- 1Department of Radiotherapy, Cancer Center, West China Hospital, Sichuan University, Chengdu, China
- 2State Key Laboratory of Biotherapy and Cancer Center, West China Hospital, Collaborative Innovation Center for Biotherapy, Sichuan University, Chengdu, China
- 3Department of Radiology, The Affiliated Hospital of Southwest Medical University, Luzhou, China
- 4School of Computer Science & Engineering, South China University of Technology, Guangzhou, China
- 5Department of Head and Neck Oncology, Cancer Center, West China Hospital, Sichuan University, Chengdu, China
- 6Department of Pathology, The Affiliated Hospital of Southwest Medical University, Luzhou, China
Purpose: To build and evaluate a radiomics-based nomogram that improves the predictive performance of the LVSI in cervical cancer non-invasively before the operation.
Method: This study involved 149 patients who underwent surgery with cervical cancer from February 2017 to October 2019. Radiomics features were extracted from T2 weighted imaging (T2WI). The radiomic features were selected by logistic regression with the least absolute shrinkage and selection operator (LASSO) penalty in the training cohort. Based on the selected features, support vector machine (SVM) algorithm was used to build the radiomics signature on the training cohort. Incorporating radiomics signature and clinical risk factors, the radiomics-based nomogram was developed. The sensitivity, specificity, accuracy, and area under the curve (AUC) and Receiver operating characteristic (ROC) curve were calculated to assess these models.
Result: The radiomics model performed much better than the clinical model in both training (AUCs 0.925 vs. 0.786, accuracies 87.5% vs. 70.5%, sensitivities 83.6% vs. 41.7% and specificities 90.9% vs. 94.7%) and testing (AUCs 0.911 vs. 0.706, accuracies 84.0% vs. 71.3%, sensitivities 81.1% vs. 43.4% and specificities 86.4% vs. 95.0%). The combined model based on the radiomics signature and tumor stage, tumor infiltration depth and tumor pathology yielded the best performance (training cohort, AUC = 0.943, accuracies 89.5%, sensitivities 85.4% and specificities 92.9%; testing cohort, AUC = 0.923, accuracies 84.6%, sensitivities 84.0% and specificities 85.1%).
Conclusion: Radiomics-based nomogram was a useful tool for predicting LVSI of cervical cancer. This would aid the selection of the optimal therapeutic strategy and clinical decision-making for individuals.
Introduction
Cervical cancer is the fourth most frequently diagnosed cancer and the fourth leading cause of cancer death in women in 2018 worldwide (1). For early-stage disease, both radical hysterectomies with lymph nodes dissection and pelvic radiation therapy with vaginal brachytherapy are equally effective with approximate 5-year survival rates of 87% to 92% (2–4). Following surgery, postoperative radiotherapy (PORT) is indicated for patients with adverse pathologic factors by the National Comprehensive Cancer Network (NCCN) and the 2018 International Federation of Gynecology and Obstetrics (FIGO) report (5, 6). Increased morbidity and complications have been specifically demonstrated when surgery and radiotherapy are combined (7, 8). Therefore, a preoperative and noninvasive assessment to predict adverse pathologic factors is of great importance to optimize a treatment plan to lower the incidence of post-treatment morbidity and improve the quality of life.
Lymph-vascular space invasion (LVSI) is defined as the presence of carcinoma cells within the lymphatic and/or blood vessels (9). LVSI has been widely recognized as a risk factor in cervical cancer. Previous studies suggest that the presence of LVSI predicts risk of nodal metastasis which has direct impact on the prognosis of cervical cancer patients (10). NCCN guidelines recommend that adjuvant pelvic radiation ± chemotherapy should be taken by patients, when “Sedlis Criteria” was met (Table 1). The tumor size and the depth of invasion could be predicted preoperatively with a good performance, while studies on LVSI are much less and the predictive performance of LVSI is unsatisfying (11, 12). As the important but missing link for patients to select an appropriate treatment, the accurate prediction of LVSI before surgery is urgent in clinical practice.
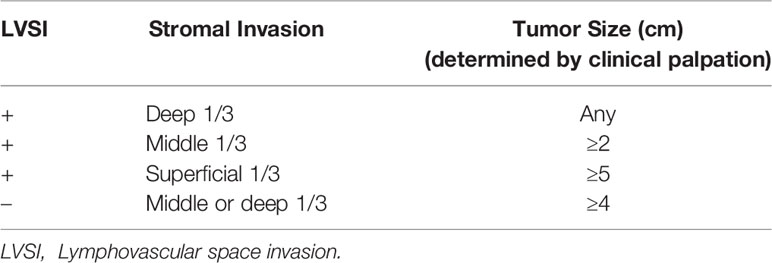
Table 1 Sedlis Criteria for external pelvic radiation after radical hysterectomy in node-negative, margin-negative, parametria-negative cases.
Radiomics analysis can extract a large number of quantitative features from medical images and convert them into various high-dimensional data, providing information on tumor heterogeneity (13–15). Successful evaluation and predictive capabilities have been achieved in a variety of challenging clinical analysis by developing appropriate model refinement features. Radiomic analysis can be used to diagnose diseases such as lymph node metastatic status of rectal cancer, bladder cancer, and breast cancer cost-effectively and non-invasively (16–20). T2 weighted MRI and diffusion-weighted imaging are also widely used in the staging of localized cervical cancer.
Therefore, the objective of the study was to build and evaluate a radiomics-based nomogram for improving the predictive performance of the LVSI in cervical cancer.
Materials and Methods
Patient
Our hospital ethics committee approved this retrospective study and patient informed consent was obtained. 301 consecutive patients with cervical cancer with biopsy-proven cervical carcinoma received initial treatment in our hospital between February 2017 and October 2019 were enrolled in our study. The inclusion criteria were as follows: (i) patients who underwent surgery with pathologically confirmed cervical cancer; (ii) All patients who received a pretreatment MRI scan; (iii) primary cervical lesions were visible on sagittal T2WI. The exclusion criteria were as follows: (i) history of preoperative therapy (neoadjuvant chemotherapy, radiotherapy, or conization); (ii) absence of preoperative MR in this hospital (iii) insufficient image quality; (iv) rare types of cervical tumor. MRI scans were reviewed by two radiologists with 7 and 5 years of experience. The patient selection process is shown in Figure 1.
MRI Acquisition Protocol
All patients recruited in this study were examined using the 3.0T MRI scanner (Achieva 3.0 T, Philips, Amsterdam, Netherlands) equipped with a 16-channel abdominal coil. The MR scan covered the area from the superior edge of the iliac crest to the lower edge of the pubic symphysis. The parameters of some 3.0T MRI sequences were as follows: axial fat-suppressed turbo spin-echo (TSE) T2-weighted images (TR/TE: 4854/85ms, FOV = 300 × 300 mm; matrix = 232 × 171; slice thickness/gap: 5/1mm; NEX = 2).
Image Segmentation
3D slicer software was explored for three-dimensional manual segmentation (open-source software; https://download.slicer.org/). All manual segmentations of the tumor tissues on axial T2WI were done by a radiologist who had 5 years of experience in gynecological MR imaging. And each segmentation was validated by a senior radiologist, who had 7 years of experience. The representative images of lesions are in Figure 2. The workflow of the radiomics analysis is presented in Figure 3.
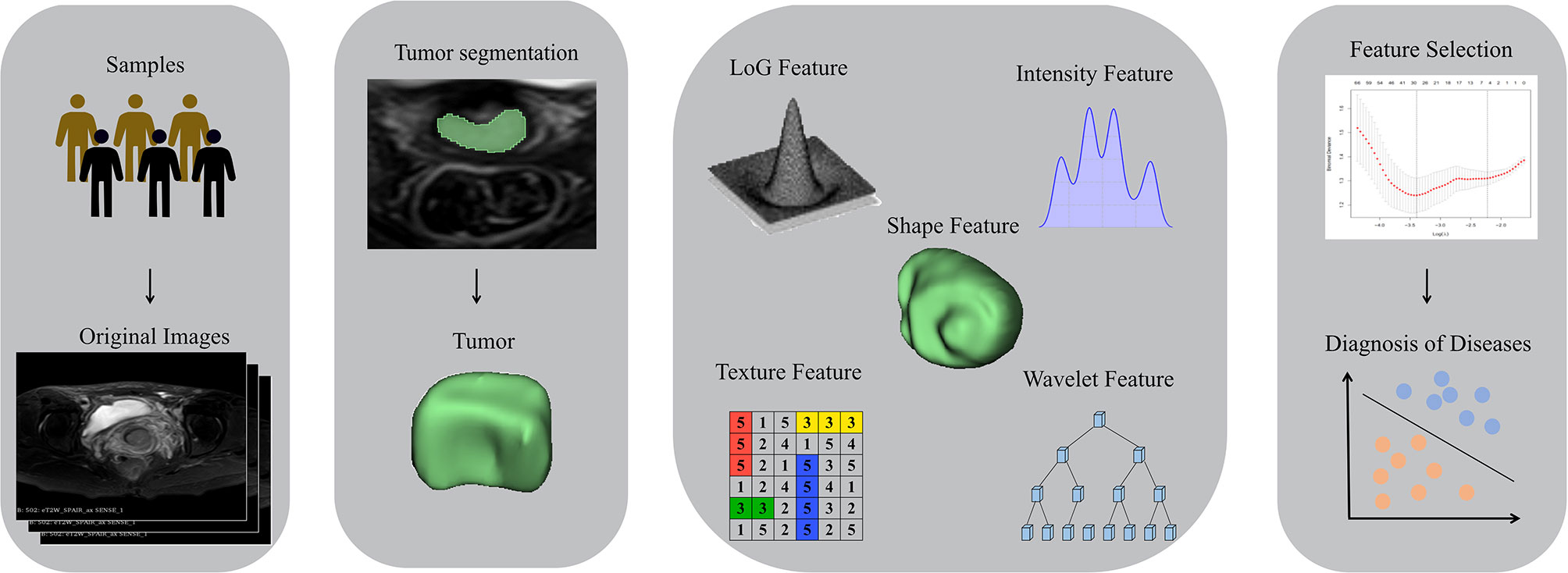
Figure 3 Workflow of radiomics analysis. T2WI images were collected. Regions of interests (ROI) of the tumor lesions were manually delineated. Radiomics features were extracted. Discriminative features were selected by the LASSO regression model. Prediction model was constructed by radiomics signature; ROC curves were performed for further statistical analyses.
Radiomic Feature Extraction
After manual segmentation of the tumor was completed, open-source software called pyradiomics was used to complete the extraction of the radiographic features of the tumor. Standardized operations on T2WI images were used to obtain a standardized normal-distribution image distribution. On T2WI of the tumor, we extracted 1682 radiological features from seven image types. These features are used to quantify tumor size (e.g., volume), shape (e.g., circumference, diameter), grayscale co-occurrence matrices (e.g., energy, contrast, entropy), grayscale run-length matrices, and grayscale dependency matrices. All features are implemented using python 3.7.
Radiomics Feature Selection and Development of Radiomics Models
Although radiographic features reflect the characteristics of the tumor tissue from different perspectives, not all information is relevant to cervical cancer. We used the LASSO algorithm as a means of our feature extraction to select non-zero coefficients in the training data by 10-fold crossover regression to obtain the desired radiomics features. Chalkidou et al. proposed that a minimum of 10 to 15 observations per predictor variable is required to build a reasonably stable model. The variables need to be modeled in a reasonably stable manner. Therefore, recursive feature elimination (RFE) features are selected to avoid further overfitting. Radiomics Model Building.
After feature selection, we use a support vector machine (SVM) model to predict sample types. This SVM model uses a linear kernel and the regularization parameter C to determine the best possible effect of the model. The C value that maximizes the AUC is used as the best parameter for the training group.
Development of Clinical Model
The stromal invasion depth, MTD and FIGO stages showed a significant difference (p < 0.05) between LVSI positive and LVSI negative groups in either the training cohort or the validation cohort in Table 2. The stromal invasion depth, MTD and FIGO stages were studied to build the clinical predictive model. SVM model was used to predict sample types.
Development of the Radiomics-Based Nomogram
The combined model was built using the logistic regression method with forward stepwise selection. The radiomics signature and clinical risk factors were used in the combined model. To make the model a more easy-to-use tool for preoperative prediction of the LVSI status, the combined model was visualized as the radiomics-based nomogram. The formula of the radiomics signature of the final radiomics model is shown in Supplementary S1.
Assessment of Predictive Models
The performances of these predictive models were first assessed on the training cohort and then validated on the validation cohort using receiver operating characteristic (ROC) curve. Calibration curve were used to assess the agreement between nomogram prediction probabilities of the LVSI status and actual outcomes. To assess the difference between the radiomics-based nomogram and the clinical model, the DeLong test was performed.
Statistical Analysis
Statistical analysis was conducted with SPSS 21, R 3.4.1 and python 3.7. The independent-sample t-test was used to compare the mean value on age between different groups. The chi-squared test or Fisher’s exact test was used to evaluate the significance of the categorical variables in the training and validation cohorts, respectively. Two-tailed p-values <0.05 were considered statistically significant. The logistic regression with the LASSO penalty and the SVM model was implemented using python 3.7 in the scikit-learn package.
Result
Demographic, Clinical, and Histopathological Characteristics
Of the 301 patients underwent surgery with cervical cancer from February 2017 to October 2019, 26 patients with preoperative neoadjuvant chemotherapy and 18 patients with preoperative conization were excluded; 56 patients and 42 patients were excluded for having no preoperative MRI in the hospital or no assessable lesions on MRI; 10 patients were excluded for rare tumor type of cervix; finally, 149 patients fulfilled the eligibility criteria and were enrolled in following analysis (Figure 1).
Patient characteristics are summarized in Table 2. There were no significant differences in the clinical characteristics between the training and validation cohorts. The stromal invasion and p-LN status showed a statistical difference between patients with and without LVSI both in the training and validation cohorts, as were shown in Table 2.
Feature Selection, Performance of Clinical Model, and Radiomics Model
Thirty features were obtained in the feature extraction of radiomics, and in order to avoid overfitting of the model. We used RFE algorithm to achieve further feature extraction, and to make the selection of feature number more reasonable, AUC, accuracies, sensitivity and specificity were selected as references. The results of the AUC in Figure 4 showed that the features are more suitable at 14, and the combination performs more compared to other combinations of features between 10 and 15, and other groups of feature combinations are shown in the Supplementary S2. Among these extracted features mainly include 2 exp features and 8 wave features, and the details are in Table 3.
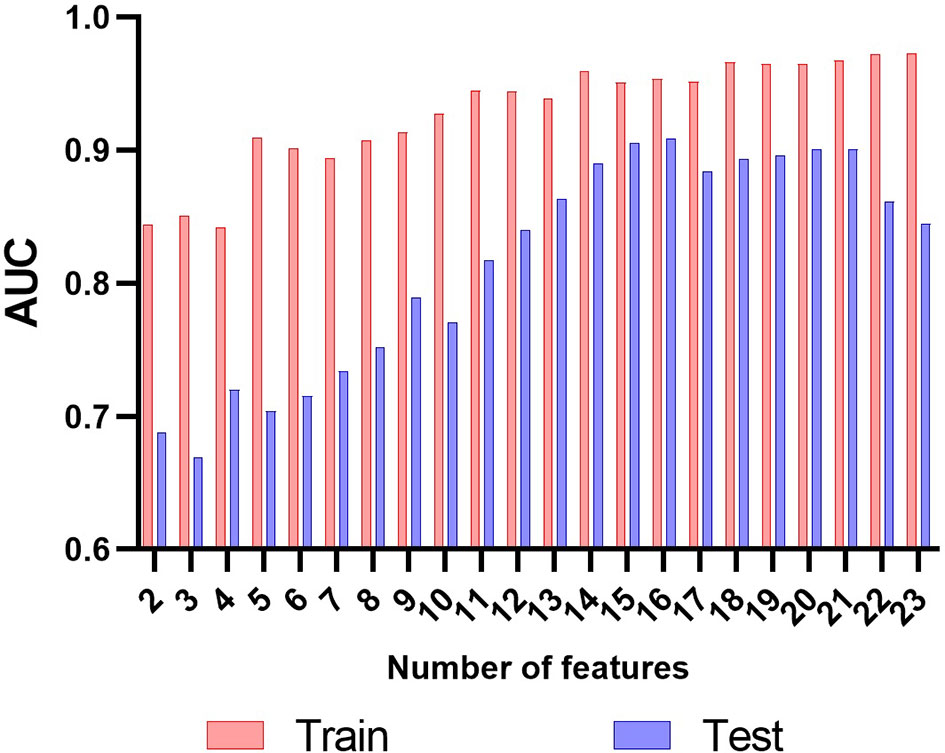
Figure 4 The results of the AUC in radiomics feature selection. The results of the AUC showed that the features at 14 got a good performance both in the training cohort and in the testing cohort.
Clinical model that combine stromal invasion depth, FIGO stage, and MTD exhibit unsatisfying performance in the training cohort (AUC=0.786, accuracy=70.5%, sensitivity=41.7%, and specificity=94.7%) and the testing cohort (AUC=0.706, accuracy=71.3%, sensitivity=43.4%, and specificity=95.0%). The radiomics model performed much better than the clinical model in both training (AUC=0.925, accuracy=87.5%, sensitivity=83.6%, and specificity=90.7%) and the testing cohort (AUC=0.911, accuracy=84.0%, sensitivity=81.1%, and specificity=86.4%)(Table 4). The ROC curves were shown in Figure 5.
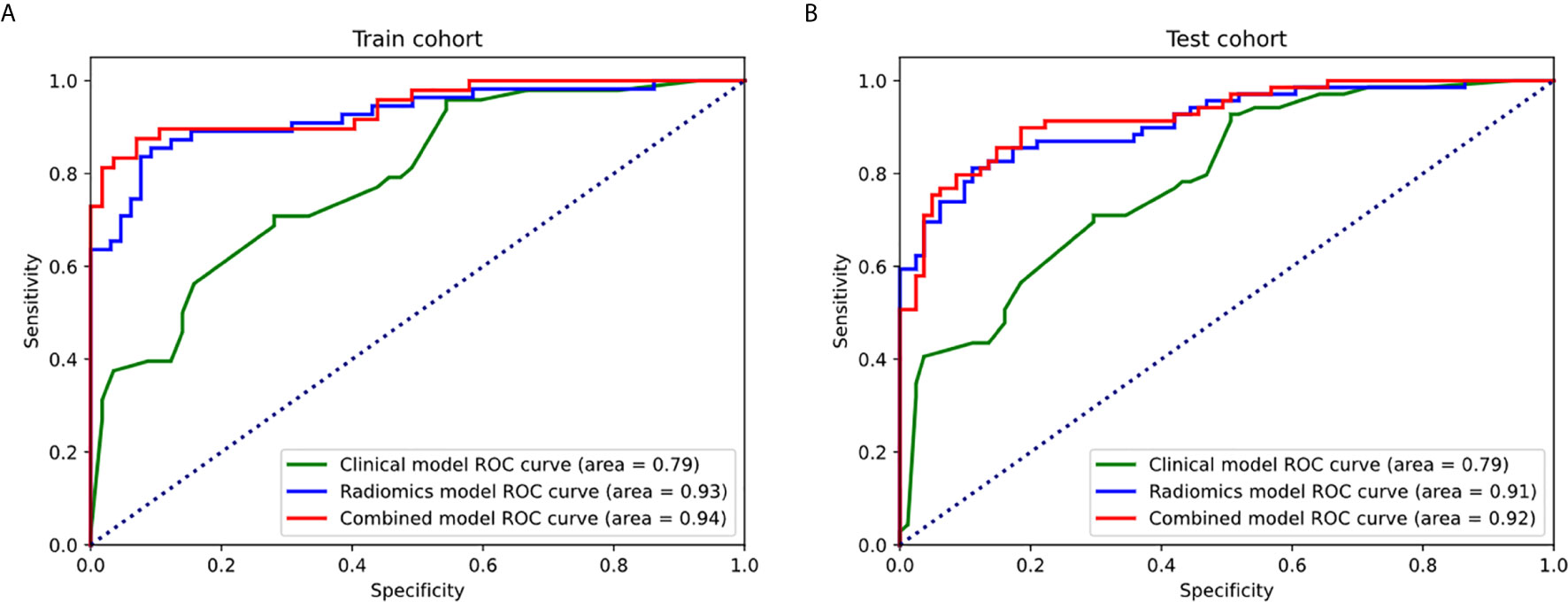
Figure 5 The ROC curves of the clinical model, radiomics models, and combined model in the training cohort (A) and testing cohort (B).
Performance of the Radiomics Nomogram
During the development of combined model, tumor stage, tumor infiltration depth and radiomics signature were selected. Compared with the radiomics model and clinical model, the combined model reached the highest AUC in the training cohort (AUC=0.943, accuracy=89.5%, sensitivity=85.4%, and specificity=92.9%) and the testing cohort (AUC=0.923, accuracy=84.6%, sensitivity=84.0%, and specificity=85.1%)(Table 4 and Figure 5). The DeLong tests revealed a significant difference between the clinical model and combined model in the training and validation cohorts (p = 0.012 and 0.038). The radiomics-based nomogram for visualization of the combined model is shown in Figure 6. The calibration curves of the radiomics-based nomogram demonstrated satisfactory agreement between the predictive and observational possibility of the LVSI status in both the training and validation cohorts.
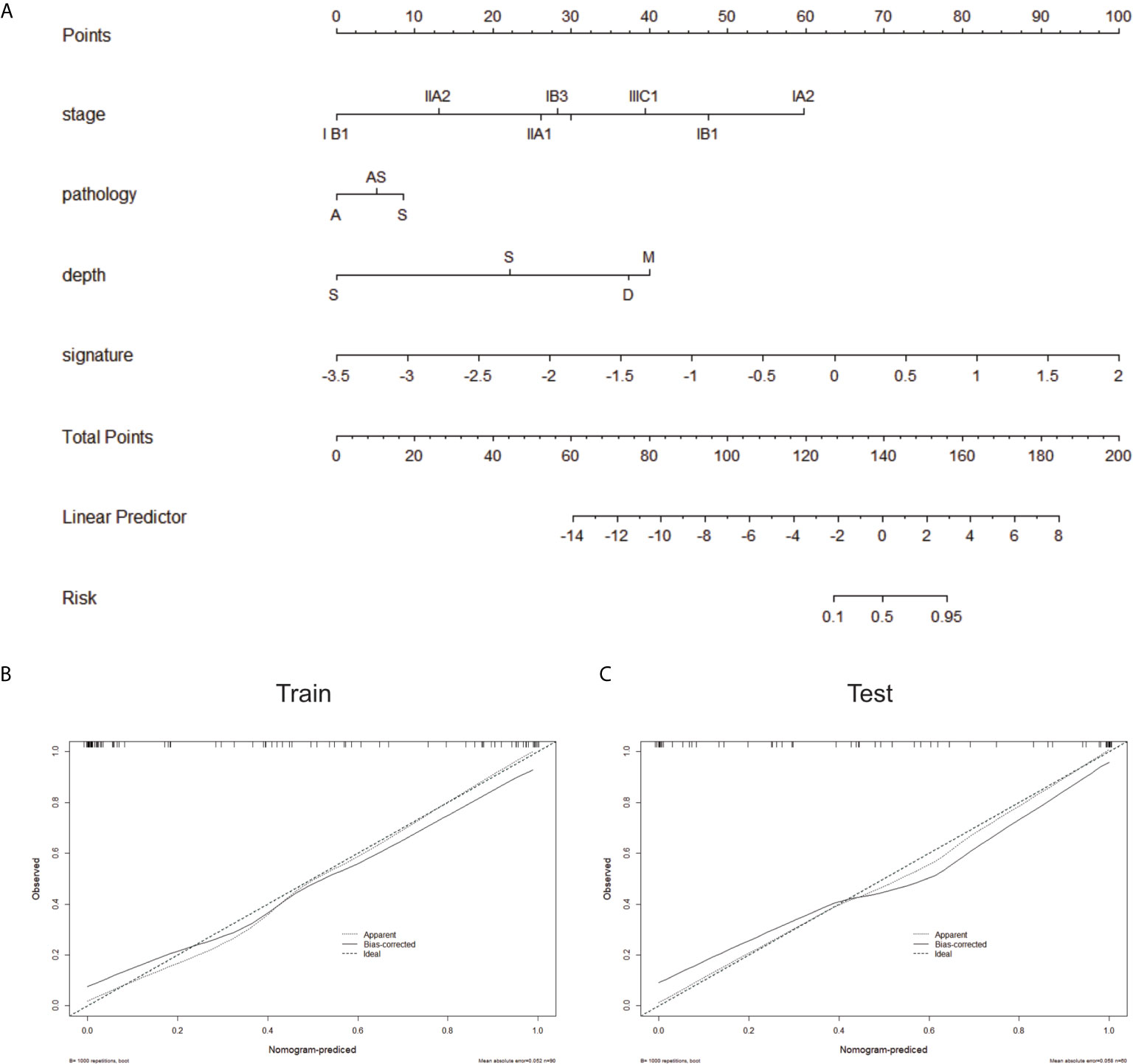
Figure 6 (A) The radiomics-based nomogram. Calibration curves in the training cohort (B) and validation cohort (C). Closer fit to the diagonal line indicates a better evaluation.
Discussion
Adverse pathologic factors are categorized into high-risk, intermediate-risk, or low-risk. High-risk factors include positive surgical margins, lymph node metastases, and parametrial spread. Patients with any high-risk factor need PORT with chemotherapy (GOG 109 trial) (21). Intermediate-risk factors include tumor size more than 4 cm, lymphovascular invasion, and deep stromal invasion. Patients with any two of three intermediate-risk factors require PORT (22, 23). Many attempts have been made to evaluate adverse pathologic factors at primary staging. MRI and ultrasound can accurately evaluate deep stromal invasion, parametrial spread, and tumor size (24–28). For the detection of pelvic lymph node metastases, PET/CT, PET/MRI, and MRI with USPIO have a better diagnostic performance than conventional MRI, CT, and US, providing noninvasive imaging methods particularly in patients at high risk of metastatic disease (29–31).
As one of the intermediate-risk factors, the presence of LVSI guides treatment selection. For patients with negative margins after cone biopsy and no findings of LVSI, observation may be an option if fertility preservation is desired. For patients with stage IA1 disease with LVSI, conization (with negative margins) plus laparoscopic pelvic SLN mapping/lymphadenectomy is a reasonable strategy (32–35). For early-stage patients receiving radical hysterectomy, both the NCCN guidelines and the 2018 FIGO report recommend that patients need PORT with chemotherapy in the presence of positive lymph nodes and/or surgical margins and/or parametrial involvement (5, 6). NCCN guidelines also recommend that pelvic radiation ± chemotherapy should be taken in nodes-negative cases, when “Sedlis Criteria” was met. The “Sedlis Criteria,” which are intermediate-risk factors used to guide adjuvant treatment decisions, include 1) greater than one-third stromal invasion; 2) capillary lymphatic space involvement; or 3) cervical tumor diameters more than 4 cm (Table 2). The reported rate of adjuvant RT following radical hysterectomy and pelvic lymphadenectomy is 10% to 64% (2, 8, 36–40). Thus, a significant proportion of patients undergo treatment by both modalities with potentially compounding morbidities.
Although LVSI status is important in treatment plan guiding, the status of LVSI lacks effective prediction before an operation. While other risk factors have relatively predictive accuracy, which makes LVSI the bottleneck in optimizing treatment. Transvaginal or transrectal ultrasound (TRUS/TVUS) and conventional MRI have a high diagnostic accuracy in tumor size and deep stromal invasion, with reported accuracy 95% and 93%, 93% and 88%, respectively (27–29, 41–43). TRUS/TVUS and MRI with DWI have shown good diagnostic performance in a parametrial invasion, with a reported accuracy of 87%-99% and 78%-99%, respectively (44–46). PET/MRI and radiomics based on conventional MRI yielded high diagnostic accuracy in lymph node metastases (47–49). Preoperative imaging had a relatively high diagnostic efficacy in other risk factors described above, however, few studies concentrated on the preoperative prediction of LVSI with diagnostic imaging. In the retrospective research by Chen et al., it was shown that Gross tumor volume and the maximum diameter of resettable cervical cancer at MRI demonstrated capability in predicting LVSI, with an AUC of 0.700 (50). Yang et al. reported that the Mini-ADC value appears to be a simple and effective tool for the prediction of LVI status in invasive CC with an AUC of 0.885 (51). To enhance the diagnostic performance, radiomics was utilized in the prediction of LVSI. Li et al. found that the radiomics showed discrimination between LVSI and non-LVSI groups. The AUC was 0.710 in the training cohort and 0.633 in the validation cohort (52). In Wu et al.’s research, radiomics analysis of multiparametric MRI evaluates the presence of LVSI. The area under AUC of anatomical, diffusion, and permeability parameters in discriminating the presence of LVSI ranged from 0.659 to 0.814 (53). Unsatisfactory diagnostic accuracy was reported according to these previous studies. According to “Sedlis criteria”, patients were recommended to PORT when two of three immediate-risk factors exist. When the tumor size and depth of stromal invasion could be evaluated with high accuracy, the accurately assessment of LVSI seems more urgent.
Medical imaging acts as a non-invasive phenotypic feature, and the radiographic features of it are extracted quantitatively, which may feedback into the heterogeneity between samples. In this study, the acquired 4 LBP and 18 wavelet features were included in the radiomics features of T2. The wavelet transform, as a filtering algorithm, can perform local amplification of spatial information by highlighting certain information features through the transform. Therefore, certain characteristics of the tumor can be amplified, for example, the tumor’s partial state information and the degree of gray level dependence can be further highlighted. Furthermore, the LBP operator, as an algorithm for describing the local texture features of an image, can not only perform the task of special diagnosis extraction but also highlight the texture features of the image. Besides, some of the remaining features are also done to some extent to highlight the image texture features. For example, logarithm_firstorder_Kurtosis by measuring the kurtosis of the median value distribution of the image ROI, a low kurtosis can make the texture sharper. Wang et al. found that a non-Gaussian-based diffusion-weighted model helps to initially differentiate pelvic cancer grade as well as stage by kurtosis. And Tapera et al. found that pelvic cancer spherical again presented significant in Bartlett’s sphericity tests, p<0.001. With the quantitative radiomics analysis, it is possible to use high-dimensional image information as the basis of disease diagnosis. MRI-based radiomics showed high AUCs in differentiating LVSI (+) from LVSI (-) in the training cohort and validation cohort. Our results suggest that the radiomics analysis of a T2WI map could identify the status of LVSI in cervical cancer with sensitivity and specificity of 0.875 and 0.836 in the training cohort, of 0.811 and 0.864 in the testing cohort, respectively. The AUC of the combined model was 0.943 in the training cohort and 0.922 in the testing cohort, which is the best in all published researches of LVSI in cervical cancer. Combined with the precise prediction of LVSI, tailored treatment could be received by early-stage cervical cancer patients.
There are some limitations to our study. This study was a single-center retrospective study. The retrospective nature of this study may be a reason for potential bias. Secondly, although the number of enrolled patients in this study is the highest in published articles on radiomics-analysis of LVSI on cervical cancer, the available patient number was still low. Results from our database should be supplemented with further prospective and external validation by a larger sample size.
In conclusion, the radiomics-based combined model showed a good performance for predicting LVSI of cervical cancer. The radiomics-based nomogram represents an effective tool for the preoperative individualized prediction of LVSI. This would aid the selection of the optimal therapeutic strategy and clinical decision-making for individuals.
Data Availability Statement
The original contributions presented in the study are included in the article/Supplementary Material. Further inquiries can be directed to the corresponding author.
Ethics Statement
The studies involving human participants were reviewed and approved by Ethics Committee of West China Hospital, Sichuan University. The patients/participants provided their written informed consent to participate in this study.
Author Contributions
WD and ZL designed the methods. XuX and YW collected the data and segmented images. WD, YW and ZL analysed the data. WD and DL wrote and finalized the paper.
Conflict of Interest
The authors declare that the research was conducted in the absence of any commercial or financial relationships that could be construed as a potential conflict of interest.
Acknowledgments
The authors thank Dr. Jiayun Yu for helpful discussions.
Supplementary Material
The Supplementary Material for this article can be found online at: https://www.frontiersin.org/articles/10.3389/fonc.2021.637794/full#supplementary-material
References
1. Bray F, Ferlay J, Soerjomataram I, Siegel R, Torre L. Global Cancer Statistics 2018: GLOBOCAN Estimates of Incidence and Mortality Worldwide for 36 Cancers in 185 Countries. CA A Cancer J Clin (2018) 68:394–424. doi: 10.3322/caac.21492
2. Landoni F, Maneo A, Colombo A, Placa F, Mangioni C. Randomised Study of Radical Surgery Versus Radiotherapy for Stage Ib-IIa Cervical Cancer. Lancet (1997) 350:535–40. doi: 10.1016/S0140-6736(97)02250-2
3. Piver MS, Marchetti DL, Patton T, Halpern J, Blumenson L, Driscoll DL. Radical Hysterectomy and Pelvic Lymphadenectomy Versus Radiation Therapy for Small (Less Than or Equal to 3 Cm) Stage IB Cervical Carcinoma. Am J Clin Oncol (1988) 11:21–4. doi: 10.1097/00000421-198802000-00006
4. Hopkins MP, Morley GW. Radical Hysterectomy Versus Radiation Therapy for Stage IB Squamous Cell Cancer of the Cervix. Cancer (1991) 68:272–7. doi: 10.1002/1097-0142(19910715)68:2<272::AID-CNCR2820680210>3.0.CO;2-X
7. Barter JF, Seng JS, Shingleton HM, Hatch KD, Orr JW. Complications of Combined Radical Hysterectomy—Postoperative Radiation Therapy in Women With Early Stage Cervical Cancer. Gynecol Oncol (1989) 32:292–6. doi: 10.1016/0090-8258(89)90627-6
8. Ayhan A, Al R, Baykal C, Demirtas E, Yüce K. Prognostic Factors in FIGO Stage IB Cervical Cancer Without Lymph Node Metastasis and the Role of Adjuvant Radiotherapy After Radical Hysterectomy. Int J Gynecol Cancer 14 (2004) 14:286–92. doi: 10.1111/j.1048-891X.2004.014212.x
9. Kikuchi E, Margulis V, Karakiewicz PI, Roscigno M, Mikami S, Lotan Y, et al. Lymphovascular Invasion Predicts Clinical Outcomes in Patients With Node-Negative Upper Tract Urothelial Carcinoma. J Clin oncol: Off J Am Soc Clin Oncol (2009) 27:612–8. doi: 10.1200/JCO.2008.17.2361
10. Parra-Herran C, Taljaard M, Djordjevic B, Reyes MC, Schwartz L, Schoolmeester JK, et al. Pattern-Based Classification of Invasive Endocervical Adenocarcinoma, Depth of Invasion Measurement and Distinction From Adenocarcinoma in Situ: Interobserver Variation Among Gynecologic Pathologists. Modern pathol: an Off J United States Can Acad Pathol Inc (2016) 29:879–92. doi: 10.1038/modpathol.2016.86
11. Zhang Q, Ouyang H, Ye F, Chen S, Xie L, Zhao X, et al. Multiple Mathematical Models of Diffusion-Weighted Imaging for Endometrial Cancer Characterization: Correlation With Prognosis-Related Risk Factors. Eur J Radiol (2020) 130:109102. doi: 10.1016/j.ejrad.2020.109102
12. Li S, Liu J, Zhang F, Yang M, Zhang Z, Liu J, et al. Novel T2 Mapping for Evaluating Cervical Cancer Features by Providing Quantitative T2 Maps and Synthetic Morphologic Images: A Preliminary Stud. J Magnetic Resonance Imaging: JMRI (2020) 2:1636–42. doi: 10.1002/jmri.27297
13. Gillies RJ, Kinahan PE, Hricak H. Radiomics: Images Are More Than Pictures, They Are Dat. Radiology (2016) 278:563–77. doi: 10.1148/radiol.2015151169
14. Aerts HJ, Velazquez ER, Leijenaar RT, Parmar C, Grossmann P, Carvalho S, et al. Decoding Tumour Phenotype by Noninvasive Imaging Using a Quantitative Radiomics Approach. Nat Commun (2014) 5:1–9. doi: 10.1038/ncomms5644
15. Aerts, Hugo JWL. The Potential of Radiomic-Based Phenotyping in Precision Medicine: A Revie. JAMA Oncol (2016) 2:1636–42. doi: 10.1001/jamaoncol.2016.2631
16. Song L, Yin J. Application of Texture Analysis Based on Sagittal Fat-Suppression and Oblique Axial T2-Weighted Magnetic Resonance Imaging to Identify Lymph Node Invasion Status of Rectal Cance. Front Oncol (2020) 10:1364. doi: 10.3389/fonc.2020.01364
17. Zhou X, Yi Y, Liu Z, Zhou Z, Tian J. Radiomics-Based Preoperative Prediction of Lymph Node Status Following Neoadjuvant Therapy in Locally Advanced Rectal Cance. Front Oncol (2020) 10:604. doi: 10.3389/fonc.2020.00604
18. Yang YS, Feng F, Qiu YJ, Zheng GH, Wang YT. High-Resolution MRI-Based Radiomics Analysis to Predict Lymph Node Metastasis and Tumor Deposits Respectively in Rectal Cancer. Abdominal Radiol (2020) 46:873–84. doi: 10.1007/s00261-020-02733-x
19. Cui X, Wang N, Zhao Y, Chen S, Li S, Xu M, et al. Preoperative Prediction of Axillary Lymph Node Metastasis in Breast Cancer Using Radiomics Features of DCE-MR. Sci Rep (2019) 9:1–8. doi: 10.1038/s41598-019-38502-0
20. Liu C, Ding J, Spuhler K, Gao Y, Serrano M, Moriatry M. Preoperative Prediction of Sentinel Lymph Node Metastasis in Breast Cancer by Radiomic Signatures From Dynamic Contrast-Enhanced MRI. J Magnetic Resonance Imaging Jmri (2018) 49:131–40. doi: 10.1002/jmri.26224
21. Peters WA III, Liu P, Barrett RJ, Stock RJ, Monk BJ, Berek JS, et al. Concurrent Chemotherapy and Pelvic Radiation Therapy Compared With Pelvic Radiation Therapy Alone as Adjuvant Therapy After Radical Surgery in High-Risk Early-Stage Cancer of the Cervix. Obstetrical Gynecol Survey (2000) 55:491–2. doi: 10.1097/00006254-200008000-00017
22. Rotman M, Sedlis A, Piedmonte MR, Bundy B, Lentz SS, Muderspach LI, et al. A Phase III Randomized Trial of Postoperative Pelvic Irradiation in Stage IB Cervical Carcinoma With Poor Prognostic Features: Follow-Up of a Gynecologic Oncology Group Study. Int J Radiat Oncol Biol Phys (2006) 65:169–76. doi: 10.1016/j.ijrobp.2005.10.019
23. Arlan F, Fuller Jr., Elliott N, Kosloff C. Determinants of Increased Risk for Recurrence in Patients Undergoing Radical Hysterectomy for Stage IB and IIA Carcinoma of the Cervix. Gynecol Oncol (1989) 33:34–9. doi: 10.1016/0020-7292(89)90423-2
24. Rizzo S, Calareso G, Maccagnoni S, Angileri SA, Landoni F, Raimondi S, et al. Pre-Operative MR Evaluation of Features That Indicate the Need of Adjuvant Therapies in Early Stage Cervical Cancer Patients. A single-centre experience Eur J Radiol (2014) 83:858–64. doi: 10.1016/j.ejrad.2014.01.029
25. Testa AC, Ludovisi M, Manfredi R, Zannoni G, Gui B, Basso D, et al. Transvaginal Ultrasonography and Magnetic Resonance Imaging for Assessment of Presence, Size and Extent of Invasive Cervical Cancer. Ultrasound Obstetrics Gynecol (2009) 34:335–44. doi: 10.1002/uog.7325
26. Rauch GM, Kaur H, Choi H, Ernst RD, Klopp AH, Boonsirikamchai P, et al. Optimization of MR Imaging for Pretreatment Evaluation of Patients With Endometrial and Cervical Cancer. Radiographics (2014) 34:1082–98. doi: 10.1148/rg.344140001
27. Pálsdóttir K, Fischerova D, Franchi D, Testa A, Di Legge A, Epstein E. Preoperative Prediction of Lymph Node Metastasis and Deep Stromal Invasion in Women With Invasive Cervical Cancer: Prospective Multicenter Study Using 2D and 3D Ultrasound. Ultrasound Obstetrics Gynecol (2015) 45:470–5. doi: 10.1002/uog.14643
28. Epstein E, Testa A, Gaurilcikas A, Di Legge A, Ameye L, Atstupenaite V, et al. Early-Stage Cervical Cancer: Tumor Delineation by Magnetic Resonance Imaging and Ultrasound—a European Multicenter Trial. Gynecol Oncol (2013) 128:449–53. doi: 10.1016/j.ygyno.2012.09.025
29. Lee SI, Catalano OA, Dehdashti F. Evaluation of Gynecologic Cancer With MR Imaging, 18F-FDG PET/CT, and PET/MR Imaging. J Nucl Med (2015) 56:436–43. doi: 10.2967/jnumed.114.145011
30. Papadia A, Gasparri ML, Genoud S, Bernd K, Mueller MD. The Combination of Preoperative PET/CT and Sentinel Lymph Node Biopsy in the Surgical Management of Early-Stage Cervical Cancer. J Cancer Res Clin Oncol (2017) 143:2275–81. doi: 10.1007/s00432-017-2467-6
31. Rockall AG. Diagnostic Performance of Nanoparticle-Enhanced Magnetic Resonance Imaging in the Diagnosis of Lymph Node Metastases in Patients With Endometrial and Cervical Cancer. J Clin Oncol (2005) 23:2813–21. doi: 10.1200/JCO.2005.07.166
32. Yoneda JY, Braganca JF, Sarian LO, Borba PP, Zeferino LC. Surgical Treatment of Microinvasive Cervical Cancer: Analysis of Pathologic Features With Implications on Radicalit. Int J Gynecol Cancer (2015) 25:694. doi: 10.1097/IGC.0000000000000416
33. Tierney KE, Lin PS, Amezcua C, Matsuo K, Ye W, Felix JC, et al. Cervical Conization of Adenocarcinoma in Situ: A Predicting Model of Residual Disease. Am J Obstetrics Gynecol (2014) 210:366.e361–366.e365. doi: 10.1016/j.ajog.2013.12.030
34. Plante M, Renaud MC, Hoskins IA, Roy M. Vaginal Radical Trachelectomy: A Valuable Fertility-Preserving Option in the Management of Early-Stage Cervical Cancer. A Series of 50 Pregnancies and Review of the Literature. Gynecol Oncol (2005) 98:3–10. doi: 10.1016/j.ygyno.2005.04.014
35. Marchiole P, Benchaib M, Buenerd A, Lazlo E, Dargent D, Mathevet P. Oncological Safety of Laparoscopic-Assisted Vaginal Radical Trachelectomy (LARVT or Dargent’s Operation): A Comparative Study With Laparoscopic-Assisted Vaginal Radical Hysterectomy (LARV). Gynecol Oncol (2007) 106:132–41. doi: 10.1016/j.ygyno.2007.03.009
36. Chang SJ, Kim WY, Yoo SC, Yoon JH, Chun M, Chang KH, et al. A Validation Study of New Risk Grouping Criteria for Postoperative Treatment in Stage IB Cervical Cancers Without High-Risk Factors: Rethinking the Gynecologic Oncology Group Criteria. Eur J Obstetrics Gynecol Reprod Biol (2009) 147:91–6. doi: 10.1016/j.ejogrb.2009.07.013
37. Sartori E, Tisi G, Chiudinelli F, Face BL, Franzini R, Pecorelli S. Early Stage Cervical Cancer: Adjuvant Treatment in Negative Lymph Node Cases. Gynecol Oncol (2007) 107:S170–4. doi: 10.1016/j.ygyno.2007.07.026
38. Schorge JO, Molpus KL, Koelliker D, Nikrui N, Goodman A, Fuller AF, et al. And IIA Cervical Cancer With Negative Lymph Nodes: The Role of Adjuvant Radiotherapy After Radical Hysterectomy. Gynecol Oncol (1997) 66:31–5. doi: 10.1006/gyno.1997.4691
39. Ho CM, Chien TY, Huang SH, Wu CJ, Shih BY, Chang SC. Multivariate Analysis of the Prognostic Factors and Outcomes in Early Cervical Cancer Patients Undergoing Radical Hysterectomy. Gynecol Oncol (2004) 93:458–64. doi: 10.1016/j.ygyno.2004.01.026
40. Marnitz S, Khler C, Affonso RJ, Schneider A, Chiantera V, Tsounoda A, et al. Validity of Laparoscopic Staging to Avoid Adjuvant Chemoradiation Following Radical Surgery in Patients With Early Cervical Cancer. Oncology (2012) 83:346–53. doi: 10.1159/000341659
41. Fischerova D, Cibula D, Stenhova H, Vondrichova H, Belacek J. Transrectal Ultrasound and Magnetic Resonance Imaging in Staging of Early Cervical Cancer. Int J Gynecol Cancer (2010) 18:766–72. doi: 10.1111/j.1525-1438.2007.01072.x
42. Choi HJ, Ju W, Myung SK, Kim Y. Diagnostic Performance of Computer Tomography, Magnetic Resonance Imaging, and Positron Emission Tomography or Positron Emission Tomography/Computer Tomography for Detection of Metastatic Lymph Nodes in Patients With Cervical Cancer: Meta-Analysis. Cancer Sci (2010) 101:1471–9. doi: 10.1111/j.1349-7006.2010.01532.x
43. Canaz E, Ozyurek ES, Erdem B, Talmac MA, Ozaydin IY, Akbayir O, et al. Preoperatively Assessable Clinical and Pathological Risk Factors for Parametrial Involvement in Surgically Treated FIGO Stage IB–IIA Cervical Cancer. Int J Gynecol Cancer (2017) 27:1722–8. doi: 10.1097/IGC.0000000000001060
44. Park JJ, Kim CK, Park SY, Park BK, Kim B. Value of Diffusion-Weighted Imaging in Predicting Parametrial Invasion in Stage IA2–IIA Cervical Cancer. Eur Radiol (2014) 24:1081–8. doi: 10.1007/s00330-014-3109-x
45. Qu J-R, Qin L, Li X, Luo J-P, Li J, Zhang H-K, et al. Predicting Parametrial Invasion in Cervical Carcinoma (Stages IB1, IB2, and IIA): Diagnostic Accuracy of T2-Weighted Imaging Combined With DWI at 3 T. Am J Roentgenol (2018) 210:677–84. doi: 10.2214/AJR.17.18104
46. Shen G, Zhou H, Jia Z, Deng H. Diagnostic Performance of Diffusion-Weighted MRI for Detection of Pelvic Metastatic Lymph Nodes in Patients With Cervical Cancer: A Systematic Review and Meta-Analysis. Br J Radiol (2015) 88:20150063. doi: 10.1259/bjr.20150063
47. Sarabhai T, Schaarschmidt BM, Wetter A, Kirchner J, Aktas B, Forsting M, et al. Comparison of 18 F-FDG PET/MRI and MRI for Pre-Therapeutic Tumor Staging of Patients With Primary Cancer of the Uterine Cervix. Eur J Nucl Med Mol Imaging (2018) 45:67–76. doi: 10.1007/s00259-017-3809-y
48. Yu YY, Zhang R, Dong RT, Hu QY, Yu T, Liu F, et al. Feasibility of an ADC-Based Radiomics Model for Predicting Pelvic Lymph Node Metastases in Patients With Stage IB–IIA Cervical Squamous Cell Carcinoma. Br J Radiol (2019) 92:20180986. doi: 10.1259/bjr.20180986
49. Wu Q, Wang S, Chen X, Wang Y, Dong L, Liu Z, et al. Radiomics Analysis of Magnetic Resonance Imaging Improves Diagnostic Performance of Lymph Node Metastasis in Patients With Cervical Cancer. Radiother Oncol (2019) 138:141–8. doi: 10.1016/j.radonc.2019.04.035
50. Chen XL, Chen GW, Xu GH, Ren J, Li H. Tumor Size at Magnetic Resonance Imaging Association With Lymph Node Metastasis and Lymphovascular Space Invasion in Resectable Cervical Cancer: A Multicenter Evaluation of Surgical Specimen. Int J Gynecol Cancer (2018) 28:1. doi: 10.1097/IGC.0000000000001327
51. Yang W, Wei Qiang J, Hai P, Chen B. Minimum Apparent Diffusion Coefficient for Predicting Lymphovascular Invasion in Invasive Cervical Cancer. J Magnetic Resonance Imaging (2017) 45:1771–9. doi: 10.1002/jmri.25542
52. Li Z, Li H, Wang S, Dong D, Yin F, Chen A, et al. MR-Based Radiomics Nomogram of Cervical Cancer in Prediction of the Lymph-Vascular Space Invasion Preoperatively. J Magnetic Resonance Imaging (2019) 49:1420–6. doi: 10.1002/jmri.26531
Keywords: LVSI, radiomics, cervical cancer, diagnostic performance, invasion
Citation: Du W, Wang Y, Li D, Xia X, Tan Q, Xiong X and Li Z (2021) Preoperative Prediction of Lymphovascular Space Invasion in Cervical Cancer With Radiomics –Based Nomogram. Front. Oncol. 11:637794. doi: 10.3389/fonc.2021.637794
Received: 08 December 2020; Accepted: 15 June 2021;
Published: 12 July 2021.
Edited by:
Youyong Kong, Southeast University, ChinaCopyright © 2021 Du, Wang, Li, Xia, Tan, Xiong and Li. This is an open-access article distributed under the terms of the Creative Commons Attribution License (CC BY). The use, distribution or reproduction in other forums is permitted, provided the original author(s) and the copyright owner(s) are credited and that the original publication in this journal is cited, in accordance with accepted academic practice. No use, distribution or reproduction is permitted which does not comply with these terms.
*Correspondence: Zhiping Li, lizhiping620312@163.com
†These authors have contributed equally to this work