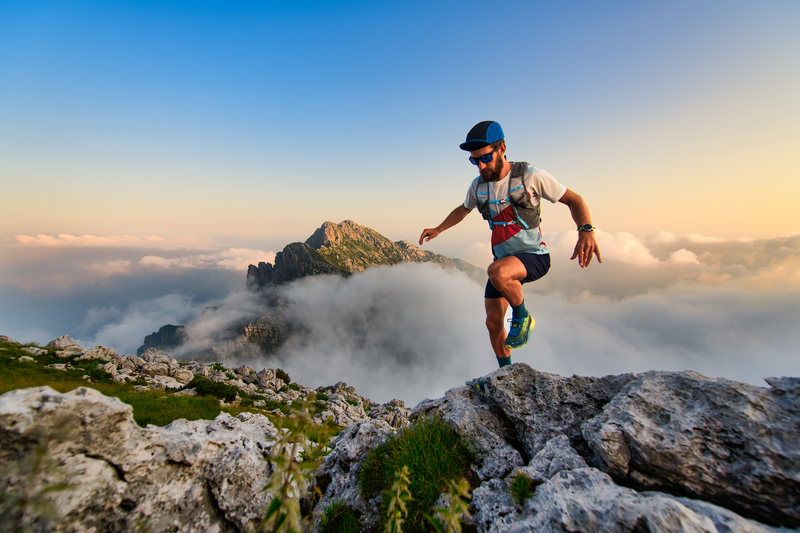
94% of researchers rate our articles as excellent or good
Learn more about the work of our research integrity team to safeguard the quality of each article we publish.
Find out more
ORIGINAL RESEARCH article
Front. Oncol. , 11 May 2021
Sec. Gastrointestinal Cancers
Volume 11 - 2021 | https://doi.org/10.3389/fonc.2021.608641
Background: Solute carrier family 1 member 5 (SLC1A5) is a major glutamine transporter and plays a key role in tumor growth. The main objectives of this study were to visualize the prognostic landscape of SLC1A5 in multiple cancers and determine the relations between SLC1A5 expression and tumor immunity.
Methods: SLC1A5 expression and its effect on tumor prognosis were analyzed using multiple online tools Oncomine, Gene Expression Profiling Interactive Analysis, PrognoScan, and Kaplan-Meier plotter with their own datasets as well as the data from The Cancer Genome Atlas. The correlations between SLC1A5 and tumor immune infiltrates were determined via TIMER.
Results: SLC1A5 expression was significantly higher in several types of cancers, including hepatocellular carcinoma (HCC), compared with corresponding normal tissues. High SLC1A5 expression correlated with poor overall survival and with disease-free survival related to alcohol consumption. Moreover, SLC1A5 expression correlated positively with the numbers of tumor-infiltrating B cells, CD4+ T and CD8+ T cells, macrophages, neutrophils, and dendritic cells in HCC and in lower-grade glioma (LGG). Also, SLC1A5 expression showed strong correlations with diverse immune marker sets in HCC and LGG, indicating its role in regulating tumor immunity.
Conclusions: SLC1A5 represents a useful prognostic biomarker in multiple cancers, and its expression correlates highly with tumor immune-cell infiltration, especially in HCC and LGG.
Hepatocellular carcinoma (HCC) is one of the most common malignancies and ranks third among the causes of cancer-related deaths worldwide, with a 5-year survival rate of < 5% (1, 2). The majority of cases are caused by virus infections, non-alcoholic steatohepatitis, or alcohol-related liver diseases (3–5). Commonly, liver cancer is detected in late stages and thus is difficult to treat, and it does not respond well to traditional chemotherapy. Immune checkpoint therapy has been developed as a new method for treating various cancers, including HCC (6). During the past several years, immunotherapy has achieved impressive results, with promising prospects for immune checkpoint inhibitors (ICIs) in patients with different cancers, including HCC (7–10).
However, only a limited proportion of patients respond well to current immunotherapies (11–13). Recently, high infiltration of immune cells, such as natural killer (NK) cells and CD8+ and CD4+ T effector cells, was reported to be associated with a response to clinical anti-PD1/PD-L1-based therapy (14, 15). Some immune cells in the tumor microenvironment (TME), including dendritic cells (DC), CD8+ and CD4+ T lymphocytes, and NK cells, also play an important role in cancer initiation and progression (16). Thus, there is an urgent need for the elucidation of the molecular mechanisms of cancer immunology including HCC and development of new immune-related therapies for different types of cancers.
Glutamine is a conditionally essential nutrient for rapidly proliferating tumor cells, as glutaminolysis is critical for activation of the mammalian target of the rapamycin complex 1 (mTORC1) nutrient-signaling pathway, which regulates cell growth and protein translation in cancer cells. Recent research indicates that glutamine uptake influences specific immune cell infiltration in breast cancer (17). Also, JHU083 (a glutamine antagonist) can suppress the growth of MYC-driven medulloblastoma (18) and improves the antitumor effects of anti-PD1 therapy in a mouse model (MC38) (19). These studies indicate a plausible prospect for using a combination of a glutamine inhibitor and immune-checkpoint inhibitor to treat cancers.
Solute carrier family 1 member 5 (SLC1A5), also known as alanine–serine-cysteine transporter 2 (ASCT2), is a cell-surface solute-carrying transporter that mediates uptake of neutral amino acids including glutamine and acts as one of the key amino acid transporters. It has received great attention because of its role in supporting tumor metabolism (20). Elevated SLC1A5 expression has been linked to poor survival in many human cancers, including those of the liver (21), lung (22), breast (23), colon (24), and head and neck squamous (25). Although inhibition of SLC1A5 failed to affect HCC proliferation (26), a variant of SLC1A5 was found to be a gatekeeper for glutamine metabolism and metabolic reprogramming in cancer cells (27). Elucidation of the correlation of SLC1A5 with tumor immune-cell infiltration might help in understanding the mechanisms of response and resistance to the respective inhibitors, ultimately paving the way for development of predictive biomarkers and novel treatments.
We performed a systematic analysis of the correlation of SLC1A5 expression with the prognosis of cancer patients attested to in several databases, including Oncomine, PrognoScan, Gene Expression Profiling Interactive Analysis (GEPIA), and Kaplan-Meier plotter. Furthermore, we explored the correlation of SLC1A5 expression with immune infiltration via the Tumor Immune Estimation Resource (TIMER) and GEPIA databases. Our findings showed the prognostic value of SLC1A5 in HCC and lower-grade glioma (LGG) and provided novel insights into the correlation of and mechanism active between SLC1A5 expression and tumor immunity.
The mRNA concentrations of SLC1A5 in diverse types of cancers were identified from the Oncomine database (https://www.oncomine.org/resource/login.html) (28). The threshold for significance was determined according to the following values: p < 0.001, fold change > or = 1.5, and gene ranking of all.
The level 4 gene-expression data (FPKM normalized) of TCGA were downloaded from the UCSC Xena browser (https://gdc.xenahubs.net) (29). The SLC1A5 expression in 24 types of cancers for which expression data from adjacent normal controls were available were first log2(TPM+1)-transformed and then used to assess expression difference by using the Wilcoxon test. The SLC1A5 expression difference between tumor and normal tissues in LGG, ovarian carcinoma (OV), testicular germ cell tumors (TGCT), and uterine carcinosarcoma (UCS) were analyzed in GEPIA (30).
The Kaplan-Meier method was used to assess the correlation of SLC1A5 expression and the overall survival rate in 33 types of cancers using 7,489 cancer samples from TCGA. With multivariate Cox analysis, we evaluated the influence of SLC1A5 expression and other clinical factors on OS in both LGG and HCC. A p value < 0.05 was set as the threshold for significance. The time-dependent receiver operating characteristic (ROC) curve was used to estimate the predictive performance of SLC1A5 expression in LGG and HCC.
Additionally, the correlation between SLC1A5 expression and survival in pan-cancer was analyzed by PrognoScan (http://www.prognoscan.org/) (31). The SLC1A5 expression was searched for all available microarray datasets in PrognoScan to determine its relation to prognosis, such as OS, DSS, relapse-free survival (RFS), and distant metastasis-free survival (DMFS) using Cox p value < 0.05 as the threshold for significance.
Kaplan-Meier plotter (http://kmplot.com/analysis/) was used to analyze the relation between SLC1A5 expression and survival days in liver cancer with different clinicopathologic factors (32), which were assessed by hazard ratio (HR) with 95% confidence intervals and log-rank p values.
The correlation between SLC1A5 expression and the abundance of tumor-infiltrating immune cells (TIICs), including CD4+ cells, CD8+ cells, B cells, neutrophils, macrophages, and DC was analyzed using the TIMER web tool (https://cistrome.shinyapps.io/timer/) (33). The relation between SLC1A5 expression and tumor purity was also determined (34). In addition, the correlation between SLC1A5 expression and gene markers of TIICs, including B cells, CD8+ cells, follicular helper T cells (Tfh), T-helper 1 (Th1) cells, T-helper 2 (Th2) cells, T-helper 9 (Th9) cells, T-helper 17 (Th17) cells, T-helper 22 (Th22) cells, regulatory T cells (Tregs), exhausted T cells, M1 macrophages, M2 macrophages, tumor-associated macrophages (TAM), monocytes, NK cells, neutrophils, and DCs also was explored in LGG and HCC via correlation modules.
Gene set enrichment analysis (GSEA) was performed using normalized gene expression data obtained from TCGA with the R packages clusterProfiler, gerichplot, and ggplot2 (35). The Broad Molecular Signatures Database (MSigDB v 7.1) set C5 was used, which contain genes annotated by the same GO term. Enriched gene sets in SLC1A5 higher expression group were identified using 1,000 permutations of the phenotype labels. Terms with a p value < 0.05 were considered significantly enriched.
All statistical analyses were conducted using R software (Version 3.6.2). The overall survival curves were estimated by the Kaplan-Meier method, and the differences between survival distributions were assessed with the two-sided log-rank test as implemented in the R package survival. A modified drawing survival curve function ‘ggsurvplot,’ as implemented in the R package survminer, was used to draw Kaplan-Meier survival curves. The correlation of gene expression with the measure of interest was evaluated by Spearman’s correlation, and the strength of the correlation was determined using the following criteria for the absolute value: 0.00–0.19 = very weak; 0.20–0.39 = weak; 0.40–0.59 = moderate; 0.60–0.79 = strong; and 0.80–1.0 = very strong. For the comparison of two groups, statistical significance for non-normally distributed variables was analyzed using the Wilcoxon rank-sum test. A p value < 0.05 was considered statistically significant.
The SLC1A5 mRNA concentrations in different tumors and normal tissues linked to multiple cancer types were analyzed in Oncomine. The SLC1A5 mRNA concentrations were significantly higher in the central nervous system (CNS), breast, colorectum, esophagus, stomach, head and neck, leukemia, lung, lymphoma, melanoma, myeloma, and sarcoma tissues compared with the corresponding normal tissues (Figure 1A and Supplementary Table 1). In addition, lower expression of SLC1A5 was observed in breast, esophagus, leukemia, lung, and sarcoma tumors in some datasets.
Figure 1 SLC1A5 expression in cancers. (A) Altered expression in different cancer tissues compared with normal tissues in ONCOMINE. Number in each cell is the number of datasets. (B) Expression in different solid tumor types from TCGA database. *p < 0.05, **p < 0.01, ***p < 0.001. (C) Expression in four complementary solid tumor types from TCGA and GTEx projects in GEPIA.
To determine SLC1A5 expression in cancer in more detail, we examined expression in pan-cancers based on the TCGA database. Under the cutoff value of p < 0.05, no tumor showed significantly lower SLC1A5 expression than its corresponding normal tissue. Meanwhile, SLC1A5 was significantly more abundant in 16 types of tumor tissues, namely cervical squamous-cell carcinoma and endocervical adenocarcinoma (CESC), cholangiocarcinoma (CHOL), colon adenocarcinoma (COAD), esophageal carcinoma (ESCA), glioblastoma multiforme (GBM), head and neck squamous-cell carcinoma (HNSE), kidney chromophobe cancer (KICH), renal clear-cell carcinoma (KIRC), HCC, lung adenocarcinoma (LUAD), lung squamous-cell carcinoma (LUSC), pancreatic adenocarcinoma (PAAD), rectal adenocarcinoma (READ), stomach adenocarcinoma (STAD), thyroid carcinoma (THCA), and uterine corpus endometrial carcinoma (UCEC) than in their corresponding normal tissues (Figure 1B). As shown in Figure 1C, SLC1A5 was significantly overexpressed in LGG but not in OV, TGCT, or UCS.
Next, we investigated the prognostic value of SLC1A5 for pan-cancer recorded in different databases. We used gene expression data from TCGA to assess SLC1A5-related OS in 33 types of cancers with the Kaplan-Meier method. We revealed SLC1A5 to be an adverse prognostic factor in KIRC (p = 0.003), LGG (p < 0.001), HCC (p < 0.001), MESO (p < 0.001), and UVM (p = 0.009) (Figure 2). Conversely, SLC1A5 had a marginally protective role in STAD (p = 0.032) (Figure 2E).
Figure 2 Overall survival (OS) curves comparing high and low expression of SLC1A5 kidney clear-cell carcinoma (KIRC) (A); lower-grade glioma (LGG) (B); hepatocellular carcinoma (HCC) (C); mesothelioma (MESO) (D); stomach adenocarcinoma (STAD) (E), and uveal melanoma (UVM) (F) in TCGA database.
As shown in Figures 3A, B, our multivariate analyses revealed that SLC1A5 expression was an independent factor for prognosis (p < 0.05). SLC1A5 expression showed promising prognostic power, as the AUC for predicting overall survival was 0.727 and 0.717 in LGG and HCC (Figure 3C).
Figure 3 Multivariate Cox analysis of SLC1A5 expression and other clinicopathological variables in LGG (A) and HCC (B). (C) ROC curve of differential SLC1A5 expression in LGG and HCC. OS = overall survival; LGG, lower-grade glioma; HCC, hepatocellular carcinoma; and ROC, receiver operating characteristic.
In PrognoScan, we explored the relation between SLC1A5 expression and the prognosis of each type of cancer. Notably, highly expressed SLC1A5 correlated significantly with poor OS in five cancer types, namely bladder (HR = 1.51; 95% CI 1.04, 2.19; p = 0.0315), blood (HR = 1.69; 95% CI 1.05, 2.73; p = 0.0317), brain (HR = 1.96; 95% CI 1.20, 3.19; p = 0.0069), breast (HR = 1.39; 95% CI 1.15, 1.68; p = 5.9e-5), and skin (HR = 3.87; 95% CI 1.34, 11.14; p = 0.0122). In some datasets, high SLC1A5 expression correlated with a better prognosis in breast (DMFS HR = 0.64; 95% CI 0.42, 0.94; p = 0.0363) and ovarian (OS HR = 0.66; 95% CI 0.47, 0.93; p = 0.0181) (Supplementary Table 2) lesions.
Using the Kaplan-Meier plotter database, we further assessed the prognostic value of SLC1A5 expression in breast, ovarian, lung, and gastric cancer. High SLC1A5 expression was significantly associated with a poor prognosis in breast (OS HR = 1.4; 95% CI 1.13, 1.74; p = 0.002), gastric (OS HR = 1.88; 95% CI 1.58, 2.24; p = 2.7e-13), and lung (OS HR = 1.51; 95% CI 1.33, 1.72; p = 1.6e-10) (Supplementary Figures 1A–G). Meanwhile, high SLC1A5 expression was significantly associated with a better prognosis in ovarian cancer (OS HR = 0.85; 95% CI 0.74, 0.96; p = 0.011) (Supplementary Figure 1H).
To understand better the relevance and underlying mechanisms of SLC1A5 expression in cancers, we assessed the relation between SLC1A5 expression and the clinical characteristics of HCC patients in the Kaplan-Meier plotter database. High SLC1A5 expression correlated with both poor OS (HR = 2.68; p = 0.0035) and PFS (HR = 1.83; p = 0.021) in alcohol consumers (Table 1). Elevated SLC1A5 expression correlated significantly with worse OS in HCC patients with the following clinical characteristics: male, stage 3, grade 2, AJCC_T3 and microvascular invasion, Asian, no alcohol consumption, and without hepatitis virus infection (p < 0.05). These results demonstrated the prognostic significance of SLC1A5 expression in HCC patients based on their clinical characteristics, especially for patients with advanced stages of cancer.
Table 1 Correlation of SLC1A5 mRNA expression and prognosis in hepatocellular carcinoma (HCC) with different clinicopathological factors in Kaplan-Meier plotter.
Considering that the previous study had implicated tumor-infiltrating lymphocytes as independent predictors of sentinel lymph node status and survival in cancer patients (36), we assessed the relation between SLC1A5 expression and infiltrating immune cells in 39 types of cancers including HCC using the TIMER database. The extent of SLC1A5 expression correlated significantly with tumor purity in 20 types of cancer. In addition, SLC1A5 mRNA correlated significantly with the extent of infiltration of B cells in 14 cancer types, CD4+ cells in 15 cancer types, CD8+ cells in 12 cancer types, DC in 20 cancer types, macrophages in 17 cancer types, and neutrophils in 17 cancer types (Supplementary Table 3).
We further observed that high SLC1A5 expression correlated with abundant infiltration of immune cell types in LGG and HCC (Figure 4). For LGG, SLC1A5 expression had significantly positive correlations with the extent of infiltration of B cells (r = 0.556; p = 3.29e-40), CD4+ cells (r = 0.8; p = 3.35e-107), macrophages (r = 0.729; p = 1.10e-79), neutrophils (r = 0.76; p = 2.23e-90), and DC (r = 0.756; p = 2.48e-89). In HCC, high expression of SLC1A5 correlated positively with the extent of infiltration of B cells (r = 0.399; p = 1.38e-14), CD8+ cells (r = 0.395; p = 3.39e-14), CD4+ cells (r = 0.407; p = 3.94e-15), macrophages (r = 0.567; p = 2.17e-30), neutrophils (r = 0.438; p = 1.29e-17), and DC (r = 0.51; p = 6.45e-24). These results indicated that SLC1A5 expression correlated with immune cell infiltration of tissues of LGG and HCC.
Figure 4 Correlation of SLC1A5 expression with immune infiltration in lower-grade glioma (LGG) and hepatocellular carcinoma (HCC). (A) Expression was significantly negatively correlated with tumor purity and had significant positive correlations with numbers of infiltrating B cells, CD4+ cells, macrophages, neutrophils, and DC in LGG but not CD8+ cells (n = 516). (B) Expression was significantly negatively related to tumor purity and had significant positive correlations with numbers of infiltrating B cells, CD8+ cells, CD4+ cells, macrophages, neutrophils, and DC in HCC (n = 371).
To further investigate the potential relation between SLC1A5 and infiltrating immune cells, we examined the correlations between SLC1A5 expression and several immune cell markers in LGG and HCC in the TIMER database. Because the tumor purity of clinical samples influences the analysis of immune infiltration, the correlation analysis was adjusted for purity (Table 2). After this adjustment, SLC1A5 expression correlated significantly with 36 of 45 immune cell markers in HCC and 40 of 45 in LGG (Table 2). Only M1 macrophages in HCC and M2 macrophages in LGG revealed no marker correlated with SLC1A5 expression. These findings confirmed that SLC1A5 expression correlated with immune cell infiltration in LGG and HCC. The strongest correlations were found between SLC1A5 with PU.1 (SPI1) and SLC1A5 and CD86. Interestingly, we found a significantly positive correlation between SLC1A5 expression and prototypical immunotherapy biomarkers, including PD-1 and CTLA4 (Table 2). These findings suggest that SLC1A5 could affect the response to immunotherapy.
GSEA was implemented to reveal differences in enrichment of GO terms in the high SLC1A5-expression group. For the sake of simplicity, only the top five GO terms of high expression in LGG and HCC are listed here on the basis of the normalized enrichment score (NES). In LGG, as shown in Figure 5A, high SLC1A5 phenotype sets were enriched in the top five GO items, namely humoral immune response, production of molecular mediator of immune response, B-cell-mediated immunity, immunoglobulin production, and negative regulation of cellular amide metabolic process. In HCC, five significant GO items also were identified in the SLC1A5 high-expression phenotype, namely immune response-regulating cell-surface receptor signaling pathway, leukocyte migration, negative regulation of immune system process, regulation of lymphocyte activation, and positive regulation of cytokine production (Figure 5B). These results indicate the presence of a strong relation between tumor immunity and SLC1A5 expression in LGG and HCC.
Figure 5 GSEA results showing differential enrichment of genes in GO with high and low SLC1A5 expression in LGG (A) and HCC (B).
Although SLC1A5 has not been well investigated for its role in tumor immunity, it is known that SLC1A5 is required in Th1 and T helper-cell 17 (Th17) induction and is a major regulator of glutamine transport in T lymphocytes (37–39). The current study demonstrated that high SLC1A5 expression correlated significantly with a poor prognosis in bladder and breast cancers, renal clear-cell carcinoma, LGG, HCC, mesothelioma, and uveal melanoma. We further found that high expression of SLC1A5 was especially associated with a poor prognosis of HCC in males, Asians, those without hepatitis viral infections, and those having tumors with micro-vascular invasion. Additionally, SLC1A5 expression correlated significantly with infiltration of multiple immune cells based on the mRNA amounts of markers for different immune cell types in LGG and HCC. Taken together, these findings demonstrated the presence of a strong correlation of SLC1A5 expression with prognosis and tumor immunology in LGG and HCC.
Glutamine is an essential nutrient regulating energy production, redox homeostasis, and signaling in cancer cells. There also are some glutamine inhibitors with plausible clinical application prospects. SLC1A5, a key transporter in charge of moving glutamine across the cytomembrane and mitochondrial membrane, is involved in several cancers (40, 41). Recently, SLC1A5 has received great attention for its membrane protein characteristics and potential role in cancers, which has made it a druggable therapeutic target. Schulte et al. (42) discovered the first small molecule inhibitor targeted at SLC1A5 specifically, named V-9302. Blocking the expression of SLC1A5 through V-9302 reduces cancer cell growth and proliferation and increases cell death and oxidative stress, thereby leading to antitumor efficacy both in vitro and in vivo (39, 42). Other inhibitors of SLC1A5, such as 6-diazo-5-oxo-l-norleucine (DON), benzylserine, and L-γ-glutamyl-p-nitroanilide (GPNA), also showed suppression of tumor growth (22, 43).
The expression of SLC1A5 is upregulated in breast cancer, colon cancer, lung cancer, melanoma, neuroblastoma, glioblastoma, and prostate cancer (40). In this study, we found that SLC1A5 was overexpressed in most cancer tissues compared with normal ones using independent datasets in Oncomine (see Figure 1A). The 17 types of the total 24 tumor types in the TCGA dataset showed significantly higher expression of SLC1A5 than their normal counterparts (Figures 1B, C). Although there exist some variations in different types of cancer, which might be caused by the different data collection criteria used in each study and underlying causative mechanisms, SLC1A5 generally is upregulated in cancers. These cancers with high SLC1A5 expression may be dependent on glutamine and respond to glutamine inhibitors, either alone or in combination with other therapies.
Further, we demonstrated the prognostic value of SLC1A5 in pan-cancer. Prognostic analysis of data from PrognoScan and Kaplan-Meier plotter showed that high SLC1A5 expression correlated with a poor prognosis in bladder, brain, breast, gastric, and skin cancers. Gene expression analysis of the TCGA dataset revealed that high expression of SLC1A5 correlated significantly with a poorer prognosis in KIRC, LGG, HCC, MESO, and UVM. Meanwhile, in STAD, high SLC1A5 correlated with a better prognosis. SLC1A5 expression shows promising prognostic power, as the AUC for predicting overall survival in LGG and HCC was 0.717 and 0.727, respectively (Figure 3). These results strongly highlight the role of upregulated SLC1A5 as a prognostic factor for poor survival in LGG and HCC. Especially, high SLC1A5 expression might impact the prognosis of HCC patients with the following characteristics: male, stage 3, grade 2, AJCC_T3, Asian, and micro-vascular invasion. One possible mechanism for the explanation of the correlation detected between SLC1A5 expression and poor prognosis might be that SLC1A5 contributes to cancer cell uptake of glutamine, which led to activation of the mTORC1 pathway as well as promotion of cancer cell proliferation. Besides, a recently reported novel splice variant of SLC1A5 is a mitochondrial glutamine transporter, which is critical for metabolic reprogramming of cancer cells, thus supporting carcinogenesis (27). Paradoxically, Bothwell et al. have shown that SLC1A5 knockout in human epithelial and mesenchymal HCC cell lines failed to affect cellular proliferation or the mTORCA pathway (26). These results imply that SLC1A5 is nonessential for these cultured cell lines. However, our results clearly indicate that SLC1A5 can serve as a prognostic biomarker in diverse cancers, including HCC.
Another important aspect of this study is that SLC1A5 expression correlated with the extents of multiple immune cell infiltration in cancers, especially LGG and HCC. As shown in Figure 4, our study with the TIMER database demonstrated that SLC1A5 expression had a moderate to strong correlation with all six immune cell-type infiltrations in LGG and HCC, except for CD8+ T cells in LGG. The TME is a complex milieu of non-cancerous cells consisting mainly of immune cells around tumor cells. Genes highly expressed in TME cells are believed to have negative associations with tumor purity. SLC1A5 expression in both LGG and HCC showed significantly negative correlations with their tumor purities, indicating its comparative expression in the TME. Moreover, the correlation between SLC1A5 expression and the marker genes of immune cells implicate SLC1A5 in regulating tumor immunology in LGG and HCC. In both of these cancers, most of the 48 marker genes showed a positive correlation with SLC1A5 expression, with the highest correlation being found between SLC1A5 and a T helper cell 9 (Th9) marker PU.1 (SPI1), which is an oncogene (44). A previous study suggested that Th9 cells play a tumor-promoting role in HCC, and a high infiltrating Th9 number reflects worse survival (45). Additionally, a weak to moderate correlation was found between SLC1A5 expression and the regulation of several markers of other T helper cells (Th1, Th2, Th17, Th22) in LGG and HCC. It has been shown that Th1 cells were significantly associated with a good prognosis in patients with HCC, whereas Th2, TH17, and TH22 cells were related to tumor growth, metastasis, and poor clinical outcomes in HCC (46–49). Furthermore, increased SLC1A5 expression was positively correlated with the expression of regulatory T cells (Tregs) and the induction of T-cell exhaustion markers (CCR8, CD25, PD-1, and CTLA4 in HCC and LGG). Regulatory T cells (Tregs) suppress T-cell immunity in HCC and are associated with a bad prognosis (50) and T-cell exhaustion leading to immune escape represents one of the mechanisms for cancer cells to get rid of control from the immune system (51). CD8+ T-cell markers showed a significantly positive correlation with SLC1A5 expression in HCC. The general consensus is that tumors with CD8+ T cell infiltration could be triggered to some level of antitumor immunity by anti–PD-1 therapies (52). Previous research has showed that in SLC1A5-deficient T cells, glutamine uptake and mTORC1 activation on TCR engagement were largely impaired, and the polarization of Th1 and Th17 were also blocked (53). This might in part explain the widely positive correlations between SLC1A5 and T cells (54). Moreover, the SLC1A5 expression was found to be positively correlated with several gene markers for monocyte, macrophage, natural killer cell (NK), neutrophil, and DC. Monocyte and macrophage can induce NK cell dysfunction in advanced-stage HCC (55). While DC was found with at least three subtypes, DC vaccine could improve PD-1 inhibitor therapeutic effect in a HCC mouse model (56). Our findings suggest that SLC1A5 correlates positively with these immune cells, while the specific biological functions and processes need to be further explored. Furthermore, we searched for GO term enriched in SLC1A5-overexpressing datasets and found that upregulated SLC1A5 expression was primarily linked with immune cell and immune process in LGG and HCC. These results support the idea that high expression of SLC1A5 in patients with LGG and HCC could change their tumor immunology, which may eventually influence patient survival. Previous studies have provided possible mechanisms that can explain why SLC1A5 expression correlates positively with immune cell infiltration and a poor prognosis. Glutamine, as an immunomodulatory nutrient and fuel for tumor cells, is transported by SLC1A5. In addition, the consequence of glutamine metabolism in cancer cells is the creation of a hypoxic, acidic, and nutrient-depleted tumor microenvironment (TME) (19, 57). The tumor immune microenvironment in HCC has been characterized as shifted from help resisting tumors toward immunosuppression (58). The second-highest correlation was found between SLC1A5 expression and CD86, a marker gene of tumor-associated macrophages with the ability to capture PD1-targeting antibodies on its surface and consequently limiting immunotherapeutic efficacy (59). In particular, the expression of SLC1A5 correlates significantly with the prototypical immunotherapeutic gene biomarkers, including PD-1 and CTLA4. These correlations collectively indicate a potential role for SLC1A5 expression as a biomarker to predict a clinical benefit of using immune-checkpoint inhibitors to treat cancers, especially LGG and HCC. Future investigation is needed to realize the definitive clinical value of SLC1A5 as predictive markers in immunotherapy.
Powell and colleagues reported that combining JHU83 with anti-PD1 antibody can dramatically improve anti-PD1 antitumor effects compared with anti-PD1 therapy alone (19, 60). Two Phase II clinical trials have been conducted that combined glutamine and a glutamate pathway inhibitor with immune-checkpoint inhibitors. As a potential target in abnormal metabolism of glutamine, SLC1A5 has an outstanding significance for combination therapy studies.
Even though we integrated data across multiple databases, this study had a few limitations. First, the correlation of SLC1A5 expression and immunity in cancer was analyzed on the basis of publicly available expression datasets. Thus, some experiments need to be performed in vivo/in vitro, and single-cell RNA sequencing should be carried out to clarify the different role of SLC1A5 in immune and cancer cells. Second, despite the finding that SLC1A5 expression correlates significantly with immune cell infiltration in LGG and HCC, there was no database reflecting the correlation of SLC1A5 expression and immunotherapeutic response in these cancer types. Future clinical trials focusing on SLC1A5 expression and immunotherapeutic response in patients with LGG or HCC can help reach a direct conclusion. Finally, combining Leone’s report (19) and our results, we think that it is necessary to investigate the clinical value of a combined SLC1A5 inhibitor (or glutamine inhibitor, alternatively) and an immune-checkpoint inhibitor for treating LGG or HCC patients.
In summary, our results demonstrate that SLC1A5 is a powerful independent prognostic biomarker for multiple cancers. Specially for LGG and HCC, SLC1A5 can be used to evaluate the extent of immune cell infiltration in the tumor tissues. On the basis of these findings, we suggest further research on the subject to improve understanding of the immunotherapeutic impact of SLC1A5.
The original contributions presented in the study are included in the article/Supplementary Material. Further inquiries can be directed to the corresponding authors.
JZ, ZY, MT, WM, and HG participated in literature search and data collection. JZ and ZY conducted the data analysis. JZ, LL, and MDL participated in writing of the manuscript. MDL conceived the study and was involved in every step of the work. All authors contributed to the article and approved the submitted version.
This study was supported by the National Science and Technology Major Project of China (2018ZX10302206-001-009), the China Precision Medicine Initiative (2016YFC0906300), Major Program of National Natural Science Foundation of China (81790634), Research Center for Air Pollution and Health of Zhejiang University, and State Key Laboratory for Diagnosis and Treatment of Infectious Diseases of the First Affiliated Hospital of Zhejiang University.
The authors declare that the research was conducted in the absence of any commercial or financial relationships that could be construed as a potential conflict of interest.
The Supplementary Material for this article can be found online at: https://www.frontiersin.org/articles/10.3389/fonc.2021.608641/full#supplementary-material
1. Gao Q, Zhu H, Dong L, Shi W, Chen R, Song Z, et al. Integrated Proteogenomic Characterization of HBV-Related Hepatocellular Carcinoma. Cell (2019) 179(2):1240. doi: 10.1016/j.cell.2019.10.038
2. Chemin I, Zoulim F. Hepatitis B Virus Induced Hepatocellular Carcinoma. Cancer Lett (2009) 286(1):52–9. doi: 10.1016/j.canlet.2008.12.003
3. El-Serag HB. Hepatocellular Carcinoma. N Engl J Med (2011) 365(12):1118. doi: 10.1056/NEJMra1001683
4. Welzel TM, Graubard BI, Quraishi SM, Zeuzem S, Davila JA, Elserag HB, et al. Population-Attributable Fractions of Risk Factors for Hepatocellular Carcinoma in the United States. Am J Gastroenterol (2013) 108(8):1314–21. doi: 10.1038/ajg.2013.160
5. Kremsdorf D, Soussan P, Paterlinibrechot P, Brechot C. Hepatitis B Virus-Related Hepatocellular Carcinoma: Paradigms for Viral-Related Human Carcinogenesis. Oncogene (2006) 25(27):3823–33. doi: 10.1038/sj.onc.1209559
6. Yau T, Hsu C, Kim T, Choo SP, Kang Y, Hou M, et al. Nivolumab in Advanced Hepatocellular Carcinoma: Sorafenib-experienced Asian Cohort Analysis. J Hepatol (2019) 71(3):543–52. doi: 10.1016/j.jhep.2019.05.014
7. Longo V, Gnoni A, Gardini AC, Pisconti S, Licchetta A, Scartozzi M, et al. Immunotherapeutic Approaches for Hepatocellular Carcinoma. Oncotarget (2017) 8(20):33897–910. doi: 10.18632/oncotarget.15406
8. Borghaei H, Langer CJ, Gadgeel SM, Papadimitrakopoulou VA, Patnaik A, Powell SF, et al. 24-Month Overall Survival From KEYNOTE-021 Cohort G: Pemetrexed and Carboplatin With or Without Pembrolizumab as First-Line Therapy for Advanced Nonsquamous Non–Small Cell Lung Cancer. J Thorac Oncol (2019) 14(1):124–9. doi: 10.1016/j.jtho.2018.08.004
9. Siefkerradtke AO, Apolo AB, Bivalacqua TJ, Spiess PE, Black PC. Immunotherapy With Checkpoint Blockade in the Treatment of Urothelial Carcinoma. J Urol (2017) 199(5):1129–42. doi: 10.1016/j.juro.2017.10.041
10. Peinemann F, Unverzagt S, Hadjinicolaou AV, Moldenhauer I. Immunotherapy for Metastatic Renal Cell Carcinoma: A Systematic Review. J Evid Based Med (2019) 12(4):253–62. doi: 10.1111/jebm.12362
11. Topalian SL, Drake CG, Pardoll DM. Immune Checkpoint Blockade: A Common Denominator Approach to Cancer Therapy. Cancer Cell (2015) 27(4):450–61. doi: 10.1016/j.ccell.2015.03.001
12. Kudo M. Immune Checkpoint Inhibition in Hepatocellular Carcinoma: Basics and Ongoing Clinical Trials. Oncology (2017) 92(1):50–62. doi: 10.1159/000451016
13. Yau T, Kang Y, Kim T, Elkhoueiry AB, Santoro A, Sangro B, et al. Nivolumab (NIVO) + Ipilimumab (IPI) Combination Therapy in Patients (Pts) With Advanced Hepatocellular Carcinoma (aHCC): Results From CheckMate 040. J Clin Oncol (2019) 37:4012–. doi: 10.1200/JCO.2019.37.15_suppl.4012
14. Hsu J, Hodgins JJ, Marathe M, Nicolai CJ, Bourgeoisdaigneault M, Trevino TN, et al. Contribution of NK Cells to Immunotherapy Mediated by PD-1/PD-L1 Blockade. J Clin Invest (2018) 128(10):4654–68. doi: 10.1172/JCI99317
15. Tang H, Wang Y, Chlewicki LK, Zhang Y, Guo J, Liang W, et al. Facilitating T Cell Infiltration in Tumor Microenvironment Overcomes Resistance to PD-L1 Blockade. Cancer Cell (2016) 29(3):285–96. doi: 10.1016/j.ccell.2016.02.004
16. Garner H, de Visser KE. Immune Crosstalk in Cancer Progression and Metastatic Spread: A Complex Conversation. Nat Rev Immunol (2020) 20(8):483–97. doi: 10.1038/s41577-019-0271-z
17. Ansari RE, Craze ML, Althobiti M, Alfarsi L, Ellis IO, Rakha EA, et al. Enhanced Glutamine Uptake Influences Composition of Immune Cell Infiltrates in Breast Cancer. Br J Cancer (2020) 122(1):94–101. doi: 10.1038/s41416-019-0626-z
18. Hanaford A, Alt J, Rais R, Wang S, Kaur H, Thorek DLJ, et al. Orally Bioavailable Glutamine Antagonist Prodrug JHU-083 Penetrates Mouse Brain and Suppresses the Growth of MYC-driven Medulloblastoma. Trans Oncol (2019) 12(10):1314–22. doi: 10.1016/j.tranon.2019.05.013
19. Leone R, Zhao L, Englert J, Sun IM, Oh MH, Sun IH, et al. Glutamine Blockade Induces Divergent Metabolic Programs to Overcome Tumor Immune Evasion. Science (2019) 366(6468):1013–21. doi: 10.1126/science.aav2588
20. Cormerais Y, Massard PA, Vucetic M, Giuliano S, Tambutté E, Durivault J, et al. The glutamine transporter ASCT2 (SLC1A5) promotes tumor growth independently of the amino acid transporter LAT1 (SLC7A5)s. J Biol Chem (2018) 293(8):2877–87. doi: 10.1074/jbc.RA117.001342
21. Sun H, Yu X, Wu W, Chen J, Shi M, Zheng L, et al. GLUT1 and ASCT2 as Predictors for Prognosis of Hepatocellular Carcinoma. PloS One (2016) 11(12):e0168907. doi: 10.1371/journal.pone.0168907
22. Hassanein M, Hoeksema M, Shiota M, Qian J, Harris BK, Chen H, et al. SLC1A5 Mediates Glutamine Transport Required for Lung Cancer Cell Growth and Survival. Clin Cancer Res (2013) 19(3):560–70. doi: 10.1158/1078-0432.CCR-12-2334
23. van Geldermalsen M, Wang Q, Nagarajah R, Marshall AD, Thoeng A, Gao D, et al. ASCT2/SLC1A5 Controls Glutamine Uptake and Tumour Growth in Triple-Negative Basal-Like Breast Cancer. Oncogene (2016) 35(24):3201–8. doi: 10.1038/onc.2015.381
24. Schulte ML, Hight MR, Ayers GD, Liu Q, Shyr Y, Washington MK, et al. Non-Invasive Glutamine PET Reflects Pharmacological Inhibition of BRAF(V600E) In Vivo. Mol Imaging Biol (2017) 19(3):421–8. doi: 10.1007/s11307-016-1008-z
25. Zhang Z, Liu R, Shuai Y, Huang Y, Jin R, Wang X, et al. ASCT2 (SLC1A5)-Dependent Glutamine Uptake is Involved in the Progression of Head and Neck Squamous Cell Carcinoma. Br J Cancer (2020) 122(1):82–93. doi: 10.1038/s41416-019-0637-9
26. Bothwell P, Kron C, Wittke E, Czerniak BN, Bode BP. Targeted Suppression and Knockout of ASCT2 or LAT1 in Epithelial and Mesenchymal Human Liver Cancer Cells Fail to Inhibit Growth. Int J Mol Sci (2018) 19(7):2093. doi: 10.3390/ijms19072093
27. Yoo HC, Park SJ, Nam M, Kang JM, Kim K, Yeo JH, et al. A Variant of SLC1A5 is a Mitochondrial Glutamine Transporter for Metabolic Reprogramming in Cancer Cells. Cell Metab (2020) 31(2):267. doi: 10.1016/j.cmet.2019.11.020
28. Rhodes DR, Kalyana-Sundaram S, Mahavisno V, Varambally R, Yu J, Briggs BB, et al. Oncomine 3.0: Genes, Pathways, and Networks in a Collection of 18,000 Cancer Gene Expression Profiles. Neoplasia (2007) 9(2):166–80. doi: 10.1593/neo.07112
29. Blum A, Wang PI, Zenklusen JC. Snapshot: TCGA-Analyzed Tumors. Cell (2018) 173(2):530. doi: 10.1016/j.cell.2018.03.059
30. Tang Z, Li C, Kang B, Gao G, Li C, Zhang Z. GEPIA: A Web Server for Cancer and Normal Gene Expression Profiling and Interactive Analyses. Nucleic Acids Res (2017) 45(W1):W98–W102. doi: 10.1093/nar/gkx247
31. Mizuno H, Kitada K, Nakai K, Sarai A. PrognoScan: A New Database for Meta-Analysis of the Prognostic Value of Genes. BMC Med Genomics (2009) 2(1):18. doi: 10.1186/1755-8794-2-18
32. Lánczky A, Nagy Á, Bottai G, Munkácsy GN, Szabó A, Santarpia L, et al. miRpower: A Web-Tool to Validate Survival-Associated miRNAs Utilizing Expression Data From 2178 Breast Cancer Patients. Breast Cancer Res Treat (2016) 160(3):439–46. doi: 10.1007/s10549-016-4013-7
33. Li T, Fan J, Wang B, Traugh N, Chen Q, Liu JS, et al. Timer: A Web Server for Comprehensive Analysis of Tumor-Infiltrating Immune Cells. Cancer Res (2017) 77(21):e108–e10. doi: 10.1158/0008-5472.CAN-17-0307
34. Li B, Severson E, Pignon J, Zhao H, Li T, Novak J, et al. Comprehensive Analyses of Tumor Immunity: Implications for Cancer Immunotherapy. Genome Biol (2016) 17(1):174. doi: 10.1186/s13059-016-1028-7
35. Subramanian A, Tamayo P, Mootha VK, Mukherjee S, Ebert BL, Gillette MA, et al. Gene Set Enrichment Analysis: A Knowledge-Based Approach for Interpreting Genome-Wide Expression Profiles. Proc Natl Acad Sci USA (2005) 102(43):15545–50. doi: 10.1073/pnas.0506580102
36. Azimi F, Scolyer RA, Rumcheva P, Moncrieff M, Murali R, Mccarthy SW, et al. Tumor-Infiltrating Lymphocyte Grade Is an Independent Predictor of Sentinel Lymph Node Status and Survival in Patients With Cutaneous Melanoma. J Clin Oncol (2012) 30(21):2678–83. doi: 10.1200/JCO.2011.37.8539
37. Koeken VACM, Lachmandas E, Riza A, Matzaraki V, Li Y, Kumar V, et al. Role of Glutamine Metabolism in Host Defense Against Mycobacterium Tuberculosis Infection. J Infect Dis (2018) 219(10):1662–70. doi: 10.1093/infdis/jiy709
38. Song W, Li D, Tao L, Luo Q, Chen L. Solute Carrier Transporters: The Metabolic Gatekeepers of Immune Cells. Acta Pharm Sin B (2020) 10(1):61–78. doi: 10.1016/j.apsb.2019.12.006
39. Schulte ML, Fu A, Zhao P, Li J, Geng L, Smith ST, et al. Pharmacological Blockade of ASCT2-dependent Glutamine Transport Leads to Antitumor Efficacy in Preclinical Models. Nat Med (2018) 24(2):194–202. doi: 10.1038/nm.4464
40. Liu Y, Zhao T, Li Z, Wang L, Yuan S, Sun L. The Role of ASCT2 in Cancer: A Review. Eur J Pharmacol (2018) 837:81–7. doi: 10.1016/j.ejphar.2018.07.007
41. Willems L, Jacque N, Jacquel A, Neveux N, Maciel TT, Lambert M, et al. Inhibiting Glutamine Uptake Represents an Attractive New Strategy for Treating Acute Myeloid Leukemia. Blood (2013) 122(20):3521–32. doi: 10.1182/blood-2013-03-493163
42. Schulte ML, Khodadadi AB, Cuthbertson ML, Smith JA, Manning HC. 2-Amino-4-bis(aryloxybenzyl)aminobutanoic Acids: A Novel Scaffold for Inhibition of ASCT2-mediated Glutamine Transport. Bioorg Med Chem Lett (2016) 26(3):1044–7. doi: 10.1016/j.bmcl.2015.12.031
43. Hassanein M, Qian J, Hoeksema M, Wang J, Jacobovitz M, Ji X, et al. Targeting SLC1a5-mediated Glutamine Dependence in non-Small Cell Lung Cancer. Int J Cancer (2015) 137(7):1587–97. doi: 10.1002/ijc.29535
44. Klemsz MJ, Mckercher SR, Celada A, Van Beveren C, Maki RA. The Macrophage and B Cell-Specific Transcription Factor PU.1 is Related to the Ets Oncogene. Cell (1990) 61(1):113–24. doi: 10.1016/0092-8674(90)90219-5
45. Tan H, Wang S, Zhao L. A Tumour-Promoting Role of Th9 Cells in Hepatocellular Carcinoma Through CCL20 and STAT3 Pathways. Clin Exp Pharmacol Physiol (2017) 44(2):213–21. doi: 10.1111/1440-1681.12689
46. Budhu A, Forgues M, Ye Q-H, Jia H-L, He P, Zanetti KA, et al. Prediction of Venous Metastases, Recurrence, and Prognosis in Hepatocellular Carcinoma Based on a Unique Immune Response Signature of the Liver Microenvironment. Cancer Cell (2006) 10(2):99–111. doi: 10.1016/j.ccr.2006.06.016
47. Mantovani A, Allavena P, Sica A, Balkwill F. Cancer-Related Inflammation. Nature (2008) 454(7203):436–44. doi: 10.1038/nature07205
48. Zhang J-P, Yan J, Xu J, Pang X-H, Chen M-S, Li L, et al. Increased Intratumoral IL-17-producing Cells Correlate With Poor Survival in Hepatocellular Carcinoma Patients. J Hepatol (2009) 50(5):980–9. doi: 10.1016/j.jhep.2008.12.033
49. Qin S, Ma S, Huang X, Lu D, Zhou Y, Jiang H. Th22 Cells are Associated With Hepatocellular Carcinoma Development and Progression. Chin J Cancer Res (2014) 26(2):135–41. doi: 10.3978/j.issn.1000-9604.2014.02.14
50. Langhans B, Nischalke HD, Krämer B, Dold L, Lutz P, Mohr R, et al. Role of Regulatory T Cells and Checkpoint Inhibition in Hepatocellular Carcinoma. Cancer Immunol Immunother (2019) 68(12):2055–66. doi: 10.1007/s00262-019-02427-4
51. Wang J-C, Xu Y, Huang Z-M, Lu X-J. T Cell Exhaustion in Cancer: Mechanisms and Clinical Implications. J Cell Biochem (2018) 119(6):4279–86. doi: 10.1002/jcb.26645
52. Ott PA, Hodi FS, Kaufman HL, Wigginton JM, Wolchok JD. Combination Immunotherapy: A Road Map. J Immunother Cancer (2017) 5:16–. doi: 10.1186/s40425-017-0218-5
53. Nakaya M, Xiao Y, Zhou X, Chang J-H, Chang M, Cheng X, et al. Inflammatory T Cell Responses Rely on Amino Acid Transporter ASCT2 Facilitation of Glutamine Uptake and mTORC1 Kinase Activation. Immunity (2014) 40(5):692–705. doi: 10.1016/j.immuni.2014.04.007
54. Ren W, Liu G, Yin J, Tan B, Wu G, Bazer FW, et al. Amino-Acid Transporters in T-cell Activation and Differentiation. Cell Death Dis (2017) 8(3):e2655–e. doi: 10.1038/cddis.2016.222
55. Wu Y, Kuang D-M, Pan W-D, Wan Y-L, Lao X-M, Wang D, et al. Monocyte/Macrophage-Elicited Natural Killer Cell Dysfunction in Hepatocellular Carcinoma is Mediated by CD48/2B4 Interactions. Hepatology (2013) 57(3):1107–16. doi: 10.1002/hep.26192
56. Teng C-F, Wang T, Shih F-Y, Shyu W-C, Jeng L-B. Therapeutic Efficacy of Dendritic Cell Vaccine Combined With Programmed Death 1 Inhibitor for Hepatocellular Carcinoma. J Gastroenterol Hepatol (2021). doi: 10.1111/jgh.15398
57. Shevchenko I, Bazhin AV. Metabolic Checkpoints: Novel Avenues for Immunotherapy of Cancer. Front Immunol (2018) 9:1816. doi: 10.3389/fimmu.2018.01816
58. Cancer Genome Atlas Research Network. Electronic Address Wbe, Cancer Genome Atlas Research N. Comprehensive and Integrative Genomic Characterization of Hepatocellular Carcinoma. Cell (2017) 169(7):1327–41.e23. doi: 10.1016/j.cell.2017.05.046
59. Arlauckas SP, Garris CS, Kohler RH, Kitaoka M, Cuccarese MF, Yang KS, et al. In Vivo Imaging Reveals a Tumor-Associated Macrophage–Mediated Resistance Pathway in Anti–PD-1 Therapy. Sci Trans Med (2017) 9(389):eaal3604. doi: 10.1126/scitranslmed.aal3604
Keywords: glutamine, hepatocellular carcinoma, immune cell infiltration, lower-grade glioma, prognosis determination, SLC1A5
Citation: Zhao J, Yang Z, Tu M, Meng W, Gao H, Li MD and Li L (2021) Correlation Between Prognostic Biomarker SLC1A5 and Immune Infiltrates in Various Types of Cancers Including Hepatocellular Carcinoma. Front. Oncol. 11:608641. doi: 10.3389/fonc.2021.608641
Received: 05 November 2020; Accepted: 13 April 2021;
Published: 11 May 2021.
Edited by:
Yumin Li, Lanzhou University, ChinaReviewed by:
Gopi Sundaram, Central Food Technological Research Institute (CSIR), IndiaCopyright © 2021 Zhao, Yang, Tu, Meng, Gao, Li and Li. This is an open-access article distributed under the terms of the Creative Commons Attribution License (CC BY). The use, distribution or reproduction in other forums is permitted, provided the original author(s) and the copyright owner(s) are credited and that the original publication in this journal is cited, in accordance with accepted academic practice. No use, distribution or reproduction is permitted which does not comply with these terms.
*Correspondence: Ming D. Li, bWwya21Aemp1LmVkdS5jbg==; Lanjuan Li, bGpsaUB6anUuZWR1LmNu
Disclaimer: All claims expressed in this article are solely those of the authors and do not necessarily represent those of their affiliated organizations, or those of the publisher, the editors and the reviewers. Any product that may be evaluated in this article or claim that may be made by its manufacturer is not guaranteed or endorsed by the publisher.
Research integrity at Frontiers
Learn more about the work of our research integrity team to safeguard the quality of each article we publish.