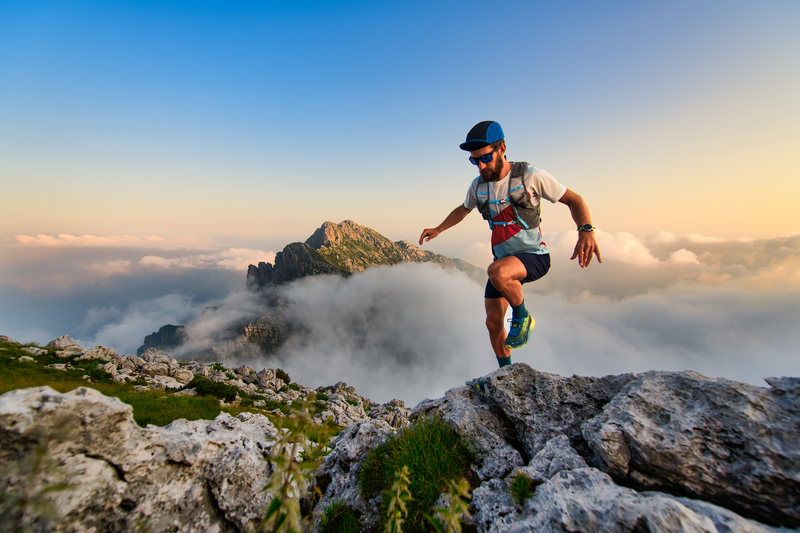
94% of researchers rate our articles as excellent or good
Learn more about the work of our research integrity team to safeguard the quality of each article we publish.
Find out more
ORIGINAL RESEARCH article
Front. Oncol. , 13 May 2021
Sec. Gastrointestinal Cancers
Volume 11 - 2021 | https://doi.org/10.3389/fonc.2021.633995
Objectives: The present study aims to discover the risk factors of multiple metastases and develop a functional nomogram to forecast multiple metastases in metastatic colorectal cancer (mCRC) patients.
Methods: mCRC cases were retrospectively collected from the Surveillance, Epidemiology, and End Results (SEER) database between 2010 and 2016. Survival times between multiple metastases and single metastasis were compared using Kaplan–Meier analysis and log-rank tests. Risk factors for multiple metastases were determined by univariate and multivariate logistic regression analyses, and a nomogram was developed to forecast the probability of multiple metastases in mCRC patients. We assessed the nomogram performance in terms of discrimination and calibration, including concordance index (C-index), area under the curve (AUC), and decision curve analysis (DCA). Bootstrap resampling was used as an internal verification method, and at the same time we select external data from Renmin Hospital of Wuhan University as independent validation sets.
Results: A total of 5,302 cases were included in this study as training group, while 120 cases were as validation group. The patients with single metastasis and multiple metastases were 3,531 and 1,771, respectively. The median overall survival (OS) and cancer-specific survival (CSS) for patients with multiple metastases or single metastasis were 19 vs. 31 months, and 20 vs. 33 months, respectively. Based on the univariate and multivariate analyses, clinicopathological characteristics were associated with number of metastasis and were used to establish nomograms to predict the risk of multiple metastases. The C-indexes and AUC for the forecast of multiple metastases were 0.715 (95% confidence interval (CI), 0.707–0.723), which showed the nomogram had good discrimination and calibration curves of the nomogram showed no significant bias from the reference line, indicating a good degree of calibration. In the validation group, the AUC was 0.734 (95% CI, 0.653–0.834), and calibration curve also showed no significant bias, indicating the favorable effects of our nomogram.
Conclusions: We developed a new nomogram to predict the risk of multiple metastases. The nomogram shows the good prediction effect and can provide assistance for clinical diagnosis and treatment.
Colorectal cancer (CRC) is the third most commonly diagnosed malignancy and the second leading cause of cancer death, which almost cause 900,000 deaths annually (1, 2). CRC is largely an asymptomatic disease until it reaches an advanced stage; therefore, the majority of CRC patients are diagnosed at advanced stage. Advanced colorectal cancer often metastasizes through the bloodstream, lymphatic system, and intraperitoneal route, which is an important reason for poor prognosis in CRC patients (1, 2). Despite the extremely poor prognosis of mCRC, advances in epidemiological studies of mCRC have been limited.
The most common sites of metastasis are the lymph nodes, liver, and lungs in CRC. Occasionally, some special distant sites such as the bone, ovary, peritoneum, and brain may be involved. Although it is known that CRC patients with distant metastasis have a poor prognosis, there is a lack of further stratified analysis of the prognosis of patients with distant metastasis, such as exploring the difference between patients with single metastasis and multiple metastases to clarify the causality and prognostic value. Understanding the patterns of metastasis, especially multiple metastases in CRC, is vitally crucial to improving diagnosis, treatment, and health education for patients.
The current tumor-node-metastasis (TNM) staging system only evaluated whether a patient has metastasis, but it cannot predict whether the patient will have metastasis. In order to make up for the deficiency of the current TNM staging system, some related biomarkers have been explored, studied, and applied in clinical practice. For example, Mismatch repair (MMR) status or microsatellite instability (MSI) has been commonly recommended as the most used and significant molecular marker in clinical management of CRC patients (3, 4). In addition, the expression status of various genes, such as Serine/threonine-protein kinase B-Raf (BRAF) and V-Ki-ras2 Kirsten rat sarcoma viral oncogene homolog (KRAS), were also found to be closely associated with the metastasis of CRC patients (5). However, genetic testing methods have some limitations, such as only suitable for certain groups of patients and bring about certain economic burden to patients. As a result, a statistical model tool, called a nomogram, which comprehensively incorporates the effects of diverse clinical pathological factors, has become a supplement to the above method. Many nomogram scoring systems related to the CRC had been reported recently. For instance, Li et al. also proposed a nomogram, which combined clinical risk factors with radiological characteristics for the prediction of lymph node metastasis in CRC patients (6). Sun et al. built a nomogram associated preoperative plasma fibrinogen with neutrophil-to-lymphocyte ratio to predict the relapse in rectal cancer patients (7). Wang et al. constructed a competitive risk nomogram to predict the specific risk of death in elderly colorectal cancer patients (8). However, these studies lack a hierarchical analysis of metastasis, especially multiple metastases. Although some studies attempted to develop nomograms to predict metastasis, these nomograms only predicted specific metastasis site such as the liver, lung, bone, lacking a hierarchical analysis of metastasis status to distinguish single metastasis and multiple metastases for CRC (9–12). Therefore, there was almost no research that investigates the potential risk factors of multiple metastases and developed a nomogram to predict the risk of multiple metastases. The main reason why such studies are rarely carried out is the relatively limited data on patients with multiple metastases.
The primary objective of this study was to investigate the potential risk factors of multiple metastases and develop a functional nomogram to predict multiple metastases in mCRC patients by using the SEER database. Furthermore, we also selected patients from Renmin Hospital of Wuhan University as an independent external verification cohort to verify the external applicability of the nomogram.
We designed a retrospective study in a large population of mCRC patients from the SEER database. The SEER program of the United States National Cancer Institute is an authoritative source which collects patient demographic information, cancer diagnostic information, and outcomes from 18 population-based cancer registries that cover approximately 28% of the U.S. population.
We identified an open cohort in which cases are diagnosed with CRC between 2010 and 2016 employing the SEER-Stat software (SEER*Stat 8.3.6.1, http://seer.cancer.gov/seerstat/software/). In this study, the inclusion criteria were: 1) Patients diagnosed with CRC were older than 18 years old between 2010 and 2016; 2) When the patient was diagnosed, there was only one primary tumor and no multiple cancers; 3) The patient’s confirmed evidence was confirmed by the pathologist under the microscope; 4) There is clear information about distant metastasis; 5) Patients with active follow-up for at least 1 month. The exclusion criteria were: 1) Patients with incomplete information about our concerned information, including age, sex, race, marital status, insurance status, T stage, N stage, pathological grade, histological type, tumor size, tumor location, lymph node surgery scope, serum carcinoembryonic antigen (CEA) level, tumor deposits (TDs), perineural invasion (PIN), or regional node examination information; 2) Patients were diagnosed as appendix tumors; 3) Patients’ surgery information was unclear or patients without surgery. A complete flow chart describing the selection process is shown on Figure 1. After submitting a request to the SEER database project and obtaining permission, data freely downloaded from the SEER database did not require patients’ informed consent. In our research, a total of 156,545 cases were obtained from the SEER database. Based on the criteria described above, a total of 5,302 cases were included in the research
Figure 1 The study flow diagram of the selection process. CEA, carcinoembryonic antigen; TDs, Tumor Deposits; PI, Perineural Invasion.
To further validate our nomogram, patients diagnosed as mCRC from Renmin Hospital of Wuhan University between 2017 and 2020 were included as external validation set. The validation group included 120 mCRC patients who were recruited according to the same criteria.
Metastasis is characterized by the spread of cancer cells from the primary organ to other organs or tissues through the bloodstream, lymphatic system, or intraperitoneal planting (2). Outcome variable was metastatic state, which was defined as single and multiple metastases. Single metastasis included one of the liver, lung, distant lymph node, peritoneum, bone, brain, or other organs; multiple metastases contained at least two of the above metastases. In the logistic regression model, the occurrence of multiple metastases was considered an outcome event. In the SEER database, CS Mets DX project code in the Collaborative Stage (CS) project can identify single and multiple metastases. Although CS Mets DX project code was not provided in 2016, more specific metastatic sites were provided, including the liver, lung, brain, bone, distant lymph nodes, and other organs, which could still differentiate single and multiple metastases.
We extracted data for demographic factors and clinicopathological parameters. Demographic factors included age, race, sex, marital status, and insurance status. Age was divided into two parts: below 50 years old and at least 50 years old. Race was classified into white, the black, and others (containing American Indian, Asian, and Pacific Islander). Marital status was categorized into married, never married, and others (including divorced, separated, widowed, and unmarried or domestic partner). Clinicopathological parameters included serum CEA level, tumor location, pathological grade, histological type, T stage, N stage, LODDS, tumor size, PIN, and TDs. Serum CEA level was classified into negative and positive. Tumor location was divided into proximal colon cancer, distal colon cancer, and rectum cancer. The definition of proximal colon cancer and distal colon cancer lesions were consistent with a previous study: proximal colon cancer was defined as location of the tumor, including the cecum, ascending colon, hepatic flexure, and proximal transverse colon, while distal colon included the distal transverse colon splenic flexure, descending colon, and sigmoid colon. Pathological grade was classified into I/II (well differentiated/moderately differentiated) and III/IV (poorly differentiated/undifferentiated). Histological type was divided into adenocarcinoma and non-adenocarcinoma. The Collaborative Stage Site-Specific Factor (CS-SSF) 4 and CS-SSF 8 were used to extract the information of TDs and PIN, respectively. The TDs were defined as the presence of one or more peritumoral nodules in the pericolorectal adipose tissue of the primary carcinoma without histological evidence of residual lymph nodes in the nodules, which may present as discontinuous diffusion, venous infiltration with extravascular diffusion, or complete lymph node replacement (13). TDs and PIN were classified into existing and non-existing. T stage and N stage were restaged according to the 8th edition American Joint Cancer Committee (AJCC) through CS Extension, CS Lymph Nodes, Regional Nodes Positive and Regional Examined. After restaging, T stage was categorized into T1/T2, T3, T4, and N stage was divided into N0, N1, and N2. The log of positive lymph nodes (LODDS) was calculated by using the following formula: log [(0.5 + the amounts of positive LNs)/(0.5 + the amounts of negative LNs)] (14). The LODDS value in our cohort ranged from −2.30 to 1.95. We used X-tile software to obtain the best cut-off values for LODDS and tumor size. LODDS was grouped into LODDS1 (−2.3 to −0.9), LODDS2 (−0.9 to 0.2), and LODDS3 (0.2–1.95). Tumor size was divided into <5.4 cm, 5.4–6.9 cm, and >6.9 cm. In addition, the selection of variables in the validation group was based on the risk factors involved in the construction of the nomogram.
Univariate and multivariate logistic regression analyses were used to predict the risk factors of multiple metastases and derivate models. Variables with P <0.3 in univariate analysis were incorporated into multivariate analysis to build a full model. Then we use stepwise regression to determine the final model. Basing only on the t-statistics of their estimated coefficients, a model was built by continuously adding or deleting variables. This semi-automated process was called stepwise regression, which could provide more powerful information at fingertips and was especially useful for filtering a large number of potential independent variables and/or for fine-tuning the model by storing or removing variables compared with ordinary multiple regression.
The C-index and AUC were used to evaluate the discrimination which was the ability of the predictive model to distinguish populations who have experienced an event from those who have not. On logistic regression model, the value of AUC is the same as that of the C-index. When the AUC is 1, it means the model has a perfect discrimination, while 0.5 represents a random chance of correctly identifying the events. The C-index and its 95% CI were calculated by logistic regression. The degree of calibration is another measure of performance of a prediction model, which tests the degree of agreement between the predicted results and the actual results. The patients were divided into different score groups, and the actual multiple metastasis rate in each group was calculated and was named as observation rate. The predicted rate of each group was calculated according to the mean predicted rate and the standard deviation (SD). The predictive accuracy and discriminative ability of the nomogram were determined by concordance index (C-index) and calibration curve (15). As for the internal verification method, we take the method of bootstrap resampling. Bootstrap resampling is currently one of the most widely used internal verification methods (16, 17). Our nomogram was internally validated by discrimination and calibration with 1,000 times bootstraps. In addition, a new tool to evaluate the value of nomogram in clinical application, called DCA, was used to evaluate the effects of clinical benefits and visualize such effects in the present study (18). The purpose of DCA is to evaluate an individual’s risk of adverse outcomes and to recommend some intervention or treatment for high-risk individuals. Finally, we used AUC and calibration curve to evaluate utility of our nomogram in the validation set.
R software version 4.0.0 (The R Foundation for Statistical Computing, Vienna, Austria. http://www.r-project.org) was used to run the statistical analysis. The categorical variables were expressed as count (percentage), and chi-square tests were used to compare demographic factors, clinicopathological parameters between the multiple metastases and single metastasis. OS and CSS between the single metastasis and multiple metastases were compared using Kaplan–Meier analysis, and different survival curves were analyzed by log-rank tests. In this study, the R packages including “survival”, “survminer”, “rms”, “MASS”, “pROC”, “Hmisc”, “survivalROC” and “DecisionCurve” were used to draw the Kaplan–Meier curves, build the nomogram, plot the AUC, conduct DCA and calibration curve.
In our research, a total of 156,545 cases were obtained from the SEER database. Based on the criteria described above, a total of 5,302 cases were included in the research. The demographic factors and clinicopathological parameters of patients in the present study are summarized in Table 1. Briefly, 3,531 patients (66.60%) existed single metastasis, and 1,771 patients (33.40%) had multiple metastases. More than half of the patients were age ≥50 (80.52%), white (74.75%), male (50.87%) and married (56.43%), and LODDS1 (59.20%). The vast majority of patients had positive CEA (77.78%), adenocarcinoma (98.08%), well/moderately differentiated (75.14%) or T3 tumors (58.76%). In contrast, most patients did not have TDs (76.95%), PIN (72.37%). Single metastasis and multiple metastasis groups were different in composition, including sex, marital status, year of diagnosis, tumor site, tumor size, PIN, TDs, histological type, grade, T stage, N stage, and LODDS. Compared with development group, the demographic variables in the validation group were similar, and the clinical variables, including marital status, and race, were significantly different. In the validation group, single metastasis and multiple metastases were different in composition, including tumor site, T stage, LODDS, and grade, which was different from the development group. More detailed information was shown in Table 1.
The median OS for patients with single metastasis and multiple metastases was 31 (95%CI, 30–33) months and 19 (95%CI, 18–20) months, respectively. The median CSS for patients with single metastasis and multiple metastases was 33 (95%CI, 31–35)and 20 (95%CI, 18–21) months, respectively. The Kaplan–Meier survival curves and log-rank test revealed a significant OS and CSS advantage for single metastasis (p < 0.001 for log-rank test; Figure 2). The 1-, 3-, and 5-year OS estimates were 79.4% (95%CI: 78.0–80.9%) and 64.1% (95%CI: 61.7–66.6%), 44.1% (95%CI: 42.0–46.3%) in the single metastasis group and 23.9% (95%CI: 21.3–26.9%), 25.3% (95%CI: 22.8–28.1%) and 10.0%(95%CI: 9.5–14.4%) in the multiple metastases group. The 1-, 3-, and 5-year CSS estimates between single metastasis group and multiple metastases group were 81.0% (95%CI: 79.6–82.4%) and 65.2% (95%CI: 62.8–67.7%), 46.5% (95%CI: 44.3–48.7%) and 25.1% (95%CI: 22.4–28.1%), 29.5% (95%CI: 27.0–32.3%) and 12.0% (95%CI: 9.5–16.2%), respectively.
Figure 2 Kaplan–Meier survival curves of survival. (A) Overall survival of single metastases versus multiple metastases. (B) Cancer-specific survival of single metastases versus multiple metastases.
We performed logistic regression analysis to explore the risk factors of multiple metastases. Sex, marital status, tumor site, tumor size, serum CEA level, PIN, TDs, grade, histological type, T stage, N stage, and LODDS were statistically significant using univariate logistic regression analysis (Table 2). In multivariate stepwise logistic regression analysis, we identified that age, grade, tumor size, PIN, serum CEA level, T stage, TDs, tumor site, histological type, and LODDS were determined as independent risk factors of multiple metastases (Table 2). Among them, three indicators including serum positive CEA (OR, 2.18; 95%CI, 1.86–2.57; p < 0.001), T4 stage (OR, 2.61; 95%CI, 1.78–3.94; p < 0.001), non-adenocarcinoma (OR, 2.01; 95%CI, 1.30–3.14; p = 0.002) had the largest impact on multiple metastases (Figure 3).
Table 2 Univariate/Multivariate Logistic regression analyses of risk factors for multiple metastases in colorectal cancer.
Figure 3 Forest plot with odds ratios for multiple metastases. CEA, carcinoembryonic antigen; LODDS, the log of positive lymph nodes; I/II, well/moderately differentiated; III/IV, poorly/undifferentiated.
Based on the multivariable stepwise logistic regression analysis for multiple metastases, all the independent significant risk factors were integrated to build the nomogram for multiple metastases prediction. The predictive nomogram for multiple metastases was illustrated in Figure 4.
Figure 4 The nomogram for predicting the risk of multiple metastases in CRC. CEA, carcinoembryonic antigen; LODDS, the log of positive lymph nodes.
In this study, C-index value and AUC were applied to evaluate the discrimination ability of the nomogram; moreover, C-index value and AUC were adjusted through 1,000 bootstraps as internal validation to ensure that the nomogram had good effect in predicting multiple metastases. The adjusted value of the C-index was 0.715 (95%CI, 0.707–0.723), and as mentioned above, the AUC was the same as the C-index value (Figure 5A). Furthermore, the calibration curves of the nomogram for predicting multiple metastases also used 1,000 bootstraps for internal validation. The adjusted calibration curves showed no significant deviation from the reference line, indicating a good level of confidence (Figure 5B). As an emerging method for evaluating the prediction of model, DCA suffices the practical needs of clinical decision-making by considering the clinical effects of specific models. The DCA curves for the predictive nomogram are presented in Figure 5C. DCA had shown that when the high-risk threshold was 0.3, the application of this nomogram could make nearly 10% of patients get a net benefit without harming other patients, which means that it has good clinical application significance in predicting multiple metastases. Finally, the AUC was 0.734 (95% CI, 0.653–0.834), and calibration curve also showed no significant bias from the reference line in the independent invalidation group, indicating the favorable effects of our nomogram (Figure 6).
Figure 5 AUC values of ROC predicted multiple metastases (A). The calibration curve of predictive nomograms for predicting multiple metastases (B). Decision curve analysis of the predictive nomogram for predicting multiple metastases (C). (panel A) AUC, Area under the curve; 95CI%, 95% confidence interval. (panel B) Apparent, Calibration curve of nomogram without bootstrap adjustment; Bias-corrected, Calibration curve of nomogram with 1,000 bootstrap adjustment; Ideal, Ideal calibration curve; (panel C) Nomogram: The benefit curve represented by the nomogram; All: Net benefit curve when all samples are multiple metastases; None: Net benefit curve when all samples are single metastasis.
Figure 6 AUC values of ROC predicted multiple metastases (A). The calibration curve of predictive nomograms for predicting multiple metastases (B). (panel A) AUC, area under the curve; 95CI%, 95% confidence interval. (panel B) Ideal, Ideal calibration curve; Non-parametric, Actual calibration curve of nomogram.
In this population-based study, we not only identified the risk factors of multiple metastases, but also develop a nomogram to predict multiple metastases in patients with mCRC, which filled the gaps in this field. Our data showed that patients with multiple metastases have a worse prognosis than patients with single metastasis. Further, we identified independent risk factors of multiple metastases, including traditional indicators such as age, grade, tumor size, tumor location, T stage, histological type, and serum CEA level and novel indicators such as PIN, TDs, and LODDS. Subsequently, on the basis of independent risk factors, we established a nomogram for multiple metastasis prediction. The discrimination and calibration of the nomogram were proved, and this nomogram has a good predictive effect on multiple metastases. Moreover, our nomogram has an independent validation group for external verification.
At present, researches are more focused on the effect of specific distant metastatic sites on survival in patients with CRC. Luo et al. found the number of metastatic foci was an independent prognostic factor, and the prognosis of patients with single metastatic foci was better than that of patients with multiple organs involved (19). Although the study also involved the number of metastasis, it focused on specific sites of metastasis, including the liver, lung, bone, and brain and ignored the metastasis of distant lymph nodes and other organs, without strictly distinguishing between single and multiple metastases. Robinson et al. explored the relationship between tumor primary site and metastasis pattern. They found patients with rectal primaries were more inclined to present with synchronous pulmonary metastasis than patients with colon primaries (20). Bingmer et al. explored the association between primary tumor location and overall survival in CRC of liver metastases and found that right colon cancer had significantly worse survival than left colon cancer (21). However, the above-mentioned studies mainly focus on specific single or two metastasis sites, such as liver metastasis and pulmonary metastasis. There is a lack of an overall research of multiple metastases, especially for the risk factors of multiple metastases, even though previous studies have identified multiple metastases as an independent prognostic factor.
Based on multiple metastases as independent prognostic factors, we further explored the independent risk factors of multiple metastases in mCRC, which was ignored in previous studies. We totally identified ten independent risk factors of multiple metastases, including age, grade, tumor size, tumor site, PNI, serum CEA level, histological type, T stage, TDs, and LODDS. Among them, three indicators including positive serum CEA level (HR, 2.18; 95%CI, 1.86–2.57; p < 0.001), T4 stage (HR, 2.61; 95%CI, 1.78–3.94; p < 0.001), existing TDs (HR, 2.24; 95%CI, 1.95–2.58; p < 0.001) had the largest impact on the multiple metastases (Figure 3).
Based on these independent risk factors, we firstly proposed a nomogram to predict multiple metastases. Compared with the other nomograms involving distant metastasis of CRC, our nomogram focused on the prediction of multiple metastases, often neglected in past research. More importantly, our nomogram not only introduced common clinicopathological parameters but also included some novel indicators, including LODDS, TDs and PIN, which were not available in previous relevant nomograms. More details about nomograms’ differences were summarized in Table S2.
LODDS is an emerging indicator that has been evaluated by many scholars as lymph node stage and may take on a better role than the AJCC lymph node staging system version (14). Zhang et al. proved that the new LODDS classification was an independent prognostic factor for CRC patients, which required the calculation of additional risk group stratification through internal and external databases (22). LODDS was identified as an independent risk factor for multiple metastases in our study, which was not involved in previous studies. At present, more and more study focused on tumor deposits. Lord et al. explored the significance of TDs in neoadjuvant therapy for rectal cancer. They found that, similar to untreated patients, the presence of TDS in rectal cancer patients after neoadjuvant therapy was related to disease progression and poor prognosis (23). Pricolo et al. revealed the TDs and PIN were associated with worse survival in stage III colon cancer, and they recommended a combination of TDs and lymph node examination as “N2c” (24). D’Souza et al. found that the presence of tumor deposits on CT was associated with disease recurrence and had the strongest association with poor outcome in sigmoid colon cancer (25). Lino-Silva et al. found tumor deposits were invariably associated with worse prognosis, especially with the increasing rate of distant metastasis (26). Our research found TD was an independent risk factor of multiple metastases in mCRC, which extends the research direction of TDs. Furthermore, the study has found that serum CEA level is a prognostic factor and an ideal biomarker for CRC patients (27). Routine CEA monitoring was made use in postoperative follow-up to monitor recurrence and distant metastasis after CRC resection surgery. As multivariate analysis manifested, mCRC patients with positive serum CEA levels were more inclined to have higher multiple metastatic probabilities. In related studies, histological differentiation had been defined as an important feature in evaluating the advantages of adjuvant chemotherapy (28). The results of this study indicate that when the grade of the tumor shows poorly differentiated/undifferentiated grade, it is more likely to cause multiple metastases. Some scholars suggested that patients with higher T stage suffered from a higher risk of liver metastases (29). The higher T stage was related to deeper infiltration, which might lead to the metastasis of malignant tumor cells to the blood vessels. Multivariate research has revealed that higher T stage was associated with a higher risk of multiple metastases. In parallel results, tumor size was an independent factor for OS in patients with ulcerative infiltrating colorectal adenocarcinoma (30). Previous studies have shown that younger patients were apt to experience a higher risk of lung, liver, and bone metastases (19, 29). Our research extends this conclusion to multiple metastases. In addition, the rates of pulmonary and liver metastasis in left CRC patients were significantly higher than those in right CRC patient, but the prognosis was better than those with right CRC in terms of OS, which was slightly different from our research (29). In our study, right CRC had a highest rate of multiple metastases. In short, our study extended these variables’ application to the risk factors of multiple metastases. Regarding age, we need to emphasize that because there are only 246 patients older than 85 years old, and there was no significant difference in the number of metastases from patients aged 50 to 85 years old, so we included them as a whole into the population older than 50 years old. However, it must be noted that elderly patients, especially those over 85 years old, usually had a poorer basic state of the whole body and were often accompanied by other diseases. This required further detailed research and could also help improve the quality of the model. In addition, previous studies have found that racial differences affect the metastasis of specific sites. Compared with blacks and whites, other races have a relatively lower risk of metastasis (9, 12). However, in our research, we did not find the impact of racial differences on the risk of multiple metastases. Therefore, race is not included in our model, but objectively there is a huge difference in race between the development group and the validation group. Limited to the number of patients of other races, especially the number of Asians especially (only 56 cases) in SEER database, the influence of race on multiple metastases had not been fully explored. Obviously, this would be one of the directions of future research, which could help to further optimize the quality of the model.
We acknowledge that this study had some limitations. First, as a large retrospective study, these findings must take into account the inherent selection bias. The data provided by the SEER database allow researchers to explore associations that are difficult to uncover because of the limited sample size. However, the unobservable confounders limited the interpretation of observational data, even though we tried to reduce the bias with multivariable analysis. Second, there are some conflicting data about metastases information in the SEER program. For example, a patient has both liver and lung metastases, but the patient M stage showed “M1a”, which may affect data collation and result analysis. Third, the number of accessible variables provided by the SEER database was limited. For instance, salvage therapies, clinical treatment response, specific chemotherapeutic agents, and immunotherapy were not included in the data, which may otherwise affect the interpretation of results. In addition, the SEER program lacks several important biomarker expression states, such as MSI, NRAS, KRAS, and BRAF, which were closely associated with metastases in colorectal cancer. Comparing with other nomogram relating to metastasis, the AUC of our nomogram was relatively low, prompting us to further optimize the model parameters. Finally, although we conducted external verification, due to the limitation of the number of cases in the external verification group, there may be unknown deviations.
Despite these limitations, our study further confirmed by stratified analysis that the degree of metastasis was a prognostic factor in mCRC. Patients with multiple metastases had poorer OS and CSS than those with simple metastasis. Furthermore, we investigated independent risk factors for multiple metastases in mCRC and constructed a nomogram based on these independent risk factors to predict multiple metastases, which had been ignored in previous studies. In general, our study focused on the risk factors for multiple metastases and the construction of a nomogram, which filled in the gaps in previous similar studies.
The datasets presented in this study can be found in online repositories. The names of the repository/repositories and accession number(s) can be found in the article/Supplementary Material. The validation set data is available from the corresponding author.
The study was approved by the Ethics Committee of the Renmin hospital of Wuhan University, and the requirement of written informed consent was waived.
TF supervised the project. YHG and TF conceived and designed the original study. YHG and RSX downloaded and analyzed the data of the training set. JR collected data from the external validation set and participated in part of the data analysis and final revision of the manuscript. YHG, RSX and JR contributed to the interpretation of the data. JR and WS were the main drafters of the manuscript, with the participation of YHG and RSX. TF, YHG, JR and WL revised the manuscript. All authors contributed to the article and approved the submitted version.
The authors declare that the research was conducted in the absence of any commercial or financial relationships that could be construed as a potential conflict of interest.
Thanks to all the staff of Renmin Hospital of Wuhan University who contributed to the study.
The Supplementary Material for this article can be found online at: https://www.frontiersin.org/articles/10.3389/fonc.2021.633995/full#supplementary-material
Supplementary Table 1 | Exclusion criteria and reasons that we excluded patients. Abbreviations: CEA, carcinoembryonic antigen; LODDS, the log of positive lymph nodes; AJCC, American Joint Cancer Committee.
Supplementary Table 2 | Comparison of nomograms related to predicting colorectal cancer metastasis by using SEER. Abbreviations: CEA, Carcinoembryonic antigen; LODDS, the log of positive lymph nodes; TDs, Tumor Deposits; PI, Perineural Invasion; LNH, lymph nodes harvested; AUC, Area under the curve.
1. Dekker E, Tanis PJ, Vleugels J, Kasi PM, Wallace MB. Colorectal Cancer. Lancet (2019) 394:1467–80. doi: 10.1016/S0140-6736(19)32319-0
2. Keum N, Giovannucci E. Global Burden of Colorectal Cancer: Emerging Trends, Risk Factors and Prevention Strategies. Nat Rev Gastroenterol Hepatol (2019) 16:713–32. doi: 10.1038/s41575-019-0189-8
3. Yamashita R, Long J, Longacre T, Peng L, Berry G, Martin B, et al. Deep Learning Model for the Prediction of Microsatellite Instability in Colorectal Cancer: A Diagnostic Study. Lancet Oncol (2021) 22:132–41. doi: 10.1016/S1470-2045(20)30535-0
4. Watanabe T, Wu TT, Catalano PJ, Ueki T, Satriano R, Haller DG, et al. Molecular Predictors of Survival After Adjuvant Chemotherapy for Colon Cancer. N Engl J Med (2001) 344:1196–206. doi: 10.1056/NEJM200104193441603
5. Gryfe R, Kim H, Hsieh ET, Aronson MD, Holowaty EJ, Bull SB, et al. Tumor Microsatellite Instability and Clinical Outcome in Young Patients With Colorectal Cancer. N Engl J Med (2000) 342:69–77. doi: 10.1056/NEJM200001133420201
6. Li M, Zhang J, Dan Y, Yao Y, Dai W, Cai G, et al. A Clinical-Radiomics Nomogram for the Preoperative Prediction of Lymph Node Metastasis in Colorectal Cancer. J Transl Med (2020) 18:46. doi: 10.1186/s12967-020-02215-0
7. Sun Y, Zhang Y, Huang Z, Lin H, Lu X, Huang Y, et al. Combination of Preoperative Plasma Fibrinogen and Neutrophil-to-Lymphocyte Ratio (the F-NLR Score) as a Prognostic Marker of Locally Advanced Rectal Cancer Following Preoperative Chemoradiotherapy. World J Surg (2020) 44:1975–84. doi: 10.1007/s00268-020-05407-3
8. Wang Z, Wang Y, Yang Y, Luo Y, Liu J, Xu Y, et al. A Competing-Risk Nomogram to Predict Cause-Specific Death in Elderly Patients With Colorectal Cancer After Surgery (Especially for Colon Cancer). World J Surg Oncol (2020) 18:30. doi: 10.1186/s12957-020-1805-3
9. Mo S, Cai X, Zhou Z, Li Y, Hu X, Ma X, et al. Nomograms for Predicting Specific Distant Metastatic Sites and Overall Survival of Colorectal Cancer Patients: A Large Population-Based Real-World Study. Clin Transl Med (2020) 10:169–81. doi: 10.1002/ctm2.20
10. Fakih MG. Metastatic Colorectal Cancer: Current State and Future Directions. J Clin Oncol (2015) 33:1809–24. doi: 10.1200/JCO.2014.59.7633
11. Han L, Dai W, Mo S, Xiang W, Li Q, Xu Y, et al. Nomogram to Predict the Risk and Survival of Synchronous Bone Metastasis in Colorectal Cancer: A Population-Based Real-World Analysis. Int J Colorectal Dis (2020) 35:1575–85. doi: 10.1007/s00384-020-03612-z
12. Luo T, Wang Y, Shan X, Bai Y, Huang C, Li G, et al. Nomogram Based on Homogeneous and Heterogeneous Associated Factors for Predicting Distant Metastases in Patients With Colorectal Cancer. World J Surg Oncol (2021) 19:30. doi: 10.1186/s12957-021-02140-6
13. Ciardiello F, Tortora G. EGFR Antagonists in Cancer Treatment. N Engl J Med (2008) 358:1160–74. doi: 10.1056/NEJMra0707704
14. Conci S, Ruzzenente A, Sandri M, Bertuzzo F, Campagnaro T, Bagante F, et al. What is the Most Accurate Lymph Node Staging Method for Perihilar Cholangiocarcinoma? Comparison of UICC/AJCC Pn Stage, Number of Metastatic Lymph Nodes, Lymph Node Ratio, and Log Odds of Metastatic Lymph Nodes. Eur J Surg Oncol (2017) 43:743–50. doi: 10.1016/j.ejso.2016.12.007
15. Wang Y, Li J, Xia Y, Gong R, Wang K, Yan Z, et al. Prognostic Nomogram for Intrahepatic Cholangiocarcinoma After Partial Hepatectomy. J Clin Oncol (2013) 31:1188–95. doi: 10.1200/JCO.2012.41.5984
16. Balachandran VP, Gonen M, Smith JJ, DeMatteo RP. Nomograms in Oncology: More Than Meets the Eye. Lancet Oncol (2015) 16:e173–80. doi: 10.1016/S1470-2045(14)71116-7
17. Tang XR, Li YQ, Liang SB, Jiang W, Liu F, Ge WX, et al. Development and Validation of a Gene Expression-Based Signature to Predict Distant Metastasis in Locoregionally Advanced Nasopharyngeal Carcinoma: A Retrospective, Multicentre, Cohort Study. Lancet Oncol (2018) 19:382–93. doi: 10.1016/S1470-2045(18)30080-9
18. Vickers AJ, Elkin EB. Decision Curve Analysis: A Novel Method for Evaluating Prediction Models. Med Decis Making (2006) 26:565–74. doi: 10.1177/0272989X06295361
19. Luo D, Liu Q, Yu W, Ma Y, Zhu J, Lian P, et al. Prognostic Value of Distant Metastasis Sites and Surgery in Stage IV Colorectal Cancer: A Population-Based Study. Int J Colorectal Dis (2018) 33:1241–9. doi: 10.1007/s00384-018-3091-x
20. Robinson JR, Newcomb PA, Hardikar S, Cohen SA, Phipps AI. Stage IV Colorectal Cancer Primary Site and Patterns of Distant Metastasis. Cancer Epidemiol (2017) 48:92–5. doi: 10.1016/j.canep.2017.04.003
21. Bingmer K, Ofshteyn A, Bliggenstorfer JT, Kethman W, Ammori JB, Charles R, et al. Primary Tumor Location Impacts Survival in Colorectal Cancer Patients After Resection of Liver Metastases. J Surg Oncol (2020) 122:745–52. doi: 10.1002/jso.26061
22. Zhang QW, Zhang CH, Pan YB, Biondi A, Fico V, Persiani R, et al. Prognosis of Colorectal Cancer Patients is Associated With the Novel Log Odds of Positive Lymph Nodes Scheme: Derivation and External Validation. J Cancer (2020) 11:1702–11. doi: 10.7150/jca.38180
23. Lord AC, Graham MC, D’Souza N, Pucher PH, Brown G, Nagtegaal ID. The Significance of Tumour Deposits in Rectal Cancer After Neoadjuvant Therapy: A Systematic Review and Meta-Analysis. Eur J Cancer (2019) 122:1–8. doi: 10.1016/j.ejca.2019.08.020
24. Pricolo VE, Steingrimsson J, McDuffie TJ, McHale JM, McMillen B, Shparber M. Tumor Deposits in Stage Iii Colon Cancer: Correlation With Other Histopathologic Variables, Prognostic Value, and Risk Stratification-Time to Consider “N2c”. Am J Clin Oncol (2020) 43:133–8. doi: 10.1097/COC.0000000000000645
25. D’Souza N, Shaw A, Lord A, Balyasnikova S, Abulafi M, Tekkis P, et al. Assessment of a Staging System for Sigmoid Colon Cancer Based on Tumor Deposits and Extramural Venous Invasion on Computed Tomography. JAMA Netw Open (2019) 2:e1916987. doi: 10.1001/jamanetworkopen.2019.16987
26. Lino-Silva LS, Xinaxtle DL, Salcedo-Hernandez RA. Tumor Deposits in Colorectal Cancer: The Need for a New “Pn” Category. Ann Transl Med (2020) 8:733. doi: 10.21037/atm.2020.03.175
27. Shibutani M, Maeda K, Nagahara H, Ohtani H, Sakurai K, Toyokawa T, et al. Significance of CEA and CA19-9 Combination as a Prognostic Indicator and for Recurrence Monitoring in Patients With Stage II Colorectal Cancer. Anticancer Res (2014) 34:3753–8.
28. Barresi V, Reggiani BL, Ieni A, Caruso RA, Tuccari G. Histological Grading in Colorectal Cancer: New Insights and Perspectives. Histol Histopathol (2015) 30:1059–67. doi: 10.14670/HH-11-633
29. Hu X, Marietta A, Dai WX, Li YQ, Ma XJ, Zhang L, et al. Prediction of Hepatic Metastasis and Relapse in Colorectal Cancers Based on Concordance Analyses With Liver Fibrosis Scores. Clin Transl Med (2020) 9:13. doi: 10.1186/s40169-020-0264-3
Keywords: nomogram, multiple metastasis, colorectal cancer, risk factors, surveillance epidemiology and end results
Citation: Ge Y, Xiang R, Ren J, Song W, Lu W and Fu T (2021) A Nomogram for Predicting Multiple Metastases in Metastatic Colorectal Cancer Patients: A Large Population-Based Study. Front. Oncol. 11:633995. doi: 10.3389/fonc.2021.633995
Received: 26 November 2020; Accepted: 15 April 2021;
Published: 13 May 2021.
Edited by:
Shuyun Rao, George Washington University, United StatesReviewed by:
Shaobo Mo, Fudan University, ChinaCopyright © 2021 Ge, Xiang, Ren, Song, Lu and Fu. This is an open-access article distributed under the terms of the Creative Commons Attribution License (CC BY). The use, distribution or reproduction in other forums is permitted, provided the original author(s) and the copyright owner(s) are credited and that the original publication in this journal is cited, in accordance with accepted academic practice. No use, distribution or reproduction is permitted which does not comply with these terms.
*Correspondence: Tao Fu, dGZ1MDAxQHdodS5lZHUuY24=
†These authors have contributed equally to this work
Disclaimer: All claims expressed in this article are solely those of the authors and do not necessarily represent those of their affiliated organizations, or those of the publisher, the editors and the reviewers. Any product that may be evaluated in this article or claim that may be made by its manufacturer is not guaranteed or endorsed by the publisher.
Research integrity at Frontiers
Learn more about the work of our research integrity team to safeguard the quality of each article we publish.