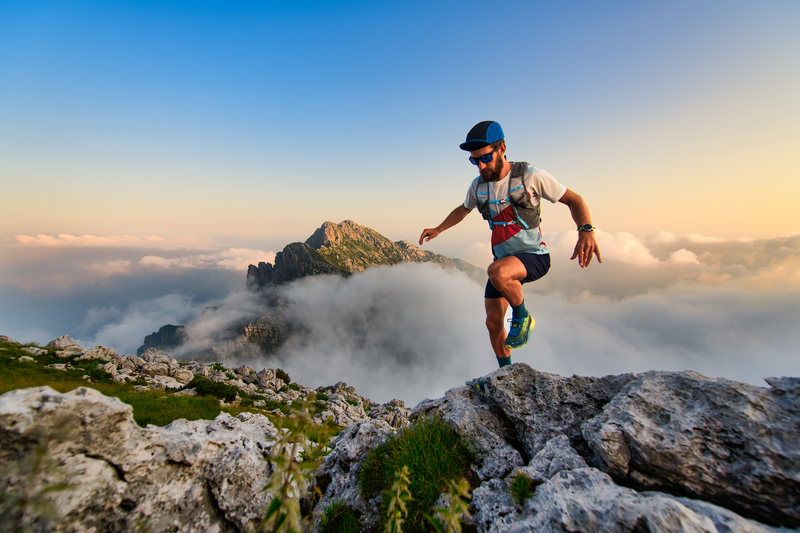
94% of researchers rate our articles as excellent or good
Learn more about the work of our research integrity team to safeguard the quality of each article we publish.
Find out more
ORIGINAL RESEARCH article
Front. Oncol. , 25 March 2021
Sec. Gastrointestinal Cancers
Volume 11 - 2021 | https://doi.org/10.3389/fonc.2021.614398
This article is part of the Research Topic The Evolving Landscape, Clinical Implications and Future Perspective of Biomarkers in Gastrointestinal Cancers View all 19 articles
Background: Identification of a simplified prediction model for lymph node metastasis (LNM) for patients with early colorectal cancer (CRC) is urgently needed to determine treatment and follow-up strategies. Therefore, in this study, we aimed to develop an accurate predictive model for LNM in early CRC.
Methods: We analyzed data from the 2004-2016 Surveillance Epidemiology and End Results database to develop and validate prediction models for LNM. Seven models, namely, logistic regression, XGBoost, k-nearest neighbors, classification and regression trees model, support vector machines, neural network, and random forest (RF) models, were used.
Results: A total of 26,733 patients with a diagnosis of early CRC (T1) were analyzed. The models included 8 independent prognostic variables; age at diagnosis, sex, race, primary site, histologic type, tumor grade, and, tumor size. LNM was significantly more frequent in patients with larger tumors, women, younger patients, and patients with more poorly differentiated tumor. The RF model showed the best predictive performance in comparison to the other method, achieving an accuracy of 96.0%, a sensitivity of 99.7%, a specificity of 92.9%, and an area under the curve of 0.991. Tumor size is the most important features in predicting LNM in early CRC.
Conclusion: We established a simplified reproducible predictive model for LNM in early CRC that could be used to guide treatment decisions. These findings warrant further confirmation in large prospective clinical trials.
Colorectal cancer (CRC) is a major cause of morbidity and mortality worldwide, its importance is expected to continue increasing over time (1, 2). In recent years, increased awareness and the introduction of population-based surveillance and screening programs have led to achieving higher rates of precancerous dysplastic lesions or early CRC detection (3, 4).
Early CRC is a tumor that is confined to the mucosa and/or submucosa regardless of the presence of regional lymph node metastasis (LNM). In certain cases of early CRC, endoscopic resection is a less invasive and cost-effective treatment compared to surgery (5–7). However, the CRC patients with LNM or distant metastasis cannot be adequately cured by local endoscopic treatment alone, and therefore subsequently require surgical resection for achieving a curative treatment.
LNM is found in approximately 6–16% of the patients with submucosal invasive CRC (8–10), however, the number might be underestimated, as clinicians make important treatment decisions based on limited examinations, such as computed tomography (CT) and ultrasonography.
Thus, an accurate and fast assessment of locoregional and/or distant metastases in patients with early CRC is essential to determine whether these patients should undergo additional surgical resections or be needed surveillance regularly. Currently, no universally accepted indications and criteria exist for additional surgical resection after endoscopic resection, even though a fast and accurate assessment of the risk of locoregional LNM after local endoscopic treatment of patients with early CRC is necessary.
Therefore, the aim of present study was to develop a novel prediction model for LNM by using simple histopathological and clinical parameters with high reliability, that can be used to improve patient risk stratification in early CRC.
This study used the Surveillance, Epidemiology, and End Results (SEER) Program database from the National Cancer Institute, which is publicly available U.S. cancer registries. The registry collects and publishes cancer incidence, mortality, and survival data from 17 population-based cancer registries, covering approximately 34.6% of the U.S. population (Iowa, Los Angeles, Connecticut, Utah, Greater California, Idaho, Georgia Center for Cancer Statistics, San Francisco-Oakland, San Jose-Monterey, Louisiana, Hawaii, Massachusetts, Alaska Native tumor registry, Kentucky, New Mexico, New York, Seattle-Puget Sound) (11). The database is roughly represent the U.S. population and includes information on over 9 million cancer cases with over 550,000 new cases added to the database annually. It offers a powerful resource for researchers focused on understanding the natural history of CRC and improving quality healthcare for the patients (11, 12). This retrospective cohort study was evaluated and approved by the Institutional Review Board of the Kyung Hee University Hospital at Gangdong (KHNMC IRB 2020-01-015).
The SEER registry collects data including age at diagnosis, sex, race, primary site, histologic type, tumor grade, tumor size, and tumor depth. Using the SEER 1975–2016 database (released 4/15/2019), we analyzed data from all patients diagnosed with T1 CRC for the years 2004-2016. T1 CRC was defined as infiltration of the tumor into the submucosa. We extracted clinical demographic data, including age at diagnosis, sex, race and tumor information including location, size, grade, histologic type, and American Joint Committee on Cancer 7th TNM stages by using SEER disease codes. Tumor location was determined by using the following codes: C18.0 (cecum); C18.1 (appendix); C18.2 (ascending colon); C18.3 (hepatic flexure); C18.4 (transverse colon); C18.5 (splenic flexure); C18.6 (descending colon); C18.7 (sigmoid colon); C18.8 (overlapping lesion of colon); C18.9 (colon); rectosigmoid (C19.9); and rectum (C20.9). The morphology of cancer was categorized according to the ICD-0-3 histology and behavior codes: 8010/3, (carcinoma, NOS); 8020/3, (carcinoma, undifferentiated, NOS); 8140/3, (adenocarcinoma, NOS); 8144/3, (adenocarcinoma, intestinal type); 8210/3, (adenocarcinoma in adenomatous polyp); 8211/3, (tubular adenocarcinoma); 8255/3, (adenocarcinoma with mixed subtypes); 8261/3, (adenocarcinoma in villous adenoma); 8262/3, (villous adenocarcinoma); 8263/3, (adenocarcinoma in tubulovillous adenoma); 8440/3, (cystadenocarcinoma, NOS); 8470/3, (mucinous cystadenocarcinoma, NOS); 8480/3, (mucinous adenocarcinoma); 8481/3, (mucin-producing adenocarcinoma); 8490/3, (signet ring cell carcinoma); and8221/3, (adenocarcinoma in multiple adenomatous polyps). For tumor differentiation grading, we used a four tier classification including well differentiated, moderately differentiated, poorly differentiated, undifferentiated, which is proposed by WHO grading system (13). In order to exclude potentially confounding factor, the patients who received preoperative radiation treatment were excluded. The overall scheme of the workflow is illustrated in Figure 1.
In this study, we used seven machine-learning (ML) models that are commonly used to predict LNM in patients with early CRC. For the linear model, the logistic regression model (LR) was selected (14). The neural network model (NN), which is one of the important classes of nonlinear prediction models and has been reported in a previous study was used (15). For the kernel-based model, we applied the support vector machine (SVM), which is adopted in many clinical applications (16). For the decision tree approach, the classification and regression trees model (CART), XGBoost (XGB) model and random forest (RF) model, which have also been used in clinical research were included (17–19). Finally, for the basic prediction technique, k-nearest neighbor algorithm (kNN) was selected (20).
We used random oversampling method to improve the classifier performance for the minority classes in our imbalanced classes (21). First, the patients were randomly assigned to a training set (90%) and a test set (10%), where the two class (LNM group vs. non-LNM group) proportions in each set were the same. In the training set, we performed k-fold cross-validation (k = 10), and grid search was used to find the best parameter combinations. For each set of parameters, we fitted the model in turn with 9/10 of data and used 1/10 of data for validation.
To ensure a fair comparison of the models, we used the confusion matrix, area under the curve (AUC), sensitivity (recall), specificity, accuracy, average precision (AP), false positive rate, and precision as performance indicators. We used the AU-ROC as the performance index and the AP value as the criterion for the precision-recall (PR) curve (22). The average value of the parameter was finally executed on the test set.
All data were obtained using the SEER*Stat software (8.3.6 version; Surveillance Research Program, National Cancer Institute). All analyses were performed with Python (version 3.6.9) and R statistical software (version 3.6.0). Demographic differences between the two groups were tested using the Student’s t-test and Pearson chi‐square test. To better evaluate the performance of the models, we used a paired t test to compare the AU-ROC further in each resampling calculation. A two‐sided P ≤ 0.05 was considered statistically significant.
A total of 347,956 patients with CRC between 2004 and 2016 were collected, of which about 292,201 patients were excluded from the study because they were diagnosed with T0 or advanced CRC with or without distant metastasis. After excluding 28,197 patients with insufficient data and 825 patients treated with preoperative radiation therapy, 26,733 patients with a diagnosis of early CRC (T1) were analyzed. The model included eight independent prognostic variables, including age at diagnosis, sex, race, primary site, histologic type, tumor grade, and, tumor size. The analyzed patients were divided into the LNM (2,543 patients, 9.5%) and non-LNM groups (24,190 patients, 90.5%). The younger people (< 60 years) tended to have more LNM at diagnosis compared with the older group (P < 0.001). Significantly higher LNM in women compared with men was observed in the patients with early CRC (P < 0.001). The proportion of LNM in the distal colon included the descending colon, sigmoid colon, and the rectosigmoid junction, was significantly higher than that in the colon proximal to the splenic flexure (P < 0.001). The overall racial and/or ethnic distribution was 69.7% non-Hispanic whites, 11.9% non-Hispanic blacks, 9.0% Hispanics, 8.9% non-Hispanic Asians or Pacific Islander, and 0.5% others (non-Hispanic American, Indian, Alaska natives). Among all patients evaluated, 20.8% (n=5,572) had well differentiated tumor; 71.2% (n=19,026), moderately differentiated; 7.1% (n=1,902), poorly differentiated; 0.9% (n=233), undifferentiated cancer. The mean tumor size was significantly larger in the early CRC patients with LNM than in those of without LNM (22.8mm vs. 20.6 mm) (P < 0.001). Table 1 shows the overall distribution of baseline characteristics of the study population.
We trained the SVM a combination of a C value of 1.0 and a kernel smoothing parameter σ of 0.001. For kNN, a relatively large number of k = 14 was optimal. XGB was performed using the parameters with a maximum depth of 6 and a minimum child weight of 1. For NN, the hyper-parameters were changed during training to obtain the optimal model based on the validation set. The final selected hyper-parameters were a learning rate of 0.001, epoch of 300, hidden layer of 3, dropout rate of 0.3, and batch size of 128. For RF, a relatively large number of randomly selected 61 subtrees provided the best performance.
The average ROC curves and PR curves during the training are shown in Figure 2. Most models had AUC values above 0.81, but the values of LR, XGB, and SVM were lower. The confusion matrix was also calculated for the seven models (Table 2). As shown in Table 2, LR, XGB, and SVM generated a large number of FNs, and kNN and CART models had a large number of FPs during the prediction process. The RF model produced the minimum number of FN (= 5) and FP (= 171). Table 3 shows the AUC, sensitivity, specificity, precision, negative predictive value (NPV), false discovery rate (FDR), accuracy, AP, F1, and Matthews correlation coefficient of each model. The linear model LR showed the worst performance; its accuracy rate was up to 0.60, whereas the accuracy of RF was up to 0.96.
Figure 2 Evaluation of the predictive models. (A) Average ROC curves of seven models. (B) Average PR curves, indicating the tradeoff between precision and recall.
The accuracy of the other models was less than 0.90. RF achieved the highest AUC value of 0.991, and CART had an AU-ROC value of 0.944. LR had the lowest AUC value of 0.623. The RF model showed the best sensitivity and specificity, as well as the best precision, NPV, FDR, accuracy, AP score, F1 score and Matthews correlation coefficient value.
We quantified the variable importance using the coefficients of permutation importance for LNM in each model (Figure 3). For most of the models, the variables including tumor grade, depth of tumor, and age had important influences on the predictability for LNM in early CRC. Based on our quantification, tumor size showed the highest frequency for the top predictors in four of the six models.
Figure 3 Factor importance of the developed models. The (A–F) Bar graphs describe the proportion of importance of the different predictors in the model.
In this study, we established a novel predictive model by combining eight clinicopathologic parameters to predict LNM in early CRC using seven ML models. To the best of our knowledge, this is the first large-scale study to develop a predictive model for LNM by combining easily available simple clinical and pathological data in patients with early CRC. Clinicians are often confronted with the difficulty of selecting candidates who will benefit from surgery after local endoscopic resection.
Currently, in clinical practice, risk stratification in these patients is usually performed by histopathologists carefully analyzing the specimen to determine the risk of LNM, caused by the limited capacity of CT to accurately identify LNM (23).
In previous studies, the pathological factors that showed the strongest independent predictive value for LNM in early CRC are tumor type, poor histological differentiation, and the depth of submucosal invasion (24–27). However, the high interobserver variability in the pathological assessment limits their clinical usefulness and should therefore be interpreted with caution as a univariate marker when deciding whether to proceed with surgery (28, 29). Therefore, the multivariable risk model combining the histopathological data with clinical data can reduce the inaccuracies associated with relying on individual subjective markers and to better define the optimal treatment strategy for early CRC.
With the recent rapid development of computer-aided technology, the application of ML model in cancer diagnosis has an important role; it is being widely used in the medical field with growing trend toward predictive medicine (30–32). We hereby developed an ML model by using the simple clinicopathological parameters in large data, which provided high predictive ability of LNM for patients with early CRC.
To date, a few ML models for prediction of metastasis in patients with early CRC have been developed and evaluated for prognosis and prediction in patients with early CRC (33–36). Ichimasa et al. developed the SVM model with 45 clinicopathologic factors for prediction of LNM in patients with early CRC. They reported that artificial intelligence significantly reduces unnecessary extra surgery after endoscopic resection of T1 CRC without LNM positive in comparison to the current guidelines (33). Another Japanese study showed a deep learning model for predicting LNM from pathology images with cytokeratin immunohistochemistry in early CRC (34). However, these studies were retrospective in nature with single center or small numbers of patients. Due to the low rate of metastasis in early CRC, only a limited number of events exist, leading to limited data. Furthermore, inadequate data could not provide sufficient satisfactory performance under ML algorithms and may have led to lower predictive performance ranging from 0.821 to 0.913, which is less than the result from our RF model. A recent Chinese study also presented a predicting model for LNM that incorporates both the radiomics signature, which combine multiple individual CT imaging features, and several clinical factors using the multivariable logistic regression analysis (35). Although this might be an interesting attempt, the model validity is not guaranteed considering the heterogeneity in the quality of CT image between facilities and its accuracy of approximately 78%, which is lower than the performance of the predictive model we constructed. Lastly, Kudo et al. also employed deep-learning-based modeling to predict LNM in T1 CRC (36). However, they only used NN model for nonlinear dynamic system with smaller sample size than our study and assessed LNM using only CT imaging in the cases treated by endoscopic resection, because pathologic confirmation was not available.
Meanwhile, the reason why the RF model outperforms the other ML algorithms is not easily explained. It might be attributed to that the RF models generally demonstrate the most substantial improvement over linear methods and, might be outperform kernel-based model and neural network model in many categorical variables and some outliers from the nature of large retrospective cohort data. However, to build robust prognostic models for LNM in early CRC, other variables, such as gene expression and histologic image data beyond clinical-pathological variables, should be needed.
In our study, we investigated the variable importance of the predictive models developed, as it could be useful for decision-making by clinicians. Our findings indicated that tumor size was the most important factor for predicting the presence of LNM in early CRC. The prognostic value of tumor size in CRC has long been studied, but no consensus has been reached. Zhang et al. and Kornprat et al. demonstrated a significant association between tumor size and metastasis in CRC (37, 38), whereas Miller et al. indicated no prognostic significance of tumor size in CRC (39). Furthermore, its potential prognostic role in patients with early CRC has not been well investigated. This is the first largest study to identify the prognostic value of tumor size for early CRC and provide statistical evidence for further prospective study. Despite the aforementioned, the current study has several limitations. First, since the SEER database is a nationwide program, several diagnostic criteria, such as histological grades and verification of tumor locations might be subjective, which could cause potential systematic bias. Second, detailed histopathological data, such as lymphovascular invasion, tumor budding, and precise depth of tumor invasion that have been associated with metastasis are insufficient. These data require further assessment to improve the performance of our ML algorithms. Third, our study comprised predominately of white patients; thus, the findings may not be generalized to other racial populations. Finally, the data have a class imbalance problem between the patients with and those without LNM, which means that the rate of LNM is low in early CRC. Therefore, during the tuning process, the parameters had to be further optimizing to avoid overfitting. To further improve the accuracy of the established model, it is necessary to collect more clinical data and further optimizing the parameters are necessary in subsequent studies.
In conclusion, we established and compared seven models to predict metastasis in early CRC by using easily available clinical and histopathological features in real practice. The RF model, a simplified reproducible predictive model, showed the highest predictive power compared with the other models. Tumor size the most important predictor of LNM in early CRC. Therefore, the patients with tumor larger than 3 cm, who were identified as high-risk through the model, may requires careful attention to selection and additional surgical treatment in early CRC. However, because of the limitations inherent in studies based on observational data, these findings should be confirmed in prospective clinical trials.
Publicly available datasets were analyzed in this study. This data can be found here: https://seer.cancer.gov.
MK designed the study. JA, JC and HS analyzed and interpreted the data and wrote the manuscript. JJ and JY supervised the project and revised the paper. All authors contributed to the article and approved the submitted version.
This research was supported by the Basic Science Research Program of the National Research Foundation of Korea (NRF), which is funded by the Korean Ministry of Science, ICT and Future Planning [grant number NRF- 2019R1C1C1003524].
The authors declare that the research was conducted in the absence of any commercial or financial relationships that could be construed as a potential conflict of interest.
1. Bray F, Ferlay J, Soerjomataram I, Siegel RL, Torre LA, Jemal A. Global cancer statistics 2018: GLOBOCAN estimates of incidence and mortality worldwide for 36 cancers in 185 countries. CA Cancer J Clin (2018) 68:394–424. doi: 10.3322/caac.21492
2. Ferlay J, Shin HR, Bray F, Forman D, Mathers C, Parkin DM. Estimates of worldwide burden of cancer in 2008: GLOBOCAN 2008. Int J Cancer (2010) 127:2893–917. doi: 10.1002/ijc.25516
3. Logan RF, Patnick J, Nickerson C, Coleman L, Rutter MD, Von Wagner C, et al. Outcomes of the Bowel Cancer Screening Programme (BCSP) in England after the first 1 million tests. Gut (2012) 61:1439–46. doi: 10.1136/gutjnl-2011-300843
4. Inadomi JM. Screening for Colorectal Neoplasia. N Engl J Med (2017) 376:149–56. doi: 10.1056/NEJMcp1512286
5. Seitz U, Bohnacker S, Seewald S, Thonke F, Brand B, Braiutigam T, et al. Is endoscopic polypectomy an adequate therapy for malignant colorectal adenomas? Presentation of 114 patients and review of the literature. Dis Colon Rectum (2004) 47:1789–96; discussion 1796–87. doi: 10.1007/s10350-004-0680-2
6. Kawamura YJ, Sugamata Y, Yoshino K, Abo Y, Nara S, Sumita T, et al. Endoscopic resection for submucosally invasive colorectal cancer: is it feasible? Surg Endosc (1999) 13:224–7. doi: 10.1007/s004649900949
7. Kashida H, Kudo SE. Early colorectal cancer: concept, diagnosis, and management. Int J Clin Oncol (2006) 11:1–8. doi: 10.1007/s10147-005-0550-5
8. Ricciardi R, Madoff RD, Rothenberger DA, Baxter NN. Population-based analyses of lymph node metastases in colorectal cancer. Clin Gastroenterol Hepatol (2006) 4:1522–7. doi: 10.1016/j.cgh.2006.07.016
9. Tominaga K, Nakanishi Y, Nimura S, Yoshimura K, Sakai Y, Shimoda T. Predictive histopathologic factors for lymph node metastasis in patients with nonpedunculated submucosal invasive colorectal carcinoma. Dis Colon Rectum (2005) 48:92–100. doi: 10.1007/s10350-004-0751-4
10. Bayar S, Saxena R, Emir B, Salem RR. Venous invasion may predict lymph node metastasis in early rectal cancer. Eur J Surg Oncol (2002) 28:413–7. doi: 10.1053/ejso.2002.1254
11. Daly MC, Paquette IM. Surveillance, Epidemiology, and End Results (SEER) and SEER-Medicare Databases: Use in Clinical Research for Improving Colorectal Cancer Outcomes. Clin Colon Rectal Surg (2019) 32:61–8. doi: 10.1055/s-0038-1673355
12. Weiss JM, Pfau PR, O’connor ES, King J, Loconte N, Kennedy G, et al. Mortality by stage for right- versus left-sided colon cancer: analysis of surveillance, epidemiology, and end results–Medicare data. J Clin Oncol (2011) 29:4401–9. doi: 10.1200/JCO.2011.36.4414
13. Nagtegaal ID, Odze RD, Klimstra D, Paradis V, Rugge M, Schirmacher P, et al. The 2019 WHO classification of tumours of the digestive system. Histopathology (2020) 76:182–8. doi: 10.1111/his.13975
14. Menard S. Applied logistic regression analysis. SAGE Publications (2002). doi: 10.4135/9781412983433
15. Nigrin A. Neural networks for pattern recognition. MIT press ACM SIGART Bulletin (1993). doi: 10.1145/182053.1064827
16. Cortes C, Vapnik V. Support-vector networks. Mach Learn (1995) 20:273–97. doi: 10.1007/BF00994018
18. Zhang H, Guo H, Wang J. Research on type 2 diabetes mellitus precise prediction models based on XGBoost algorithm. Chin J Lab Diagn (2018) 22:408–12. doi: 10.3969/j.issn.1007-4287.2018.03.008
19. Marshall RJ. The use of classification and regression trees in clinical epidemiology. J Clin Epidemiol (2001) 54:603–9. doi: 10.1016/S0895-4356(00)00344-9
20. Altman NS. An introduction to kernel and nearest-neighbor nonparametric regression. Am Stat (1992) 46:175–85. doi: 10.1080/00031305.1992.10475879
21. Lemaître G, Nogueira F, Aridas CK. Imbalanced-learn: A python toolbox to tackle the curse of imbalanced datasets in machine learning. J Mach Learn Res (2017) 18:559–63. doi: arXiv:1609.06570
22. Bewick V, Cheek L, Ball J. Statistics review 13: receiver operating characteristic curves. Crit Care (2004) 8:508. doi: 10.1186/cc3000
23. Dighe S, Purkayastha S, Swift I, Tekkis PP, Darzi A, A’hern R, et al. Diagnostic precision of CT in local staging of colon cancers: a meta-analysis. Clin Radiol (2010) 65:708–19. doi: 10.1016/j.crad.2010.01.024
24. Xu F, Xu J, Lou Z, Di M, Wang F, Hu H, et al. Micropapillary component in colorectal carcinoma is associated with lymph node metastasis in T1 and T2 Stages and decreased survival time in TNM stages I and II. Am J Surg Pathol (2009) 33:1287–92. doi: 10.1097/PAS.0b013e3181a5387b
25. Bosch SL, Teerenstra S, De Wilt JH, Cunningham C, Nagtegaal ID. Predicting lymph node metastasis in pT1 colorectal cancer: a systematic review of risk factors providing rationale for therapy decisions. Endoscopy (2013) 45:827–41. doi: 10.1055/s-0033-1344238
26. Glasgow SC, Bleier JI, Burgart LJ, Finne CO, Lowry AC. Meta-analysis of histopathological features of primary colorectal cancers that predict lymph node metastases. J Gastrointestinal Surg (2012) 16:1019–28. doi: 10.1007/s11605-012-1827-4
27. Sun Z-Q, Ma S, Zhou Q-B, Yang S-X, Chang Y, Zeng X-Y, et al. Prognostic value of lymph node metastasis in patients with T1-stage colorectal cancer from multiple centers in China. World J Gastroenterol (2017) 23:8582. doi: 10.3748/wjg.v23.i48.8582
28. Brockmoeller SF, West NP. Predicting systemic spread in early colorectal cancer: Can we do better? World J Gastroenterol (2019) 25:2887–97. doi: 10.3748/wjg.v25.i23.2887
29. Harris EI, Lewin DN, Wang HL, Lauwers GY, Srivastava A, Shyr Y, et al. Lymphovascular invasion in colorectal cancer: an interobserver variability study. Am J Surg Pathol (2008) 32:1816. doi: 10.1097/PAS.0b013e3181816083
30. Kourou K, Exarchos TP, Exarchos KP, Karamouzis MV, Fotiadis DI. Machine learning applications in cancer prognosis and prediction. Comput Struct Biotechnol J (2015) 13:8–17. doi: 10.1016/j.csbj.2014.11.005
31. Statnikov A, Wang L, Aliferis CF. A comprehensive comparison of random forests and support vector machines for microarray-based cancer classification. BMC Bioinf (2008) 9:319. doi: 10.1186/1471-2105-9-319
32. Xiao Y, Wu J, Lin Z, Zhao X. A deep learning-based multi-model ensemble method for cancer prediction. Comput Methods Programs Biomed (2018) 153:1–9. doi: 10.1016/j.cmpb.2017.09.005
33. Ichimasa K, Kudo S-E, Mori Y, Misawa M, Matsudaira S, Kouyama Y, et al. Artificial intelligence may help in predicting the need for additional surgery after endoscopic resection of T1 colorectal cancer. Endoscopy (2018) 50:230–40. doi: 10.1055/s-0043-122385
34. Takamatsu M, Yamamoto N, Kawachi H, Chino A, Saito S, Ueno M, et al. Prediction of early colorectal cancer metastasis by machine learning using digital slide images. Comput Methods Programs Biomed (2019) 178:155–61. doi: 10.1016/j.cmpb.2019.06.022
35. Huang YQ, Liang CH, He L, Tian J, Liang CS, Chen X, et al. Development and Validation of a Radiomics Nomogram for Preoperative Prediction of Lymph Node Metastasis in Colorectal Cancer. J Clin Oncol (2016) 34:2157–64. doi: 10.1200/JCO.2015.65.9128
36. Kudo SE, Ichimasa K, Villard B, Mori Y, Misawa M, Saito S, et al. Artificial Intelligence System to Determine Risk of T1 Colorectal Cancer Metastasis to Lymph Node. Gastroenterology (2020) 160:1075–84. doi: 10.1053/j.gastro.2020.09.027
37. Zhang H, Chen C-S, Cong J-C, Qiao L, Hasegawa T, Takashima S. Clinicopathological characteristics of advanced colorectal cancer 30 mm or smaller in diameter. Chin Med Sci J Chung-kuo i hsueh k’o hsueh tsa chih (2007) 22:98–103.
38. Kornprat P, Pollheimer MJ, Lindtner RA, Schlemmer A, Rehak P, Langner C. Value of tumor size as a prognostic variable in colorectal cancer: a critical reappraisal. Am J Clin Oncol (2011) 34:43–9. doi: 10.1097/COC.0b013e3181cae8dd
Keywords: machine learning, colorectal cancer, prediction, metastasis, model
Citation: Ahn JH, Kwak MS, Lee HH, Cha JM, Shin HP, Jeon JW and Yoon JY (2021) Development of a Novel Prognostic Model for Predicting Lymph Node Metastasis in Early Colorectal Cancer: Analysis Based on the Surveillance, Epidemiology, and End Results Database. Front. Oncol. 11:614398. doi: 10.3389/fonc.2021.614398
Received: 06 October 2020; Accepted: 11 March 2021;
Published: 25 March 2021.
Edited by:
Jaw-Yuan Wang, Kaohsiung Medical University Hospital, TaiwanReviewed by:
Zhangya Pu, Central South University, ChinaCopyright © 2021 Ahn, Kwak, Lee, Cha, Shin, Jeon and Yoon. This is an open-access article distributed under the terms of the Creative Commons Attribution License (CC BY). The use, distribution or reproduction in other forums is permitted, provided the original author(s) and the copyright owner(s) are credited and that the original publication in this journal is cited, in accordance with accepted academic practice. No use, distribution or reproduction is permitted which does not comply with these terms.
*Correspondence: Min Seob Kwak, a3dhYzYzQGtodS5hYy5rcg==
Disclaimer: All claims expressed in this article are solely those of the authors and do not necessarily represent those of their affiliated organizations, or those of the publisher, the editors and the reviewers. Any product that may be evaluated in this article or claim that may be made by its manufacturer is not guaranteed or endorsed by the publisher.
Research integrity at Frontiers
Learn more about the work of our research integrity team to safeguard the quality of each article we publish.