- 1Department of Obstetrics and Gynecology, Peking Union Medical College Hospital, Chinese Academy of Medical Sciences and Peking Union Medical College, Beijing, China
- 2Department of Obstetrics and Gynecology, National Cancer Center/Cancer Hospital, Chinese Academy of Medical Sciences and Peking Union Medical College, Beijing, China
- 3Department of Obstetrics and Gynecology, The West China Second University Hospital of Sichuan University, Chengdu, China
- 4Department of Obstetrics and Gynecology, Xiangya Hospital of Central South University, Changsha, China
- 5Department of Obstetrics and Gynecology, Chongqing University Cancer Hospital, Chongqing, China
- 6Department of Obstetrics and Gynecology, Beijing Obstetrics and Gynecology Hospital, Capital Medical University, Beijing, China
- 7Department of Obstetrics and Gynecology, Liaoning Cancer Hospital & Institute, Cancer Hospital of China Medical University, Shenyang, China
- 8Department of Obstetrics and Gynecology, Hunan Cancer Hospital, The Affiliated Cancer Hospital of Xiangya School of Medicine, Central South University, Changsha, China
- 9Department of Obstetrics and Gynecology, The First Hospital of Jilin University, Jilin, China
Objective: This study assessed the predictive value of preoperative computed tomography (CT) scans and clinical factors for optimal debulking surgery (ODS) in patients with advanced ovarian cancer (AOC).
Methods: Patients with AOC in International Federation of Gynecology and Obstetrics (FIGO) stage III-IV who underwent primary debulking surgery (PDS) between 2016 and 2019 from nine tertiary Chinese hospitals were included. Large-volume ascites, diffuse peritoneal thickening, omental cake, retroperitoneal lymph node enlargement (RLNE) below and above the inferior mesenteric artery (IMA), and suspected pelvic bowel, abdominal bowel, liver surface, liver parenchyma and portal, spleen, diaphragm and pleural lesions were evaluated on CT. Preoperative factors included age, platelet count, and albumin and CA125 levels.
Results: Overall, 296 patients were included, and 250 (84.5%) underwent ODS. The prediction model included age >60 years (P=0.016; prediction index value, PIV=1), a CA125 level >800 U/ml (P=0.033, PIV=1), abdominal bowel metastasis (P=0.034, PIV=1), spleen metastasis (P<0.001, PIV=2), diaphragmatic metastasis (P=0.014, PIV=2), and an RLNE above the IMA (P<0.001, PIV=2). This model had superior discrimination (AUC=0.788>0.750), and the Hosmer-Lemeshow test indicated its stable calibration (P=0.600>0.050). With the aim of maximizing the accuracy of prediction and minimizing the rate of inappropriate explorations, a total PIV ≥5 achieved the highest accuracy of 85.47% and identified patients who underwent suboptimal PDS with a specificity of 100%.
Conclusions: We developed a prediction model based on two preoperative clinical factors and four radiological criteria to predict unsatisfactory debulking surgery in patients with AOC. The accuracy of this prediction model needs to be validated and adjusted in further multicenter prospective studies.
Introduction
Epithelial ovarian cancer is currently the most malignant carcinoma of the female reproductive system with the highest mortality rate (1). Approximately two-thirds of ovarian cancer patients are initially diagnosed with advanced ovarian cancer (AOC) mainly due to the lack of early detection methods and specific symptoms for ovarian cancer (2). Primary debulking surgery (PDS) followed by platinum-based chemotherapy has been the standard treatment for patients with International Federation of Gynecology and Obstetrics (FIGO) stage IIIC or IV (3, 4) for many years. However, the traditional management of ovarian cancer has changed since two multicenter randomized phase III trials [EORTC 55971 (5) and CHORUS (6)] reported that neoadjuvant chemotherapy (NACT) followed by interval debulking surgery (IDS) was not inferior to PDS. NACT refers to chemotherapy as the primary treatment, which is administered to reduce the tumor burden before debulking surgery is performed (7, 8). The SCORPION (9) and JCOG0602 (10) clinical trials commonly considered that NACT-IDS was not inferior to PDS-CT due to less surgical aggressiveness and postoperative morbidity rates as well as better quality of life scores.
RD after debulking surgery is one of the most important independent risk factors for survival in AOC patients (11), regardless of surgery complexity or the administration of NACT (12, 13). Optimal debulking surgery (ODS) is considered optimal if the residual tumor (RD) is less than 1 cm in maximum diameter or thickness, which is associated with better survival outcomes than those achieved with suboptimal debulking surgery (SDS) (14, 15). Thus, improving the satisfactory cytoreduction rate and prolonging the overall survival of ovarian cancer patients are long-term goals for gynecological oncologists. The main value of NACT is reducing the tumor load and improving the feasibility of surgery and rate of ODS (16). However, many scholars have suggested that this benefit of surgery after NACT does not translate to a corresponding survival advantage and even increases the resistance of ovarian cancer patients to adjuvant chemotherapy (17–19). Two RCTs (5, 6) and some retrospective studies (20, 21) have shown no significant differences in survival outcomes between patients who receive NACT-IDS and those who receive PDS. Therefore, we explored a preoperative method of selecting the appropriate initial treatment option for AOC patients with the aim of achieving a high rate of ODS while avoiding excessive NACT (22).
Several researchers have explored specific preoperative detection methods for predicting ODS rates, including clinical factors, laboratory test results, radiological examination, laparoscopy evaluation and molecular features (23–28). Computed tomography (CT), as the most important and widely accepted imaging examination, is used to identify tumor distribution and a high tumor burden before performing cytoreduction (24). Recent studies have reported that the combination of clinical factors and CT could have a high accuracy of predicting ODS rates (29–32). The synthesized prediction system evaluates the correlation between clinical factors and CT findings to predict ODS rates and shows better sensitivity and specificity than clinical experience or limited screening methods. Several Western scholars have reported imaging-based models for predicting SDS rates in ovarian cancer patients (24, 29–36). However, it is difficult to apply and promote these models in various Chinese hospitals, especially in hospitals with doctors who have less experience in gynecological oncology. Although debulking surgery to reduce the sizes of residual tumors to the greatest extent possible should be the focus of cytoreductive efforts, complete resection is not feasible for all these hospitals due to the different levels of experience of gynecological teams. Therefore, it is necessary to develop an evidenced-based model to predict ODS for application in Chinese centers with multiple levels of experience.
We wanted to initially perform a retrospective study on a model development cohort and then perform a prospective study on a model validation cohort to make adjustments. We herein aimed to perform a multicenter retrospective cohort study to assess the values of preoperative CT and clinical factors and develop a scoring system for predicting the absence of ODS in patients with AOC undergoing primary surgery.
Materials and Methods
Study Population
Nine Chinese tertiary hospitals participated in this retrospective cohort study: Peking Union Medical College Hospital (PUMCH) as the main research center and eight other centers. Patients with FIGO stage III or IV ovarian, fallopian tube, or primary peritoneal cancer who underwent PDS between 09/01/2016 and 09/01/2019 were included. Patients with any of the following characteristics were excluded: 1) received neoadjuvant chemotherapy; 2) lacked critical clinical or operation data; and 3) underwent repeated collections. All patients provided written informed consent under approval by the ethics committee of their hospital. Patients who met the inclusion criteria were divided into two groups: 1) the ODS group: no gross RD and RD<1 cm in maximum tumor diameter and 2) the SDS group: RD≥1 cm in maximum tumor diameter.
Data Collection
Key clinical data and CT image locations were recorded into unified electronic case report forms (CRFs) under an institutional review board-approved protocol and were obtained within 4 weeks before primary surgery. All contrast-enhanced CT scans were performed on modern technology conventional and spiral CT scanners, and were reviewed by the digital picture archiving system and at least two experienced radiologists. The preoperative clinical factors included the patient’s age, platelet count, albumin level, and CA125 level. The effect of age was shown to be independent of other variables, including the stage and grade (37). Compared to older patients, younger women with AOC have a survival advantage (38). In addition, increasing evidence indicates that the platelet count is a useful biomarker of long-term outcomes in patients with OC (39). The median OS was significantly decreased in patients with thrombocytosis or elevated CA125 levels (40). The following CT image locations were evaluated: 1) medium/large-volume ascites (defined as the presence of ascites on at least 2/3 of CT slices); 2) diffuse peritoneal thickening (defined as the presence of at least 2 separate peritoneal implants each>4 mm in size); 3) omental cake; 4) pelvic bowel metastasis≥1 cm (including the rectum and sigmoid colon); 5) abdominal bowel metastasis≥1 cm (including the small intestine as well as the ascending, transverse, and descending colon); 6) liver surface lesion (including the hepatorenal space); 7) liver parenchyma and portal lesion; 8) spleen metastasis (including the spleen surface and parenchyma); 9) diaphragmatic metastasis≥1 cm; 10) pleural metastasis≥1 cm; 11) retroperitoneal lymph node enlargement (RLNE) below the level of the inferior mesenteric artery (IMA)≥1 cm (including pelvic lymph nodes); 12) and RLNE above the level of the IMA≥1 cm (including the superior phrenic lymph node). The data used to support the findings of this study are available from the corresponding author upon request.
Statistical Analysis
All statistical analyses were performed using SPSS software (version 23.0; SPSS Inc., Chicago, IL, USA). Student’s t-tests and Mann-Whitney U tests were used to compare continuous variables. Pearson’s chi-squared tests and Fisher’s exact tests were used to compare categorical variables. Continuous variables with a normal distribution are presented as the means ± standard deviations (SDs), and nonnormally distributed variables are reported as the medians ± interquartile ranges (IQRs) (41). Each of the clinical factors and radiological criteria were individually evaluated by using logistic regression for the univariate analysis. Then, all the variables with significant differences based on the univariate analysis were calculated by binary logistic regression with stepwise forward selection. The associations were evaluated by odds ratios (ORs) and corresponding 95% confidence intervals (CIs). Then, a prediction model was constructed using the variables with significant differences based on the multivariate analysis, and the prediction index value (PIV) was systematically calculated by the weight of each variable and corresponding functions. Statistical significance was set at P<0.050.
The receiver operating characteristic (ROC) curve was drawn to evaluate the discrimination performance of the prediction model. The area under the curve (AUC) and 95% CI were determined to assess its prediction ability. The Hosmer-Lemeshow (H-L) goodness of fit test was used to evaluate the calibration performance of the prediction model (41). The sensitivity, specificity, positive predictive value (PPV), negative predictive value (NPV), and accuracy of each PIV were calculated according to different cut-off values. Sensitivity was defined as the number of patients receiving SDS and were correctly identified (true positives) divided by the total number of patients receiving SDS (true positives + false negatives). Specificity was defined as the number of patients receiving ODS who were correctly identified (true negatives) divided by the total number of patients receiving ODS (true negatives + false positives). The PPV was calculated as the number of true positives divided by the total number of positive results (true positives + false positives), and the NPV was defined as the number of true negatives divided by the total number of negative results (true negatives + false negatives). Accuracy was calculated as the number of true positives plus true negatives (total number correct) divided by the total number of patients studied (42). Youden’s index (or the C-index), which indicates the maximum potential effectiveness of a biomarker, is a common summary measure of the ROC curve (43). We used Youden’s index to determine the cut-off PIV point that yielded the maximum sensitivity and specificity for predicting suboptimal cytoreduction.
Results
Flow Diagram and Characteristics of Included Patients
Figure S1 shows the flow chart of this study population. A total of 303 patients from nine centers were enrolled. After excluding five patients without critical information and two duplicate patients, a total of 296 AOC patients who met the inclusion criteria were ultimately included. A total of 250 patients were in the ODS group (including 163 patients with no RD and 87 patients with an RD of 0-1 cm), and 46 patients were in the SDS group. We kept the power of the study maximum. Table 1 shows the preoperative clinical factors and radiological criteria of the included ovarian cancer patients. The mean patient age was 53.5 (± 10.5) years in the ODS group and 55.7 (± 12.8) years in the SDS group. There was no significant difference in the perioperative platelet count (P=0.781) or perioperative albumin level (P=0.869) between the two groups. The most common abnormalities observed on CT images were omental cake (53.4%), diffuse peritoneal thickening (38.9%), and pelvic bowel metastasis (34.5%). For most of the radiological criteria, there were significant differences between the two groups, thus indicating that the comparison of these variables was meaningful.
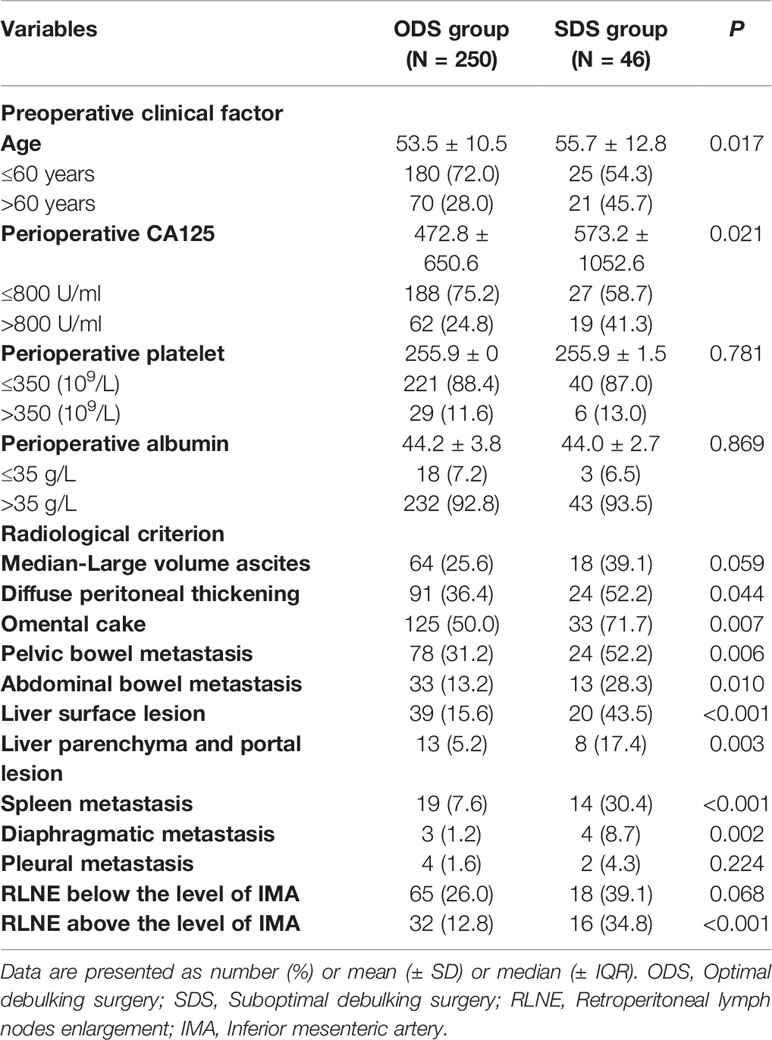
Table 1 The preoperative clinical factors and radiological criteria and of included ovarian cancer patients.
Assessment of the Prediction Model
Table 2 shows the univariate analysis of the included ovarian cancer patients. In the univariate analysis, two clinical factors were associated with suboptimal cytoreduction: age>60 years (P=0.019) and perioperative CA125 level>800 U/ml (P=0.023). Nine radiological criteria were related to suboptimal cytoreduction: diffuse peritoneal thickening (P=0.046), omental cake (P=0.008), pelvic bowel metastasis (P=0.007), abdominal bowel metastasis (P=0.012), liver surface lesion (P<0.001), liver parenchyma and portal lesion (P=0.005), spleen metastasis (P<0.001), diaphragmatic metastasis (P=0.008), and RLNE above the level of the IMA (P<0.001). Then, we performed a multivariate analysis based on the significant factors from the univariate analysis. We further calculated the PIV, which was assigned based on the multivariate regression coefficient and OR. Table 3 shows the prediction model of all significant clinical and radiological criteria based on the multivariate analysis for ODS. The predictive index parameters related to a high risk of successful SDS included age>60 years (P=0.016, PIV=1), CA125 level>800 U/ml (P=0.033, PIV=1), abdominal bowel metastasis (P=0.034, PIV=1), spleen metastasis (P<0.001, PIV=2), diaphragmatic metastasis (P=0.014, PIV=2), and RLNE above the level of the IMA (P<0.001, PIV=2).
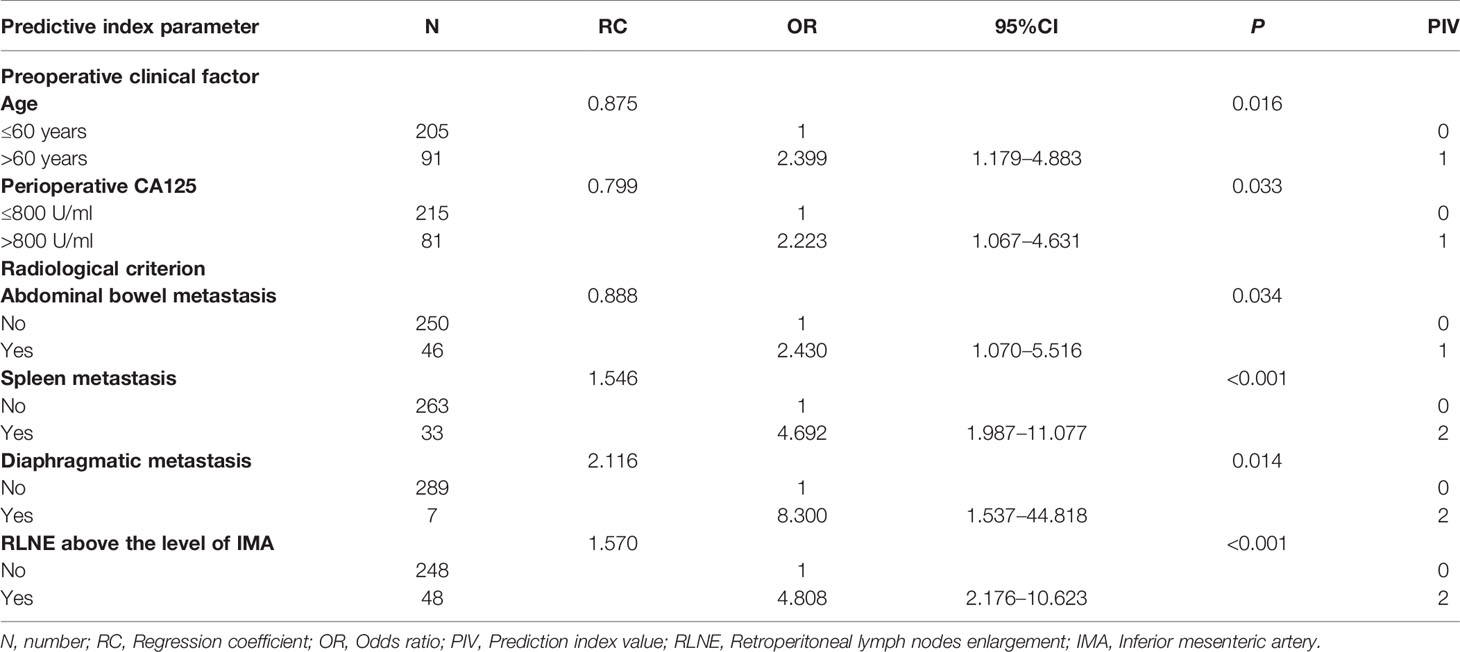
Table 3 The model of significant clinical and radiological criteria based on multivariate analysis for predicting suboptimal debulking surgery.
Evaluation of the Prediction Model
Figure 1 shows the ROC curve of the prediction model. The AUC was 0.788 (greater than 0.750), showing that this prediction model has superior discrimination. In addition, there was no significant difference between the predictive value and real value in the Hosmer-Lemeshow test (X2 = 2.752, P=0.600>0.050), which indicated the stable calibration of the prediction model. Table 4 shows the overall prediction model according to different cut-off values. The PIV ranged from 0 to nine points. The sensitivity, specificity, PPV, NPV, and overall accuracy of each PIV were determined. Youden’s index of the ROC curve was 41.5%, which corresponded to a sensitivity of 89.1% and a specificity of 52.4%. Consequently, with the aim of maximizing the accuracy of prediction and minimizing the rate of inappropriate explorations, a PIV of ≥5 achieved the highest accuracy of 85.47% and identified patients who underwent SDS with a specificity of 100%.
Discussion
At present, NACT-IDS is an alternative treatment option for AOC patients who may initially have a low incidence of ODS (7, 8). However, there is no unified standard method of selecting appropriate patients or determining the best time to perform PDS. Some gynecologists reported that the following clinical factors were associated with a high rate of unsatisfactory cytoreduction: 1) extensive implant metastasis in the upper abdomen or thorax and a large tumor burden throughout the whole body and 2) a poor performance status that could not tolerate cytoreduction (e.g., advanced age, high-risk complications, or the combination of a large amount of ascites and hydrothorax) (16, 44). However, this type of clinical report lacks scientific evidence and cannot be widely accepted and applied by different gynecologists in various hospitals. Therefore, the selection of appropriate AOC patients for undergoing primary surgery to obtain the highest success rate of ODS has been a focus in the past few decades.
Laparoscopy can be used to assess the probability of having no residual tumor before PDS and to evaluate tumor size, the degree of tumor spread, and tumor infiltration in surrounding tissues (26, 27, 45). Fagotti et al. developed a widely accepted staging laparoscopy scoring system for determining candidates for primary surgery (46) that includes omental cake (PIV=1), extensive peritoneal (PIV=1) and diaphragmatic (PIV=1) carcinosis, mesenteric retraction (PIV=2), bowel (PIV=2) and stomach (PIV=2) infiltration, and superficial spleen and/or liver metastasis (PIV=2). At a PIV≥8, the probability of ODS during laparotomy is equal to 0; thus, NACT is initially recommended. This laparoscopy model can avoid an unnecessary exploratory laparotomy and improve individualized treatment for AOC patients. However, as an invasive manipulation procedure, laparoscopy has the risks of anesthesia and surgical complications (11). In addition, the evaluation of lesions under retroperitoneal and retrohepatic areas is limited (47). Furthermore, we must consider the cost-effectiveness benefit: laparoscopy is more time consuming and expensive than other preoperative examinations (47). Due to the above limitations, it is difficult to perform a staging laparoscopy before PDS in most Chinese hospitals. In addition, the molecular features played important roles in patients with OC, affecting the status of the BRCA gene and homologous recombination deficiency (HRD) (28, 48). However, primary targeted therapy for OC lacks sufficient evidence, and most patients cannot afford the high cost of gene detection. Therefore, we aimed to develop a preoperative evaluation model that combines clinical features with imaging examination features to allow patients to obtain the greatest benefit.
The model used to predict the rate of unsatisfactory debulking surgery in our study ultimately included two preoperative clinical factors and four radiological criteria based on the multivariate analysis of all enrolled patients. The two clinical factors, age>60 years and CA125 level>800 U/ml, indicated that elderly patients who have a high tumor burden and a poor general condition are not suitable for PDS. The four radiological criteria showed that tumors located in the upper abdomen or thorax, such as the diaphragm, spleen, and upper retroperitoneal lymph nodes, greatly influence the cytoreduction rate, as they are truly difficult to remove in debulking surgery. The evaluation of retroperitoneal disease extension plays a very important prognostic role for patients with OC (49, 50). Our prediction model is a scoring system; therefore, the difficulty of PDS and the rate of having no RD are not determined by a single high-risk factor but rather by the accumulation degree of high-risk factors. This may be attributed to poor surgical tolerance, a long surgical time, and surgical complications during PDS if an AOC patient has a high score in our prediction model. Therefore, our model is basically consistent with the experience of gynecological oncologists (44, 51) but has more scientific- and evidence-based support.
Several scholars have published imaging-based models for predicting SDS rates in ovarian cancer patients (24, 29–31, 33–36, 52); these are summarized in Table 5. Among them, the model from Suidan et al. at Memorial Sloan-Kettering Cancer Center (MSKCC) is one of the most common and high evidence-based imaging models (29). However, in our practical application, it was found that the imaging classification of this model is excessively detailed and complex and often requires professional senior imaging doctors to read CT scans. It is difficult for gynecologists at different levels to apply this model, and there is also great difficulty in promoting this model in Chinese hospitals. Therefore, we initially roughly divided the body into several large areas based on our previous surgical experience (e.g., the pelvic and abdominal bowels, spleen, liver, and RLN). Our model may be more operable and applicable than the MSKCC model for gynecologic oncologists in various Chinese hospitals. In addition, our prediction model has a specificity of 100% and an accuracy of 85.47%, which shows superior discrimination and stable calibration.
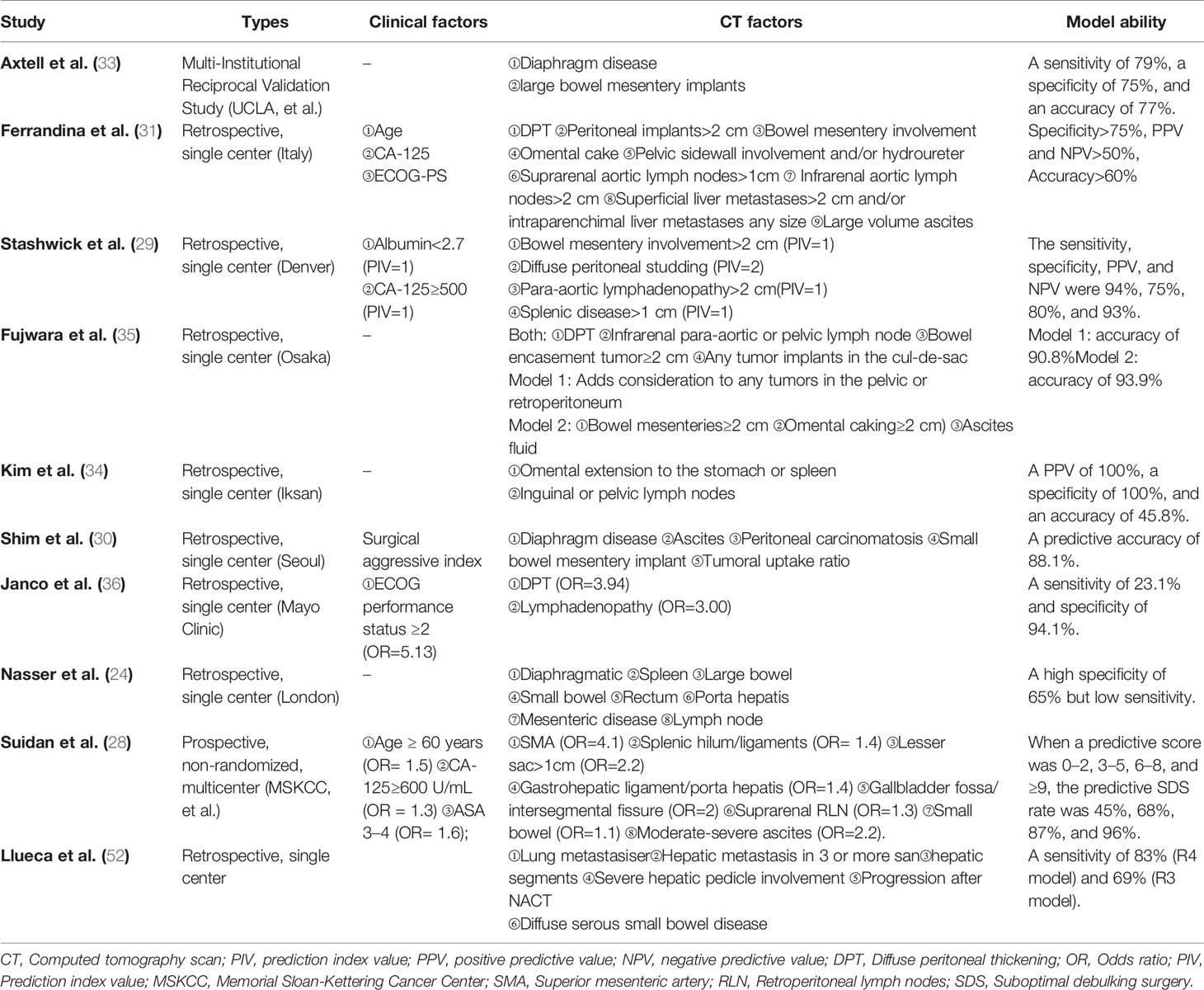
Table 5 The model comparison of predicting suboptimal debulking surgery based on radiological criteria in ovarian cancer from published reports.
On the other hand, Llueca et al. proposed a predictive model with the peritoneal cancer index (PCI), which can provide more detailed information about peritoneal spread. The PCI is used to quantitatively assess cancer distribution in the peritoneum based on the sizes of lesions in 13 abdominopelvic regions, and patients were classified into three categories with scores of 1–10, 11–20, and >20 (53). In the pilot study, their models predicted suboptimal or complete and optimal cytoreductive surgery with sensitivities of 83% (R4 model) and 69% (R3 model). A PCI>20 was a major risk factor for unresectability in patients with AOC (52). In our study, peritoneal metastasis was evaluated in the variable statistics but was not included in the final multivariate model. PCI is a preferable supplement to our model, which could be improved by including this assessment of the PCI. In addition, several studies reported that minimally invasive interval debulking surgery (MI-IDS) played a positive role in the quality of life and surgery complications (27, 54). However, the range of surgical resection in MI-IDS is limited, and a thorough peritoneal evaluation is not possible. It is difficult to reach ODS, resulting in residual tumors and worsened oncologic outcomes. MI-IDS may considered be limited to low-complexity standard debulking surgery (55, 56).
Moreover, the greatest advantage of this study is that it was a multicenter study that collected patient data from nine large tertiary hospitals in China. The large-scale study, wide area coverage, and high quantity of enrolled patients ensured the diversity and credibility of this report. This retrospective study is not only the basis for creating a predictive model but can also be further verified in subsequent multicenter prospective studies. After modification and identification, it may ultimately be promoted in most hospitals in China, thus achieving the purpose of this whole study.
There are several limitations to this study. First, due to the natural limitations of retrospective research, unknown potential confounders and selection biases may be present (57). However, we attempted to make a unified standard for collecting patients and evaluating CT sites to ensure that all data were collected in a similar manner. Moreover, we balanced the confounding factors between the two groups by Cox multivariate regression analysis when there were a few heterogeneities in baseline factors between the ODS and SDS groups. Second, the horizontal differentiation among multiple centers may lead to data deviations. The centers participating in this study were all high-quality large-scale hospitals in China, and gynecologists had sufficient experience in the diagnosis and treatment of ovarian cancer. The inclusion criteria for our study population and data collection were unified standards. The capabilities of performing debulking surgery and reading CT scans were similar among the various centers. Currently, a multicenter, nonrandom prospective study is ongoing. We expect this prediction model to be verified and adjusted by collecting additional data.
Conclusion
In conclusion, we developed a prediction model based on two preoperative clinical factors and four radiological criteria for predicting unsatisfactory debulking surgery in AOC patients: included age>60 years (PIV=1), CA125 level>800 U/ml (PIV=1), abdominal bowel metastasis (PIV=1), spleen metastasis (PIV=2), diaphragmatic metastasis (PIV=2), and RLNE above the level of the IMA (PIV=2). A total PIV of ≥5 in this model may indicate a high risk associated with undergoing SDS, with an accuracy of 85.47% and a specificity of 100%. The accuracy of this prediction model needs to be validated and adjusted in further multicenter prospective studies. We look forward to collecting additional information from ovarian cancer patients and learning from research experience at other centers.
Data Availability Statement
The raw data supporting the conclusions of this article will be made available by the authors, without undue reservation.
Ethics Statement
The studies involving human participants were reviewed and approved by the ethics committee of each hospital. In the main center, all patients provided written informed consent under approval by the ethics committee of Peking Union Medical College Hospital.
Author Contributions
L-YW and L-YP conceptualized the study. YG, MQ, and YJ contributed to the methodology. YJ, JZ, and NL validated the study. YG, MQ, and YJ contributed to the formal analysis and investigation. YG and MQ wrote and prepared the original draft. All authors wrote and prepared the original draft. L-YW and L-YP acquired the funding. All authors provided the resources. L-YW and L-YP supervised the study. All authors contributed to the article and approved the submitted version.
Funding
This project was supported by The Fund of The National Key R&D Program of China 2016YFC1303700 (Affiliated project 2016YFC1303701, 2016.9-2020.12). Furthermore, this project was also supported by CAMS Innovation Fund for Medical Sciences (CIFMS-2017-I2M-1-002, 2017.01-2020.12).
Conflict of Interest
The authors declare that the research was conducted in the absence of any commercial or financial relationships that could be construed as a potential conflict of interest.
Supplementary Material
The Supplementary Material for this article can be found online at: https://www.frontiersin.org/articles/10.3389/fonc.2020.611617/full#supplementary-material
References
1. Jemal A, Siegel R, Ward E, Murray T, Xu J, Thun MJ. Cancer statistics, 2007. CA Cancer J Clin (2007) 57(1):43–66. doi: 10.3322/canjclin.57.1.43
2. van Altena AM, Karim-Kos HE, de Vries E, Kruitwagen RF, Massuger LF, Kiemeney LA. Trends in therapy and survival of advanced stage epithelial ovarian cancer patients in the Netherlands. Gynecol Oncol (2012) 125(3):649–54. doi: 10.1016/j.ygyno.2012.02.033
3. Fago-Olsen CL, Ottesen B, Kehlet H, Markauskas A, Mosgaard BJ, Ottosen C, et al. Neoadjuvant chemotherapy as ovarian cancer treatment: ever more used with major regional differences. Danish Med J (2012) 59(8):A4477.
4. Zivanovic O, Aldini A, Carlson JW, Chi DS. Advanced cytoreductive surgery: American perspective. Gynecol Oncol (2009) 114(2 SUPPL.):S3–9. doi: 10.1016/j.ygyno.2008.11.033
5. Vergote I, Tropé CG, Amant F, Kristensen GB, Ehlen T, Johnson N, et al. Neoadjuvant chemotherapy or primary surgery in stage IIIC or IV ovarian cancer. New Engl J Med (2010) 363(10):943–53. doi: 10.1016/S0140-6736(14)62223-6
6. Kehoe S, Hook J, Nankivell M, Jayson GC, Kitchener H, Lopes T, et al. Primary chemotherapy versus primary surgery for newly diagnosed advanced ovarian cancer (CHORUS): an open-label, randomised, controlled, non-inferiority trial. Lancet (2015) 386(9990):249–57. doi: 10.1016/S0140-6736(14)62223-6
7. Morgan RJ Jr., Alvarez RD, Armstrong DK, Burger RA, Castells M, Chen LM, et al. Ovarian cancer, version 3.2012: Featured updates to the NCCN guidelines. JNCCN J Natl Compr Cancer Netw (2012) 10(11):1339–49. doi: 10.6004/jnccn.2012.0140
8. Wright AA, Bohlke K, Armstrong DK, Bookman MA, Cliby WA, Coleman RL, et al. Neoadjuvant chemotherapy for newly diagnosed, advanced ovarian cancer: Society of Gynecologic Oncology and American Society of Clinical Oncology Clinical Practice Guideline. Gynecol Oncol (2016) 143(1):3–15. doi: 10.1016/j.ygyno.2016.05.022
9. Fagotti A, Ferrandina MG, Vizzielli G, Pasciuto T, Fanfani F, Gallotta V, et al. Randomized trial of primary debulking surgery versus neoadjuvant chemotherapy for advanced epithelial ovarian cancer (SCORPION-NCT01461850). Int J Gynecol Cancer (2020) 30(11):1657–64. doi: 10.1136/ijgc-2020-001640
10. Onda T, Satoh T, Saito T, Kasamatsu T, Nakanishi T, Nakamura K, et al. Comparison of treatment invasiveness between upfront debulking surgery versus interval debulking surgery following neoadjuvant chemotherapy for stage III/IV ovarian, tubal, and peritoneal cancers in a phase III randomised trial: Japan Clinical Oncology Group Study JCOG0602. Eur J Cancer (2016) 64:22–31. doi: 10.1016/j.ejca.2016.05.017
11. Gomez-Hidalgo NR, Martinez-Cannon BA, Nick AM, Lu KH, Sood AK, Coleman RL, et al. Predictors of optimal cytoreduction in patients with newly diagnosed advanced-stage epithelial ovarian cancer: Time to incorporate laparoscopic assessment into the standard of care. Gynecol Oncol (2015) 137(3):553–8. doi: 10.1016/j.ygyno.2015.03.049
12. Aletti GD, Dowdy SC, Gostout BS, Jones MB, Stanhope CR, Wilson TO, et al. Aggressive surgical effort and improved survival in advanced-stage ovarian cancer. Obstet Gynecol (2006) 107(1):77–85. doi: 10.1097/01.AOG.0000192407.04428.bb
13. Rutten MJ, Sonke GS, Westermann AM, van Driel WJ, Trum JW, Kenter GG, et al. Prognostic Value of Residual Disease after Interval Debulking Surgery for FIGO Stage IIIC and IV Epithelial Ovarian Cancer. Obstetr Gynecol Int (2015) 2015:464123. doi: 10.1155/2015/464123
14. Pineda M, Plaxe SC, Powell MA, Ratner E. Ovarian Cancer, Version 1.2016. J Natl Compr Cancer Netw JNCCN (2016) 14(9):1134–63. doi: 10.6004/jnccn.2016.0122
15. Chi D, Eisenhauer E, Lang J, Huh J, Haddad L, Abu-Rustum N, et al. What is the optimal goal of primary cytoreductive surgery for bulky stage IIIC epithelial ovarian carcinoma (EOC)? Gynecol Oncol (2006) 103(2):559–64. doi: 10.1016/j.ygyno.2006.03.051
16. Qin M, Jin Y, Ma L, Zhang YY, Pan LY. The role of neoadjuvant chemotherapy followed by interval debulking surgery in advanced ovarian cancer: a systematic review and meta-analysis of randomized controlled trials and observational studies. Oncotarget (2018) 9(9):8614–28. doi: 10.18632/oncotarget.23808
17. Fagö-Olsen CL, Ottesen B, Kehlet H, Antonsen SL, Christensen IJ, Markauskas A, et al. Does neoadjuvant chemotherapy impair long-term survival for ovarian cancer patients? A nationwide Danish study. Gynecol Oncol (2014) 132(2):292–8. doi: 10.1016/j.ygyno.2013.11.035
18. Bristow RE, Chi DS. Platinum-based neoadjuvant chemotherapy and interval surgical cytoreduction for advanced ovarian cancer: a meta-analysis. Gynecol Oncol (2006) 103(3):1070–6. doi: 10.1016/j.ygyno.2006.06.025
19. Rauh-Hain JA, Melamed A, Wright A, Gockley A, Clemmer JT, Schorge JO, et al. Overall Survival Following Neoadjuvant Chemotherapy vs Primary Cytoreductive Surgery in Women With Epithelial Ovarian Cancer: Analysis of the National Cancer Database. JAMA Oncol (2016) 145(1):68–9. doi: 10.1001/jamaoncol.2016.4411
20. Milam MR, Tao X, Coleman RL, Harrell R, Bassett R, Dos Reis R, et al. Neoadjuvant chemotherapy is associated with prolonged primary treatment intervals in patients with advanced epithelial ovarian cancer. Int J Gynecol Cancer (2011) 21(1):66–71. doi: 10.1097/IGC.0b013e3182013e2f
21. Inciura A, Simavicius A, Juozaityte E, Kurtinaitis J, Nadisauskiene R, Svedas E, et al. Comparison of adjuvant and neoadjuvant chemotherapy in the management of advanced ovarian cancer: a retrospective study of 574 patients. BMC Cancer (2006) 6:153. doi: 10.1186/1471-2407-6-153
22. Kang S, Park SY. To predict or not to predict? The dilemma of predicting the risk of suboptimal cytoreduction in ovarian cancer. Ann Oncol (2011) 22(SUPPL.8):23–8. doi: 10.1093/annonc/mdr530
23. Zeng J, Yin J, Song X, Jin Y, Li Y, Pan L. Reduction of CA125 Levels During Neoadjuvant Chemotherapy Can Predict Cytoreduction to No Visible Residual Disease in Patients with Advanced Epithelial Ovarian Cancer, Primary Carcinoma of Fallopian tube and Peritoneal Carcinoma. J Cancer (2016) 7(15):2327–32. doi: 10.7150/jca.16761
24. Nasser S, Lazaridis A, Evangelou M, Jones B, Nixon K, Kyrgiou M, et al. Correlation of pre-operative CT findings with surgical & histological tumor dissemination patterns at cytoreduction for primary advanced and relapsed epithelial ovarian cancer: A retrospective evaluation. Gynecol Oncol (2016) 143(2):264–9. doi: 10.1016/j.ygyno.2016.08.322
25. Brun JL, Rouzier R, Selle F, Houry S, Uzan S, Darai E. Neoadjuvant chemotherapy or primary surgery for stage III/IV ovarian cancer: contribution of diagnostic laparoscopy. BMC Cancer (2009) 9:171. doi: 10.1186/1471-2407-9-171
26. Vizzielli G, Costantini B, Tortorella L, Petrillo M, Fanfani F, Chiantera V, et al. Influence of intraperitoneal dissemination assessed by laparoscopy on prognosis of advanced ovarian cancer: an exploratory analysis of a single-institution experience. Ann Surg Oncol (2014) 21(12):3970–7. doi: 10.1245/s10434-014-3783-6
27. Vizzielli G, Costantini B, Tortorella L, Pitruzzella I, Gallotta V, Fanfani F, et al. A laparoscopic risk-adjusted model to predict major complications after primary debulking surgery in ovarian cancer: A single-institution assessment. Gynecol Oncol (2016) 142(1):19–24. doi: 10.1016/j.ygyno.2016.04.020
28. Gallotta V, Conte C, D’Indinosante M, Capoluongo E, Minucci A, De Rose AM, et al. Prognostic factors value of germline and somatic brca in patients undergoing surgery for recurrent ovarian cancer with liver metastases. Eur J Surg Oncol J Eur Soc Surg Oncol Br Assoc Surg Oncol (2019) 45(11):2096–102. doi: 10.1016/j.ejso.2019.06.023
29. Suidan RS, Ramirez PT, Sarasohn DM, Teitcher JB, Iyer RB, Zhou Q, et al. A multicenter assessment of the ability of preoperative computed tomography scan and CA-125 to predict gross residual disease at primary debulking for advanced epithelial ovarian cancer. Gynecol Oncol (2017) 145(1):27–31. doi: 10.1016/j.ygyno.2017.02.020
30. Stashwick C, Post MD, Arruda JS, Spillman MA, Behbakht K, Davidson SA, et al. Surgical risk score predicts suboptimal debulking or a major perioperative complication in patients with advanced epithelial ovarian, fallopian tube, or primary peritoneal cancer. Int J Gynecol Cancer (2011) 21(8):1422–7. doi: 10.1097/IGC.0b013e31822c7704
31. Shim SH, Lee SJ, Kim SO, Kim SN, Kim DY, Lee JJ, et al. Nomogram for predicting incomplete cytoreduction in advanced ovarian cancer patients. Gynecol Oncol (2015) 136(1):30–6. doi: 10.1016/j.ygyno.2014.11.004
32. Ferrandina G, Sallustio G, Fagotti A, Vizzielli G, Paglia A, Cucci E, et al. Role of CT scan-based and clinical evaluation in the preoperative prediction of optimal cytoreduction in advanced ovarian cancer: a prospective trial. Br J Cancer (2009) 101(7):1066–73. doi: 10.1038/sj.bjc.6605292
33. Axtell AE, Lee MH, Bristow RE, Dowdy SC, Cliby WA, Raman S, et al. Multi-institutional reciprocal validation study of computed tomography predictors of suboptimal primary cytoreduction in patients with advanced ovarian cancer. J Clin Oncol (2007) 25(4):384–9. doi: 10.1200/jco.2006.07.7800
34. Kim HJ, Choi CH, Lee YY, Kim TJ, Lee JW, Bae DS, et al. Surgical outcome prediction in patients with advanced ovarian cancer using computed tomography scans and intraoperative findings. Taiwanese J obstetr Gynecol (2014) 53(3):343–7. doi: 10.1016/j.tjog.2013.10.041
35. Fujwara K, Yoshino K, Enomoto T, Fujita M, Ueda Y, Miyatake T, et al. Usefulness of computed tomography in predicting cytoreductive surgical outcomes for ovarian cancer. Arch Gynecol Obstetr (2011) 284(6):1501–7. doi: 10.1007/s00404-011-1864-3
36. Janco JMT, Glaser G, Kim B, McGree ME, Weaver AL, Cliby WA, et al. Development of a prediction model for residual disease in newly diagnosed advanced ovarian cancer. Gynecol Oncol (2015) 138(1):70–7. doi: 10.1016/j.ygyno.2015.04.013
37. Fourcadier E, Trétarre B, Gras-Aygon C, Ecarnot F, Daurès J-P, Bessaoud F. Under-treatment of elderly patients with ovarian cancer: a population based study. BMC Cancer (2015) 15:937–. doi: 10.1186/s12885-015-1947-9
38. Chan JK, Urban R, Cheung MK, Osann K, Shin JY, Husain A, et al. Ovarian cancer in younger vs older women: a population-based analysis. Br J Cancer (2006) 95(10):1314–20. doi: 10.1038/sj.bjc.6603457
39. Zhou Q, Huang F, He Z, Zuo MZ. Clinicopathological and prognostic significance of platelet count in patients with ovarian cancer. Climacteric J Int Menopause Soc (2018) 21(1):60–8. doi: 10.1080/13697137.2017.1406911
40. Chen JP, Huang QD, Wan T, Tu H, Gu HF, Cao JY, et al. Combined score of pretreatment platelet count and CA125 level (PLT-CA125) stratified prognosis in patients with FIGO stage IV epithelial ovarian cancer. J Ovarian Res (2019) 12(1):72. doi: 10.1186/s13048-019-0544-y
41. du Prel J-B, Röhrig B, Hommel G, Blettner M. Choosing statistical tests: part 12 of a series on evaluation of scientific publications. Dtsch Arztebl Int (2010) 107(19):343–8. doi: 10.3238/arztebl.2010.0343
42. Parikh R, Mathai A, Parikh S, Chandra Sekhar G, Thomas R. Understanding and using sensitivity, specificity and predictive values. Indian J Ophthalmol (2008) 56(1):45–50. doi: 10.4103/0301-4738.37595
43. Ruopp MD, Perkins NJ, Whitcomb BW, Schisterman EF. Youden Index and optimal cut-point estimated from observations affected by a lower limit of detection. Biom J (2008) 50(3):419–30. doi: 10.1002/bimj.200710415
44. Vergote IB, Van Nieuwenhuysen E, Vanderstichele A. How to Select Neoadjuvant Chemotherapy or Primary Debulking Surgery in Patients With Stage IIIC or IV Ovarian Carcinoma. J Clin Oncol (2016) 34(32):3827–8. doi: 10.1200/jco.2016.69.7458
45. Rutten MJ, Gaarenstroom KN, Van Gorp T, van Meurs HS, Arts HJ, Bossuyt PM, et al. Laparoscopy to predict the result of primary cytoreductive surgery in advanced ovarian cancer patients (LapOvCa-trial): a multicentre randomized controlled study. BMC Cancer (2012) 12:31. doi: 10.1186/1471-2407-12-31
46. Fagotti A, Ferrandina G, Fanfani F, Garganese G, Vizzielli G, Carone V, et al. Prospective validation of a laparoscopic predictive model for optimal cytoreduction in advanced ovarian carcinoma. Am J Obstet Gynecol (2008) 199(6):642 e1–6. doi: 10.1016/j.ajog.2008.06.052
47. Rutten MJ, Leeflang MM, Kenter GG, Mol BWJ, Buist M. Laparoscopy for diagnosing resectability of disease in patients with advanced ovarian cancer. Cochrane Database Systemat Rev (2014) (2). doi: 10.1002/14651858.CD009786.pub2/abstract
48. Gallotta V, Bruno M, Conte C, Giudice MT, Davià F, Moro F, et al. Salvage lymphadenectomy in recurrent ovarian cancer patients: Analysis of clinical outcome and BRCA1/2 gene mutational status. Eur J Surg Oncol J Eur Soc Surg Oncol Br Assoc Surg Oncol (2020) 46(7):1327–33. doi: 10.1016/j.ejso.2020.01.035
49. Gallotta V, Ferrandina G, Vizzielli G, Conte C, Lucidi A, Costantini B, et al. Hepatoceliac Lymph Node Involvement in Advanced Ovarian Cancer Patients: Prognostic Role and Clinical Considerations. Ann Surg Oncol (2017) 24(11):3413–21. doi: 10.1245/s10434-017-6005-1
50. Gallotta V, Fanfani F, Fagotti A, Chiantera V, Legge F, Gueli Alletti S, et al. Mesenteric lymph node involvement in advanced ovarian cancer patients undergoing rectosigmoid resection: prognostic role and clinical considerations. Ann Surg Oncol (2014) 21(7):2369–75. doi: 10.1245/s10434-014-3558-0
51. Schorge JO, Clark RM, Lee SI, Penson RT. Primary debulking surgery for advanced ovarian cancer: Are you a believer or a dissenter? Gynecol Oncol (2014) 135(3):595–605. doi: 10.1016/j.ygyno.2014.10.007
52. Llueca A, Serra A, Delgado K, Maiocchi K, Jativa R, Gomez L, et al. A radiologic-laparoscopic model to predict suboptimal (or complete and optimal) debulking surgery in advanced ovarian cancer: a pilot study. Int J Womens Health (2019) 11:333–42. doi: 10.2147/ijwh.s198355
53. Llueca A, Escrig J, Serra-Rubert A, Gomez-Quiles L, Rivadulla I, Játiva-Porcar R, et al. Prognostic value of peritoneal cancer index in primary advanced ovarian cancer. Eur J Surg Oncol (2018) 44(1):163–9. doi: 10.1016/j.ejso.2017.11.003
54. Gueli Alletti S, Vizzielli G, Lafuenti L, Costantini B, Fagotti A, Fedele C, et al. Single-Institution Propensity-Matched Study to Evaluate the Psychological Effect of Minimally Invasive Interval Debulking Surgery Versus Standard Laparotomic Treatment: From Body to Mind and Back. J Minim Invasive Gynecol (2018) 25(5):816–22. doi: 10.1016/j.jmig.2017.12.007
55. Gueli Alletti S, Bottoni C, Fanfani F, Gallotta V, Chiantera V, Costantini B, et al. Minimally invasive interval debulking surgery in ovarian neoplasm (MISSION trial-NCT02324595): a feasibility study. Am J Obstet Gynecol (2016) 214(4):503.e1–.e6. doi: 10.1016/j.ajog.2015.10.922
56. Fagotti A, Gueli Alletti S, Corrado G, Cola E, Vizza E, Vieira M, et al. The INTERNATIONAL MISSION study: minimally invasive surgery in ovarian neoplasms after neoadjuvant chemotherapy. Int J Gynecol Cancer (2019) 29(1):5–9. doi: 10.1136/ijgc-2018-000012
Keywords: ovarian cancer, computed tomography scans, prediction model, primary debulking surgery, neoadjuvant chemotherapy, multicenter study
Citation: Gu Y, Qin M, Jin Y, Zuo J, Li N, Bian C, Zhang Y, Li R, Wu Y-m, Wang C-y, Zhang K-q, Yue Y, Wu L-y and Pan L-y (2021) A Prediction Model for Optimal Primary Debulking Surgery Based on Preoperative Computed Tomography Scans and Clinical Factors in Patients With Advanced Ovarian Cancer: A Multicenter Retrospective Cohort Study. Front. Oncol. 10:611617. doi: 10.3389/fonc.2020.611617
Received: 29 September 2020; Accepted: 18 November 2020;
Published: 07 January 2021.
Edited by:
Min Wu, Sichuan University, ChinaReviewed by:
Antoni Llueca Abella, University Jaume I (UJI), SpainValerio Gallotta, Catholic University of the Sacred Heart, Italy
Giuseppe Vizzielli, Catholic University of the Sacred Heart, Italy
Copyright © 2021 Gu, Qin, Jin, Zuo, Li, Bian, Zhang, Li, Wu, Wang, Zhang, Yue, Wu and Pan. This is an open-access article distributed under the terms of the Creative Commons Attribution License (CC BY). The use, distribution or reproduction in other forums is permitted, provided the original author(s) and the copyright owner(s) are credited and that the original publication in this journal is cited, in accordance with accepted academic practice. No use, distribution or reproduction is permitted which does not comply with these terms.
*Correspondence: Ling-ya Pan, cGFubHlAcHVtY2guY24=; Ling-ying Wu, d3VsaW5neWluZ0Bjc2NvLm9yZy5jbg==
†These authors have contributed equally to this work