shown in Table 1. In order to facilitate the subsequent data analysis, we discarded genes with missing expression values in more than 20% of LGG samples (16).
Identification of Survival-Related Autophagy Related Genes in LGG
Autophagy related genes (ATGs) were obtained from the Human Autophagy Database (http://www.autophagy.lu/index.html), which contains the latest list of genes directly or indirectly involved in autophagy. We performed univariate Cox regression analysis to find the ATGs associated with patients' overall survival (OS) in the TCGA and CGGA datasets (P < 0.05).
The Process of Constructing and Validating the Prognostic ATG Signature
In order to find an ideal prognostic signature, we constructed the prognostic signature with TCGA data, and then verified the predictive ability of the signature in independent CGGA data. Since there were a large number of survival-related ATGs in the TCGA data, we used the random survival forest algorithm (RSFVH) to reduce the number of ATGs. In addition, we used the ATGs selected above to perform Cox regression analysis and construct risk models as follows: where N is the number of ATG, Expressioni is the expression value of the ATG and x i is the coefficient of ATG in Cox regression analysis. Then, receiver operating characteristic (ROC) analysis was performed to compare the predictive power of different risk models. Finally, we used the ATG signature with the largest AUC value as the best prognostic signature (17).
After screening the best signature through the prognostic risk model, we performed survival analysis to test the predictive ability of the ATG signature in both the training and test groups.
Statistical and Bioinformatics Analysis
Multivariable Cox regression analysis was performed to explore the predictive independence of the ATG signature. ROC was used to compare the survival prediction performance of the ATG signature with other prognostic markers. Kaplan–Meier analysis was tested to verify the stratification significance of the ATG signature for patients after receiving radiotherapy and chemotherapy (18). R 3.5.1 version (downloaded from www.r-project.org) with R packages including pROC, timeROC, randomForestSRC, and survival were used for analysis, where a P-value of < 0.05 was considered statistically significant.
Results
Identification of Survival-Related ATGs of LGG Patients
A total of 232 autophagy related genes were acquired from the HADb database. By comparison, we detected 208 ATGs from 26,440 expressed genes in the TCGA dataset (n = 524) and 210 ATGs from 23,998 expressed genes in the CGGA (n = 431) dataset. After analyzing the clinical data of 955 LGG patients, we found that the median age of the 955 LGG patients was 40 years (11–87 years), and there are more men in the affected population, indicating that LGG is more likely to occur in young adult males. In addition to gene expression data, patients also have complete radiotherapy, chemotherapy and IDH mutation status information, which is listed in Table 1.
To assess the prognostic significance of ATG in LGG, we performed univariate Cox regression analysis and identified 127 and 131 ATGs that were significantly associated with OS of LGG patients from the TCGA and CGGA datasets, respectively (P < 0.05, Figure 1A, Supplementary Table 1).
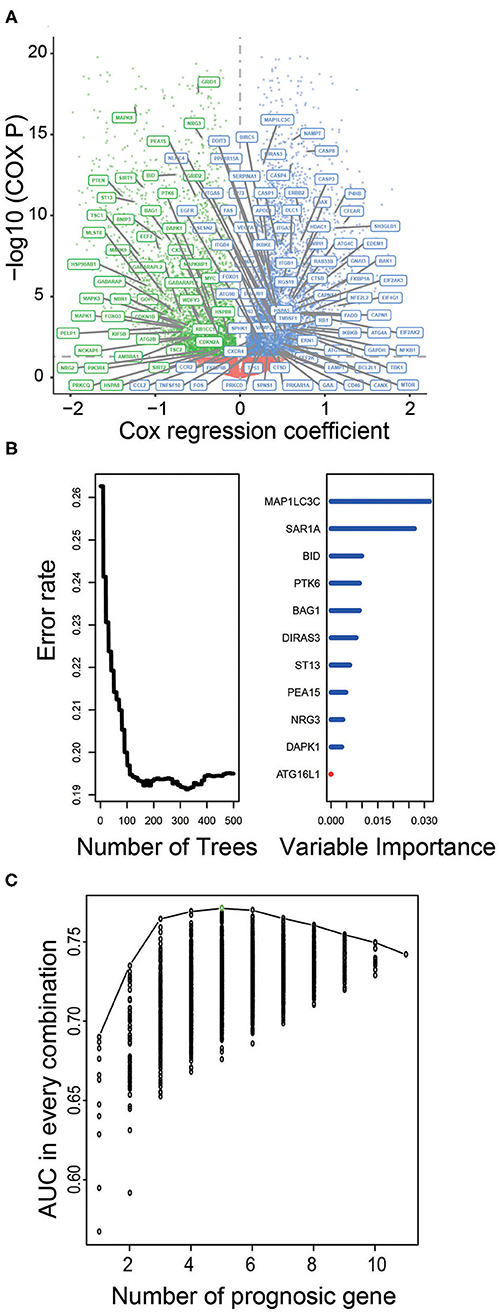
Figure 1. Identification of prognostic ATGs and constructing prognostic models in the training dataset. (A) Volcano plot showed the ATGs associated with LGG survival in the training set. (B) The prognostic ATGs were reduced to 11 by random forest supervised classification algorithm. (C) The prognostic five-ATG signature was selected for its largest AUC (AUC = 0.71).
Construction and Evaluation of Risk Prediction Model in the Training Dataset
We used the TCGA dataset as the training group to develop risk prediction model and construct prognostic signature. Since we discovered 127 ATGs associated with the survival of LGG patients (Figure 1A), we further performed RSFVH analysis and screened out 11 ATGs based on importance scores (Figure 1B). Then we used the 11 prognostic ATGs to develop the risk prediction model, and got 211-1 = 2,047 ATG risk models. We performed ROC analysis and compared the predictive ability of 2,047 ATG risk models (Supplementary Table 2). The ATG signature including five ATGs (BAG1, BID, MAP1LC3C, NRG3, PTK6) was found to have the largest AUC value (AUC signature = 0.771; Figure 1C, Table 2). The selected risk model was calculated as follows: Risk score = (−0.75 × expression value of BID) + (−0.36 × expression value of PTK6) + (−0.82 × expression value of BAG1) + (−0.45 × expression value of NRG3) + (0.27 × expression value of MAP1LC3C). The regression coefficients of the four ATGs (BID, PTK6, BAG1, and NRG3) were all negative, which means they were genes related to poor prognosis, while MAP1LC3C was the opposite, indicating a good prognosis.
Survival Prediction Performance of the Five-ATG Signature in the Training and Test Dataset
Each patient received a risk score based on the five-ATG signature. Then, the patients with LGG in the training dataset were divided into high-risk (n = 262) or low-risk group (n = 262) based on the median risk score. Through displaying the risk score, survival status and the five ATGs expression in a dot plot or heat map, we found that patients with high-risk scores had higher expression of BID, PTK6, BAG1, and NRG3 and were prone to death (Figure 2A). Kaplan–Meier analysis demonstrated that patients in the low-risk group owned longer survival times than those in the high-risk group (median survival time: 9.51 vs. 3.70 years, log-rank test P < 0.001; Figure 2B). Subsequently, we performed time-dependent ROC analysis to assess the prognostic accuracy of the five-ATG signature. In the TCGA dataset, the AUC for 1, 3, and 5 years of survival were 0.89, 0.84, and 0.76, respectively (Figure 2C).
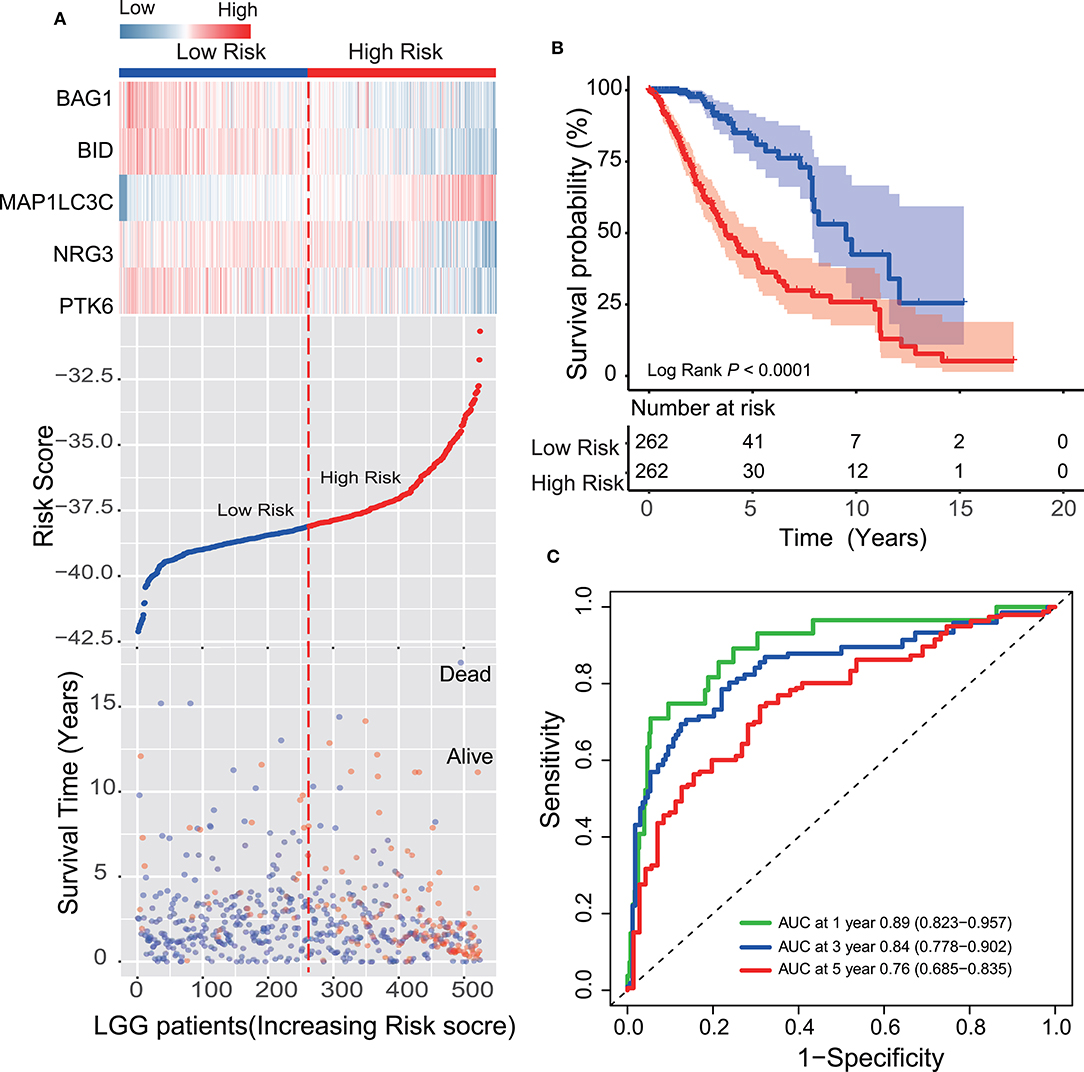
Figure 2. Survival prediction performance of the ATG signature in the training dataset. (A) Expression heatmap of the five ATGs, dot plot of risk scores and survival status of LGG patients in the training dataset. (B) Kaplan–Meier analysis demonstrated that the five-ATG signature divided patients into two risk groups with different survival. (C) Time-dependent ROC analysis of the ATG signature in the training group.
In another large independent LGG dataset from CGGA (n = 431), the median risk score divided patients into high-risk or low-risk groups. From the dot plot and heat map, we found the relationship between the risk score, survival status and the five ATGs expression (Figure 3A). Kaplan–Meier analysis verified that the survival time of LGG patients in the high-risk group was shorter than that of patients in the low-risk group (median survival time: 2.96 vs. 7.21 years, log-rank test P < 0.001; Figure 3B). Time-dependent ROC analysis results showed that the AUC of the five-ATG signature was 0.65, 0.65, and 0.62 at the survival time of 1, 3, and 5 years in the CGGA dataset (Figure 3C).
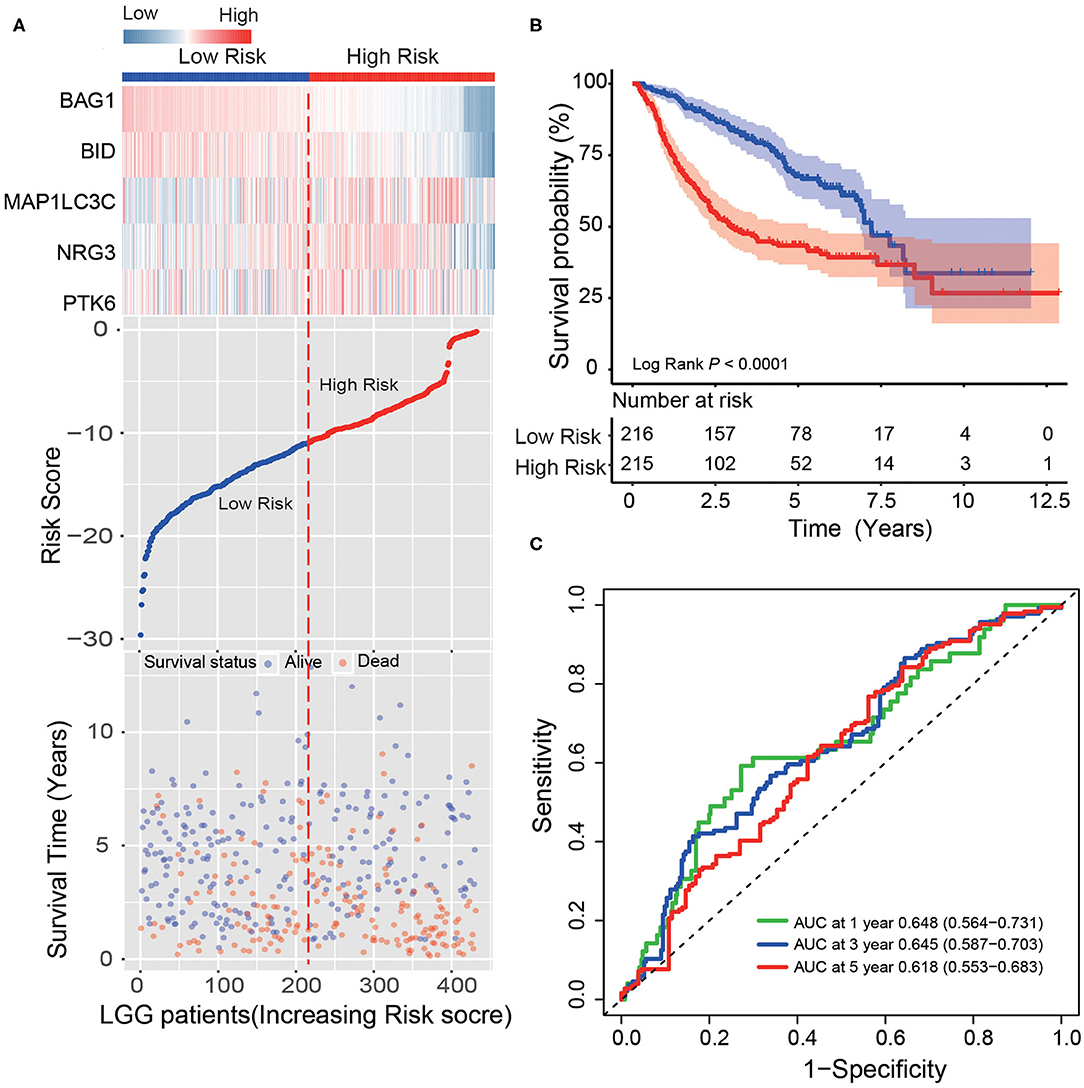
Figure 3. Validating survival prediction performance of the ATG signature in the test dataset. (A) Expression heatmap of the five ATGs, dot plot of risk scores and survival status of LGG patients in the test dataset. (B) Kaplan–Meier plot found that the five-ATG signature divided patients into high-risk and low-risk groups with different survival. (C) Time-dependent ROC analysis of the ATG signature in the test group.
The Five-ATG Signature Is an Independent Predictive Factor
After confirming that the five-ATG signature has an excellent survival prediction ability, we tested its prognostic independence. We conducted Chi-square test in the training and test groups (n = 524/431) and found that the five-ATG signature was related to IDH mutation status and radiotherapy (Table 3). Then univariate and multivariable Cox regression analyses were conducted. The multivariable Cox regression results in the training and test datasets verified that the five-ATG signature can predict patients' survival without being affected by IDH mutation status and radiotherapy (High- vs. Low-risk, HR training = 8.71, 95% CI 1.1.86–40.71, P = 0.006, n = 524; HR test = 1.93, 95% CI 1.36–2.73, P < 0.001, n = 431, Table 4).
The Five-ATG Signature Is Better Than Existing Prognostic Indicators
The prognostic indicators currently used clinically include age, TNM staging and IDH mutation status. To compare the predictive performance of the five-ATG signature with that of the existing indicators. We drew ROC curves and found that the five-ATG signature had the biggest AUC value in the training/test datasets (AUC signature 0.771/0.64; AUCIDH 0.712/0.585; AUC grade 0.625/0.632; AUC age 0.57/0.528, Figure 4), indicating that the five-ATG signature had a better survival prediction performance.
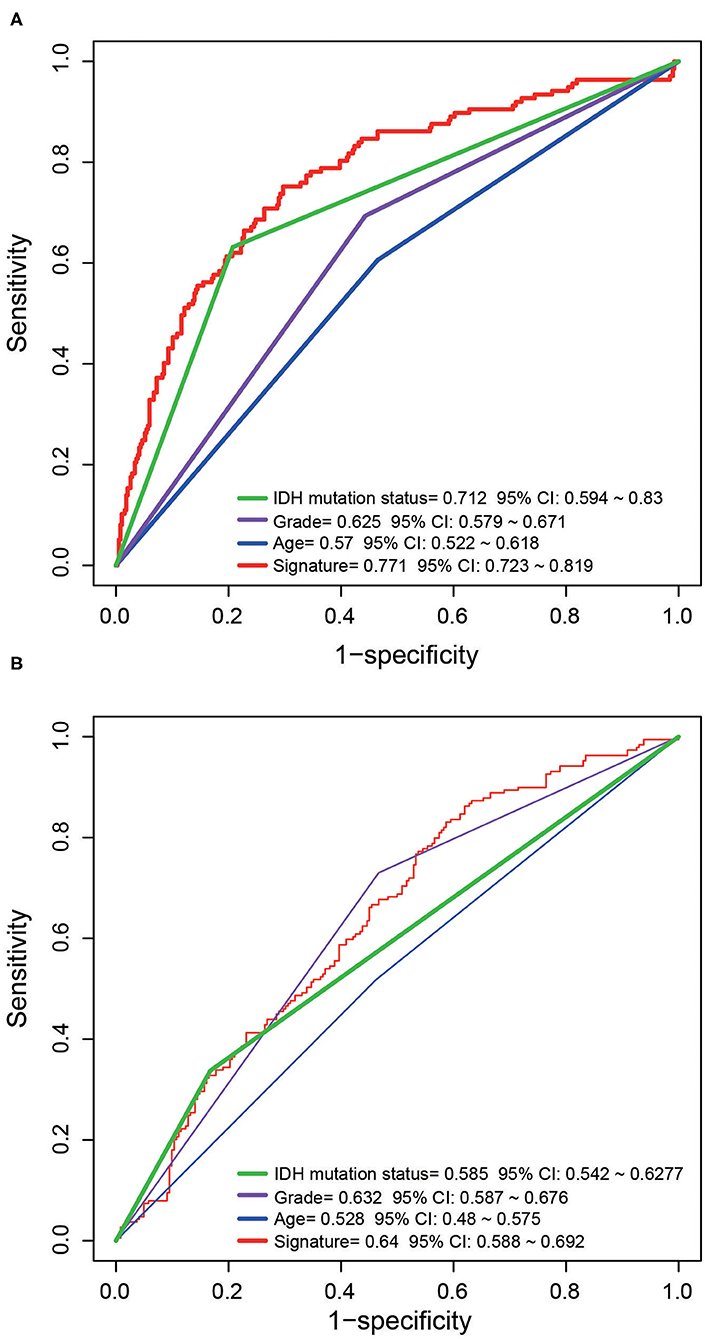
Figure 4. The predictive performance of the five-ATG signature was better than that of grade. Age and IDH mutation in the training (A) and test (B) datasets.
Radiotherapy and Chemotherapy Stratification Analysis
Since LGG is milder than the highest-grade GBM, it is controversial whether LGG patients should receive radiotherapy or chemotherapy immediately after diagnosis, or “wait and see.” For those who have received treatment (radiotherapy or chemotherapy), whether this five-ATG signature can predict prognosis is worth exploring. After determining the prognostic value of the signature, we conducted stratification analysis of all patients from TCGA and CGGA who received radiotherapy or chemotherapy. From Table 1, we obtained a total of 598 cases who received radiotherapy (284 from TCGA dataset and 314 from CGGA dataset). We observed these 598 patients who have undergone radiotherapy and found that these patients could be further divided into low- and high-risk groups with significantly different survival by the five-ATG signature (5 or 10-years survival: 68.47%/28.61 vs. 42.91%/18.71%, log-rank test P < 0.001, Figure 5A). Among the 559 patients with chemotherapy information in the two datasets, we found that a total of 366 patients received chemotherapy. Figure 5B showed that the five-ATG signature divided these 559 patients into two groups with different prognosis (5 or 10-years survival: 63.62%/33.44 vs. 42.56%/22.29%, log-rank test P < 0.001, Figure 5B).
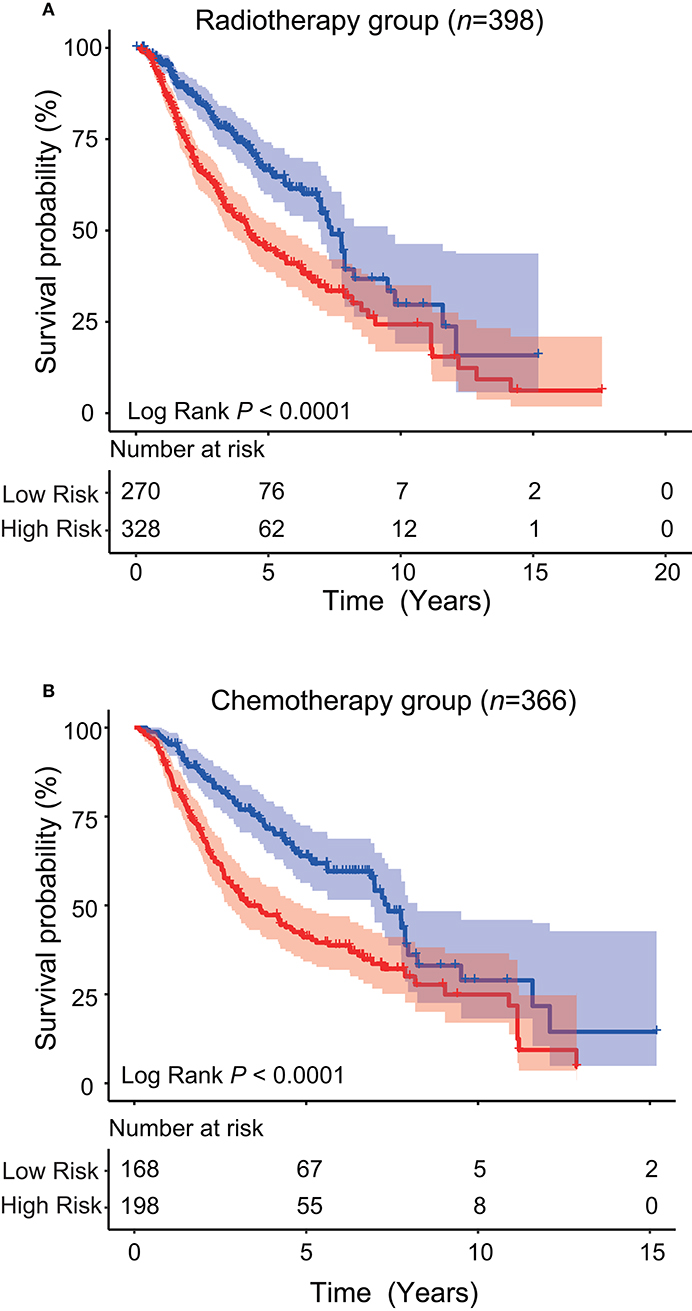
Figure 5. Stratification analysis. The five-ATG signature could further divide patients after radiotherapy (A) or patients after chemotherapy (B) into two groups with significantly different survival.
Discussion
After the new molecular classification of glioma in 2016, LGG has become a large group of tumors that is different from glioblastoma and grade I glioma in terms of prognosis. However, biological tumor heterogeneity is still a huge challenge for patient prognosis assessment and precise treatment, which makes the prognosis, treatment response and drug resistance of patients vary greatly. Recently, the exploration of the molecular characteristics of glioma has provided many potential markers that can be used for glioma classification, prognosis judgment and treatment guidance. In the present study, we analyzed gene expression and clinical data of 955 LGG cases from two different cohorts, and developed a prognostic signature based on the expression of autophagy related genes.
Due to its invasive growth characteristics, LGG cannot be completely removed by surgery, nor can be cured by existing treatment options, and gradually turns into a lethal glioblastoma. Therefore, it is justified to find new prognostic biomarkers and therapeutic strategies. In recent years, with the development of related research on the role of autophagy in tumors, researchers have discovered that ATGs could be used as prognostic biomarkers and be potential therapeutic targets. Among them, Beclin-1, and microtubule-associated protein light chain 3 (LC3) have become research hotspots. Studies have shown that abberrant expression of Beclin-1 is strongly associated with the poor survival of various tumors such as intrahepatic cholangiocarcinoma (19), endometrial adenocarcinoma (20), colorectal cancer (21). LC3 was found to be related to tumor growth and progression of triple negative breast cancer (22), and be associated with clinical prognosis of pancreatic cancer (23), hepatocellular carcinoma (24) and colorectal cancer (25). Our study found that 127 and 131 ATGs were closely related to the OS of LGG patients from the TCGA and CGGA datasets, among which a total of 80 ATGs were present in both groups. After constructing the risk model, we screened out the signature composed of five ATGs (BID, BAG1, PTK6, NRG3, MAP1LC3C) with the best prognosis prediction performance, indicating these five ATGs play an important role in glioma.
BAG1 (Bcl-2-associated athanogene) and BID (BH3 interacting domain death agonist) are key genes that play a regulatory role in the process of apoptosis. BAG1 is an oncogene that can enhance the anti-apoptotic effect of BLC-2 (26) and is aberrantly expressed in multiple cancer types. It has been suggested that BAG1 can be used as a potential drug target (27). BID is a member of the BCL-2 family and promotes apoptosis (28). Studies have found that BID is associated with the survival of thyroid cancer and clear-cell renal cell carcinoma. PTK6 (Protein tyrosine kinase six) is a cytoplasmic non-receptor protein kinase thatis highly expressed in tumors such as breast cancer, bladder cancer, lung cancer, and ovarian carcinoma. It has been detected that PTK6 overexpression is correlate with the poor prognosis of bladder cancer (29), prostate cancer (30), and breast cancer (31). NRG3 (neuregulin three) is abundantly expressed in brain tissue. NRG3 has been demonstrated to be involved in oligodendrocyte survival through binding and activating erbB4 (32). This study found that BID, BAG1, PTK6, NRG3 were significantly related to the poor prognosis of LGG through gene expression profile data analysis, supplementing the possible role of these genes in LGG. MAP1LC3C (microtubule associated protein one light chain three gamma) is a member of ATG8 family and plays a critical role in the process of autophagy. In addition, studies have shown that cancer cells with low MAP1LC3C levels exhibit enhanced cell invasion. Consistent with this finding, we found that LC3C is an indicator of good clinical prognosis for LGG.
In recent years, gene signatures have been proven to be good molecular biomarkers for various types of cancer due to their powerful ability to distinguish the prognosis of cancer patients (33). In combination with the important role of autophagy in tumors, researchers are currently exploring the effectiveness of ATG-gene signature in evaluating tumor prognosis. Gu et al. found an autophagy-related prognostic signature for breast cancer (34). Zhou et al. developed an ATG-signature which could predict the post-operative survival of colorectal cancer patients (35). Yue et al. identified an ATG-signature that can be used to analyze the prognosis of pancreatic adenocarcinoma patients (36). In gliomas, Wang et al. constructed a signature with four autophagy-related genes and validated its prognostic performance in GBM; Xu et al. found an autophagy-related signature that can divided patients into different survival outcomes groups (37). For LGG patients, only one seven-ATG signature was constructed for individualized survival prediction (38). Our study constructed a five-ATG signature which had a good predictive performance and could be an independent prognostic factor. Moreover, we found the significance of the five-ATG signature in guiding the prognosis of LGG patients after radiotherapy or chemotherapy.
Conclusion
In conclusion, our study found multiple ATGs related to the survival of LGG and developed a five-ATG signature based on the risk score model, which could be a promising prognostic biomarker for LGG patients. Further research on these five ATGs may contribute to provide targets for LGG autophagy therapy.
Data Availability Statement
The original contributions presented in the study are included in the article/Supplementary Material, further inquiries can be directed to the corresponding author/s.
Author Contributions
J-CG: data collection, data analysis, interpretation, and drafting. FL and YZ: study design, study supervision, and final approval of the manuscript. Q-SW, LD, and S-SF: technical support and critical revision of the manuscript. All authors read and approved the final manuscript.
Funding
This work was supported by grant from National Natural Science Foundation of China (32000439) and China Postdoctoral Science Foundation (2019M660576, 2019M660572).
Conflict of Interest
The authors declare that the research was conducted in the absence of any commercial or financial relationships that could be construed as a potential conflict of interest.
Supplementary Material
The Supplementary Material for this article can be found online at: https://www.frontiersin.org/articles/10.3389/fonc.2021.644443/full#supplementary-material
Abbreviations
LGG, lower-grade glioma; ATG, Autophagy related gene; TCGA, The Cancer Genome Atlas; CGGA, the Chinese Glioma Genome Atlas; ROC, receiver operating characteristic; KM, Kaplan–Meier; AUC, area under the ROC curve; OS, overall survival.
References
1. Eckel-Passow JE, Lachance DH, Molinaro AM, Walsh KM, Decker PA, Sicotte H, et al. Glioma groups based on 1p/19q, IDH, and TERT promoter mutations in tumors. N Engl J Med. (2015) 372:2499–508. doi: 10.1056/NEJMoa1407279
2. Tom MC, Cahill DP, Buckner JC, Dietrich J, Parsons MW, Yu JS. Management for different glioma subtypes: are all low-grade gliomas created equal? Am Soc Clin Oncol Educ Book. (2019) 39:133–45. doi: 10.1200/EDBK_238353
3. Wesseling P, Capper D. WHO 2016 Classification of gliomas. Neuropathol Appl Neurobiol. (2018) 44:139–50. doi: 10.1111/nan.12432
4. Duffau H. Paradoxes of evidence-based medicine in lower-grade glioma: To treat the tumor or the patient? Neurology. (2018) 91:657–62. doi: 10.1212/WNL.0000000000006288
5. Cancer Genome Atlas Research N, Brat DJ, Verhaak RG, Aldape KD, Yung WK, Salama SR, et al. Comprehensive, integrative genomic analysis of diffuse lower-grade gliomas. N Engl J Med. (2015) 372:2481–98. doi: 10.1056/NEJMoa1402121
6. Levy JMM, Towers CG, Thorburn A. Targeting autophagy in cancer. Nat Rev Cancer. (2017) 17:528–42. doi: 10.1038/nrc.2017.53
7. Huang T, Song X, Yang Y, Wan X, Alvarez AA, Sastry N, et al. Autophagy and hallmarks of cancer. Crit Rev Oncog. (2018) 23:247–67. doi: 10.1615/CritRevOncog.2018027913
8. Shukla S, Patric IR, Patil V, Shwetha SD, Hegde AS, Chandramouli BA, et al. Methylation silencing of ULK2, an autophagy gene, is essential for astrocyte transformation and tumor growth. J Biol Chem. (2014) 289:22306–18. doi: 10.1074/jbc.M114.567032
9. Pallichankandy S, Rahman A, Thayyullathil F, Galadari S. ROS-dependent activation of autophagy is a critical mechanism for the induction of anti-glioma effect of sanguinarine. Free Radic Biol Med. (2015) 89:708–20. doi: 10.1016/j.freeradbiomed.2015.10.404
10. Escamilla-Ramirez A, Castillo-Rodriguez RA, Zavala-Vega S, Jimenez-Farfan D, Anaya-Rubio I, Briseno E, et al. Autophagy as a potential therapy for malignant glioma. Pharmaceuticals (Basel). (2020) 13:156. doi: 10.3390/ph13070156
11. Trejo-Solis C, Serrano-Garcia N, Escamilla-Ramirez A, Castillo-Rodriguez RA, Jimenez-Farfan D, Palencia G, et al. Autophagic and apoptotic pathways as targets for chemotherapy in glioblastoma. Int J Mol Sci. (2018) 19:3773. doi: 10.3390/ijms19123773
12. Taylor MA, Das BC, Ray SK. Targeting autophagy for combating chemoresistance and radioresistance in glioblastoma. Apoptosis. (2018) 23:563–75. doi: 10.1007/s10495-018-1480-9
13. Kocaturk NM, Akkoc Y, Kig C, Bayraktar O, Gozuacik D, Kutlu O. Autophagy as a molecular target for cancer treatment. Eur J Pharm Sci. (2019) 134:116–37. doi: 10.1016/j.ejps.2019.04.011
14. Ulasov I, Fares J, Timashev P, Lesniak MS. Editing cytoprotective autophagy in glioma: an unfulfilled potential for therapy. Trends Mol Med. (2020) 26:252–62. doi: 10.1016/j.molmed.2019.11.001
15. Levine B, Kroemer G. Biological functions of autophagy genes: a disease perspective. Cell. (2019) 176:11–42. doi: 10.1016/j.cell.2018.09.048
16. Xu J, Li Y, Lu J, Pan T, Ding N, Wang Z, et al. The mRNA related ceRNA-ceRNA landscape and significance across 20 major cancer types. Nucleic Acids Res. (2015) 43:8169–82. doi: 10.1093/nar/gkv853
17. Guo JC, Li CQ, Wang QY, Zhao JM, Ding JY, Li EM, et al. Protein-coding genes combined with long non-coding RNAs predict prognosis in esophageal squamous cell carcinoma patients as a novel clinical multi-dimensional signature. Mol Biosyst. (2016) 12:3467–77. doi: 10.1039/C6MB00585C
18. Yu G, Wang LG, Han Y, He QY. clusterProfiler: an R package for comparing biological themes among gene clusters. OMICS. (2012) 16:284–7. doi: 10.1089/omi.2011.0118
19. Bi C, Liu M, Rong W, Wu F, Zhang Y, Lin S, et al. High Beclin-1 and ARID1A expression corelates with poor survival and high recurrence in intrahepatic cholangiocarcinoma: a histopathological retrospective study. BMC Cancer. (2019) 19:213. doi: 10.1186/s12885-019-5429-3
20. Giatromanolaki A, Koukourakis MI, Koutsopoulos A, Chloropoulou P, Liberis V, Sivridis E. High Beclin 1 expression defines a poor prognosis in endometrial adenocarcinomas. Gynecol Oncol. (2011) 123:147–51. doi: 10.1016/j.ygyno.2011.06.023
21. Koukourakis MI, Giatromanolaki A, Sivridis E, Pitiakoudis M, Gatter KC, Harris AL. Beclin 1 over- and underexpression in colorectal cancer: distinct patterns relate to prognosis and tumour hypoxia. Br J Cancer. (2010) 103:1209–14. doi: 10.1038/sj.bjc.6605904
22. Hamurcu Z, Delibasi N, Gecene S, Sener EF, Donmez-Altuntas H, Ozkul Y, et al. Targeting LC3 and Beclin-1 autophagy genes suppresses proliferation, survival, migration and invasion by inhibition of Cyclin-D1 and uPAR/Integrin beta1/ Src signaling in triple negative breast cancer cells. J Cancer Res Clin Oncol. (2018) 144:415–30. doi: 10.1007/s00432-017-2557-5
23. Yang YH, Liu JB, Gui Y, Lei LL, Zhang SJ. Relationship between autophagy and perineural invasion, clinicopathological features, and prognosis in pancreatic cancer. World J Gastroenterol. (2017) 23:7232–41. doi: 10.3748/wjg.v23.i40.7232
24. Hsu CC, Hsieh PM, Chen YS, Lo GH, Lin HY, Dai CY, et al. Axl and autophagy LC3 expression in tumors is strongly associated with clinical prognosis of hepatocellular carcinoma patients after curative resection. Cancer Med. (2019) 8:3453–63. doi: 10.1002/cam4.2229
25. Guo GF, Wang YX, Zhang YJ, Chen XX, Lu JB, Wang HH, et al. Predictive and prognostic implications of 4E-BP1, Beclin-1, and LC3 for cetuximab treatment combined with chemotherapy in advanced colorectal cancer with wild-type KRAS: Analysis from real-world data. World J Gastroenterol. (2019) 25:1840–53. doi: 10.3748/wjg.v25.i15.1840
26. Tang SC. BAG-1, an anti-apoptotic tumour marker. IUBMB Life. (2002) 53:99–105. doi: 10.1080/15216540211473
27. Kizilboga T, Baskale EA, Yildiz J, Akcay IM, Zemheri E, Can ND, et al. Bag-1 stimulates Bad phosphorylation through activation of Akt and Raf kinases to mediate cell survival in breast cancer. BMC Cancer. (2019) 19:1254. doi: 10.1186/s12885-019-6477-4
28. Gahl RF, Dwivedi P, Tjandra N. Bcl-2 proteins bid and bax form a network to permeabilize the mitochondria at the onset of apoptosis. Cell Death Dis. (2016) 7:e2424. doi: 10.1038/cddis.2016.320
29. Xu XL, Ye YL, Wu ZM, He QM, Tan L, Xiao KH, et al. Overexpression of PTK6 predicts poor prognosis in bladder cancer patients. J Cancer. (2017) 8:3464–73. doi: 10.7150/jca.21318
30. Wozniak DJ, Hitchinson B, Gilic MB, Bie W, Gaponenko V, Tyner AL. Vemurafenib Inhibits Active PTK6 in PTEN-null Prostate Tumor Cells. Mol Cancer Ther. (2019) 18:937–46. doi: 10.1158/1535-7163.MCT-18-0862
31. Irie HY, Shrestha Y, Selfors LM, Frye F, Iida N, Wang Z, et al. PTK6 regulates IGF-1-induced anchorage-independent survival. PLoS ONE. (2010) 5:e11729. doi: 10.1371/journal.pone.0011729
32. Carteron C, Ferrer-Montiel A, Cabedo H. Characterization of a neural-specific splicing form of the human neuregulin 3 gene involved in oligodendrocyte survival. J Cell Sci. (2006) 119:898–909. doi: 10.1242/jcs.02799
33. Burska AN, Roget K, Blits M, Soto Gomez L, van de Loo F, Hazelwood LD, et al. Gene expression analysis in RA: towards personalized medicine. Pharmacogenomics J. (2014) 14:93–106. doi: 10.1038/tpj.2013.48
34. Gu Y, Li P, Peng F, Zhang M, Zhang Y, Liang H, et al. Autophagy-related prognostic signature for breast cancer. Mol Carcinog. (2016) 55:292–9. doi: 10.1002/mc.22278
35. Zhou Z, Mo S, Dai W, Ying Z, Zhang L, Xiang W, et al. Development and validation of an autophagy score signature for the prediction of post-operative survival in colorectal cancer. Front Oncol. (2019) 9:878. doi: 10.3389/fonc.2019.00878
36. Yue P, Zhu C, Gao Y, Li Y, Wang Q, Zhang K, et al. Development of an autophagy-related signature in pancreatic adenocarcinoma. Biomed Pharmacother. (2020) 126:110080. doi: 10.1016/j.biopha.2020.110080
37. Xu Y, Li R, Li X, Dong N, Wu D, Hou L, et al. An autophagy-related gene signature associated with clinical prognosis and immune microenvironment in gliomas. Front Oncol. (2020) 10:571189. doi: 10.3389/fonc.2020.571189
Keywords: lower-grade glioma, autophagy related gene, prognostic biomarker, signature, survival
Citation: Guo J-C, Wei Q-S, Dong L, Fang S-S, Li F and Zhao Y (2021) Prognostic Value of an Autophagy-Related Five-Gene Signature for Lower-Grade Glioma Patients. Front. Oncol. 11:644443. doi: 10.3389/fonc.2021.644443
Received: 21 December 2020; Accepted: 15 February 2021;
Published: 09 March 2021.
Edited by:
Meng Zhou, Wenzhou Medical University, ChinaReviewed by:
Chengliang Yin, Chinese PLA General Hospital Beijing, ChinaHongqing Cai, Chinese Academy of Medical Sciences, China
Copyright © 2021 Guo, Wei, Dong, Fang, Li and Zhao. This is an open-access article distributed under the terms of the Creative Commons Attribution License (CC BY). The use, distribution or reproduction in other forums is permitted, provided the original author(s) and the copyright owner(s) are credited and that the original publication in this journal is cited, in accordance with accepted academic practice. No use, distribution or reproduction is permitted which does not comply with these terms.
*Correspondence: Yi Zhao, biozy@ict.ac.cn; Feng Li, 1575054688@qq.com
†These authors have contributed equally to this work and share first authorship