- 1Samsung Genome Institute, Samsung Medical Center, Sungkyunkwan University School of Medicine, Seoul, South Korea
- 2Department of Health Science and Technology, Samsung Advanced Institute for Health Sciences and Technology, Sungkyunkwan University, Seoul, South Korea
- 3Department of Pathology and Translational Genomics, Samsung Medical Center, Sungkyunkwan University School of Medicine, Seoul, South Korea
- 4Division of Hematology and Oncology, Department of Medicine, Samsung Medical Center, Sungkyunkwan University School of Medicine, Seoul, South Korea
- 5Department of Molecular Cell Biology, Sungkyunkwan University School of Medicine, Suwon, South Korea
Primary refractory/relapsed diffuse large B-cell lymphoma (rrDLBCL) is an unresolved issue for DLBCL treatment and new treatments to overcome resistance is required. To explore the genetic mechanisms underlying treatment resistance in rrDLBCL and to identify candidate genes, we performed targeted deep sequencing of 430 lymphoma-related genes from 58 patients diagnosed with rrDLBCL. Genetic alterations found between the initial biopsy and biopsy at recurrence or refractory disease were investigated. The genes most frequently altered (> 20%) were (in decreasing order of frequency) CDKN2A, PIM1, CD79B, TP53, MYD88, MYC, BTG2, BTG1, CDKN2B, DTX1, CD58, ETV6, and IRF4. Genes mutation of which in pretreatment sample were associated with poor overall survival included NOTCH1, FGFR2, BCL7A, BCL10, SPEN and TP53 (P < 0.05). FGFR2, BCL2, BCL6, BCL10, and TP53 were associated with poor progression-free survival (P < 0.05). Most mutations were truncal and were maintained in both the initial biopsy and post-treatment biopsy with high dynamics of subclones. Immune-evasion genes showed increased overall mutation frequency (CD58, B2M) and variant allele fraction (CD58), and decreased copy number (B2M, CD70) at the post-treatment biopsy. Using the established mutational profiles and integrative analysis of mutational evolution, we identified information about candidate genes that may be useful for the development of future treatment strategies.
Introduction
Diffuse large B-cell lymphoma (DLBCL) is a heterogeneous disease comprising distinct types of aggressive B-cell lymphoma with different biology and clinical outcomes. Most of the patients with DLBCL respond well to standard immunochemotherapy, but 10% of patients present with primary refractory disease and an additional 30–40% experience relapse following an initial response to therapy (1, 2). Patients with primary refractory or relapsed disease require a new treatment modality to overcome the resistance to treatment, but this remains an unmet need in the management of patients with DLBCL (2, 3).
DLBCL is divided into two distinct molecular subtypes classified according to the gene expression profile (4). The germinal center B-cell-like (GCB) subtype is characterized by mutation of EZH2, translocation of BCL2, BCL6, or MYC, and activation of the phosphatidylinositol 3 kinase (PI3K)-Akt-mTOR signaling pathway; genes that function in the normal germinal center are expressed by this subtype (5, 6). The activated B-cell-like (ABC) subtype is characterized by the constitutive activation of the NF-κB signaling pathway and mutation in the genes engaging in the B-cell receptor (BCR) signaling and/or toll-like receptor signaling pathways. The ABC subtype involves mutations in TNFAIP3, CARD11, MYD88, BCL10, MALT1, and BCL6, and results in activation of the transcription factor NF-κB (7, 8).
The differences in these intracellular oncogenic signaling pathways have prognostic significance and can be exploited for therapeutic benefit. A recent study showed that these molecular subgroups based on the cell of origin of DLBCL can be further divided into five clusters based on mutations, somatic copy number alterations (SCNAs), and structural variants (SVs) of the genome (9). Each ABC-type and GCB-type DLBCLs could be classified into two subclasses showing different survival outcomes and a class featuring TP53 mutation had a moderate survival outcome. DLBCLs belonging to the ABC-type DLBCL can be classified into two molecular groups. One molecular group is characterized by gain of 18q and overexpression of BCL2, and mutations of CD79B and MYD88 L265P. The other ABC-type molecular group harbors translocation of BCL6 and mutations of genes involved in the NOTCH and NF-κB signaling pathways. GCB-type DLBCL can also be divided into two molecular groups. One molecular group is characterized by translocation and mutation of BCL2 with mutations of the epigenetic regulators IRF8 and TNFRSF14, and the other molecular group has mutations in linker histone genes with mutations of CD58, RHOA, CARD11, BRAF, and STAT.
The number of therapeutic agents targeting genetic variations and related signaling pathways is increasing as the numbers of discovered mutations associated with initiation, transformation, and progression increase. Novel therapeutic agents that target the BCR pathways include dasatinib (Lyn inhibitor), ibrutinib (BTK inhibitor), fostamatinib (SYK inhibitor), or enzastaurin (PKCβ inhibitor) (10–13). Overexpression of myc, through translocation or by other mechanisms can be targeted indirectly by epigenetic manipulation with a BET bromodomain inhibitor. Histone deacetylase inhibitors (e.g. vorinostat) hold promise for lymphomas with CREBBP or EP300 mutations (14).
Because recently developed novel therapies act on tumors through mechanisms involving genetic alterations, it seems worthwhile to study whether specific genetic alterations can predict a poor response to current immunochemotherapy. In this study, we performed targeted deep sequencing of 430 lymphoma-related genes for 58 patients diagnosed with relapsed/refractory DLBCL (rrDLBCL). Various mutations, SCNAs, and SVs were investigated in a sample obtained in the initial biopsy and that obtained at the time of relapse or diagnosis of refractory disease. We also analyzed samples from 15 patients with a good response to conventional immunochemotherapy as a control and compared the data between these controls and those with rrDLBCL.
Materials and Methods
Patient Selection
Patients with rrDLBCL diagnosed between 1 January 2002 and 31 December 2018 in the Samsung Medical Center were enrolled in the study. Primary refractory DLBCL was defined as progression of the disease during initial R-CHOP treatment without a complete remission (CR) or relapse of the disease after a transient CR in < 6 months from the end of the initial therapy. Relapsed DLBCL was defined as DLBCL that reappeared after CR lasting > 6 months. If the DLBCL relapsed > 60 months after the treatment, we called it a late relapse. Sixteen of the 74 rrDLBCL patients were diagnosed with transformed DLBCL or primary CNS DLBCL and were excluded. For comparison, 15 patients with DLBCL who had been cured with a follow-up period of > 6 years were included as controls. All patients were treated with initial R-CHOP immunochemotherapy with or without radiotherapy or stem cell transplantation. This study was approved by institutional review board of Samsung Medical Center (IRB 2013-12-076-005) in accordance with the tenets of the Declaration of Helsinki.
Clinical Data
The revised International Prognostic Index (IPI) score (15) was calculated by counting the number of the following risk factors for each patient: 1) age > 60 years at the time of diagnosis, 2) stage III or IV disease, 3) elevated serum LDH level, 4) Eastern Cooperative Oncology Group performance status > 2, and 5) > 1 extranodal site. An IPI score of 0–2 is defined as low risk and 3–5 is defined as high risk.
Immunohistochemistry
Formalin-fixed, paraffin-embedded specimens were used in the ancillary study. Immunohistochemical staining of 4-μm paraffin sections were performed using a Bond Max automated immunostainer (Leica Biosystems, Melbourne, Australia). Monoclonal antibodies against CD20 (L26, 1/200; Dako, Glostrup, Denmark), CD3 (polyclonal, 1/200; Dako), CD10 (56C6, 1/250; Novocastra, Newcastle upon Tyne, UK), BCL6 (LN22, 1/80; Novocastra), MUM1 (MUM1p, 1/500; Dako), BCL2 (124, 1/100; Dako), and Myc (Y69, cat:ab32072, 1/100; Abcam, Burlingame, CA, USA) were used. The cell of origin (COO) subtype was determined using the Hans algorithm (16).
Fluorescence In Situ Hybridization Analysis of MYC, BCL2, and BCL6 Translocation
Interphase fluorescence in situ hybridization (FISH) analysis was performed for all samples using a Vysis LSI® MYC Dual Color Break Apart Rearrangement Probe (Abbott/Vysis, Des Plains, IL, USA) for detection of MYC rearrangement, a Vysis LSI® BCL2 Dual Color Break Apart Rearrangement Probe (Abbott/Vysis) for detection of BCL2 rearrangement and a Vysis LSI® BCL6 Dual Color Break Apart Rearrangement Probe (Abbott/Vysis) for detection of BCL6 rearrangement. The determined cut-off value for the detection of a rearrangement of MYC, BCL2, and BCL6 was 5%.
Targeted Panel Sequencing
Targeted panel sequencing was performed using a HemaSCAN panel that contained 430 genes related to hematological malignancies. Genomic DNA was extracted using a QIAamp DNA Mini kit (Qiagen, Valencia, CA, USA), according to the manufacturer’s protocol. DNA quality and quantity were analyzed using a Nanodrop 8,000 UV–Vis spectrometer (NanoDrop Technologies, Wilmington, DE, USA), Qubit 2.0 Fluorometer (Life Technologies, Carlsbad, CA, USA), and 2200 TapeStation Instrument (Agilent Technologies, Santa Clara, CA, USA). Genomic DNA was sheared using a Covaris S220 instrument (Covaris, Woburn, MA, USA). Target capture was performed using the SureSelect XT Reagent Kit, HSQ (Agilent Technologies), and a paired-end sequencing library was constructed with a barcode. Sequencing was performed on a HiSeq 2500 with 100-bp reads (Illumina, San Diego, CA, USA). The paired-end reads were aligned to the human reference genome (hg19) using BWA-MEM v0.7.5. Samtools v0.1.18, GATK v3.1-1, and Picard v1.93 were used for BAM file handling, local realignment, and removal of duplicate reads, respectively.
Single nucleotide variants (SNVs) with a variant allele fraction (VAFs) > 1% were detected using MuTect v1.1.4 (17), and Lofreq v0.6.1 (18). Sequencing errors were filtered out by an in-house algorithm using data extracted from each BAM file (19). Small insertions and deletions (indels) < 30 bp in size were detected using Pindel v0.2.5a4 (20). Possible germline polymorphisms were also filtered out if the allele frequency was > 0.1% in any of the normal population databases including the Genome Aggregation Database (21), Korean Reference Genome Database, or Korean Variant Archive (22). SVs and large indels > 30 bp in size were detected using JuLI (23). SCNAs of each gene were also detected using an in-house copy number caller with copy numbers > 6 being marked as amplifications and copy numbers < 0.8 designated as deletions. The tumor mutational burden (TMB) was calculated by counting the number of SNVs and indels, and converting the value to count per megabase pairs.
Statistical Analysis
Statistical analysis was conducted using R-3.6.1. Continuous variables were compared between two groups using Student’s t test, and categorical variables were compared using the chi-square test. Progression-free survival (PFS) was calculated from the date of diagnosis to the date of disease progression or relapse. Overall survival (OS) was calculated from the date of diagnosis to the date of death. Mutational frequency of each gene was compared using logistic regression analysis corrected for COO. Any type of alteration, including SNVs, indels, SCNAs, and SVs, were counted to calculate the mutational frequency. To calculate the hazard ratio (HR) and P value, the Cox proportional-hazards model corrected for COO and IPI score was used. P values were adjusted using the Benjamini and Hochberg method. A false discovery rate (FDR) < 0.1 was considered to be significant.
Pre- and Post-Chemotherapy Paired-Sample Analysis
The VAF values of the SNV/indel and copy number of amplified or deleted genes were used to compare clonal changes in the pre- and post-chemotherapy samples for each patient. The VAF value is not directly comparable because it differs according to the purity. To adjust for differences in VAF between patients, the VAF values of mutations shared between pre- and post-chemotherapy samples were fitted using robust linear regression. Mutations with VAF values between 0.4 and 0.6 in both the pre- and post-therapy samples and > 0.1 apart from the fitted value were considered as germline mutations and excluded. To summarize the differences in VAF values between patients, fitted VAF values were normalized using the following equation to maintain the median VAF value as 0.5:
where V is the fitted VAF value and m is the median of the fitted VAF value.
Only samples with calculated tumor purity >60% were used in the analysis to accurately compare the copy number of genes. For all genes with copy number ≥ 3 or ≤ 1, the changes in copy number between pretreatment and post-treatment samples was analyzed.
Molecular Classification
The data of Chapuy et al. (9) were retrained with a lasso regression model using 77 of 105 features that were available from our panel sequencing and FISH results. Among the 77 features, 71 features had non-zero coefficient. Cluster 0 was not used because it represented extremely low-purity samples and more than one alteration was used for modelling in all of our samples. When tested using the leave-one-out cross-validation, the overall accuracy of the adjusted model was 0.75 compared with the original classification (Supplementary Table 1). When applying this model, copy number changes are aggregated for each chromosomal band using the mean copy number of the included genes. For a mean copy number > 2.2 and > 3.7, amplification scores of 1 and 2 were given, respectively. For a mean copy number < 1.6 and < 1.1, deletion scores of 1 and 2 were given, respectively.
We slightly modified the seed classification of Schmitz et al. (8) and used the presence of the final features of the seed genes to assign class. Those with MYD88 L265P mutation or CD79B SNV/indel or amplification were classified as MCD, those with BCL6 fusion or NOTCH2 mutation or amplification were classified as BN2, those with NOTCH1 mutation were classified as N1, and those with BCL2 fusion or EZH2 mutation were classified as EZB.
Results
Patient Characteristics
Details of the patients and their clinicopathological characteristics are summarized in Supplementary Table 2.
A total of 58 patients were included (Figure 1): 34 patients with refractory DLBCL and 24 patients with relapsed DLBCL. For comparison, we also included the 15 cured patients who exhibited CR and no subsequent relapse. The median follow-up durations were 16.6 months (range, 5.4–62.7) for refractory DLBCL, 62 months (range, 20.4–200.5) for patients with relapsed DLBCL, and 87.5 months (range, 74.9–102) for cured patients. Among the 24 patients with relapsed DLBCL, the median time to relapse was 17.8 months (range, 10.8–56.1) in 17 patients; the other six patients had a very late relapse with a median time to relapse of 107.05 months (range, 97–182).
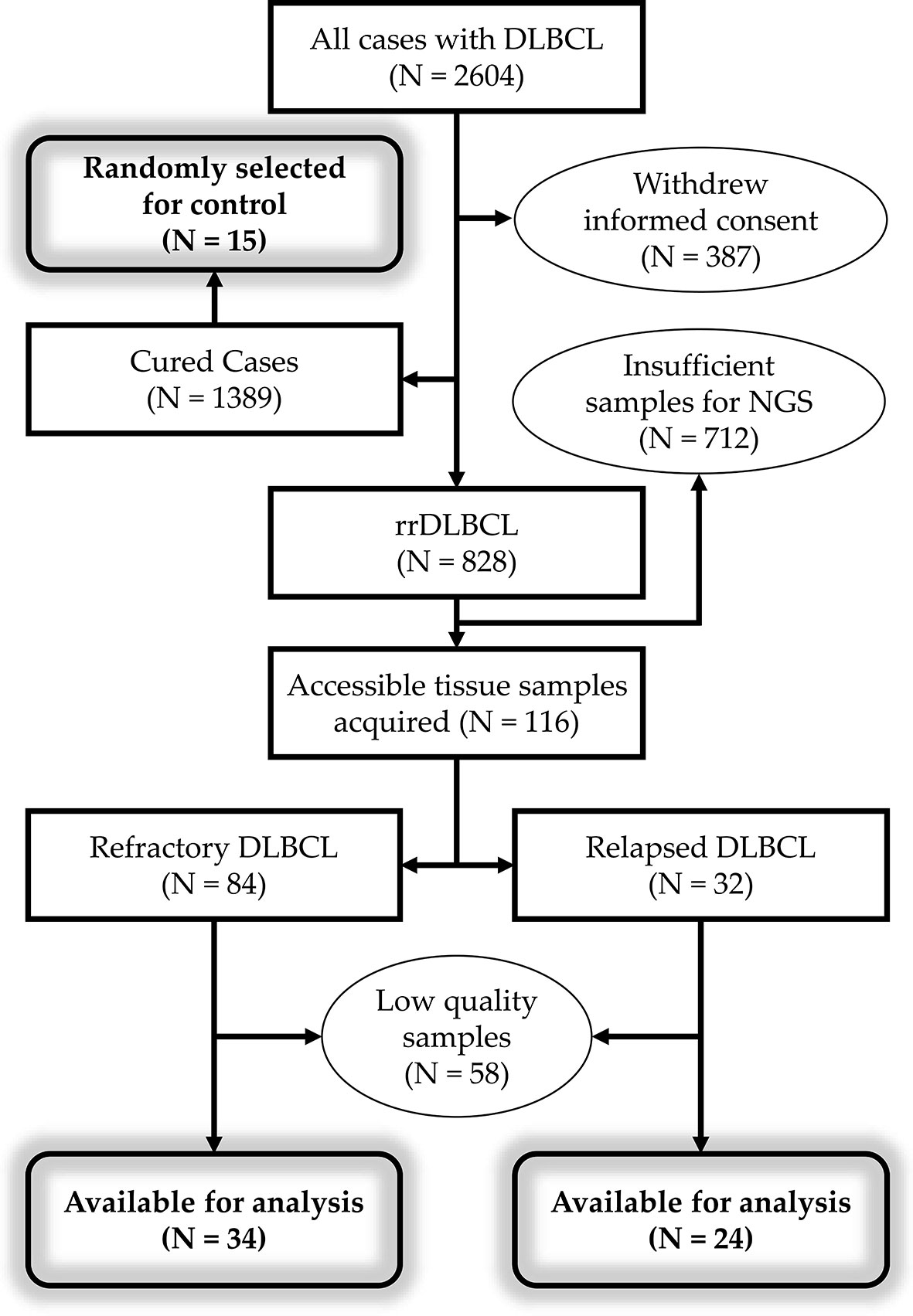
Figure 1 Workflow of patient selection. Total of 2,604 patients were diagnosed with DLBCL. Of the 2,217 patients with informed consent to review of medical records, 828 patients have refractory or relapsed disease. DNA extraction was performed using samples from 116 patients with rrDLBCL, but samples from 58 patients failed to pass the quality control. DLBCL, diffuse large B-cell lymphoma; rr, refractory/relapsed; NGS, next-generation sequencing.
The COO did not differ significantly between patients with refractory, early relapse, and cured DLBCL. The IPI score was high, indicating poor prognosis in patients with refractory disease or early relapse compared with those with late relapse or cured DLBCL (P = 0.006). MYC translocation was found in 19% of early relapsed and 30% of refractory DLBCL patients. None of the late relapsed case had MYC translocation. Only one patient with refractory disease was found to have rearrangement of MYC and BCL6. Of the 58 patients with rrDLBCL, diagnostic pretreatment biopsies and post-treatment biopsies obtained after the progression of tumor were available for 29 patients and 47 patients, respectively. Pairs of pre- and post-treatment biopsy data were available for 18 patients (Figure 2).
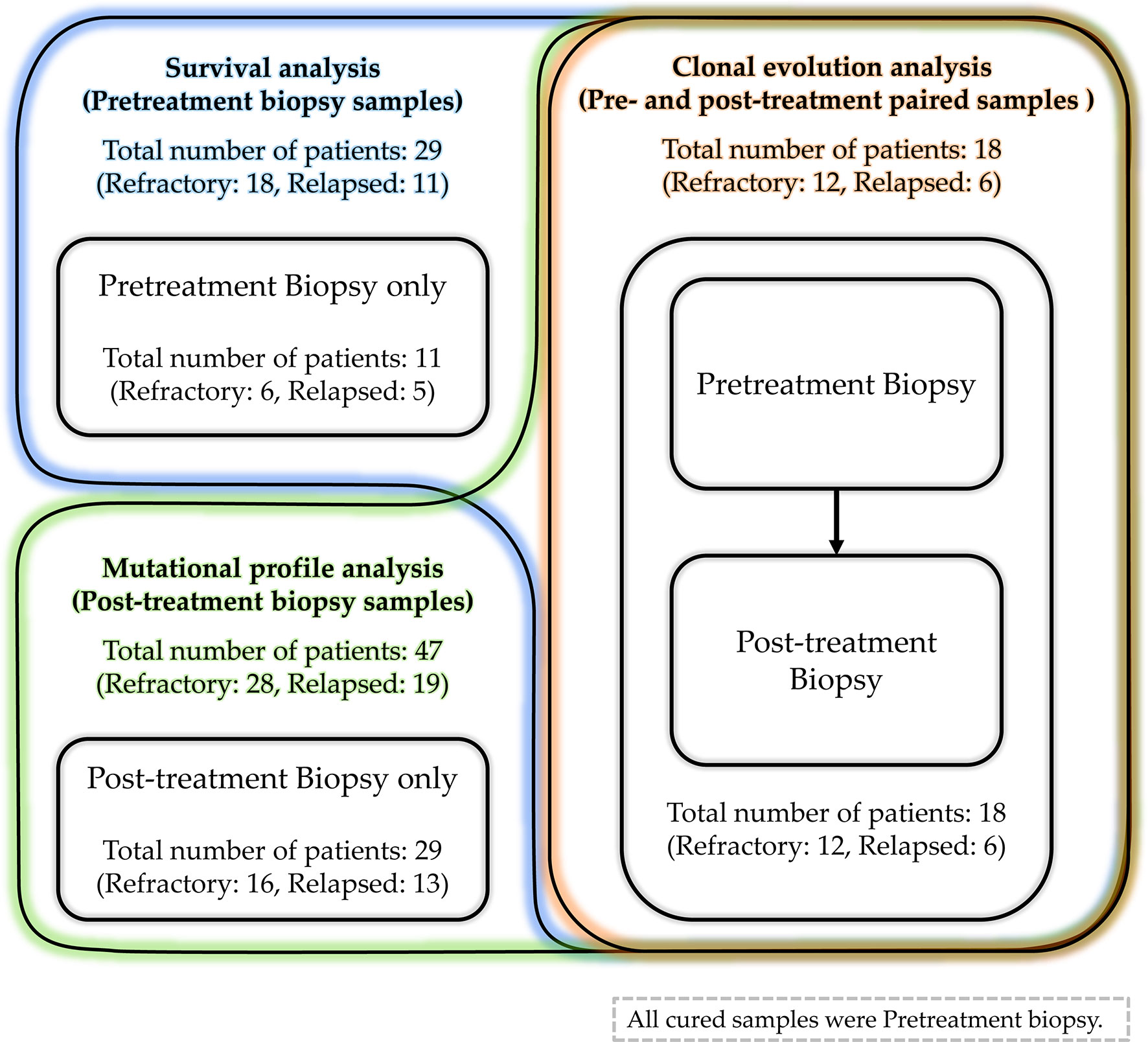
Figure 2 The details of acquired samples. The pretreatment biopsy samples were obtained from 29 patients (18 refractory DLBCL and 11 relapsed DLBCL). Among them, samples obtained from 18 patients (12 refractory DLBCL and 6 relapsed DLBCL) were paired samples and other samples from 11 patients (6 refractory DLBCL and 5 relapsed DLBCL) were unpaired pretreatment samples. The post-treatment biopsy samples were obtained from 47 patients (28 refractory DLBCL and 19 relapsed DLBCL). Excluding paired samples from 18 patients, samples from 29 patients (16 refractory DLBCL and 13 relapsed DLBCL) were unpaired post-treatment samples.
Mutational Profile of rrDLBCL
The mutational profiles of post-treatment biopsy results for 47 rrDLBCL patients are illustrated in Figure 3. At least five non-synonymous single nucleotide variants SNVs, SVs, or SCNAs were detected in rrDLBCL tumor samples (median, 17; range, 5–44).
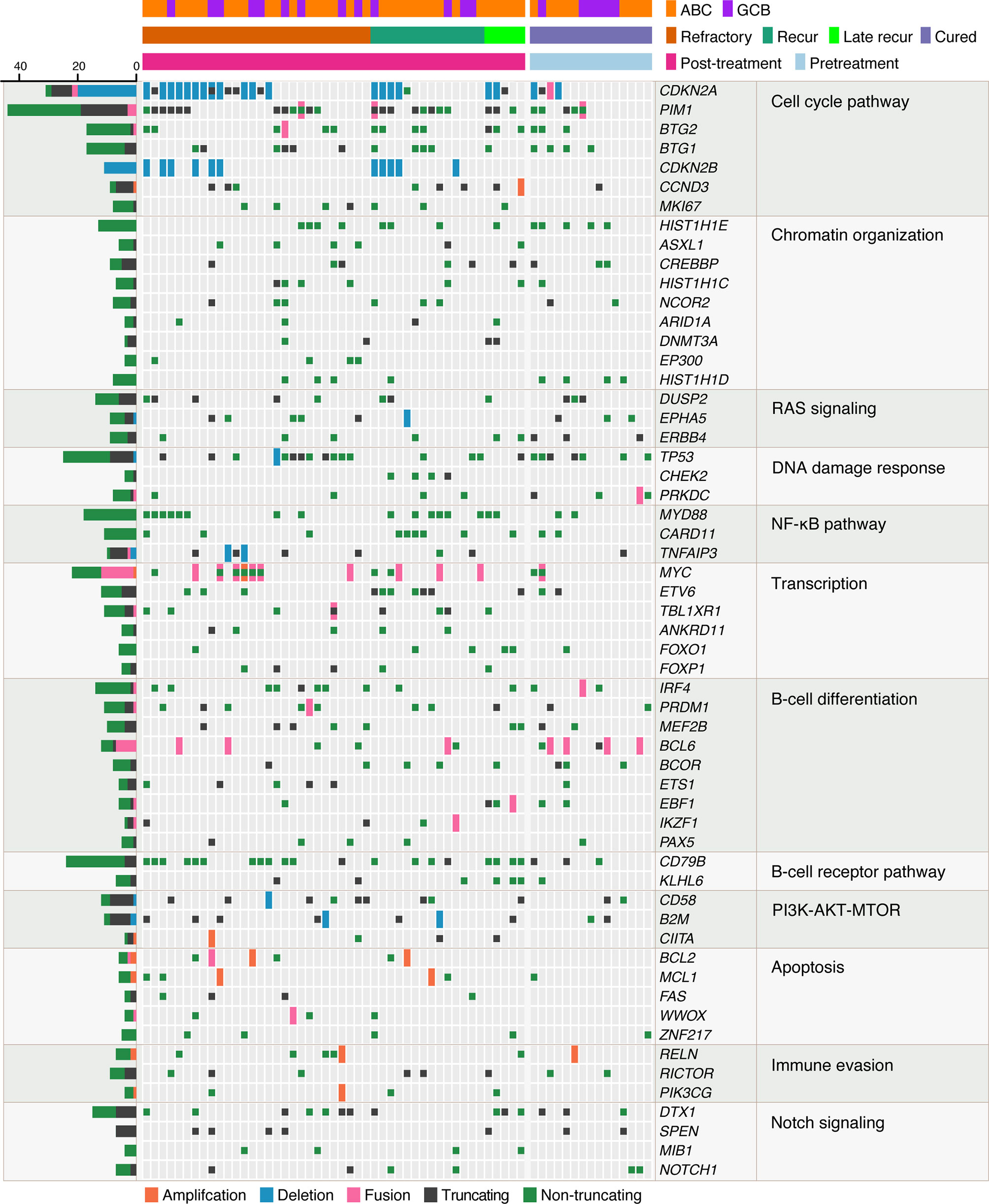
Figure 3 Mutational profiles of post-treatment tumor samples of relapsed/refractory diffuse large B-cell lymphoma (rrDLBCL) and pretreatment tumor samples of cured DLBCL. rrDLBCL patients include 28 refractory, 14 early recurred, and 5 late recurred patients. Genes mutated in > 3 samples and pathways having > 1 mutated genes are shown. The pathways and genes are ordered by the frequency of mutation. Copy numbers > 6 are marked as amplifications and copy numbers < 0.8 are marked as deletions. COO, cell of origin; ABC, activated B-cell-like subtype; GCB, germinal center B-cell-like subtype.
The genes most frequently altered (> 20% of patients), in decreasing order of frequency, were CDKN2A, PIM1, CD79B, TP53, MYD88, PCLO, MYC, BTG2, BTG1, CDKN2B, DTX1, CD58, ETV6, and IRF4. Mutations were especially frequent in MYD88 [Present study (pretreatment), 35%; Present study (post-treatment), 34% vs. Karube et al. (pretreatment), 23%; Morin et al. (post-treatment), 20%; COSMIC (pre- or post-treatment), 15%; cured, 13%], CD79B (41% and 43% vs. 9, 16, 4, and 20%, respectively), CDKN2A (38% and 51% vs. 26, not applicable, 19, and 27%, respectively), and MYC (35% and 27% vs. 9, 12, 4, and 13%, respectively) than for that reported for DLBCL previously (24–26) and in the cured group in our study (Supplementary Table 3). Mutations in PRDM1, MKI67, MYD88, and IRF4 tended to occur more frequently in patients with ABC-type DLBCL. By contrast, mutations in SOCKS1, CREBBP, NCOR2, RICTOR, PAX5, and BCL2 were more frequent in patients with GCB-type DLBCL (Figures 4 and 5, Supplementary Table 4).
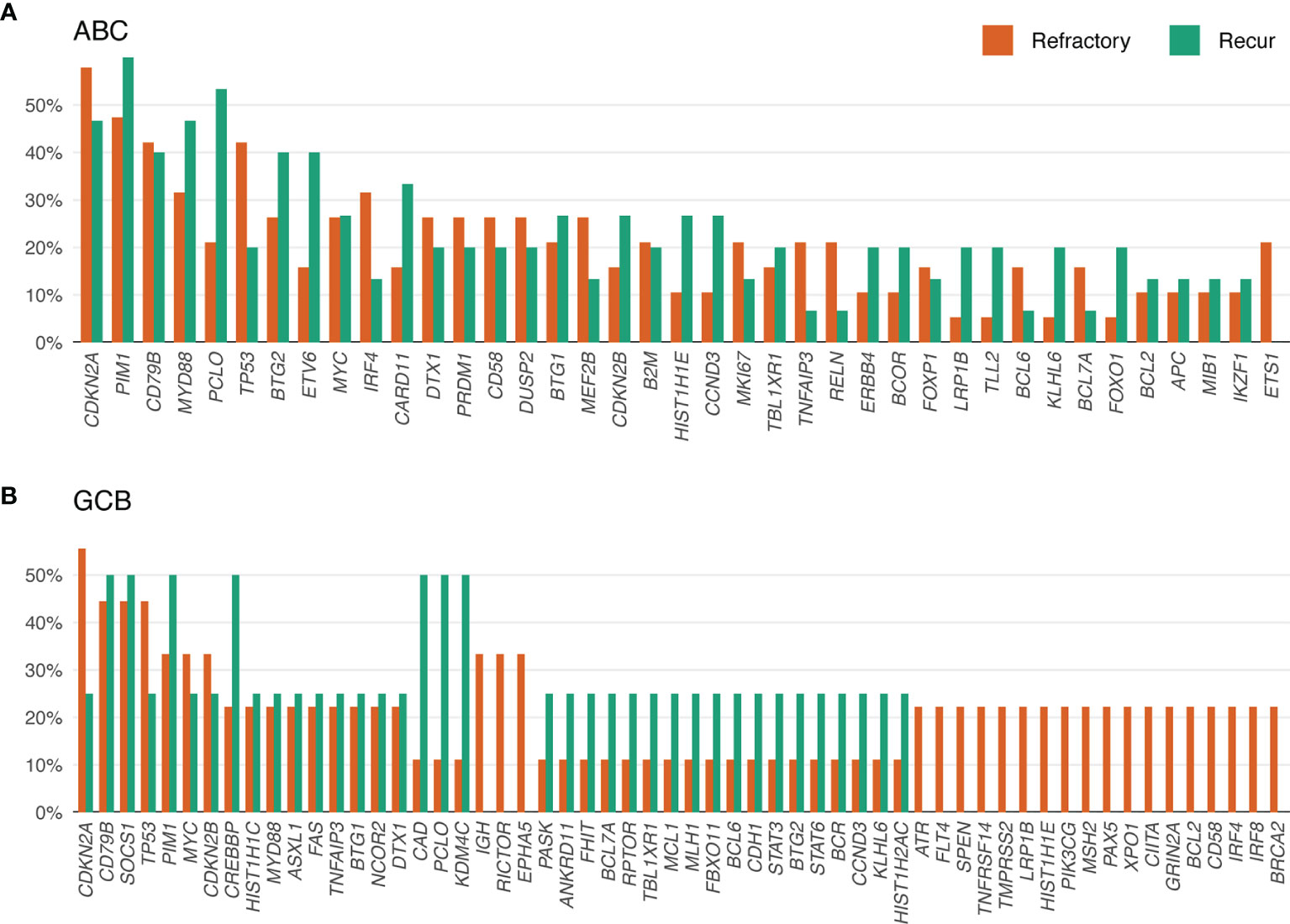
Figure 4 Mutational frequency of genes in post-treatment tumor samples from patients with relapsed/refractory diffuse large B-cell lymphoma (rrDLBCL). (A) The mutational frequency ABC-type rrDLBCL including 19 refractory and 15 recurred patients. (B) The mutational frequency of GCB-type rrDLBCL including 9 refractory and 4 recurred patients.
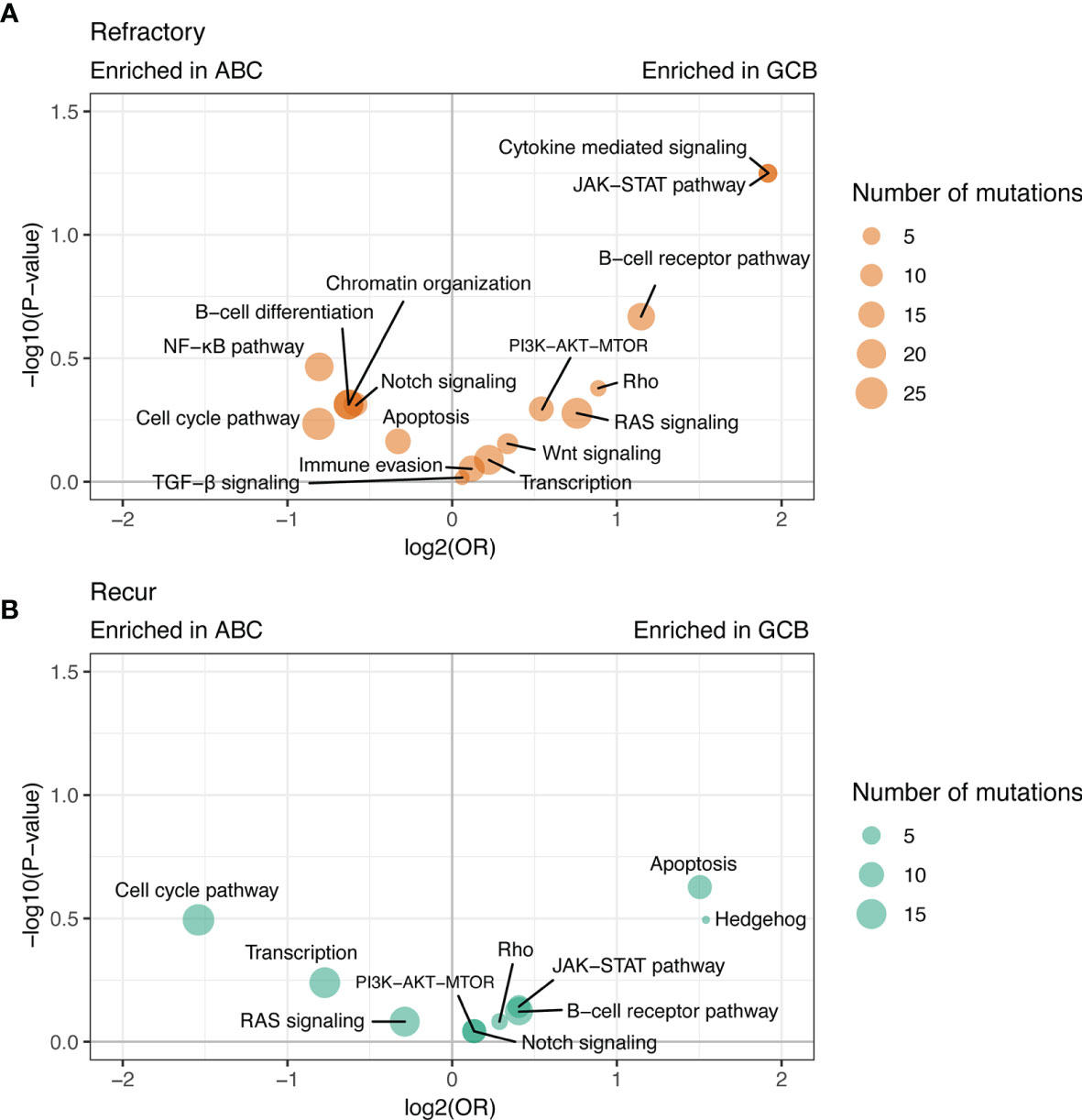
Figure 5 Differences in the frequency of mutated pathways between post-treatment tumor samples of the ABC-type rrDLBCL and GCB-type rrDLBCL. Mutations of genes are aggregated for each pathway and the differences between ABC-type rrDLBCL and GCB-type rrDLBCL are shown for (A) refractory disease and (B) recurred disease.
We examined the molecular functions and relevant signaling pathways of mutated genes. In patients with rrDLBCL, mutation of more than one gene that plays a role in the cell cycle pathway was found in 92%, in the chromatin remodeling in 81%, in the RAS signaling pathway in 81%, in the NF-κB pathway in 79%, in the DNA damage response in 79%, in transcription regulation in 79%, in the B-cell differentiation in 75%, in the BCR pathway in 64%, and in the immune evasion in 51% (Supplementary Table 4). The NOTCH and JAK–STAT pathways were affected in 45% and 30% of these patients, respectively. DTX1 was the most frequent mutation (23%) among genes involved in the NOTCH pathway followed by SPEN (11%), NOTCH1 (9%) NOTCH2 (4%), and SGK1 (2%). DTX1 is a negative regulator of NOTCH signaling (27) and a predictor of worse prognosis. SOCS1, a negative regulator of the receptor-signaling pathway via JAK–STAT was mutated in 15% of the rrDLBCL patients.
Tumor-suppressor genes involved in cellular proliferation and the DNA damage response were mutated at a high frequency and included CDKN2A (51%), CDKN2B (23%), BTG2 (28%), BTG1 (23%), and TP53 (34%). The tumor-suppressor genes CDKN2A and TP53 are two of the most frequently inactivated genomic loci in human cancers (28, 29). As expected because of its tumor-suppressor function, CDKN2A was altered by inactivating mutations including homozygous deletion (18/27 alterations), truncating mutation (6/27), non-truncating mutation (2/27), and SV (1/27). rrDLBCL harboring either the CDKN2A (24 patients) or TP53 (16 patients) alteration occurred in 37 (79%) patients, and each mutation was exclusive to the other except for three patients.
BTG2 and BTG1 are tumor-suppressor genes and members of the human BTG/TOB family. BTG2 is a p53-dependent component of the DNA damage cellular response pathway (30) and has been shown to negatively control a cell cycle check-point at the G1 to S phase transition (31). BTG2 was altered by a truncating mutation (1/14 alterations), non-truncating mutation (12/14), and SV (1/14). BTG1 was affected by a truncating mutation (4/13 alterations) and non-truncating mutation (9/13) and overlapped with a mutation of either CDKN2A or TP53 (Supplementary Table 5).
Mutations of oncogenes involved in the regulation of cell cycle and transcription, and activation of the BCR-NF-κB pathway included PIM1 (49% of patients), CD79B (43%), MYD88 (34%), MYC (28%), CARD11 (19%), and ETV6 (21%). MYC alterations comprised focal amplification (1/19 alterations), non-truncating mutation (8/19), and SV (10/19). All variants of MYD88 mutation were MYD88 L265P. PIM1 mutation comprised many different variants in each sample (median, 0; range, 0–15) and 48% were SNV/indel. Aberrant somatic hypermutation is an important molecular feature of DLBCL and targets several proto-oncogenes (32, 33). When analyzed using a method published previously (32), among the genes mutated in these rrDLBCL patients, PIM1 and BTG1 had an SHM indicator value of < 0.1, which confirmed the alterations by somatic hypermutation (Supplementary Table 6).
There were no significant differences in the rates of mutation between patients with refractory DLBCL and relapsed DLBCL. The overall mutation frequency did not differ significantly between the initial biopsy and post-treatment biopsy. The frequencies of mutations involving immune evasion (B2M, CD58), the NF-κB pathway (CARD11, TBL1XE1), the JAK–STAT pathway (SOCS1), chromatin remodeling (CREBBP, DNMT3A), and the cell cycle (CDKN2A, PIM1) were higher in the post-treatment biopsy, although the difference was not significant (Supplementary Table 3).
Prognostic Significance of Genes and Pathways Mutated in the Pretreatment Biopsy
The mutational frequency of genes and pathways in pretreatment biopsy for refractory, recurred and cured DLBCL were shown in Supplementary Table 7. Alterations in B-cell differentiation pathway and JAK–STAT pathway tended to be more frequent in cured DLBCL (86.7% and 66.7%) than recurred DLBCL (45.5% and 18.2%).
Pretreatment biopsy samples were available for 29 patients with rrDLBCL, including 18 with refractory DLBCL, eight with early relapse, and three with late relapse. Twenty-six patients died and three patients were alive with the disease at the follow-up. The median OS was 20.6 months (range, 5.4–200.5) and median PFS was 9.1 months (range, 2.3–182.0).
We used multivariate analysis to evaluate the prognostic significance of genes mutated in > 3 patients by considering the IPI and COO. Mutated genes associated with a poor OS included FGFR2, BCL2, BCL6, BCL10, and TP53 (P < 0.05). Mutations in NOTCH1, FGFR2, BCL7A, BCL10, SPEN, and TP53 were significantly associated with a poor PFS (Figure 6). When analyzed in the gene set, mutation of genes involved in B-cell differentiation was associated with a poor OS and that involved in DNA damage response was associated with a poor PFS.
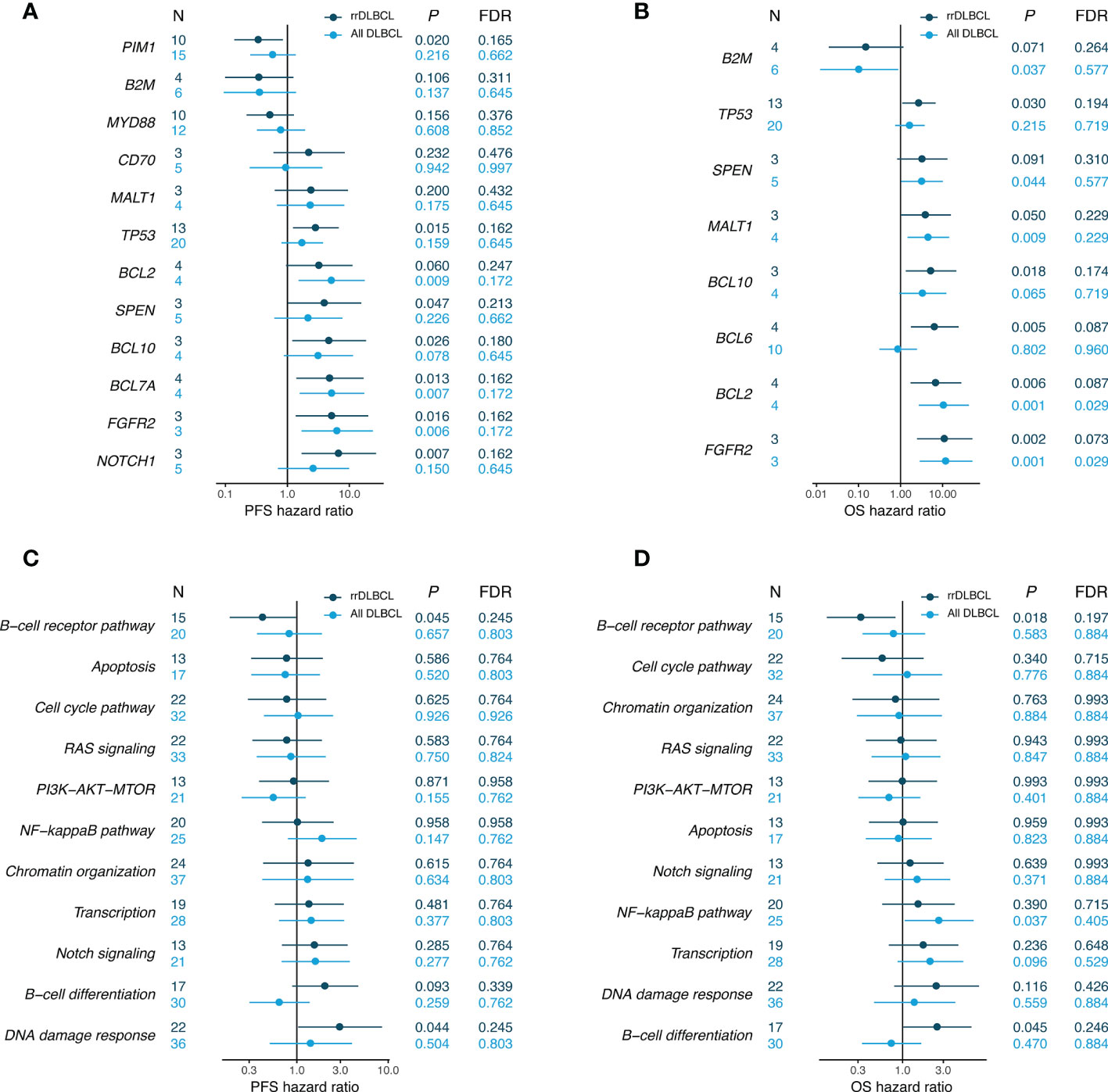
Figure 6 Associations between survival probability and mutations in pretreatment tumor samples. Hazards ratios (HRs) are shown separately for pretreatment samples of rrDLBCL and all DLBCL including cured disease. HR and P values were corrected by the COO and IPI score. (A, B) Genes with P values < 0.05 in the univariate analysis for rrDLCL are included. (C, D) Pathways with > 9 mutated rrDLBCL samples are included.
Tumor Mutational Burden
The TMB in the pretreatment samples was not associated with the COO or tumor recurrence. The median TMB (range) values according to patient group were 12.2 (3.6–30.7) for ABC subtype, 20.7 (4.5–41.5) for GCB subtype, 12.6 (3.6–41.5) for cured, 14.4 (4.5–30.7) for refractory, 14.0 (5.4–39.7) for recurrent, and 10.8 (7.2–15.3) for late recurrent.
The survival rates were compared by dividing into two groups based on the median value of 13, with no difference in survival rates between low-TMB and high-TMB groups. The respective median PFS values (95% CI, in months) were 29.4 [14.7 to not reached (NR)] and 16.1 (8.1 to NR) (P = 0.400). The median OS values were 55.5 (22.3 to NR) and 29.6 (16.0 to NR). When changes in TMB were compared between pretreatment and post-treatment samples, patients with early or late relapsed DLBCL showed higher dynamics of subclones than those with refractory DLBCL, which may reflect the longer interval between biopsies. The TMB was low at the initial biopsy in late relapse patients, who showed high dynamics of subclones leading to increased TMB at the time of relapse (Figure 7).
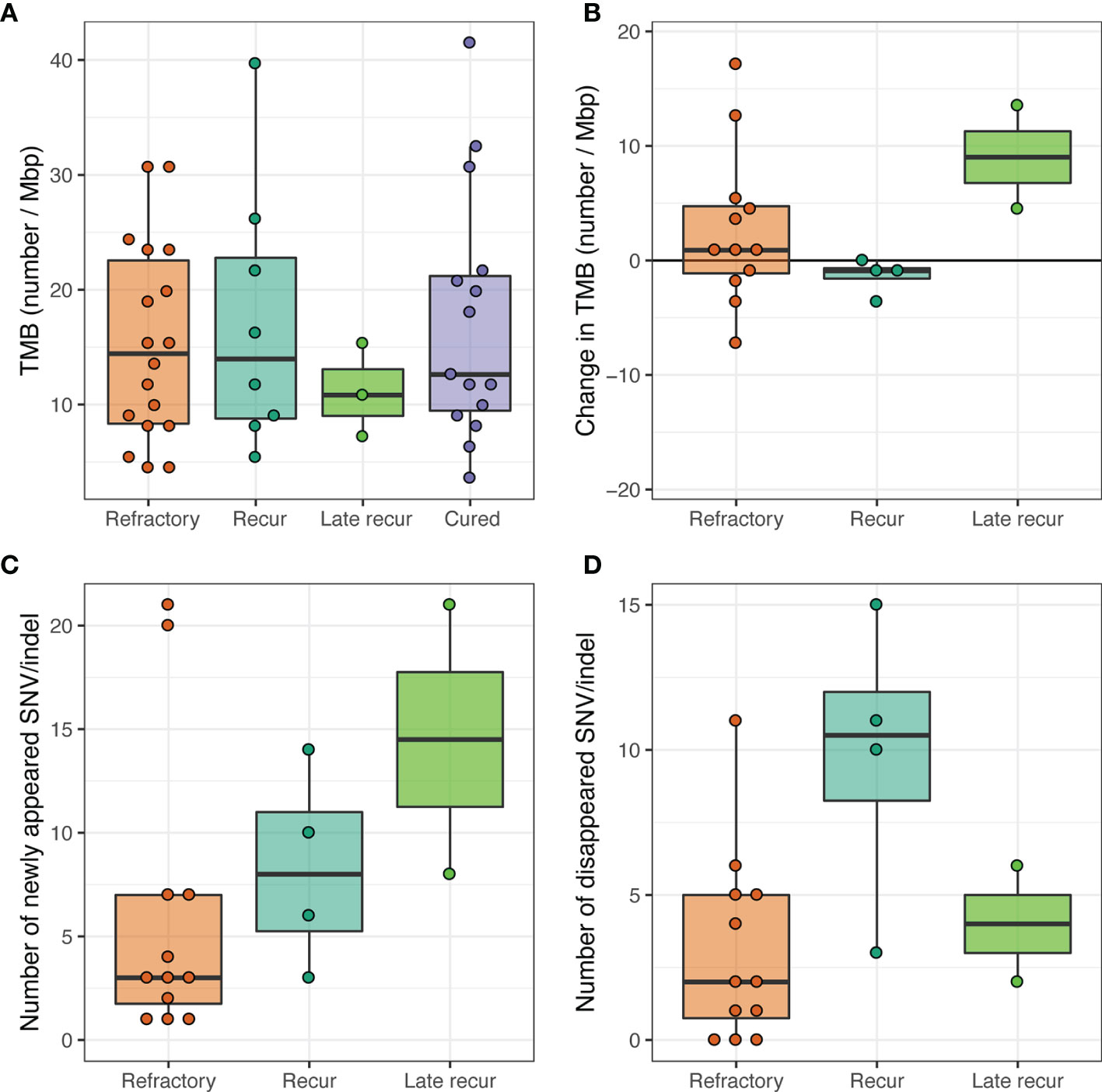
Figure 7 Tumor mutational burden (TMB). (A) TMB of pretreatment tumor samples. (B) Change in TMB between pretreatment and post-treatment paired tumor samples. (C) The number of SNV/indels that were not present in pretreatment tumor samples but appeared for the first time in post-treatment tumor samples. (D) The number of SNV/indels that were present in pretreatment tumor samples but disappeared in post-treatment tumor samples.
Clonal Evolution
To explore the mutational evolution, we compared 21 pairs of pre- and post-treatment samples from 18 patients. Median 11 (range, 4–33) SNV/indels are shared between pretreatment and post-treatment samples (Figure 8). To compare VAFs between samples with different purity, the VAF values were normalized to a median VAF value of 0.5. In some patients, CXCR4, MEF2B, and DUSP2 mutations tended to appear for the first time in a major clone after chemotherapy. The VAFs of NCOR2, GNAS, EBF1, FOXP1, RUNX1, PCLO, CD58, RICTOR, and CREBBP mutations tended to increase after chemotherapy (Figure 9A, Supplementary Table 8). NF-κB-activating driver mutations such as MYD88 and CD79B mutations were mostly truncal and changed little after the chemotherapy. Whereas Nijland et al. reported increased VAFs in SOCS1 and PIM1 (34) at relapse, only the VAF for SOCS1 showed a tendency to increase in our data.
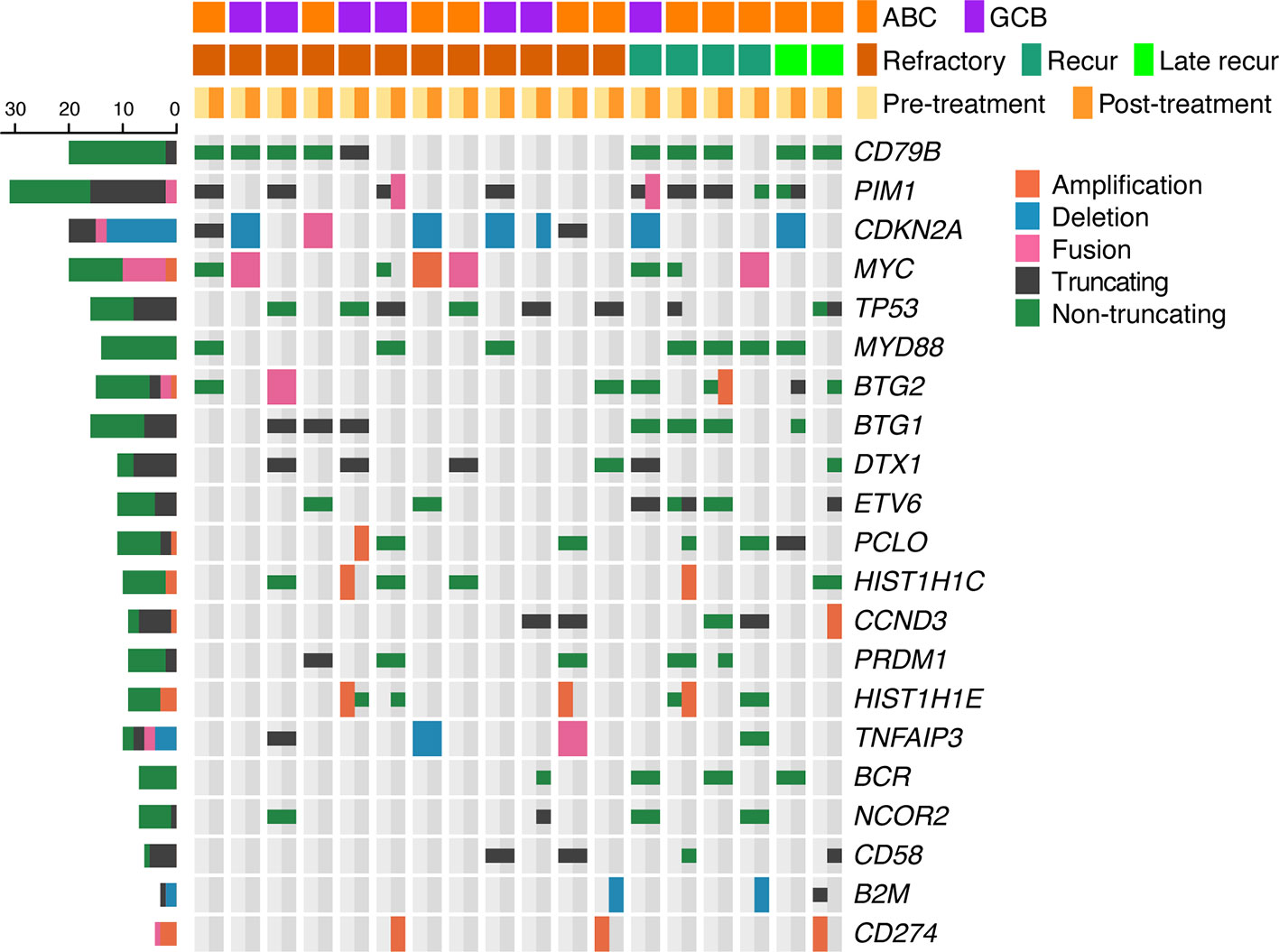
Figure 8 Mutational profiles of 18 paired pretreatment and post-treatment rrDLBCL samples. Genes mutated in > 3 cases are shown. The genes are ordered by the frequency of mutation. Unlike the Figure 3, copy numbers > 4 are marked as amplifications. COO, cell of origin; ABC, activated B-cell-like subtype; GCB, germinal center B-cell-like subtype.
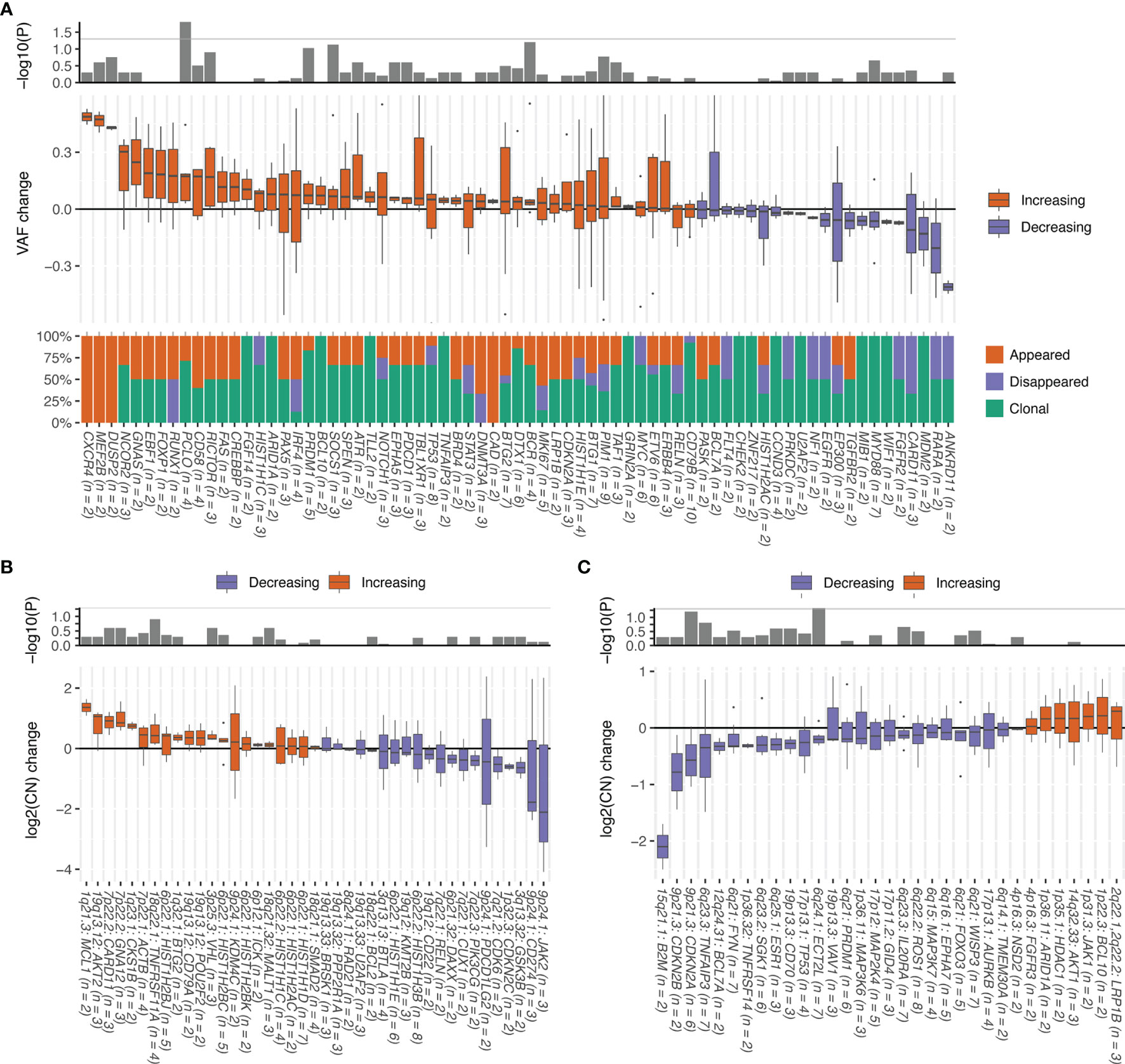
Figure 9 Change in alterations between pretreatment and post-treatment paired tumor samples. Genes altered in > 1 patient are included. P values were calculated using the Wilcoxon signed-rank test. Numbers of samples with alterations are shown in parentheses. (A) Change in variant allele fraction (VAF) of each gene and percentages of mutation SNV/indels that appeared for the first time in post-treatment tumor samples (newly appeared), disappeared in post-treatment tumor samples (disappeared), or were present in both pretreatment and post-treatment tumor samples (remaining). (B) Change in the log2 copy number of genes with amplification. (C) Change in the log2 copy number of genes with deletion.
The copy numbers of amplified genes and deleted genes were also compared (Supplementary Tables 9 and 10). The copy numbers for MCL1 (1q21.3), AKT2 (19q13.12), CARD11 (7p22.2), GNA12 (7p22.2), CKS1B (1q23.1), ACTB (7p22.1), TNFRSF11A (18q22.1), HIST1H2BJ (6p22.1), BTG2 (1q32.1), CD79A (19q13.12), POU2F2 (19q13.12), VHL (3p25.3), and HIST1H2BC (6p22.1) tended to increase (Figure 9B). By contrast, the copy numbers of B2M (15q21.1), CDKN2B (9p21.3), CDKN2A (9p21.3), TNFAIP3 (6q23.3), BCL7A (12q24.31), FYN (6q21), TNFRSF14 (1p36.32), SGK1 (6q23.2), ESR1 (6q25.1), CD70 (19p13.3), TP53 (17p13.1), and ECT2L (6q24.1) tended to decrease (Figure 9C).
CD58 mutation or B2M deletion, both of which are involved in the immune evasion, occurred for the first time in post-treatment samples in four of 18 (22%) patients. By contrast, amplification of CD274 (PD-L1), which is also involved in the immune evasion, occurred for the first time in one patient but was lost in two patients. The one patient who lost CD274 amplification (copy number = 9.7) gained CD58 mutation. The other patient who lost CD274 amplification (copy number = 5.1) gained B2M deletion. The patient who gained CD274 amplification (copy number = 11.8) did not have CD58 mutation or B2M deletion. Overall, seven (39%) patients gained or retained alterations involved in immune evasion after chemotherapy. Alterations in TP53 and CDKN2A have been associated with poor survival outcome in previous studies (26, 35). Consistent with these results, the VAFs for the mutation of these genes tended to increase and the copy number of these genes tended to decrease.
Molecular Classification
Several attempts have been made to classify DLBCL using mutational profiles (8, 9, 35), which have shown significant differences in survival rate between some subtypes. Because this molecular classification is made based on whole exome sequencing data, to use this molecular classification in clinical practice, it requires modification of the model, so that the tumors can be also classified using targeted panel sequencing data. We adjusted two published models (8, 9) and classified our DLBCL samples. When we applied the classification of Chapuy et al. to our data, only one patient was classified as C3, and C5 (Figure 10) accounted for a large percentage because of the higher frequency of the ABC subtype in our sample. Patients with C3 or C5 DLBCL had the worst survival (Figure 11), which was consistent with the data of Chapuy et al. (9). Similarly, when we applied the classification of Schmitz et al. to our data, the MCD subtype (40%) and other ABC subtype (29%) accounted for the main subtype. Among patients with DLBCL, the MCD subtype has the worst prognosis and other ABC subtype has an intermediate prognosis (8). Although statistical significance was not reached, we could reproduce the trend for survival difference in this molecular classification (Figure 12) which was classified using targeted panel sequencing data.
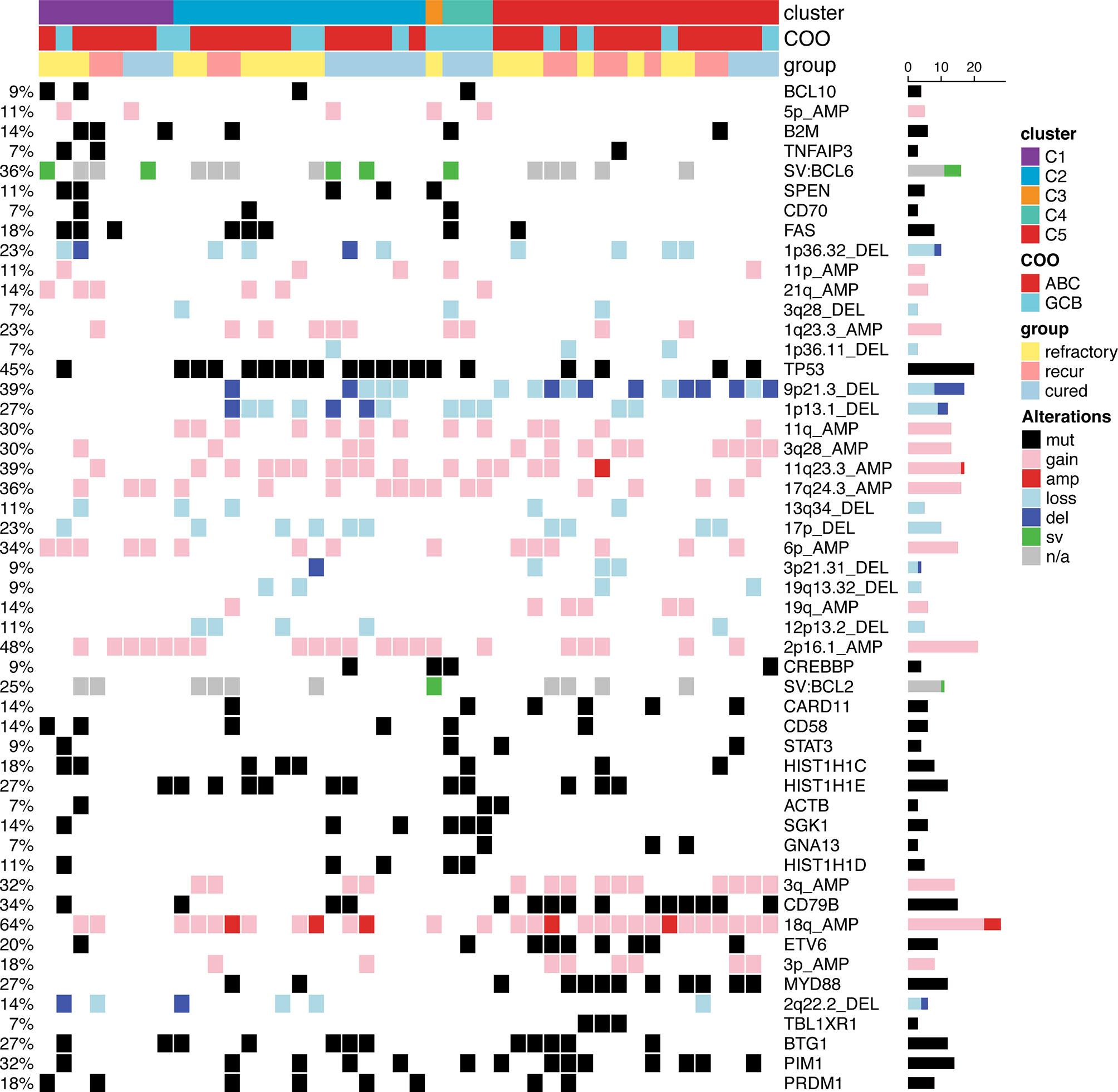
Figure 10 Molecular classification of pretreatment tumor samples according to the classification of Chapuy et al. (9). Among the 71 features used for classifications 51 features with > 2 alterations are shown. Features were ordered first by the cluster in which the feature contributed the most and second by the degree of contribution.
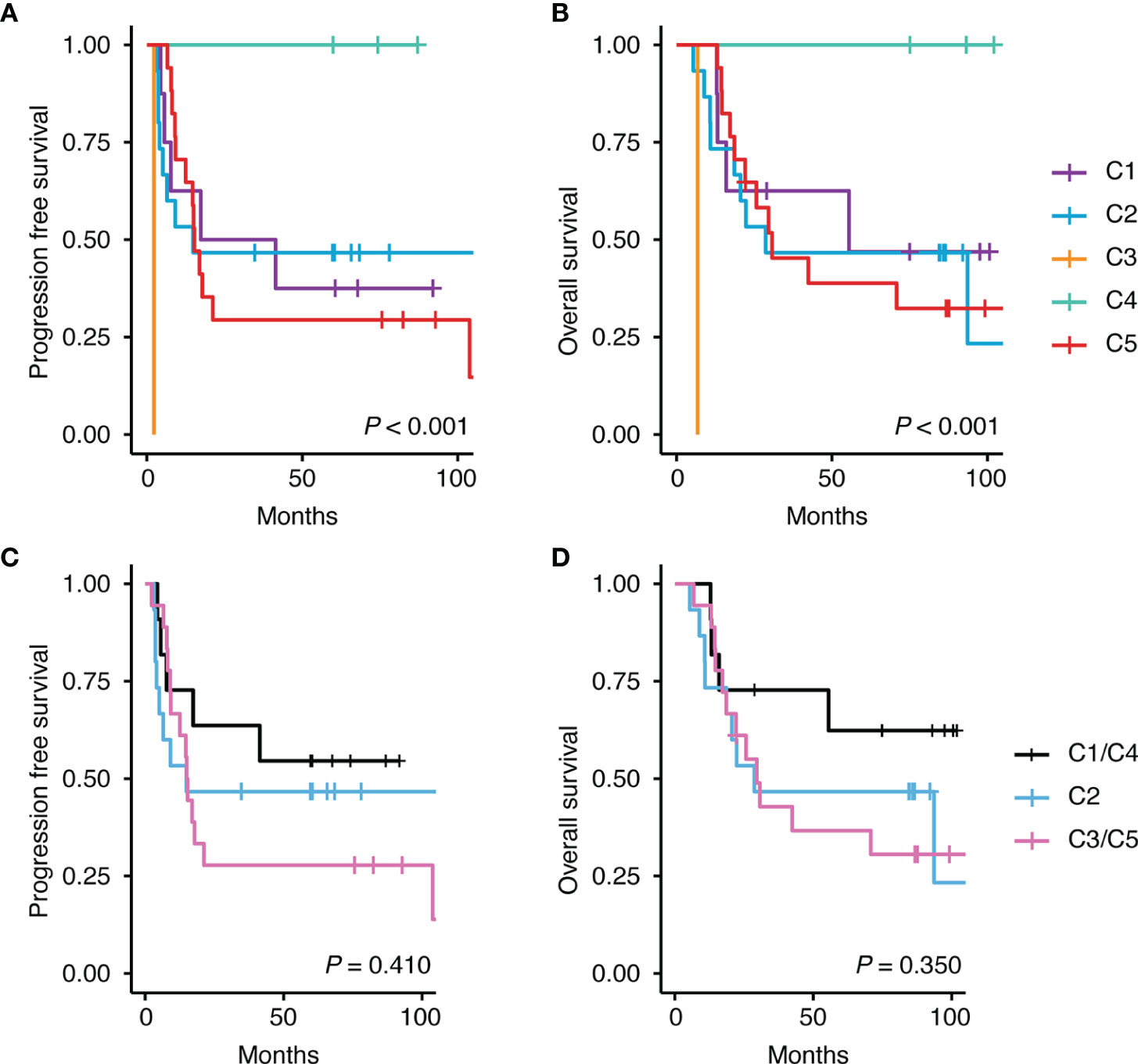
Figure 11 Differences in survival according to the classification of Chapuy et al. (9). Kaplan-Meier curves shows the difference in survival rate according to each class for (A) progression free survival and (B) overall survival. C1 and C4 were reported to have good prognosis and C3 and C5 were reported to have poor prognosis. This trend was also seen in our data on (C) progression free survival and (D) overall survival.
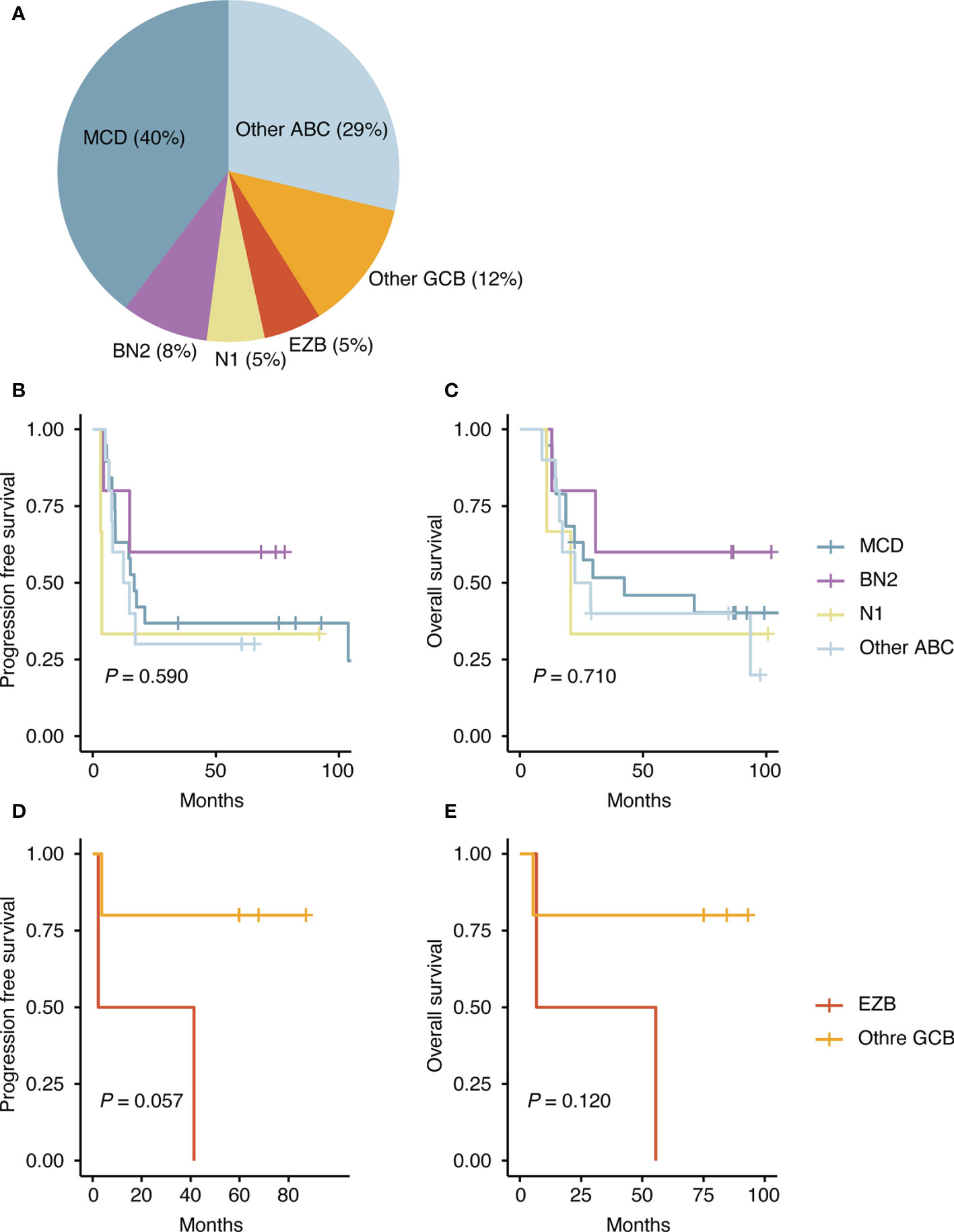
Figure 12 Molecular classification according to the classification of Schmitz et al. (8). (A) Proportion of assigned classes. (B) Difference in progression free survival among the ABC-type DLBCLs. (C) Difference in overall survival among the ABC-type DLBCLs. (D) Difference in progression free survival among the GCB-type DLBCLs. (E) Difference in overall survival among the GCB-type DLBCLs.
Discussion
The genomic characteristics of tumors provide important information for selecting patients with poor prognosis for initial treatment and for developing further treatment plans. In this study, we examined the genomic profiles of post-treatment biopsy for 47 patients with rrDLBCL and compared the genomic profiles of pre- and post-treatment paired biopsies for 18 patients to identify gene candidates that may cause treatment resistance. In addition, using the pretreatment biopsy samples, we compared the survival rates between 29 patients with rrDLBCL and 15 cured patients in an attempt to identify genes with prognostic significance. Although the number of patients was not sufficient to overcome the genetic heterogeneity of DLBCL, we identified potential targets for treatment and made several compelling observations about tumor evolution.
The genome of rrDLBCL was enriched by mutation of genes involved in the cell cycle, NF-κB pathway, chromatin remodeling, RAS signaling, transcription regulation, DNA damage, B-cell differentiation, apoptosis, the PI3K–Akt–mTOR pathway, and immune evasion. As found in a previous study (24), the mutation frequency was higher for rrDLBCL than that reported for de novo DLBCL. Driver mutations such as those in CD79B, CDKN2A, MYD88, MYC, and CCND3, which are known to negatively affect the prognosis of DLBCL (36–40), were enriched in rrDLBCL. CDKN2A encodes two proteins, p16INK4a and p14ARF. P14ARF is a central actor in the cell cycle regulation process and participates in the ARF–MDM2–p53 and Rb–E2F1 pathways (41). Mutation in CDKN2A is the most common mutation associated with rrDLBCL and is an indicator of poor prognosis after R-CHOP treatment (42). The combination of CDKN2A and TP53 mutations confers a worse survival independent of the IPI (25, 42). In our study, patients with TP53 alterations had a worse prognosis, which was independent of the COO and IPI. Mutation of TP53 has been reported in 17–23% of de novo DLBCL (25, 26) and 32% of rrDLBCL (24). In our cohort, the frequency of TP53 was very high in both rrDLBCL patients and in the cured patients for whom all TP53 mutations were disruptive mutations. Because of the high frequency of TP53 alterations in the cured group, we initially questioned the effect of TP53 on the survival of patients with DLBCL. However, the decreasing copy number of TP53 and increasing VAF for TP53 SNV/indels in the post-treatment biopsy support the idea that TP53 alterations contribute to tumor evolution in DLBCL. This frequency of TP53 variants, which was much higher than in other studies, may reflect the high coverage of our targeted sequencing or may be related to bias caused by the small number of samples in the cured group. Mutation of PIM1 is the second most common mutation after CDKN2A in rrDLBCL and its mutation rate was slightly higher in ABC-type DBCL than in GCB-type DLBCL, as shown in a previous study (43). PIM1 is a multifunctional gene that activates SOCS1 and SOCS3 to act as negative regulators of the JAK–STAT pathway (44). In addition, Pim-1 interacts with Myc (45–47), Bad (48), Bcl-2 (49), p21Cip1/WAF1 (50), p27Kip1 (51), RelA/p65 (52), and the fusion gene E2A–PBX1 (53) to promote cell cycle progression, prevent apoptosis, and induce carcinogenesis. A recent study revealed that point mutations within the kinase Pim-1 reduce sensitivity to ibrutinib in ABC-type DLBCL (54). In our cohort, PIM1 mutation frequency increased in the post-treatment biopsy, but PIM1 mutation frequency was also high in the cured group and the overall VAF did not change in the post-treatment biopsy. Therefore, the impact of PIM1 in rrDLBCL is questionable in our study.
Information about the mutational frequency at the initial diagnosis and in the post-treatment biopsy combined with changes in the VAF provide an insight into the clonal evolution and identification of genes with chemotherapy resistance. According to changes in the VAF of SNVs/indels, the mutations in rrDLBCL can be divided into three groups. The first group comprised mutations that were truncal and were maintained in both the initial and post-treatment biopsies with high dynamics of subclones. The genes showed partial loss of the major clone or acquisition of new variants at relapse. This was the most common pattern and may correspond to the late divergent mode of clonal evolution by Jiang et al. (55), which shows continuous alterations of the tumor genome with additional mutations acquired to achieve relapse. NF-κB-activating driver mutations, such as in MYD88 and CD79B, were stable and changed little after immunochemotherapy. In the second group, which included RUNX and DNMT3A, the major mutation had completely disappeared in the post-treatment biopsy with the acquisition of a new variant. The third group included CXCR4, MEF2B, DUSP2, and CAD, and had a newly appearing clone at the post-treatment biopsy that was not detected in the initial biopsy. This new clone may have derived from a small clone at the initial biopsy whose presence was below the level detected by targeted sequencing or may represent an early divergent clone.
Among the genes altered in rrDLBCL, those with an increase in VAF or with a change in copy number in the post-treatment biopsy can be considered genes involved in relapse or chemoresistance. After immunochemotherapy, the frequency of mutations in genes, including B2M, CD58, CARD11, CDKN2A, CDKN2B, PIM1, SOC1, and CREBBP, increased. The mutations in some genes showed an increase in VAF (NCOR2, GNAS, EBF1, FOXP1, RUNX1, PCLO, CD58, RICTOR, and CREBBP) or an increase in copy number (MCL1, ATK2, CARD11, GNA12, CKS1B, ACTB, TNFRSF11A) or a decrease in copy number (B2M, CDKN2B, CDKN2A, TNFAIP3, BCL7A, FYN, TNFRSF14, SGK1, CD70, TP53). PCLO is the only gene with a significant increase in VAF, but the mutation is considered a passenger mutation and is frequent due to the large size of the gene (56). CDKN2A and CDKN2B showed increased overall frequencies of mutation and copy number loss. CREBBP encodes histone acetyltransferase; its mutation is a hallmark of GCB-type DLBCL and confers significantly worse OS and PFS (57, 58). CREBBP showed both increased frequency of mutation and increased VAF. NF-κB-activating genes show an increased mutation frequency and copy number (CARD11) and decreased copy number (TNFAIP3). It is noteworthy that immune-evasion genes showed increased overall mutation frequency (CD58, B2M) as well as increased VAF (CD58) and decreased copy number (B2M, CD70).
Refractory DLBCL refers to a tumor with primary resistance to the initial treatment, and patients show either no CR or a short duration of CR with relapse within 6 months. As shown in our data, refractory DLBCL is characterized by a relatively high TMB and close mutational distance between the pre- and post-treatment biopsy, that is, low dynamics of subclones. Of note is that, among the refractory DLBCL patients, those who did not experience CR had more mutations in the immune-evasion gene and more DTX1 mutations than the other patients with rrDLBCL. There are studies suggesting the mutation of genes involved in antigen presentation as a resistance mechanism (55, 59). These findings support that immune evasion is important to refractoriness in rrDLBCL. Drugs to reverse immune evasion are actively being developed. In particular, drugs that target immunomodulatory molecules are promising. Several phase 1 or 2 clinical trials using anti-PD-1 or anti-PD-L1 monoclonal antibodies on DLBCL patients are ongoing (60). Some patients responded to the anti-PD-1 therapy, but the rate was low (61). The TMB is associated with genome instability and immunogenicity, and in concert with PD-L1 expression, has been shown to be a useful biomarker for immune check-point inhibitor selection across some cancer types (62–64). Few studies have examined the clinical impact of the TMB in malignant lymphoma, and further research on the prognostic impact of the TMB in lymphoma is needed.
In summary, rrDLBCL is an unresolved issue for DLBCL treatment and has attracted much attention in an attempt to identify rational targets for therapeutic intervention. We conducted targeted sequencing of samples from patients with rrDLBCL to understand better the molecular mechanisms relating to treatment resistance of rrDLBCL and to find candidate genes for the development of new treatments. A limitation of our study is that the number of patients may not have been sufficient to overcome the heterogeneity of the tumors. Despite this limitation, identification of candidate genes through identifying the mutational profile and integrative analysis of mutational evolution provide useful information for understanding the resistance mechanisms of rrDLBCL and for selection of therapeutic targets of rrDLBCL.
Data Availability Statement
The original contributions presented in the study are included in the article/Supplementary Material. Further inquiries can be directed to the corresponding authors.
Ethics Statement
The studies involving human participants were reviewed and approved by Institutional review board of Samsung Medical Center (IRB 2013-12-076-005). The patients/participants provided their written informed consent to participate in this study.
Author Contributions
BL, HL, WSK, and YHK designed the study. BL, HL, JC, SEY, SJK, and W-YP collected and analyzed the data. BL and HL drafted the manuscript. WSK and YHK supervised and edited the manuscript. All authors had full access to all the data in the study and take responsibility for the integrity of the data and the accuracy of the data analysis. All authors contributed to the article and approved the submitted version.
Funding
This work was supported by Intramural Research Program (Samsung 20x20 project) of the Samsung Medical Center [GFO2200161].
Conflict of Interest
The authors declare that the research was conducted in the absence of any commercial or financial relationships that could be construed as a potential conflict of interest.
Supplementary Material
The Supplementary Material for this article can be found online at: https://www.frontiersin.org/articles/10.3389/fonc.2021.628807/full#supplementary-material
References
1. Coiffier B, Thieblemont C, Van Den Neste E, Lepeu G, Plantier I, Castaigne S, et al. Long-term outcome of patients in the LNH-98.5 trial, the first randomized study comparing rituximab-CHOP to standard CHOP chemotherapy in DLBCL patients: a study by the Groupe d’Etudes des Lymphomes de l’Adulte. Blood (2010) 116:2040–5. doi: 10.1182/blood-2010-03-276246
2. Friedberg JW. Relapsed/refractory diffuse large B-cell lymphoma. Hematology (2011) 2011:498–505. doi: 10.1182/asheducation-2011.1.498
3. Gisselbrecht C, Van Den Neste E. How I manage patients with relapsed/refractory diffuse large B cell lymphoma. Br J Haematol (2018) 182:633–43. doi: 10.1111/bjh.15412
4. Alizadeh AA, Eisen MB, Davis RE, Ma C, Lossos IS, Rosenwald A, et al. Distinct types of diffuse large B-cell lymphoma identified by gene expression profiling. Nature (2000) 403:503–11. doi: 10.1038/35000501
5. Lenz G, Wright GW, Emre NC, Kohlhammer H, Dave SS, Davis RE, et al. Molecular subtypes of diffuse large B-cell lymphoma arise by distinct genetic pathways. Proc Natl Acad Sci USA (2008) 105:13520–5. doi: 10.1073/pnas.0804295105
6. Pasqualucci L. Molecular pathogenesis of germinal center-derived B cell lymphomas. Immunol Rev (2019) 288:240–61. doi: 10.1111/imr.12745
7. Lim KH, Yang Y, Staudt LM. Pathogenetic importance and therapeutic implications of NF-kappaB in lymphoid malignancies. Immunol Rev (2012) 246:359–78. doi: 10.1111/j.1600-065X.2012.01105.x
8. Schmitz R, Wright GW, Huang DW, Johnson CA, Phelan JD, Wang JQ, et al. Genetics and Pathogenesis of Diffuse Large B-Cell Lymphoma. N Engl J Med (2018) 378:1396–407. doi: 10.1056/NEJMoa1801445
9. Chapuy B, Stewart C, Dunford AJ, Kim J, Kamburov A, Redd RA, et al. Molecular subtypes of diffuse large B cell lymphoma are associated with distinct pathogenic mechanisms and outcomes. Nat Med (2018) 24:679–90. doi: 10.1038/s41591-018-0016-8
10. Vermaat JS, Pals ST, Younes A, Dreyling M, Federico M, Aurer I, et al. Precision medicine in diffuse large B-cell lymphoma: hitting the target. Haematologica (2015) 100:989–93. doi: 10.3324/haematol.2015.128371
11. Arkenau H-T, Patrikidou A, Flinn I, Hylton JC, Tong S, Ardeshna K. Fostamatinib for the treatment of diffuse large B-cell lymphoma. J Clin Oncol (2020) 38:e20067e. doi: 10.1200/JCO.2020.38.15_suppl.e20067
12. Pontoriero M, Fiume G, Vecchio E, de Laurentiis A, Albano F, Iaccino E, et al. Activation of NF-κB in B cell receptor signaling through Bruton’s tyrosine kinase-dependent phosphorylation of IκB-α. J Mol Med (2019) 97:675–90. doi: 10.1007/s00109-019-01777-x
13. Goy A, Ramchandren R, Ghosh N, Munoz J, Morgan DS, Dang NH, et al. Ibrutinib plus lenalidomide and rituximab has promising activity in relapsed/refractory non–germinal center B-cell–like DLBCL. Blood (2019) 134:1024–36. doi: 10.1182/blood.2018891598
14. Sermer D, Pasqualucci L, Wendel HG, Melnick A, Younes A. Emerging epigenetic-modulating therapies in lymphoma. Nat Rev Clin Oncol (2019) 16:494–507. doi: 10.1038/s41571-019-0190-8
15. Sehn LH, Berry B, Chhanabhai M, Fitzgerald C, Gill K, Hoskins P, et al. The revised International Prognostic Index (R-IPI) is a better predictor of outcome than the standard IPI for patients with diffuse large B-cell lymphoma treated with R-CHOP. Blood (2006) 109:1857–61. doi: 10.1182/blood-2006-08-038257
16. Hans CP, Weisenburger DD, Greiner TC, Gascoyne RD, Delabie J, Ott G, et al. Confirmation of the molecular classification of diffuse large B-cell lymphoma by immunohistochemistry using a tissue microarray. Blood (2004) 103:275–82. doi: 10.1182/blood-2003-05-1545
17. Cibulskis K, Lawrence MS, Carter SL, Sivachenko A, Jaffe D, Sougnez C, et al. Sensitive detection of somatic point mutations in impure and heterogeneous cancer samples. Nat Biotechnol (2013) 31:213–9. doi: 10.1038/nbt.2514
18. Wilm A, Aw PPK, Bertrand D, Yeo GHT, Ong SH, Wong CH, et al. LoFreq: a sequence-quality aware, ultra-sensitive variant caller for uncovering cell-population heterogeneity from high-throughput sequencing datasets. Nucleic Acids Res (2012) 40:11189–201. doi: 10.1093/nar/gks918
19. Shin H-T, Choi Y-L, Yun JW, Kim NK, Kim S-Y, Jeon HJ, et al. Prevalence and detection of low-allele-fraction variants in clinical cancer samples. Nat Commun (2017) 8:1–10. doi: 10.1038/s41467-017-01470-y
20. Ye K, Schulz MH, Long Q, Apweiler R, Ning Z. Pindel: a pattern growth approach to detect break points of large deletions and medium sized insertions from paired-end short reads. Bioinf (Oxford England) (2009) 25:2865–71. doi: 10.1093/bioinformatics/btp394
21. Karczewski KJ, Francioli LC, Tiao G, Cummings BB, Alföldi J, Wang Q, et al. The mutational constraint spectrum quantified from variation in 141,456 humans. Nature (2020) 581:434–43. doi: 10.1038/s41586-020-2308-7
22. Lee S, Seo J, Park J, Nam JY, Choi A, Ignatius JS, et al. Korean Variant Archive (KOVA): a reference database of genetic variations in the Korean population. Sci Rep (2017) 7:4287. doi: 10.1038/s41598-017-04642-4
23. Shin H-T, Kim NKD, Yun JW, Lee B, Kyung S, Lee K-W, et al. Junction Location Identifier (JuLI): Accurate Detection of DNA Fusions in Clinical Sequencing for Precision Oncology. J Mol Diagn (2020) 22:304–18. doi: 10.1016/j.jmoldx.2019.10.015
24. Morin RD, Assouline S, Alcaide M, Mohajeri A, Johnston RL, Chong L, et al. Genetic Landscapes of Relapsed and Refractory Diffuse Large B-Cell Lymphomas. Clin Cancer Res (2016) 22:2290–300. doi: 10.1158/1078-0432.CCR-15-2123
25. Karube K, Enjuanes A, Dlouhy I, Jares P, Martin-Garcia D, Nadeu F, et al. Integrating genomic alterations in diffuse large B-cell lymphoma identifies new relevant pathways and potential therapeutic targets. Leukemia (2018) 32:675–84. doi: 10.1038/leu.2017.251
26. Tate JG, Bamford S, Jubb HC, Sondka Z, Beare DM, Bindal N, et al. COSMIC: the Catalogue Of Somatic Mutations In Cancer. Nucleic Acids Res (2018) 47:D941–D7. doi: 10.1093/nar/gky1015
27. de Miranda NF, Georgiou K, Chen L, Wu C, Gao Z, Zaravinos A, et al. Exome sequencing reveals novel mutation targets in diffuse large B-cell lymphomas derived from Chinese patients. Blood (2014) 124:2544–53. doi: 10.1182/blood-2013-12-546309
28. Brown VL, Harwood CA, Crook T, Cronin JG, Kelsell DP, Proby CM. p16INK4a and p14ARF tumor suppressor genes are commonly inactivated in cutaneous squamous cell carcinoma. J Invest Dermatol (2004) 122:1284–92. doi: 10.1111/j.0022-202X.2004.22501.x
29. Pacifico A, Leone G. Role of p53 and CDKN2A inactivation in human squamous cell carcinomas. J BioMed Biotechnol (2007) 2007:43418. doi: 10.1155/2007/43418
30. Rouault JP, Falette N, Guehenneux F, Guillot C, Rimokh R, Wang Q, et al. Identification of BTG2, an antiproliferative p53-dependent component of the DNA damage cellular response pathway. Nat Genet (1996) 14:482–6. doi: 10.1038/ng1296-482
31. Yuniati L, Scheijen B, van der Meer LT, van Leeuwen FN. Tumor suppressors BTG1 and BTG2: Beyond growth control. J Cell Physiol (2019) 234:5379–89. doi: 10.1002/jcp.27407
32. Khodabakhshi AH, Morin RD, Fejes AP, Mungall AJ, Mungall KL, Bolger-Munro M, et al. Recurrent targets of aberrant somatic hypermutation in lymphoma. Oncotarget (2012) 3:1308–19. doi: 10.18632/oncotarget.653
33. Pasqualucci L, Neumeister P, Goossens T, Nanjangud G, Chaganti RS, Kuppers R, et al. Hypermutation of multiple proto-oncogenes in B-cell diffuse large-cell lymphomas. Nature (2001) 412:341–6. doi: 10.1038/35085588
34. Nijland M, Seitz A, Terpstra M, van Imhoff GW, Kluin PM, van Meerten T, et al. Mutational Evolution in Relapsed Diffuse Large B-Cell Lymphoma. Cancers (Basel) (2018) 10(11):459. doi: 10.3390/cancers10110459
35. Reddy A, Zhang J, Davis NS, Moffitt AB, Love CL, Waldrop A, et al. Genetic and Functional Drivers of Diffuse Large B Cell Lymphoma. Cell (2017) 171:481–94.e15. doi: 10.1016/j.cell.2017.09.027
36. Bosch M, Akhter A, Chen BE, Mansoor A, Lebrun D, Good D, et al. A bioclinical prognostic model using MYC and BCL2 predicts outcome in relapsed/refractory diffuse large B-cell lymphoma. Haematologica (2018) 103:288–96. doi: 10.3324/haematol.2017.179309
37. Rosenwald A, Bens S, Advani R, Barrans S, Copie-Bergman C, Elsensohn MH, et al. Prognostic Significance of MYC Rearrangement and Translocation Partner in Diffuse Large B-Cell Lymphoma: A Study by the Lunenburg Lymphoma Biomarker Consortium. J Clin Oncol (2019) 37:3359–68. doi: 10.1200/JCO.19.00743
38. Liu Y, Barta SK. Diffuse large B-cell lymphoma: 2019 update on diagnosis, risk stratification, and treatment. Am J Hematol (2019) 94:604–16. doi: 10.1002/ajh.25460
39. Lee JH, Jeong H, Choi JW, Oh H, Kim YS. Clinicopathologic significance of MYD88 L265P mutation in diffuse large B-cell lymphoma: a meta-analysis. Sci Rep (2017) 7:1785. doi: 10.1038/s41598-017-01998-5
40. Sonoki T, Harder L, Horsman DE, Karran L, Taniguchi I, Willis TG, et al. Cyclin D3 is a target gene of t ()(p21.1;q32.3) of mature B-cell malignancies. Blood (2001) 98:2837–44. doi: 10.1182/blood.v98.9.2837
41. Karayan L, Riou JF, Seite P, Migeon J, Cantereau A, Larsen CJ. Human ARF protein interacts with topoisomerase I and stimulates its activity. Oncogene (2001) 20:836–48. doi: 10.1038/sj.onc.1204170
42. Jardin F, Jais JP, Molina TJ, Parmentier F, Picquenot JM, Ruminy P, et al. Diffuse large B-cell lymphomas with CDKN2A deletion have a distinct gene expression signature and a poor prognosis under R-CHOP treatment: a GELA study. Blood (2010) 116:1092–104. doi: 10.1182/blood-2009-10-247122
43. Melchardt T, Hufnagl C, Weinstock DM, Kopp N, Neureiter D, Trankenschuh W, et al. Clonal evolution in relapsed and refractory diffuse large B-cell lymphoma is characterized by high dynamics of subclones. Oncotarget (2016) 7:51494–502. doi: 10.18632/oncotarget.9860
44. Peltola KJ, Paukku K, Aho TL, Ruuska M, Silvennoinen O, Koskinen PJ. Pim-1 kinase inhibits STAT5-dependent transcription via its interactions with SOCS1 and SOCS3. Blood (2004) 103:3744–50. doi: 10.1182/blood-2003-09-3126
45. Kim J, Roh M, Abdulkadir SA. Pim1 promotes human prostate cancer cell tumorigenicity and c-MYC transcriptional activity. BMC Cancer (2010) 10:248. doi: 10.1186/1471-2407-10-248
46. Xiang X, Yuan D, Liu Y, Li J, Wen Q, Kong P, et al. PIM1 overexpression in T-cell lymphomas protects tumor cells from apoptosis and confers doxorubicin resistance by upregulating c-myc expression. Acta Biochim Biophys Sin (Shanghai) (2018) 50:800–6. doi: 10.1093/abbs/gmy076
47. van Lohuizen M, Verbeek S, Krimpenfort P, Domen J, Saris C, Radaszkiewicz T, et al. Predisposition to lymphomagenesis in pim-1 transgenic mice: cooperation with c-myc and N-myc in murine leukemia virus-induced tumors. Cell (1989) 56:673–82. doi: 10.1016/0092-8674(89)90589-8
48. Aho TL, Sandholm J, Peltola KJ, Mankonen HP, Lilly M, Koskinen PJ. Pim-1 kinase promotes inactivation of the pro-apoptotic Bad protein by phosphorylating it on the Ser112 gatekeeper site. FEBS Lett (2004) 571:43–9. doi: 10.1016/j.febslet.2004.06.050
49. Acton D, Domen J, Jacobs H, Vlaar M, Korsmeyer S, Berns A. Collaboration of pim-1 and bcl-2 in lymphomagenesis. Curr Top Microbiol Immunol (1992) 182:293–8. doi: 10.1007/978-3-642-77633-5_36
50. Wang Z, Bhattacharya N, Mixter PF, Wei W, Sedivy J, Magnuson NS. Phosphorylation of the cell cycle inhibitor p21Cip1/WAF1 by Pim-1 kinase. Biochim Biophys Acta (BBA) - Mol Cell Res (2002) 1593:45–55. doi: 10.1016/S0167-4889(02)00347-6
51. Morishita D, Katayama R, Sekimizu K, Tsuruo T, Fujita N. Pim kinases promote cell cycle progression by phosphorylating and down-regulating p27Kip1 at the transcriptional and posttranscriptional levels. Cancer Res (2008) 68:5076–85. doi: 10.1158/0008-5472.Can-08-0634
52. Nihira K, Ando Y, Yamaguchi T, Kagami Y, Miki Y, Yoshida K. Pim-1 controls NF-κB signalling by stabilizing RelA/p65. Cell Death Differ (2009) 17:689. doi: 10.1038/cdd.2009.174
53. Feldman BJ, Reid TR, Cleary ML. Pim1 cooperates with E2a-Pbx1 to facilitate the progression of thymic lymphomas in transgenic mice. Oncogene (1997) 15:2735–42. doi: 10.1038/sj.onc.1201670
54. Kuo HP, Ezell SA, Hsieh S, Schweighofer KJ, Cheung LW, Wu S, et al. The role of PIM1 in the ibrutinib-resistant ABC subtype of diffuse large B-cell lymphoma. Am J Cancer Res (2016) 6:2489–501.
55. Jiang Y, Redmond D, Nie K, Eng KW, Clozel T, Martin P, et al. Deep sequencing reveals clonal evolution patterns and mutation events associated with relapse in B-cell lymphomas. Genome Biol (2014) 15:432. doi: 10.1186/s13059-014-0432-0
56. Lohr JG, Stojanov P, Lawrence MS, Auclair D, Chapuy B, Sougnez C, et al. Discovery and prioritization of somatic mutations in diffuse large B-cell lymphoma (DLBCL) by whole-exome sequencing. Proc Natl Acad Sci USA (2012) 109:3879–84. doi: 10.1073/pnas.1121343109
57. Juskevicius D, Jucker D, Klingbiel D, Mamot C, Dirnhofer S, Tzankov A. Mutations of CREBBP and SOCS1 are independent prognostic factors in diffuse large B cell lymphoma: mutational analysis of the SAKK 38/07 prospective clinical trial cohort. J Hematol Oncol (2017) 10:70. doi: 10.1186/s13045-017-0438-7
58. Lunning MA, Green MR. Mutation of chromatin modifiers; an emerging hallmark of germinal center B-cell lymphomas. Blood Cancer J (2015) 5:e361. doi: 10.1038/bcj.2015.89
59. Wise JF, Nakken S, Steen CB, Vodak D, Troen G, Johannessen B, et al. Mutational dynamics and immune evasion in diffuse large B-cell lymphoma explored in a relapse-enriched patient series. Blood Adv (2020) 4:1859–66. doi: 10.1182/bloodadvances.2019001325
60. Song M-K, Park B-B, Uhm J. Understanding immune evasion and therapeutic targeting associated with PD-1/PD-L1 pathway in diffuse large B-cell lymphoma. Int J Mol Sci (2019) 20:1326. doi: 10.3390/ijms20061326
61. Ansell SM, Minnema MC, Johnson P, Timmerman JM, Armand P, Shipp MA, et al. Nivolumab for relapsed/refractory diffuse large B-cell lymphoma in patients ineligible for or having failed autologous transplantation: a single-arm, phase II study. J Clin Oncol (2019) 37:481. doi: 10.1200/JCO.18.00766
62. Fabrizio DA, George TJ Jr., Dunne RF, Frampton G, Sun J, Gowen K, et al. Beyond microsatellite testing: assessment of tumor mutational burden identifies subsets of colorectal cancer who may respond to immune checkpoint inhibition. J Gastrointest Oncol (2018) 9:610. doi: 10.21037/jgo.2018.05.06
63. Galuppini F, Dal Pozzo CA, Deckert J, Loupakis F, Fassan M, Baffa R. Tumor mutation burden: from comprehensive mutational screening to the clinic. Cancer Cell Int (2019) 19:209. doi: 10.1186/s12935-019-0929-4
Keywords: refractory diffuse large B-cell lymphoma, relapsed diffuse large B-cell lymphoma, chemotherapy resistance, tumor evolution, immune evasion, prognostic marker, next-generation sequencing (NGS)
Citation: Lee B, Lee H, Cho J, Yoon SE, Kim SJ, Park W-Y, Kim WS and Ko YH (2021) Mutational Profile and Clonal Evolution of Relapsed/Refractory Diffuse Large B-Cell Lymphoma. Front. Oncol. 11:628807. doi: 10.3389/fonc.2021.628807
Received: 13 November 2020; Accepted: 04 January 2021;
Published: 11 March 2021.
Edited by:
Rakesh Verma, ARMO Biosciences, United StatesReviewed by:
Yang Zhao, Johnson & Johnson Pharmaceutical Research and Development, United StatesTijana Martinov, Fred Hutchinson Cancer Research Center, United States
Thierry Fest, University of Rennes 1, France
Copyright © 2021 Lee, Lee, Cho, Yoon, Kim, Park, Kim and Ko. This is an open-access article distributed under the terms of the Creative Commons Attribution License (CC BY). The use, distribution or reproduction in other forums is permitted, provided the original author(s) and the copyright owner(s) are credited and that the original publication in this journal is cited, in accordance with accepted academic practice. No use, distribution or reproduction is permitted which does not comply with these terms.
*Correspondence: Won Seog Kim, d3NraW1zbWNAc2trdS5lZHU=; Young Hyeh Ko, eWhrbzMxMEBza2t1LmVkdQ==
†These authors have contributed equally to this work and share first authorship