- 1Sino-German Tongji-Caritas Research Center of Ultrasound in Medicine, Department of Medical Ultrasound, Tongji Hospital, Tongji Medical College, Huazhong University of Science and Technology, Wuhan, China
- 2Department of Artificial Intelligence, Julei Technology Company, Wuhan, China
- 3Department of Ultrasound, Affiliated Renhe Hospital of China Three Gorges University, Yichang, China
- 4Department of Ultrasound, The First Affiliated Hospital of Zhengzhou University, Zhengzhou, China
- 5Department of Ultrasound, Wuchang Hospital, Wuhan University of Science and Technology, Wuhan, China
- 6Department of Ultrasonic Imaging, Xiangya Hospital, Central South University, Changsha, China
- 7Department of General Internal Medicine, Kliniken Hirslanden Beau-Site, Bern, Switzerland
Objective: The purpose of this study was to improve the differentiation between malignant and benign thyroid nodules using deep learning (DL) in category 4 and 5 based on the Thyroid Imaging Reporting and Data System (TI-RADS, TR) from the American College of Radiology (ACR).
Design and Methods: From June 2, 2017 to April 23, 2019, 2082 thyroid ultrasound images from 1396 consecutive patients with confirmed pathology were retrospectively collected, of which 1289 nodules were category 4 (TR4) and 793 nodules were category 5 (TR5). Ninety percent of the B-mode ultrasound images were applied for training and validation, and the residual 10% and an independent external dataset for testing purpose by three different deep learning algorithms.
Results: In the independent test set, the DL algorithm of best performance got an AUC of 0.904, 0.845, 0.829 in TR4, TR5, and TR4&5, respectively. The sensitivity and specificity of the optimal model was 0.829, 0.831 on TR4, 0.846, 0.778 on TR5, 0.790, 0.779 on TR4&5, versus the radiologists of 0.686 (P=0.108), 0.766 (P=0.101), 0.677 (P=0.211), 0.750 (P=0.128), and 0.680 (P=0.023), 0.761 (P=0.530), respectively.
Conclusions: The study demonstrated that DL could improve the differentiation of malignant from benign thyroid nodules and had significant potential for clinical application on TR4 and TR5.
Introduction
With the utilization of high-frequency ultrasound in clinical practice and the gradual enhancement of public health awareness especially on physical examination, the detection of thyroid nodules (TN) has increased, with a prevalence ranging from 19% to 68% in the general unselected population (1, 2). Moreover, the incidence rate of thyroid cancer has continued to increase and is now the highest cause of cancer in women under 30 years old in China (3, 4). Ultrasound has an irreplaceable role in early detection of thyroid cancer due to its accessibility, high resolution, safety, using no radiation, and provision of real-time imaging with multi-dimensions. Experience and skills of different operators influence the accurate differential diagnosis of TN, and thus, a precise and independent method is needed.
To implement standardized management of the thyroid nodules, the Thyroid Imaging Reporting and Data System (TI-RADS) Committee of American College of Radiology (ACR) published a white paper in 2017 that presented a new risk stratification system from TR1 to TR5 for classifying thyroid nodules by adding scores of the five characteristics on ultrasound, composition, echogenicity, shape, margin, and echogenic foci (5). Recommendations for biopsy or ultrasound follow-up are determined on the nodule’s ACR TI-RADS categories and its maximum diameter (6), which provides clarity for the further diagnosis and treatment measures. The guidance of ACR TI-RADS has been proven to be a reliable tool to assist doctors to differentiate between malignant and benign thyroid nodules (7–11), with a pooled sensitivity of 0.79 (95% confidence interval [CI] = 0.77-0.81) and a pooled specificity of 0.71 (95% CI = 0.70-0.72) (12, 13).
Artificial Intelligence (AI) is of unique value for its time-saving and non-dependence on radiologist’s experience, and performs extremely well on the tasks of detection, extraction and classification of the TN on ultrasound images (14–18). Recently, AI has accomplished many complex tasks on thyroid ultrasound, such as the differentiation of malignant from benign thyroid nodules using ultrasound images from multiple cohorts (19), developing a deep learning (DL) algorithm to decide whether a TN should undergo a biopsy (16), using ultrasound elastography to improve thyroid nodule discrimination (20) and applying ultrasound images to predict metastasis in the cervical lymph nodes (21, 22).
However, there are still some flaws in these studies. First, pathological results of some nodules are missing in almost all of the published studies (19). Second, all types of thyroid nodules were included, but some nodules are easily diagnosed by doctors and AI is not that necessary. For example, cystic nodules are usually echoless with clear boundaries and it is not surprising that AI performs diagnosing them as benign.
ACR TI-RADS is popularly used in routine clinical practice, and has proven value. It is still an open question if the combination of DL and TI-RADS can improve the differential diagnosis of TNs. TR1, TR2, TR3 have a very low (less than 5%) chance of malignancy (6) and the necessity for them to proceed AI analysis seem less sufficient. Adversely, malignant thyroid nodules were most distributed in TR4 and TR5. However, it is difficult for radiologists to differentiate benign from malignant nodules in the same category causing that they have same ultrasound descriptive features (23). A non-invasive method such as DL is needed to avoid the need for unnecessary biopsy.
The purpose of this study was to evaluate whether DL based on ACR TI-RADS category 4 and 5 could improve the differentiation of malignant from benign thyroid nodules, and explore the clinical application potential for it.
Materials and Methods
Source of the Data
This study was approved by the Ethics Committee of Tongji Medical College of Huazhong University of Science and Technology. Informed consent from the patients was exempted (2019S1233). All ultrasound images included were consecutively acquired from 11 operators with more than 5 years of experience from Tongji hospital, Wuhan, China (internal cohort), and Xiangya Hospital of Central South University, Changsha, China (external cohort) from June 2017 to April 2019. Ultrasound equipment manufactured by GE Healthcare (LOGIQ E9, LOGIQ S7), Samsung (RS80A), and Philips (EPIQ5, EPIQ7 and IU22), was used to generate the thyroid ultrasound images. Ultrasound images were derived from the picture archiving and communication system (PACS) workstations.
Images Enrolments and Grouping
The inclusion criteria for thyroid nodules in this study were patients who 1) underwent total or nearly total thyroidectomy or lobectomy; 2) had pathological specimens examined within one month after US examination; 3) had complete medical information including preoperative ultrasound of the thyroid nodules; 4) had no previous surgical treatment or FNA performed on the nodules.
Exclusion criteria were lesions 1) with unsatisfactory ultrasound image quality; 2) where the finding on ultrasound did not match with the pathological results in position or size; 3) received chemotherapy and/or radiotherapy such as iodine 131 treatment before ultrasound examination.
From June 2nd, 2017 to April 23th, 2019, 4910 thyroid images from 2779 consecutive patients and 213 thyroid images from 195 consecutive patients with confirmed postoperative pathological results were retrospectively collected in Tongji hospital and Xiangya Hospital of Central South University. Three doctors (C.R, Y.R, and W.G) scored these images on the five features according to ACR TI-RADS lexicon (6). The opinion of the third was referred to for cases where the first opinions differed. Only nodules of TI-RADS category 4 (dataset I) and category 5 (dataset II) were enrolled, and they were merged together as new dataset III (i.e. combination of ACR TI-RADS 4 and 5). In accordance with the pathological results, images of each category were sorted out into a benign group and a malignant group.
Establishment of Training Set and Test Set
Each inner dataset (I, II, III) was randomly divided into two sets, 90% for training and validation, and the residual 10% (test set A) for testing. In addition, another independent outer test set (test set B) was obtained for testing as well. Three convolutional neutral Network (CNN) models named ResNet-50, Inception-Resnet v2, Desnet-121 were used for analysis. The workflow of the selection and construction is shown in Figure 1.
Three independent experienced radiologists (X.J and Y.Y and Z.B) with 8 years, 9 years and 24 years of experience, respectively, read the images and gave their judgments according to the ACR TI-RADS lexicon (5, 6) and their own clinical experience. If their opinions did not agree, the opinion of the most senior radiologist was used.
Processing of Ultrasound Images
Nodules were manually marked, and the region of interest (ROI) of the thyroid nodules was cut out using rectangular boxes by Image J (version 1.48, National Institutes of Health, USA) by a radiologist, in which the cropped images include the entire thyroid nodule. All the images were resized to 299 × 299 pixels to standardize the distance scale. Due to the limited quantity of the dataset, augmentation strategy was introduced to process the images. All preprocessing steps were conducted using the Keras Image Data Generator and then fed into the input.
Construction of CNNs
The tasks on three sets (datasets I, II, and III) were trained on three pre-trained convolutional neural networks, named ResNet50, Inception-ResNet v2, Desnet 121, respectively. The initialization set of the parameters of these models was referred to ImageNet and obtained from Keras Team (https://github.com/keras-team/keras-applications/releases). The learning rate was set to 0.03 and decelerated by a factor of 0.1 for each 50 epochs when the accuracy had no further improvement in the training and validation set. Model learning continued until the least loss of the validation set appeared and the final model was determined accordingly. Optimizer of Stochastic Gradient Descent (SGD) and binary cross entropy technique were used to decrease loss in the process in CNNs. All models were trained in Python 3.6.2 (https://www.python.org) by using a computer with a GeForce GTX 2080 Ti graphics processing unit (NVIDIA, Santa Clara, California, America), a Core i9-9900K central processing unit (Intel, Santa Clara, California, America).
The class activation mapping (CAM) technique was also used to produce the heated maps which indicated the focus of the CNN model’s prediction (24, 25). The CAM can be regarded as the multiplication of the feature maps of the pooling layers and weight of the fully connected layer, which prevented loss of the special information when feature maps were transferred to eigenvector. It highlighted the specific discriminative regions demonstrated as thyroid cancer by CNN. Packages Matplotlib 3.1.1 (https://matplotlib.org) and Open cv-Python 3.4.4.19 (https://github.com/skvark/opencv-python) was employed to generate heatmaps (Figure 3).
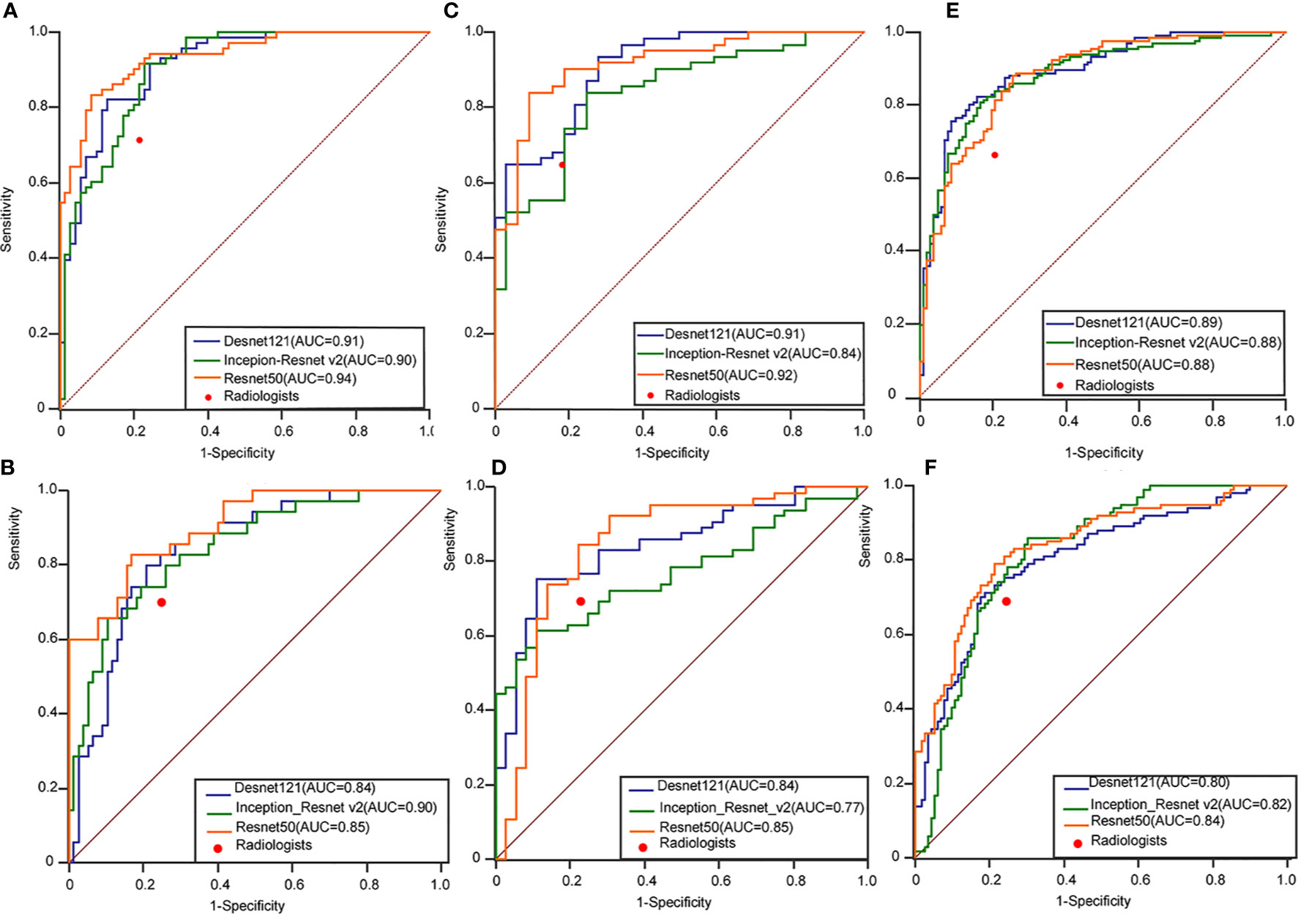
Figure 3 Performance of the ensemble D-CNN models in identifying patients with thyroid cancer in TR4 (A), TR5 (C), and TR4&5 (E) on three inner test datasets and TR4 (B), TR5 (D), and TR4&5 (F) on three outer test datasets. The red dots on each ROC curve demonstrate the performance of the radiologists. AUC, area under the curve; DCNN, deep convolutional neural network; ROC, receiver operating characteristics curve.
Statistical Analysis
The performance of the three algorithms was measured by the area under the receiver operating characteristic curve (AUROC) of the training and test dataset. The cut-off value was obtained as the threshold value when the Youden index reached its maximum. Then, the accuracy, sensitivity, specificity, positive predictive value (PPV), and negative predictive value (NPV) of each method were calculated to judge the performance of the experts and the CNNs. Delong test was introduced to evaluate the statistical difference between different AUCs. Ninety-five percent confidence interval (CI) was utilized to estimate the range of these evaluation values. P-value less than 0.05 with two tailed was considered statistically significant. Interobserver agreements on thyroid nodules were assessed using Kruskal–Wallis test. Kappa values were interpreted as follows. Less than 0.20 mean poor agreement, from 0.20 to 0.40 mean fair agreement, from 0.40 to 0.60 imply moderate agreement, between 0.60 and 0.80 imply substantial agreement, and excellent agreement tend to be over 0.80. F score was introduced to measure the efficiency of the CNNs while taking both Precision and Recall into account, the formula is as follows. When β = 1, the F1 score improves Precision and Recall as much as possible, and makes the difference between the two as small as possible.
The curve of ROC was performed and portraited using the pROC package of R software (version 1.8) and MedCalc (version 11.2, Ostend, Belgium). Outcome of evaluation values was also obtained by SPSS (version 22.0, IBM, Chicago) and R software.
Results
Characteristics of the Thyroid Nodules
A total of 2295 thyroid images from 1593 patients were used in this research (Table 1). In the internal cohort, the mean age of all patients was 45.48 ± 10.33, of which 1059 were woman, 337 were men. In the external cohort, the mean age of all patients was 45.54 ± 11.82, of which 150 were woman, 47 were men. 1146 thyroid images of TR4 and 698 thyroid images of TR5 were enrolled in training set in this research, which consisted of 637 benign images and 509 malignant images in the former, 297 benign images and 401 malignant images in the latter. 143 thyroid images of TR4 and 95 thyroid images of TR5 were predicted for the internal test in this research, while 112 of TR4 and 101 of TR5 for the external test. The characteristics of the thyroid nodules in five ACR TI-RADS features were summarized in Table 2.
DL Performance Compared With Radiologists
The performance of DL was better compared to the radiologists in three tasks. In the internal test set, the AUROC of the best algorithm in differentiation of thyroid nodules was 0.936 (95%CI 0.898-0.973) in TR4, 0.915 (95%CI 0.857-0.973) in TR5 and 0.892 (95%CI 0.850-0.933) in TR 4&5 respectively, which overwhelmingly exceeded the radiologists respectively (P < 0.001). In the external test set, the AUROC of the optimal algorithm was 0.904 (95%CI 0.833-0.951) in TR4, 0.845 (95%CI 0.759-0.909) in TR5 and 0.829 (95%CI 0.772-0.877) in TR 4&5 respectively, which again was better than the radiologists (P < 0.001).
Evaluation of the performance on differentiation of malignant from benign thyroid nodules in TR4, TR 5 and TR 4&5 were recorded in Tables 3–5, respectively. ResNet-50 performed best in the certain classification in both TR4 and TR5 dataset. Meanwhile, performance in two datasets was also excellent with a stable repeatability, of which the kappa value was all over 0.50.
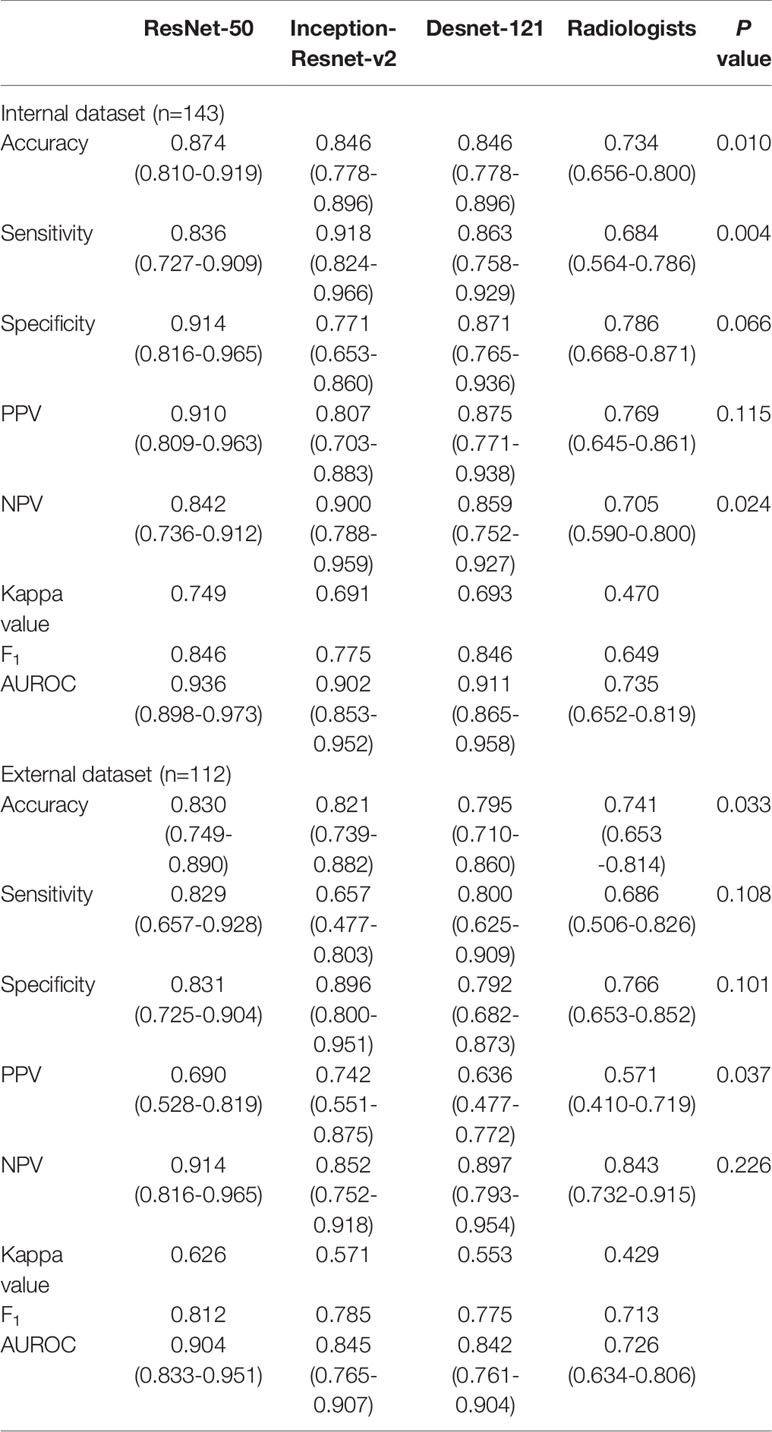
Table 3 Performance of deep learning containing three CNNs compared with the radiologists in differentiating benign and malignant thyroid nodules classified into ACR TI-RADS category 4.
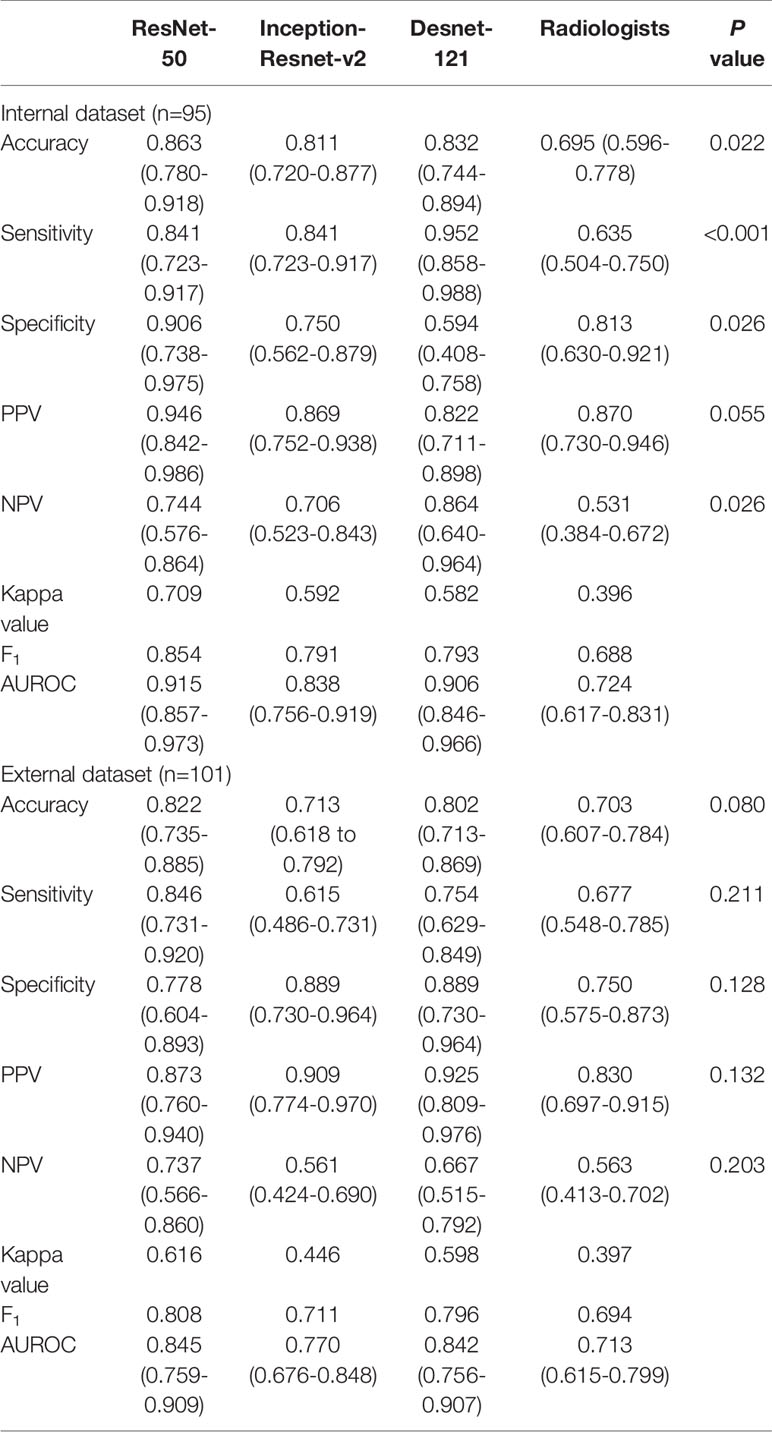
Table 4 Performance of deep learning containing three CNNs compared with the radiologists in differentiating benign and malignant thyroid nodules classified into ACR TI-RADS category 5.
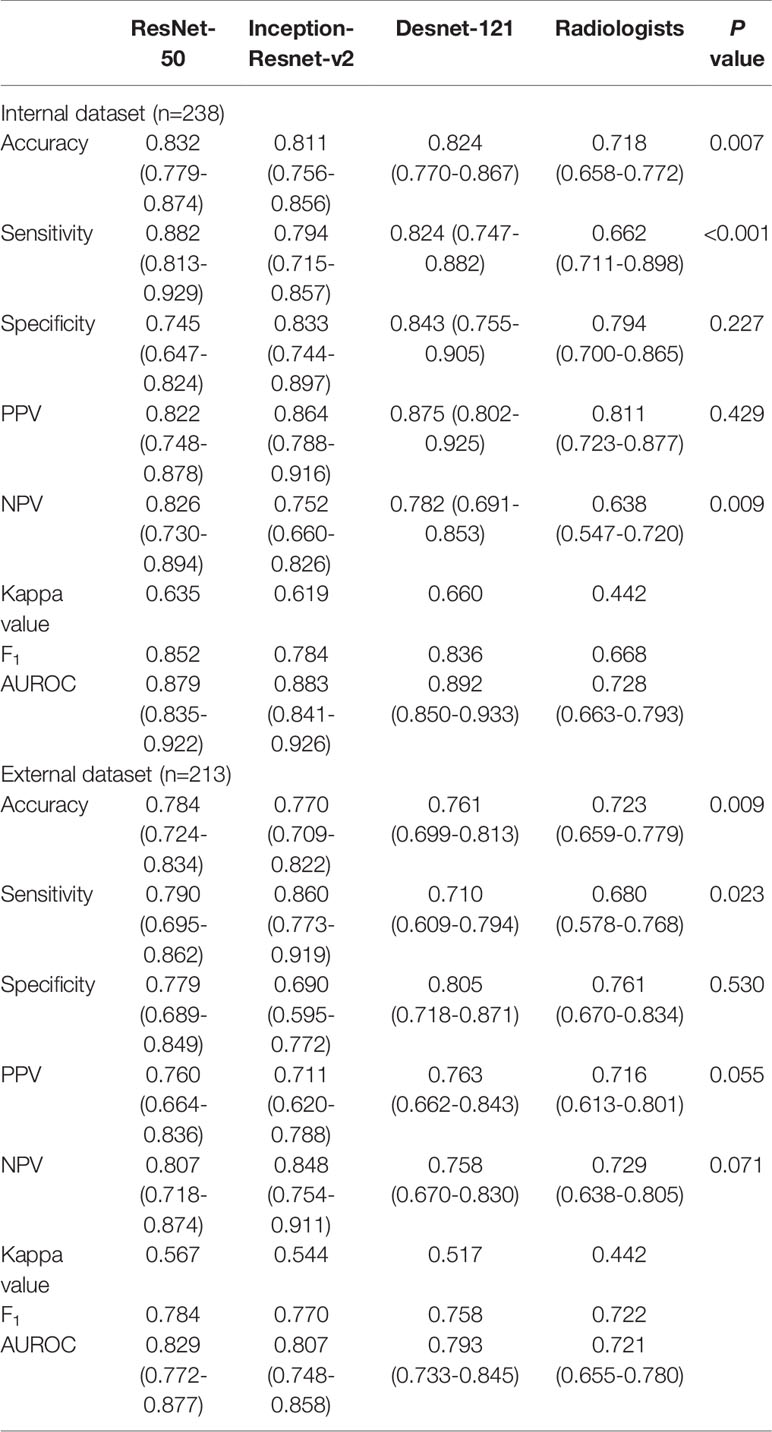
Table 5 Performance of deep learning containing three CNNs compared with the radiologists in differentiating benign and malignant thyroid nodules classified into ACR TI-RADS category 4 and 5.
Heatmaps Generated by CAM
Heatmaps were generated to present the recognition pattern of the deep learning model as demonstrated in Figure 2. The greatest predictive regions of the tumor CNNs concentrated were shown as red and yellow; whereas the areas green and blue regions were of less predictive significance. This shows that the DL algorithms focuses on the most predictive image features of thyroid nodules malignance risk.
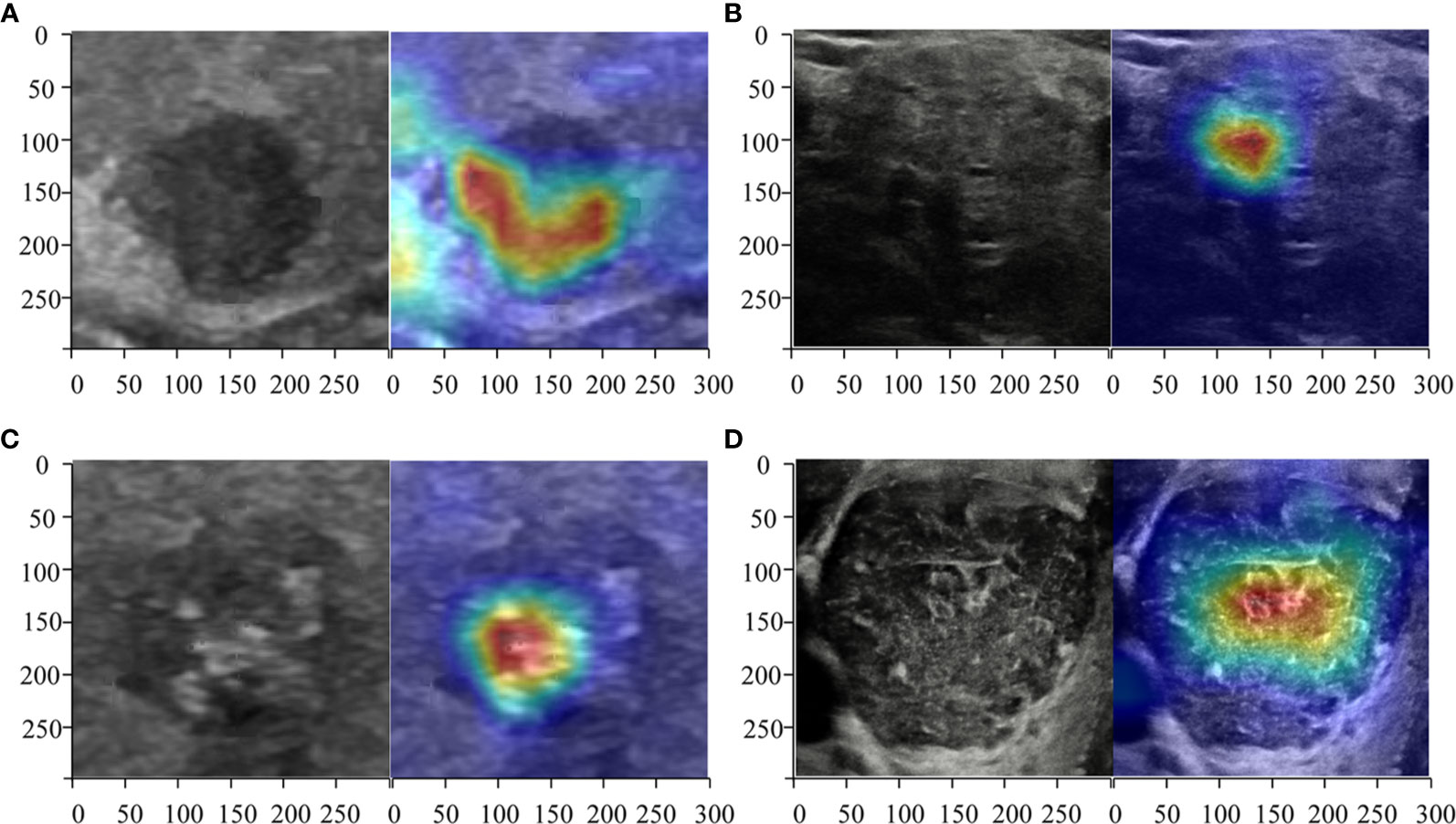
Figure 2 Heatmaps of the region of interest (ROI) of the thyroid nodules using class activation mapping (CAM). The red color showed the prediction regions the CNNs focused which estimated to be determined as the thyroid cancer. Three radiologists and DL correctly predicted a malignant (A) thyroid nodule diagnosed as micro papillary carcinoma TR4 and a benign (B) one diagnosed as non-toxic nodular goiter of TR4. ResNet50, Desnet121, and the radiologists deemed a malignant nodule (C) diagnosed as papillary carcinoma of TR5 as malignance but a DL algorithm named Inception-ResNet version 2 judged it as benign. All CNNs correctly predicted a benign (D) thyroid nodule diagnosed as Hashimoto’s thyroiditis of TR5 but the radiologists all predicted wrongly.
Discussion
In this study, we combined ACR TI-RADS with DL by training three commonly used deep learning algorithms to discriminate between benign and malignant in TR4 and TR5 thyroid nodules with available pathology. As shown in Figure 3, no matter which type of TI-RADS was used for the classification competition, DL algorithms performed better than radiologists. The accuracy in all models was higher in TR4 and TR5 for test set A and test set B, which was parallel to the performance of the radiologists. However, in the case of mixing different feature sets containing TR4 and TR5, DL still had good performance but slightly weaker than the two separated sets, which might be related to more complex tasks.
Patients with suspected thyroid nodules, nodular goiter, nodules accidentally discovered by radiological examination such as computed tomography (CT), magnetic resonance imaging (MRI), or 18F-flurodeoxyglucose positron emission computed tomography (FDP18-PET) scan showing thyroid uptake should undergo diagnostic thyroid ultrasound examination as recommended by ATA Guidelines 2015 (26). The benign and malignant ultrasound results of nodules will determine whether FNA and follow-up are to be carried out (27), and the choice of treatment methods will be influenced by ultrasound opinions and cervical lymph node conditions (28). In ultrasound diagnosis, malignant nodules have various manifestations and particularly those with atypical appearances and fuzzy boundaries lead to diagnostic difficulties (29, 30). Radiologists frequently disagree over the interpretation of these malignant tumors. DL may provide assistance for radiologists with good accuracy and consistency.
The performance of DL is often better than that of radiologists and even machine learning, in the diagnosis of thyroid nodules. Xia and colleagues (31) achieved an accuracy of 87.7% in differentiating malignant and benign nodules by constructing extreme machine learning based on collected features obtained from 203 ultrasound images of 187 patients with thyroid cancer. Li and colleagues (19) got an accuracy of 89.8% (95% CI 86.8–92.3) in internal validation set with the DCNN model versus 78.8% with the radiologists and 85.7% (95% CI 79.2–90.8) versus 72.7% (65.0–79.6%) in external validation set. Machine learning gives opinions by extracting computational features and calculating statistically significant finite features and modeling. The modeling process of machine learning requires the segmentation of images to be more accurate, while the commonly manual work is difficult to control. Limited quantities of features and smaller sample size also resulted in inferior performance and narrow application range.
Moreover, the DL result in thyroid nodules of all TR categories was not that impressive because it contained some tasks that even radiological beginners can do such as recognizing and selecting the TR1 nodules and labelling them as benign (5). Limiting the work to differentiation between subtype TR4 and TR5 is difficult for radiologists because they had similar visible features (20). As recent studies have reported, DL had achieved great success on the classification on thyroid cancer (32), when all types of thyroid nodules were included. In these studies, pathological results of some nodules were not available (19), while in our study all the nodules correlated with surgical pathology. Limitations of the TR categories on ultrasound images avoid heterogeneity of the dataset to a degree. In specific classification, our study revealed that a precise set of certain categories contributed to the higher accuracy compared with former studies (19, 32).
The result of this study may potentially be of clinical value. TI-RADS is already widely applied worldwide and combining the TI-RADS and DL provides more accurate results and should be easily accepted clinically. Previous studies had reported that interobserver agreement in the lexicon was also substantial thus the pre-classification was easily performed and credible wherever used (33). Application of the DL based on ACR TI-RADS will supply useful suggestions when there is doubt over the diagnosis and will support services where medical resources were unbalanced.
Our study also had limitations. First, this was a retrospective study with limited categories of data. The performance of our DL system is expected to increase by including more data and expanding several sets from other hospitals. And exclusion of TR3 thyroid nodules decrease clinical application to some extent. Second, ultrasound systems of different manufactures and heterogeneity of operators may give rise to the variability in the training process. The inter-reader reliability of nodule extraction was not assessed. Third, the images reviewed were static in this study that features from multi-sections were not considered.
To be summarized, the study demonstrated that DL based on ACR TI-RADS could improve the differentiation of malignant from benign thyroid nodules with great clinical application potential. With a stable repeatability, DL algorithms showed better performance than radiologists for TNs of TR4 and TR5 categories, which are the most difficult categories for diagnosis in clinical practice. Prospective studies with long-term follow-up will be needed to examine the utility of the system and assess its effectiveness in routine clinical practice.
Data Availability Statement
The raw data supporting the conclusions of this article will be made available by the authors, without undue reservation.
Ethics Statement
The studies involving human participants were reviewed and approved by Ethics Committee of Tongji Medical College of Huazhong University of Science and Technology. Written informed consent for participation was not required for this study in accordance with the national legislation and the institutional requirements.
Author Contributions
Guarantors of integrity of entire study: G-GW, W-ZL, RY, X-WC, and BZ. Literature research: G-GW, W-ZL, RY, J-YW, X-WC, and BZ. Study concepts/study design: all authors. Contributed to acquisition of data: G-GW, W-ZL, RY,Y-JY, and BZ. Clinical studies: G-GW, RY, J-WX, Y-JY, R-XC, X-WC, and BZ. Contributed reagents/materials/analysis tools: G-GW, W-ZL. Manuscript drafting or manuscript revision: all authors. Statistical analysis: G-GW, W-ZL, RY, J-WX, Y-JY, R-XC, X-WC, and BZ. All authors contributed to the article and approved the submitted version.
Funding
This work was supported Natural Science Foundation of Hubei Province (2019CFB286), Natural Science Foundation of Hunan Province (2017JJ2394), Corps Science and Technology Key Project (2019DB012).
Conflict of Interest
The authors declare that the research was conducted in the absence of any commercial or financial relationships that could be construed as a potential conflict of interest.
Acknowledgments
The authors thank ultrasound doctors in Department of Medical Ultrasound, Tongji Hospital, Tongji Medical College for data collection. The authors also thank Shu-Kun He and Yu-Qian Jia, MD, Tongji Medical College, for assisting with preprocessing of the imaging data used and Qiu-Yu Cheng MD, Tongji Medical College for assisting with calculating the statistical data.
References
1. Guth S, Theune U, Aberle J, Galach A, Bamberger CM. Very High Prevalence of Thyroid Nodules Detected by High Frequency (13 MHz) Ultrasound Examination. Eur J Clin Invest (2009) 39(8):699–706. doi: 10.1111/j.1365-2362.2009.02162.x
2. Lew JI, Solorzano CC. Use of Ultrasound in the Management of Thyroid Cancer. Oncol (2010) 15(3):253–8. doi: 10.1634/theoncologist.2009-0324
3. Burman KD, Wartofsky L. Clinical Practice. Thyroid Nodules. New Engl J Med (2015) 373(24):2347–56. doi: 10.1056/NEJMcp1415786
4. Siegel RL, Miller KD. Cancer Statistics, 2019. Cancer Stat (2019) 69: (1):7–34. doi: 10.3322/caac.21551
5. Tessler FN, Middleton WD, Grant EG. Thyroid Imaging Reporting and Data System (Ti-Rads): A User’s Guide. Radiology (2018) 287(3):1082. doi: 10.1148/radiol.2018184008
6. Tessler FN, Middleton WD, Grant EG, Hoang JK, Berland LL, Teefey SA, et al. Acr Thyroid Imaging, Reporting and Data System (Ti-Rads): White Paper of the ACR Ti-RADS Committee. J Am Coll Radiol JACR (2017) 14(5):587–95. doi: 10.1016/j.jacr.2017.01.046
7. Barbosa TLM, Junior COM, Graf H, Cavalvanti T, Trippia MA, da Silveira Ugino RT, et al. Acr TI-RADS and ATA US Scores are Helpful for the Management of Thyroid Nodules With Indeterminate Cytology. BMC Endocrine Disord (2019) 19(1):112. doi: 10.1186/s12902-019-0429-5
8. Gao L, Xi X, Jiang Y, Yang X, Wang Y, Zhu S, et al. Comparison Among TIRADS (Acr TI-RADS and KWAK- Ti-RADS) and 2015 ATA Guidelines in the Diagnostic Efficiency of Thyroid Nodules. Endocrine (2019) 64(1):90–6. doi: 10.1007/s12020-019-01843-x
9. Hong HS, Lee JY. Diagnostic Performance of Ultrasound Patterns by K-TIRADS and 2015 ATA Guidelines in Risk Stratification of Thyroid Nodules and Follicular Lesions of Undetermined Significance. AJR Am J Roentgenol (2019) 213(2):444–50. doi: 10.2214/AJR.18.20961
10. Middleton WD, Teefey SA, Reading CC, Langer JE, Beland MD, Szabunio MM, et al. Comparison of Performance Characteristics of American College of Radiology Ti-Rads, Korean Society of Thyroid Radiology TIRADS, and American Thyroid Association Guidelines. AJR Am J Roentgenol (2018) 210(5):1148–54. doi: 10.2214/AJR.17.18822
11. Lauria Pantano A, Maddaloni E, Briganti SI, Beretta Anguissola G, Perrella E, Taffon C, et al. Differences Between ATA, AACE/ACE/AME and ACR Ti-RADS Ultrasound Classifications Performance in Identifying Cytological High-Risk Thyroid Nodules. Eur J Endocrinol (2018) 178(6):595–603. doi: 10.1530/EJE-18-0083
12. Wei X, Li Y, Zhang S, Gao M. Meta-Analysis of Thyroid Imaging Reporting and Data System in the Ultrasonographic Diagnosis of 10,437 Thyroid Nodules. Head Neck (2016) 38(2):309–15. doi: 10.1002/hed.23878
13. Xu T, Wu Y, Wu RX, Zhang YZ, Gu JY, Ye XH, et al. Validation and Comparison of Three Newly-Released Thyroid Imaging Reporting and Data Systems for Cancer Risk Determination. Endocrine (2019) 64: (2):299–307. doi: 10.1007/s12020-018-1817-8
14. Liu T, Guo Q, Lian C, Ren X, Liang S, Yu J, et al. Automated Detection and Classification of Thyroid Nodules in Ultrasound Images Using Clinical-Knowledge-Guided Convolutional Neural Networks. Med Image Anal (2019) 58:101555. doi: 10.1016/j.media.2019.101555
15. Akkus Z, Cai J, Boonrod A, Zeinoddini A, Weston AD, Philbrick KA, et al. A Survey of Deep-Learning Applications in Ultrasound: Artificial Intelligence-Powered Ultrasound for Improving Clinical Workflow. J Am Coll Radiol JACR (2019) 16(9 Pt B):1318–28. doi: 10.1016/j.jacr.2019.06.004
16. Buda M, Wildman-Tobriner B. Management of Thyroid Nodules Seen on US Images: Deep Learning May Match Performance of Radiologists. Radiology (2019) 292: (3):695–701. doi: 10.1148/radiol.2019181343
17. Song W, Li S, Liu J, Qin H, Zhang B, Zhang S, et al. Multitask Cascade Convolution Neural Networks for Automatic Thyroid Nodule Detection and Recognition. IEEE J Biomed Health Inf (2019) 23(3):1215–24. doi: 10.1109/JBHI.2018.2852718
18. Li H, Weng J, Shi Y, Gu W, Mao Y, Wang Y, et al. An Improved Deep Learning Approach for Detection of Thyroid Papillary Cancer in Ultrasound Images. Sci Rep (2018) 8(1):6600. doi: 10.1038/s41598-018-25005-7
19. Li X, Zhang S, Zhang Q, Wei X, Pan Y, Zhao J, et al. Diagnosis of Thyroid Cancer Using Deep Convolutional Neural Network Models Applied to Sonographic Images: A Retrospective, Multicohort, Diagnostic Study. Lancet Oncol (2019) 20(2):193–201. doi: 10.1016/S1470-2045(18)30762-9
20. Cantisani V, David E, Grazhdani H, Rubini A, Radzina M, Dietrich CF, et al. Prospective Evaluation of Semiquantitative Strain Ratio and Quantitative 2d Ultrasound Shear Wave Elastography (SWE) in Association With TIRADS Classification for Thyroid Nodule Characterization. Ultraschall der Med (Stuttgart Germany 1980) (2019) 40(4):495–503. doi: 10.1055/a-0853-1821
21. Lee JH, Baek JH, Kim JH, Shim WH, Chung SR, Choi YJ, et al. Deep Learning-Based Computer-Aided Diagnosis System for Localization and Diagnosis of Metastatic Lymph Nodes on Ultrasound: A Pilot Study. Thyroid Off J Am Thyroid Assoc (2018) 28(10):1332–8. doi: 10.1089/thy.2018.0082
22. Jiang M, Li C, Tang S, Lv W, Yi A, Wang B, et al. Nomogram Based on Shear-Wave Elastography Radiomics can Improve Preoperative Cervical Lymph Node Staging for Papillary Thyroid Carcinoma. Thyroid Off J Am Thyroid Assoc (2020) 30(6):885–97. doi: 10.1089/thy.2019.0780
23. Chaigneau E, Russ G, Royer B, Bigorgne C, Bienvenu-Perrard M, Rouxel A, et al. TIRADS Score is of Limited Clinical Value for Risk Stratification of Indeterminate Cytological Results. Eur J Endocrinol (2018) 179(1):13–20. doi: 10.1530/EJE-18-0078
24. Zhou B, Khosla A, Lapedriza A, Oliva A, Torralba A. (2016). Learning Deep Features for Discriminative Localization. 2016 Ieee Conference on Computer Vision and Pattern Recognition. In: IEEE Conference on Computer Vision and Pattern Recognition. Las Vegas, NV, USA. pp. 2921–9. doi: 10.1109/CVPR.2016.319
25. Zhou LQ, Wu XL. Lymph Node Metastasis Prediction From Primary Breast Cancer Us Images Using Deep Learning. Radiology (2020) 294: (1):19–28. doi: 10.1148/radiol.2019190372
26. Pitoia F, Miyauchi A. 2015 American Thyroid Association Guidelines for Thyroid Nodules and Differentiated Thyroid Cancer and Their Implementation in Various Care Settings. Thyroid Off J Am Thyroid Assoc (2016) 26(2):319–21. doi: 10.1089/thy.2015.0530
27. Dighe M, Barr R, Bojunga J, Cantisani V, Chammas MC, Cosgrove D, et al. Thyroid Ultrasound: State of the Art Part 1 - Thyroid Ultrasound Reporting and Diffuse Thyroid Diseases. Med Ultrasonography (2017) 19(1):79–93. doi: 10.11152/mu-980
28. Dietrich CF, Müller T, Bojunga J, Dong Y, Mauri G, Radzina M, et al. Statement and Recommendations on Interventional Ultrasound as a Thyroid Diagnostic and Treatment Procedure. Ultrasound Med Biol (2018) 44(1):14–36. doi: 10.1016/j.ultrasmedbio.2017.08.1889
29. Dighe M, Barr R, Bojunga J, Cantisani V, Chammas MC, Cosgrove D, et al. Thyroid Ultrasound: State of the Art. Part 2 - Focal Thyroid Lesions. Med Ultrasonography (2017) 19(2):195–210. doi: 10.11152/mu-999
30. Trimboli P, Dietrich CF, David E, Mastroeni G, Ventura Spagnolo O, Sidhu PS, et al. Ultrasound and Ultrasound-Related Techniques in Endocrine Diseases. Minerva Endocrinologica (2018) 43(3):333–40. doi: 10.1016/j.ultrasmedbio.2017.08.1500
31. Xia J, Chen H, Li Q, Zhou M, Chen L, Cai Z, et al. Ultrasound-Based Differentiation of Malignant and Benign Thyroid Nodules: An Extreme Learning Machine Approach. Comput Methods Programs Biomed (2017) 147:37–49. doi: 10.1016/j.cmpb.2017.06.005
32. Gao L, Liu R, Jiang Y, Song W, Wang Y, Liu J, et al. Computer-Aided System for Diagnosing Thyroid Nodules on Ultrasound: A Comparison With Radiologist-Based Clinical Assessments. Head Neck (2018) 40: (4):778–83. doi: 10.1002/hed.25049
Keywords: artificial intelligence, thyroid imaging reporting and data system (TI-RADS), ultrasound, thyroid cancer, deep learning
Citation: Wu G-G, Lv W-Z, Yin R, Xu J-W, Yan Y-J, Chen R-X, Wang J-Y, Zhang B, Cui X-W and Dietrich CF (2021) Deep Learning Based on ACR TI-RADS Can Improve the Differential Diagnosis of Thyroid Nodules. Front. Oncol. 11:575166. doi: 10.3389/fonc.2021.575166
Received: 07 December 2020; Accepted: 07 April 2021;
Published: 27 April 2021.
Edited by:
Po-Hsiang Tsui, Chang Gung University, TaiwanReviewed by:
Chandramohan Anuradha, Christian Medical College & Hospital, IndiaJacob Jaremko, University of Alberta, Canada
Copyright © 2021 Wu, Lv, Yin, Xu, Yan, Chen, Wang, Zhang, Cui and Dietrich. This is an open-access article distributed under the terms of the Creative Commons Attribution License (CC BY). The use, distribution or reproduction in other forums is permitted, provided the original author(s) and the copyright owner(s) are credited and that the original publication in this journal is cited, in accordance with accepted academic practice. No use, distribution or reproduction is permitted which does not comply with these terms.
*Correspondence: Xin-Wu Cui, cuixinwu@live.cn; Bo Zhang, zhangbo8095@csu.edu.cn