- 1Liver Disease Center, The First Affiliated Hospital of Fujian Medical University, Fuzhou, China
- 2The United Innovation of Mengchao Hepatobiliary Technology Key Laboratory of Fujian Province, Mengchao Hepatobiliary Hospital of Fujian Medical University, Fuzhou, China
- 3The Liver Center of Fujian Province, Fujian Medical University, Fuzhou, China
- 4Department of Computer Science, Shanghai Jiao Tong University, Shanghai, China
Surgical resection remains primary curative treatment for patients with hepatocellular carcinoma (HCC) while over 50% of patients experience recurrence, which calls for individualized recurrence prediction and early surveillance. This study aimed to develop a machine learning prognostic model to identify high-risk patients after surgical resection and to review importance of variables in different time intervals. The patients in this study were from two centers including Eastern Hepatobiliary Surgery Hospital (EHSH) and Mengchao Hepatobiliary Hospital (MHH). The best-performed model was determined, validated, and applied to each time interval (0–1 year, 1–2 years, 2–3 years, and 3–5 years). Importance scores were used to illustrate feature importance in different time intervals. In addition, a risk heat map was constructed which visually depicted the risk of recurrence in different years. A total of 7,919 patients from two centers were included, of which 3,359 and 230 patients experienced recurrence, metastasis or died during the follow-up time in the EHSH and MHH datasets, respectively. The XGBoost model achieved the best discrimination with a c-index of 0.713 in internal validation cohort. Kaplan-Meier curves succeed to stratify external validation cohort into different risk groups (p < 0.05 in all comparisons). Tumor characteristics contribute more to HCC relapse in 0 to 1 year while HBV infection and smoking affect patients’ outcome largely in 3 to 5 years. Based on machine learning prediction model, the peak of recurrence can be predicted for individual HCC patients. Therefore, clinicians can apply it to personalize the management of postoperative survival.
Introduction
Hepatocellular carcinoma (HCC) is the most common primary liver cancer and ranks as the fourth leading cause of cancer-related mortality (8.2%) worldwide (1). Surgical resection remains the primary curative treatment for patients with adequate liver function (2). However, 50% to 70% of patients who undergo complete tumor resection still suffer from frequent recurrence and disease progression, ultimately leading to unfavorable prognoses (3). Therefore, the identification of patients at high risk of recurrence after surgical resection is essential for clinicians to provide appropriate surveillance and therapy.
During the past decade, researchers have primarily focused on prognosis-predictive models based on biological, demographic, and clinical factors. The most acknowledged system of the American Joint Committee on Cancer (AJCC) tumor-node-metastasis (TNM) is commonly used to determine the staging of liver cancer. However, its prognostic value in predicting tumor recurrence is widely debated (4). Recent models, including the Singapore Liver Cancer Recurrence (SLICER) score, Surgery-Specific Cancer of the Liver Italian Program (SS-CLIP), and the Korean model, were designed to detect tumor recurrence in specific groups of patients. Due to the inaccuracy and diversity of these models, they have not been widely implemented (5–7). In addition, the Early Recurrence After Surgery for Liver tumor (ERASL) model, which is based on Cox regression analysis, has been established to predict early tumor recurrence after liver resection. Despite its better discriminatory performances than other tools, the limited clinical parameters and the prediction for 2-year recurrence restrict its application in the full HCC survivorship management (8).
Machine learning, a field of computer science in which machines mimic, recognize, and learn cognitive functions of the human mind to make empirical predictions, is gaining more and more attention in recent years (9). For cancer, machine learning demonstrates the advantages of image recognition and feature selection compared to traditional methods (10, 11). Recently, automated machine learning algorithms have been developed to detect metastasis in sentinel lymph nodes of women with breast cancer, and showed better diagnostic performance than pathologists (12). In patients with bladder cancer, a novel predictive model based on machine learning algorithms was also created. In the model, disease recurrence after cystectomy was predicted with more than 70% sensitivity and specificity (13). However, few studies have applied a machine learning framework to identify HCC patients with the potential risk of recurrence after curative treatment.
Briefly, we aimed to utilize machine learning algorithms to develop a risk prediction model to predict HCC recurrence among patients who underwent surgical resection. We also explored feature importance in this process, verifying the important prognostic factors for tumor relapse. In addition, a risk heat map covering five years that visually depicts the risk of recurrence was constructed. In this way, we hope to improve the performance of HCC recurrence predictive models using big data and to provide evidential support for individualized management.
Materials and Methods
This analysis was reported according to the TRIPOD (Transparent Reporting of a Multivariable Prediction Model for Individual Prognosis or Diagnosis) guidelines (14).
Patients
The database was retrospectively derived from patients with HCC who underwent hepatic resection at Eastern Hepatobiliary Surgery Hospital, Second Military Medical University (EHSH) (n = 7,411, from May 2008 to Sept. 2018) or Mengchao Hepatobiliary Hospital, Fujian Medical University (MHH) (n = 508, from Nov. 2014 to Nov. 2018). The patients in this study met the inclusion criteria as follows: (1) pathological confirmation of HCC, (2) Child-Pugh A/B before surgery, (3) R0 surgical resection of tumor with curative intent. However, patients who (1) died within 30 days after surgery or lost to follow-up, (2) received preoperative neoadjuvant treatment (3) diagnosed with extrahepatic cancers, HCC relapse, or metastasis (4) younger than 18 years old were excluded from this study. Inclusion and exclusion of patients and following analysis can be found in Supplementary Figure 1.
Different models were constructed on the EHSH dataset, which was randomly divided into derivation and internal validation cohorts at a ratio of 8:2. The models were validated externally using the dataset from MHH. The study was approved by the Ethics Committee of the two centers, and the requirement of written informed consent was waived. All procedures were performed in accordance with the Declaration of Helsinki.
Clinical Variables
The demographics, laboratory tests, and HCC etiologies were collected from the database. The laboratory tests included various parameters of blood examination, liver and coagulation function, and hepatitis virus markers. Tumor characteristics included, but were not limited to, the number of tumors, the diameter of the largest nodule, differentiation, capsule, cirrhosis in non-cancerous tissues, and vascular invasion. Macrovascular invasion was defined as tumor invasion of large vessels, which can be detected by Computed Tomography/Magnetic Resonance Imaging (CT/MRI) (8). Microvascular invasion refers to the histologically microscopic presence of cancer cell clusters in the blood vessels lined with endothelial cells (15). Thirty-five variables were selected by health professionals based on literature review and clinical expertise.
Follow-up and Outcome
During the follow-up, serum alpha-fetoprotein (AFP) levels were measured, as well as ultrasonography, CT, or MRI of the chest and abdomen once every two months for six months, and then once every three months for the next 1.5 years. For patients who were free of cancer recurrence two years after surgery, a 6-month interval surveillance was carried out. The outcome of this study, recurrence-free survival (RFS), was defined as the time from surgery to the detection of recurrence, metastasis, or death.
General Statistical Principle
After preliminary data cleaning, multiple imputation was performed in R (v3.6.2) based on the Multivariate Imputation by Chained Equations (MICE, v3.8.0). Continuous variables, which were tested for normality by Anderson-Darling tests, were abnormally distributed. Therefore, the variables were summarized by median (IQR), and Wilcoxon rank-sum tests were used for between-groups comparisons. Categorical variables were expressed as frequency (%), and Chi-squared tests or Fisher’s exact tests were applied, as appropriate. All statistical analyses above were two-sided, while p < 0.05 was considered statistically significant, and conducted in Python (v3.7) with Scipy (v1.4.0) package.
Model Development
Cox Proportional Hazards Model (CPH)
The clinicopathologic parameters of HCC recurrence were fitted by the Cox regression using the Survival package (v3.1) in R-language. Univariable Cox regression was firstly conducted to identify potential predictors (p < 0.1). Variables identified in univariable cox model were then applied in multivariable cox regression with stepwise selection method.
Machine Learning Models
Three machine learning models, including Deep Learning-based Survival Model (DeepSurv), Extreme Gradient Boosting (XGBoost), and Random survival forest (RSF) were applied to perform the task of predicting HCC recurrence using all 35 variables preselected. DeepSurv is a multi-layer feed-forward neural network that predicts the effects of diverse variables on their hazard rate parameterized by the weights of the network (16). Based on its algorithm principle, we redeveloped DeepSurv in Python under Pytorch deep learning framework (version 1.3.1, CPU version) and optimized the hyper-parameter search. XGBoost is an improved supervised learning algorithm based on the Gradient Boosting Decision Tree algorithm, which can deal with survival problems by setting partial likelihood functions of the optimization object and log-rank tests as node split criteria (17). Our XGBoost model was implemented in Python using the XGBoost (v.0.9) package. RSF is another machine learning approach for survival analysis that eliminates the proportional hazard assumption and can fit a more general spectrum of survival problems, which conducted in R (randomForestSRC v2.9.3) (18).
Model Discrimination and Calibration
The discrimination performance among the four models in both derivation and validation sets were measured by Harrell’s c-index. Comparison of c-index among different models in each cohort was conducted afterwards (19).
As suggested by previous study Kaplan-Meier survival curves for various risk groups were used as informal evidence of discriminative ability (20). Kaplan-Meier curve for the external validation cohort after calibration allows a visual comparison of discrimination among different risk groups at the cut-off of 50th and 84th centiles.
Calibration plots of XGBoost were applied to the derivation and validation sets to determine whether each patient’s predicted risk was consistent with the actual outcome. We followed the practice of Chan et al. to draw the calibration plots (8) at 1, 2, 3, and 5 years.
Models in Different Time Intervals and Predictive Heat Map
Inspired by lifetable methodology, we applied XGBoost to different time intervals, including 0 to 1 year, 1 to 2 years, 2 to 3 years, and 3 to 5 years, with the same software. Importance scores were exported, and the Harrell’s c-index of each interval were reported at the same time. Furthermore, fifty patients from the external validation cohort were randomly selected to create a heat map for visually illustrating the risk of recurrence within five years after surgery, with aim of providing guidance and support in clinical practice.
Results
Clinicopathologic Features and Outcome
A total of 7,919 patients who underwent surgical resection from two centers were included in the study. 80% of EHSH cohort was assigned as the derivation set (n = 5,928) and the rest was designated as internal validation set (n = 1,483). By the time of data analysis, 3,359 and 230 patients experienced recurrence, metastasis or died during the follow-up time in the EHSH dataset and MHH datasets, respectively. Median follow-up period for two datasets were 3.51 (IQR: 0.41–8.32) and 2.04 (IQR: 0.23–3.88) years. Detailed outcome descriptions are provided in Supplementary Table 1.
Thirty-five predictors were included in the final analysis. Preoperative clinical and postoperative pathologic characteristics of the three cohorts are shown in Table 1.
Predictive Performance
The discriminatory performance of the four models was assessed with the Harrell’s c-index (Table 2). The c-index of the Cox regression model in three cohorts were 0.704 (EHSH derivation), 0.700 (EHSH validation), and 0.703 (MHH validation). Among four models, XGBoost achieved the highest c-index in the internal validation cohort (c-index: 0.713, P < 0.05, all comparisons). The c-index of XGBoost in the external validation cohort of MHH is 0.697, no statistically significant difference from those of CPH, DeepSurv, and RSF (0.703, P = 0.470; 0.700, P = 0.616; and 0.699, P = 0.672; respectively). Meanwhile, XGBoost model outperformed the Early Recurrence After Surgery for Liver tumor (ERASL) model (c-index: 0.672, P < 0.001; 0.673, P < 0.001; and 0.679, P = 0.185) in all three cohorts with our dataset. Thus, XGBoost was employed for the following demonstration and analysis. KM curves of the external validation dataset (Figure 1) indicated good discriminative ability of XGBoost to categorize patients into three risk groups after resection: low risk, intermediate risk (p < 0.001 in comparison to the low-risk group), high risk (p < 0.001 in comparison to the intermediate-risk group).
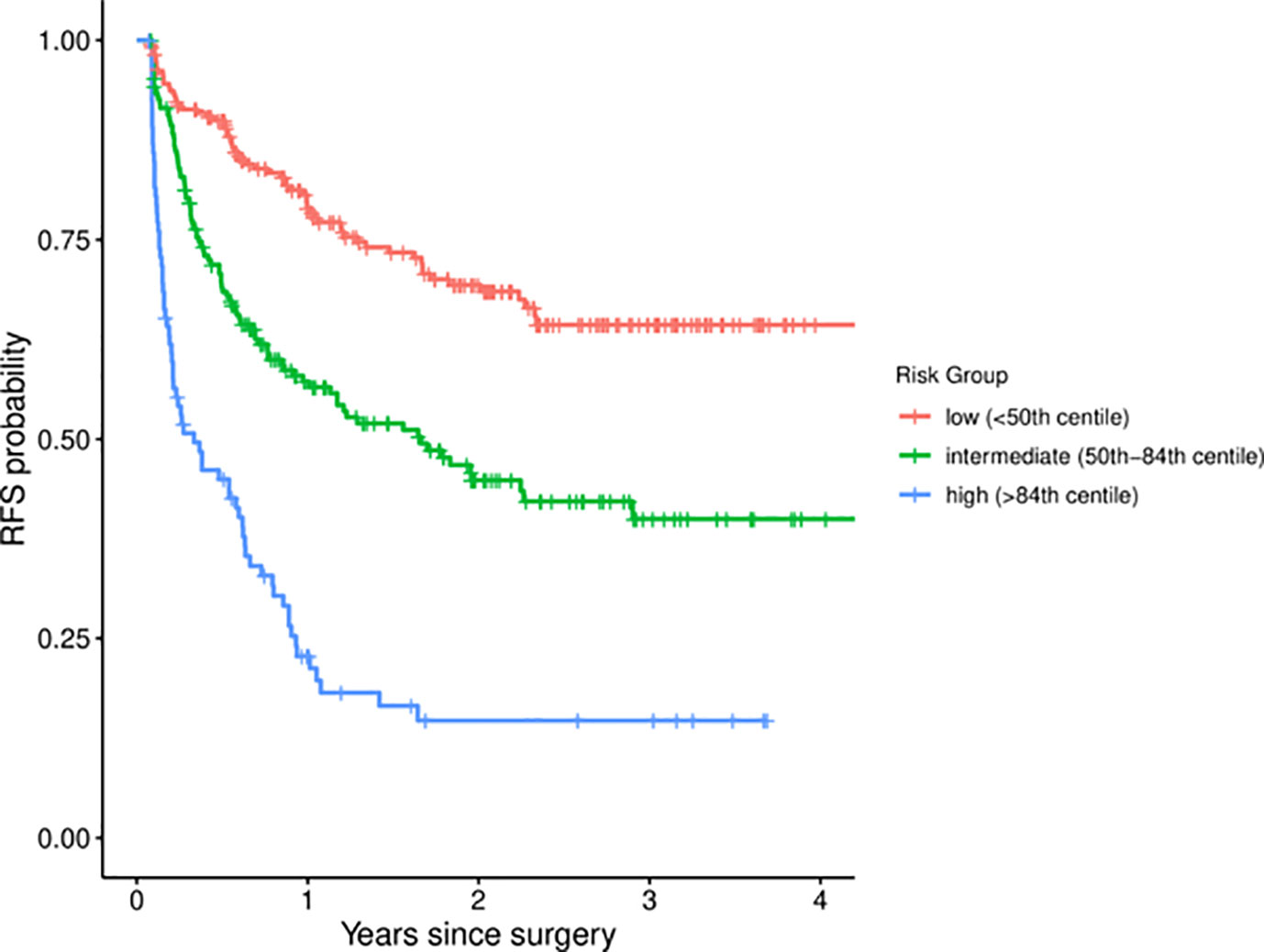
Figure 1 Kaplan-Meier curves for different risk groups among MHH patients. MHH, Mengchao Hepatobiliary Hospital.
As shown in Figure 2, the calibration plots demonstrated a satisfying agreement between predictions made by XGBoost and actual patient outcomes in all datasets.
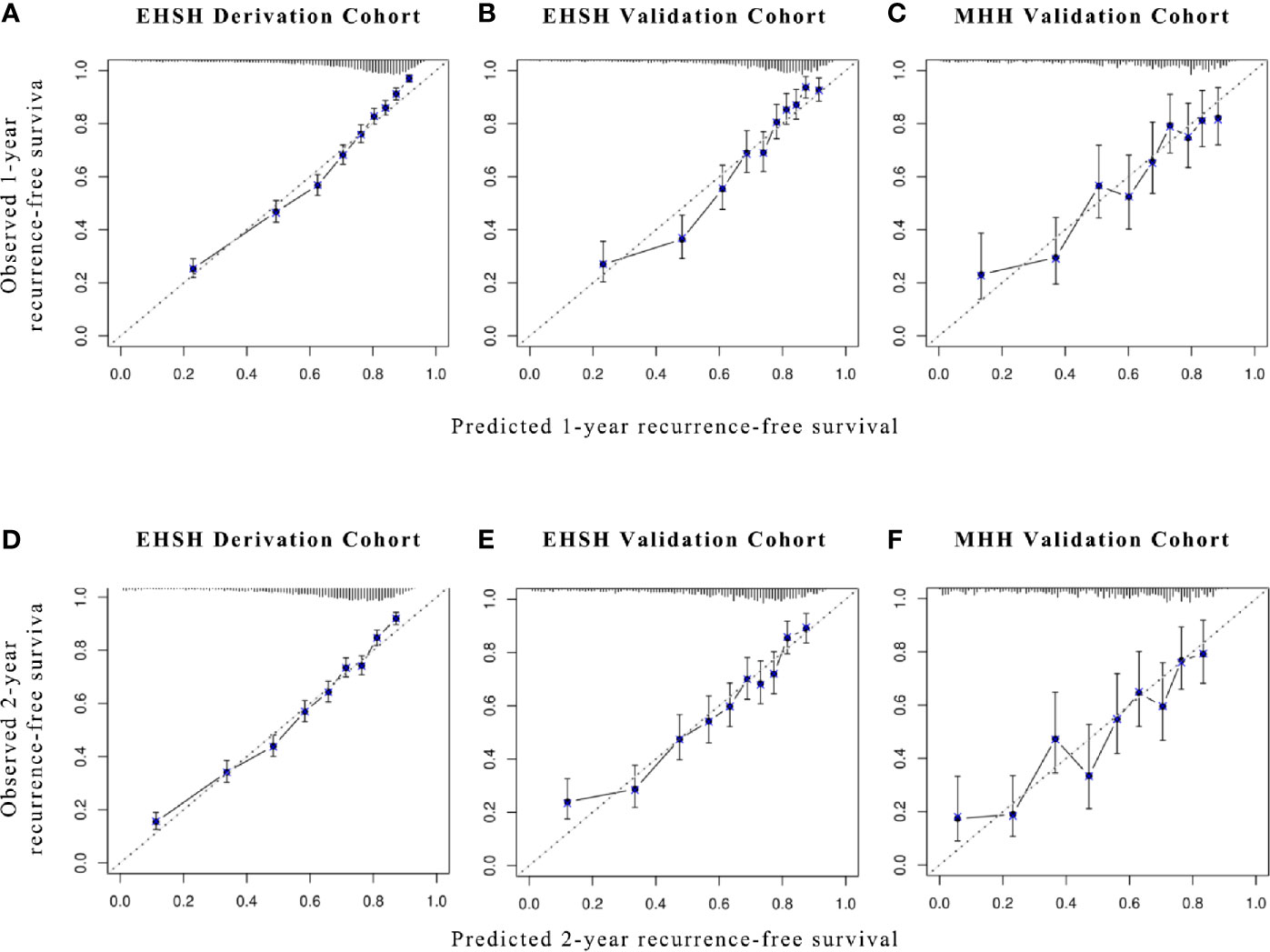
Figure 2 Calibration plots for XGBoost models in predicting 1- and 2-year RFS. Calibration plots for (A, D) EHSH derivation cohort, (B, E) EHSH validation cohort, and (C, F) MHH validation cohort in predicting 1-year (A–C) and 2-year RFS (D–F). RFS, recurrence-free survival.
Models and Feature Importance in Different Time Intervals
We established the XBGoost model in different time intervals, including 0 to 1 year, 1 to 2 year, 2 to 3 year, and 3 to 5 years, to examine the dynamics of feature importance in HCC patients. The specific predictive performance measurements using c-index and 95% CI for each time slot are listed in Table 3.
The variables with the top 10 importance scores are shown in Table 4. During 0 to 1 year after resection, the importance score of tumor thrombus (defined as the tumor extending into a vessel, typically portal vein) was 103.01, substantially higher than scores of other factors, such as tumor diameter (33.94), gamma-glutamyl transpeptidase (GGT) (20.25), and tumor capsule (19.22). For 1 to 2 year, tumor number (13.39) was the most important variable related with patient outcomes, followed by resection type (major resection 13.22), tumor thrombus (13.04), and tumor diameter (12.36). In the latter two intervals, apart from tumor number, HBV infection was found to be a relatively important variable. HBV-DNA load has the third highest importance score for 2 to 3 years and HBsAg ranked first in the last period. Furthermore, smoking, an unhealthy lifestyle, was also associated with late recurrence.
The Pattern of Recurrence Risk
Using the XGBoost model in different time intervals, a risk heat map covering four time intervals was developed that visually depicts a patient’s risk of tumor recurrence, metastasis or death after undergoing curative liver resection. In general, individual heat map indicated a trend of relatively high recurrence risk in 0 to 1 year and 3 to 5 years after surgical resection (Figure 3).
Discussion
HCC is one of the most common malignancies worldwide. Though curative resection offers the best prognosis for patients, disease recurrence remains a major obstacle to the long-term survival of patients (21). Moreover, little is known about the potential risk and peak time periods of HCC recurrence after curative surgery (22, 23). We therefore conducted this research to mediate this gap. In this study, the risk prediction model based on the XGBoost algorithm showed the best c-index in the EHSH validation set. To observe the recurrence risk of individual patients at different time intervals post-surgery, a heat map was constructed based on the XGBoost model for 50 randomly selected HCC patients. The majority of patients had a similar trend of postoperative recurrence that risks in 0 to 1 and 3 to 5 years after surgery were higher than those in 1 to 2 and 2 to 3 years.
In the past few years, several scoring systems have been developed for estimating HCC recurrence risk and stratifying patients. These systems have primarily selected significant clinical parameters through multivariate analyses and constructed conventional Cox proportional hazard models based on the limited risk factors (24–26). One of the important assumptions for Cox proportional hazards regression is that each variable makes linear contribution to model. However, in clinical studies, multiple risk factors usually have non-linear effects with recurrence-free survival, especially in cancer studies (16, 27, 28). Due to this reason, the previous models might fail to show goodness-of-fit and to make accurate prediction. Machine learning algorithms are probably superior than conventional CPH because they can fit more sophisticated non-linear relationship. According to our attempts of building different models, the XGBoost model did better prediction of liver recurrence.
Apart from an individualized heatmap for illustrating recurrence risk, a feature importance analysis was conducted based on the XGBoost model and was used to evaluate dynamics of variables contributing to the interesting outcome. Specifically, tumor characteristics, such as tumor thrombus, tumor number, tumor size, and tumor differentiation, contributed more to the model’s predictive performance in our study. In addition, macrovascular invasion (MaVI), microvascular invasion (MVI), gamma-glutamyl transpeptidase (GGT), intraoperative blood transfusion and major resection also showed a more significant contribution to the predictive performance of the model. Furthermore, smoking as an unhealthy lifestyle also hampered prognosis of HCC patients. These findings are supported by previous research as follows.
Firstly, previous studies found that patients with portal vein tumor thrombosis (PVTT) usually decreased liver function reserves, which was a high-risk factor for disease progression and recurrence (29, 30). In addition to tumor thrombus, tumor volume is also associated with HCC recurrence. In another study, tumor volume was shown to be a predictor of HCC recurrence after liver transplantation (31). A clinical study in Korea confirmed that the maximal size of HCC and the number of tumors were significantly correlated with the recurrence of HCC after liver transplantation (32). In line with our results, MVI was also a unique parameter assessed in the ERASL, SLICER, SS-CLIP, and Korean models (5–8). The dissemination and spread of tumors through micro-vessels may explain the advanced tumor stage, tumor progression, and worse outcomes (33–35).
Secondly, perioperative blood transfusions were independently associated with survival and cancer recurrence after surgical resection (36). A meta-analysis found that allogeneic blood transfusions were associated with poor clinical prognoses in patients with HCC who underwent radical hepatectomy (37). The association between major resection and blood loss as well as RFS of HCC patients has been examined: the more complicated hepatectomy is, the more likely patients are to suffer from intraoperative blood loss, leading to shorter time to recurrence (38).
Thirdly, liver function presented by GGT was another crucial prognostic factor to predict tumor recurrence (39). GGT was first found to modulate the metabolism of glutathione (GSH) and facilitate amino-acid recovery for GSH synthesis (40). Recently, GGT was reported to be involved in tumor initiation, progression, and invasion. As such, GGT may induce the production of endogenous reactive oxygen species (ROS), leaving cells exposed to persistent oxidative stress, leading to DNA damage and tumor growth (41, 42).
Moreover, smoking was associated with an increased risk of HCC (43, 44) and disease-free survival of patients who underwent resection (45). In the current study, we found that smoking was associated with a recurrence risk of 2 to 3 and 3 to 5 years after HCC. The underlying mechanism might be that nicotine increases the expression of α-7-nicotinic acetylcholine receptor (α-7-nAChR), leading to recurrence through the JAK2/STAT3 signaling pathway (46). A previous study found that the history and amount of smoking were both risk factors for the progressive recurrence of HBV-related HCC (47).
Finally, early disease recurrence (0–1 year) is often thought to be a result of intrahepatic metastases, while late recurrence is more likely to result from newly-onset tumors with multicenter origins (48, 49). In accordance with this theory, HBV-DNA load and HBsAg contribute significantly to HCC recurrence from two to five years in our study, which likely induce genomic alternations and pro-oncotic signaling for de novo HCC in the long term (50).
Our results suggest that clinicians can provide personalized management of recurrence risk after surgical resection in HCC patients based on information provided by heat maps and feature importance, which may improve postoperative survival outcomes. The risk heat map allows clinical teams to detect patients most at risk of HCC recurrence, schedule appointments for them in the “heat zones” that most likely for recurrence, and take interventions as needed. For example, clinicians may give greater attention to malignant characteristics of tumors, including the presence of tumor thrombus, larger tumor sizes, multiple tumor nodules, and micro- or macro-vascular invasion, if the heat map indicates a high risk within one year after surgery.
There are certain underlying limitations to our study. Firstly, our model is primarily based on two Chinese institutions of patients with HCC in hepatitis B virus-endemic areas. It is necessary to validate our model in international cohorts to extend our results to patients with HCC of various etiologies. Second, some other variables that may be associated with the prognosis of HCC patients, such as postoperative adjunctive therapies and serum inflammatory markers, were not evaluated in this study. In addition, further prospective studies with longer follow-ups are essential to extend the performance of our model further.
In summary, we have developed a model based on a machine learning algorithm that better predicts the risk of disease recurrence in individual patients following hepatic resection in a large population. We further applied this model to four time periods to describe patterns of HCC relapse, and to explore important risk factors. The heat map offers clinicians a decision support tool to identify individuals prone to recurrence, while also allowing clinicians to identify the prognostic factors, which are clinically useful in terms of individualized patient monitoring, surveillance, and management. Future prospective studies are needed to verify our conclusions.
Data Availability Statement
The raw data supporting the conclusions of this article will be made available by the authors, upon reasonable request.
Author Contributions
YH and HC contributed equally to the manuscript as they both took charge of study design and implementation, as well as drafting manuscript. YZ also participated in study design and literature review. Both ZL and HM conducted statistical and machine learning analysis. Corresponding author, JL, made a huge contribution to the manuscript in terms of revising the draft and reviewing the final version. All authors contributed to the article and approved the submitted version.
Funding
This study was funded by Startup Fund for scientific research, Fujian Medical University (Grant Number: 2019QH1297).
Conflict of Interest
The authors declare that the research was conducted in the absence of any commercial or financial relationships that could be construed as a potential conflict of interest.
Acknowledgments
Thanks to all the staff of Mengchao Hepatobiliary Hospital who contributed to the study.
Supplementary Material
The Supplementary Material for this article can be found online at: https://www.frontiersin.org/articles/10.3389/fonc.2020.593741/full#supplementary-material
Supplementary Figure 1 | Flowchart of inclusion and exclusion criteria and analysis strategy.
Abbreviations
α-7-nAChR, 7-nicotinic acetylcholine receptor; APTT, Activated partial thromboplastin time; ALBI, Albumin-bilirubin grade; AJCC, American Joint Committee on Cancer; CPH, Cox Proportional Hazards Model; DeepSurv, Deep Learning-based Survival Model; ERASL, Early Recurrence After Surgery for Liver tumor model; EHSH, Eastern Hepatobiliary Surgery Hospital, Second Military Medical University; XGBoost, Extreme Gradient Boosting; FLD, Fatty liver disease; GSH, glutathione; HBG, Hemoglobin concentration; HCC, Hepatocellular carcinoma; HBV, Hepatitis B virus; MaVI, Macrovascular invasion; MHH, Mengchao Hepatobiliary Hospital, Fujian Medical University; MVI, Microvascular invasion; PVTT, Portal vein tumor thrombosis; PT, Prothrombin time; RSF, Random survival forest; ROS, Reactive oxygen species; RFS, Recurrence-free survival; AFP, Serum alpha-fetoprotein; SLICER, Singapore Liver Cancer Recurrence score; SS-CLIP, Surgery-Specific Cancer of the Liver Italian Program; TT, Thrombin time; TBIL, Total bilirubin.
References
1. Bray F, Ferlay J, Soerjomataram I, Siegel RL, Torre LA, Jemal A. Global cancer statistics 2018: GLOBOCAN estimates of incidence and mortality worldwide for 36 cancers in 185 countries. CA Cancer J Clin (2018) 68(6):394–424. doi: 10.3322/caac.21492
2. Netbook N.C.C. Hepatobiliary Cancers1 (Version 4.2019). (2019). Available at: https://www.nccn.org/professionals/physician_gls/pdf/hepatobiliary.pdf.
3. Tabrizian P, Jibara G, Shrager B, Schwartz M, Roayaie S. Recurrence of hepatocellular cancer after resection: patterns, treatments, and prognosis. Ann Surg (2015) 261(5):947–55. doi: 10.1097/SLA.0000000000000710
4. Zhang JF, Shu ZJ, Xie CY, Li Q, Jin XH, GU W, et al. Prognosis of unresectable hepatocellular carcinoma: comparison of seven staging systems (TNM, Okuda, BCLC, CLIP, CUPI, JIS, CIS) in a Chinese cohort. PloS One (2014) 9(3):e88182. doi: 10.1371/journal.pone.0088182
5. Ang SF, Ng ESH, Li H, Ong YH, Choo SP, Ngeow J, et al. The Singapore Liver Cancer Recurrence (SLICER) Score for relapse prediction in patients with surgically resected hepatocellular carcinoma. PloS One (2015) 10(4):e0118658. doi: 10.1371/journal.pone.0118658
6. Huang S, Huang GQ, Zhu GQ, Liu WY, You J, Shi KQ, et al. Establishment and Validation of SSCLIP Scoring System to Estimate Survival in Hepatocellular Carcinoma Patients Who Received Curative Liver Resection. PloS One (2015) 10(6):e0129000. doi: 10.1371/journal.pone.0129000
7. Shim JH, Jun MJ, Han S, Lee YJ, Lee SG, Kim KM, et al. Prognostic nomograms for prediction of recurrence and survival after curative liver resection for hepatocellular carcinoma. Ann Surg (2015) 261(5):939–46. doi: 10.1097/SLA.0000000000000747
8. Chan AWH, Zhong J, Berhane S, Toyoda H, Cucchetti A, Shi K, et al. Development of pre and post-operative models to predict early recurrence of hepatocellular carcinoma after surgical resection. J Hepatol (2018) 69(6):1284–93. doi: 10.1016/j.jhep.2018.08.027
9. Camacho DM, Collins KM, Powers RK, Costello JC, Collins JJ. Next-Generation Machine Learning for Biological Networks. Cell (2018) 173(7):1581–92. doi: 10.1016/j.cell.2018.05.015
10. Deo RC. Machine Learning in Medicine. Circulation (2015) 132(20):1920–30. doi: 10.1161/CIRCULATIONAHA.115.001593
11. Singal AG, Mukherjee A, Elmunzer BJ, Higgins PD, Lok AS, Zhu J, et al. Machine learning algorithms outperform conventional regression models in predicting development of hepatocellular carcinoma. Am J Gastroenterol (2013) 108(11):1723–30. doi: 10.1038/ajg.2013.332
12. Ehteshami Bejnordi B, Veta M, Johannes van Diest P, van Ginneken B, Karssemeijer N, Litjens G, et al. Diagnostic Assessment of Deep Learning Algorithms for Detection of Lymph Node Metastases in Women With Breast Cancer. Jama (2017) 318(22):2199–210. doi: 10.1001/jama.2017.14580
13. Hasnain Z, Mason J, Gill K, Miranda G, Gill IS, Kuhn P, et al. Machine learning models for predicting post-cystectomy recurrence and survival in bladder cancer patients. PloS One (2019) 14: (2):e0210976. doi: 10.1371/journal.pone.0210976
14. Moons KM, Altman DG, Reitsma JB, Ioannidis JP, Macaskill P, Steyerberg EW, et al. Transparent Reporting of a multivariable prediction model for Individual Prognosis or Diagnosis (TRIPOD): explanation and elaboration. Ann Internal Med (2015) 162(1):1. doi: 10.7326/M14-0698
15. Zhou J, Sun HC, Wang Z, Cong WM, Wang JH, Zeng MS, et al. Guidelines for diagnosis and treatment of primary liver cancer in China (2017 Edition). Liver Cancer (2018) 7(3):235–60. doi: 10.1159/000488035
16. Katzman JL, Shaham U, Cloninger A, Bates J, Jiang T, Kluger Y, et al. DeepSurv: personalized treatment recommender system using a Cox proportional hazards deep neural network. BMC Med. Res. Methodol. (2018) 18: (1):24. doi: 10.1186/s12874-018-0482-1
17. Babajide Mustapha I, Saeed F. Bioactive Molecule Prediction Using Extreme Gradient Boosting. Molecules (2016) 21(8):983. doi: 10.3390/molecules21080983
18. Wang H, Zhou L. Random survival forest with space extensions for censored data. Artif Intell Med (2017) 79:52–61. doi: 10.1016/j.artmed.2017.06.005
19. Kang L, Chen W, Petrick NA, Gallas BD. Comparing two correlated C indices with right-censored survival outcome: a one-shot nonparametric approach. Stat Med (2015) 34(4):685–703. doi: 10.1002/sim.6370
20. Royston P, Altman DG. External validation of a Cox prognostic model: principles and methods. BMC Med Res Method (2013) 13(1):33–3. doi: 10.1186/1471-2288-13-33
21. Forner A, Reig M, Bruix J. Hepatocellular carcinoma. Lancet (2018) 391(10127):1301–14. doi: 10.1016/S0140-6736(18)30010-2
22. Bruix J, Reig M, Sherman M. Evidence-Based Diagnosis, Staging, and Treatment of Patients With Hepatocellular Carcinoma. Gastroenterology (2016) 150(4):835–53. doi: 10.1053/j.gastro.2015.12.041
23. Mahvi DA, Liu R, Grinstaff MW, Colson YL, Raut CP. Local Cancer Recurrence: The Realities, Challenges, and Opportunities for New Therapies. CA Cancer J Clin (2018) 68(6):488–505. doi: 10.3322/caac.21498
24. Kamarajah SK, Frankel TL, Sonnenday C, Cho CS, Natahan H. Critical evaluation of the American Joint Commission on Cancer (AJCC) 8th edition staging system for patients with Hepatocellular Carcinoma (HCC): A Surveillance, Epidemiology, End Results (SEER) analysis. J Surg Oncol (2018) 117(4):644–50. doi: 10.1002/jso.24908
25. Cho HJ, Kim B, Lee JD, Kang DR, Kim JK, Lee JH, et al. Development of Risk Prediction Model for Hepatocellular Carcinoma Progression of Indeterminate Nodules in Hepatitis B Virus-Related Cirrhotic Liver. Am J Gastroenterol (2017) 112(3):460–70. doi: 10.1038/ajg.2016.480
26. Sasaki K, Firl DJ, Hashimoto K, Fujiki M, Diago-Uso T, Quintini C, et al. Development and validation of the HALT-HCC score to predict mortality in liver transplant recipients with hepatocellular carcinoma: a retrospective cohort analysis. Lancet Gastroenterol Hepatol (2017) 2(8):595–603. doi: 10.1016/S2468-1253(17)30106-1
27. Rajkomar A, Dean J, Kohane I. Machine Learning in Medicine. N Engl J Med (2019) 380(14):1347–58. doi: 10.1056/NEJMra1814259
28. Austin PC, Pencinca MJ, Steyerberg EW. Predictive accuracy of novel risk factors and markers: A simulation study of the sensitivity of different performance measures for the Cox proportional hazards regression model. Stat Methods Med Res (2017) 26(3):1053–77. doi: 10.1177/0962280214567141
29. Liu PH, Huo TI, Miksad RA. Hepatocellular Carcinoma with Portal Vein Tumor Involvement: Best Management Strategies. Semin Liver Dis (2018) 38(3):242–51. doi: 10.1055/s-0038-1666805
30. Li M, Zhao Y, Liu X, Zhang S, Jiang Y, Yang Z. Early risk warning system for distant metastasis of hepatitis B virus-associated hepatocellular carcinoma with portal vein tumor thrombus. Oncol Lett (2020) 19(4):3249–57. doi: 10.3892/ol.2020.11423
31. Kashkoush S, El Moghazy W, Kawahara T, Gala-Lopez B, Toso C, Kneteman NM. Three-dimensional tumor volume and serum alpha-fetoprotein are predictors of hepatocellular carcinoma recurrence after liver transplantation: refined selection criteria. Clin Transplant (2014) 28(6):728–36. doi: 10.1111/ctr.12373
32. Chang Y, Cho Y, Lee JH, Lee YB, Cho EJ, Yu SJ, et al. Comparison of Models for Tumor Recurrence after Liver Transplantation for the Patients with Hepatocellular Carcinoma: A Multicenter Long-Term Follow-Up Study. Cancers (Basel) (2019) 11(9):1295. doi: 10.3390/cancers11091295
33. Hou YF, Wei YG, Yang JY, Wen TF, Xu MQ, Yan LN, et al. Microvascular invasion patterns affect survival in hepatocellular carcinoma patients after second hepatectomy. J Surg Res (2016) 200(1):82–90. doi: 10.1016/j.jss.2015.06.069
34. Feng LH, Dong H, Lau WY, Yu H, Zhu YY, Zhao Y, et al. Novel microvascular invasion-based prognostic nomograms to predict survival outcomes in patients after R0 resection for hepatocellular carcinoma. J Cancer Res Clin Oncol (2017) 143(2):293–303. doi: 10.1007/s00432-016-2286-1
35. Lim KC, Chow PKH, Allen JC, Chia GSM, Lim M, Cheow PC, et al. Microvascular invasion is a better predictor of tumor recurrence and overall survival following surgical resection for hepatocellular carcinoma compared to the Milan criteria. Ann Surg (2011) 254(1):108–13. doi: 10.1097/SLA.0b013e31821ad884
36. Tai YH, Wu HL, Mandell MS, TSou MY, Chang KY. The association of allogeneic blood transfusion and the recurrence of hepatic cancer after surgical resection. Anaesthesia (2020) 75(4):464–71. doi: 10.1111/anae.14862
37. Xun Y, Tian H, Hu L, Yan P, Yang K, Guo T. The impact of perioperative allogeneic blood transfusion on prognosis of hepatocellular carcinoma after radical hepatectomy: A systematic review and meta-analysis of cohort studies. Medicine (Baltimore) (2018) 97(43):e12911. doi: 10.1097/MD.0000000000012911
38. Katz SJ, Shia J, Liau KH, Gonen M, Ruo L, Jarnagin W, et al. Operative Blood Loss Independently Predicts Recurrence and Survival After Resection of Hepatocellular Carcinoma. Ann Surg (2009) 249(4):617–23. doi: 10.1097/SLA.0b013e31819ed22f
39. Corti A, Franzini M, Paolicchi A, Pompella A. Gamma-glutamyltransferase of cancer cells at the crossroads of tumor progression, drug resistance and drug targeting. Anticancer Res (2010) 30(4):1169–81.
40. Kunutsor SK, Apekey TA, Seddoh D. Gamma glutamyltransferase and metabolic syndrome risk: a systematic review and dose-response meta-analysis. Int J Clin Pract (2015) 69(1):136–44. doi: 10.1111/ijcp.12507
41. Koenig G, Seneff S. Gamma-Glutamyltransferase: A Predictive Biomarker of Cellular Antioxidant Inadequacy and Disease Risk. Dis Markers (2015) 2015:818570. doi: 10.1155/2015/818570
42. Moon DO, Kim BY, Jang JH, Kim MO, Jayasooriya RG, Kang CH, et al. K-RAS transformation in prostate epithelial cell overcomes H2O2-induced apoptosis via upregulation of gamma-glutamyltransferase-2. Toxicol In Vitro (2012) 26(3):429–34. doi: 10.1016/j.tiv.2012.01.013
43. Koh WP, Robien K, Wang R, Govindarajan S, Yuan JM, Yu MC. Smoking as an independent risk factor for hepatocellular carcinoma: the Singapore Chinese Health Study. Br J Cancer (2011) 105(9):1430–5. doi: 10.1038/bjc.2011.360
44. Koh WP, Yuan JM, Sun CL, Lee HP. Middle-aged and older Chinese men and women in Singapore who smoke have less healthy diets and lifestyles than nonsmokers. J Nutr (2005) 135(10):2473–7. doi: 10.1093/jn/135.10.2473
45. Zhang TT, Zhao XQ, Liu Z, Mao ZY, Bai L. Factors affecting the recurrence and survival of hepatocellular carcinoma after hepatectomy: a retrospective study of 601 Chinese patients. Clin Transl Oncol (2016) 18(8):831–40. doi: 10.1007/s12094-015-1446-0
46. Li CL, Lin YK, Chen HA, Huang CY, Huang MT, Chang YJ. Smoking as an Independent Risk Factor for Hepatocellular Carcinoma Due to the α7-Nachr Modulating the JAK2/STAT3 Signaling Axis. J Clin Med (2019) 8(9):1391. doi: 10.3390/jcm8091391
47. Zhang XF, Wei T, Liu XM, Liu C, Lv Y. Impact of cigarette smoking on outcome of hepatocellular carcinoma after surgery in patients with hepatitis B. PloS One (2014) 9(1):e85077. doi: 10.1371/journal.pone.0085077
48. Poon RT, Fan ST, Ng IO, Lo CM, Liu CL, Wong J. Different risk factors and prognosis for early and late intrahepatic recurrence after resection of hepatocellular carcinoma. Cancer (2000) 89(3):500–7. doi: 10.1002/1097-0142(20000801)89:3<500::AID-CNCR4>3.0.CO;2-O
49. Furuta M, Ueno M, Fujimoto A, Hiyami S, Yasukawa S, Kojima F, et al. Whole genome sequencing discriminates hepatocellular carcinoma with intrahepatic metastasis from multi-centric tumors. J Hepatol (2017) 66(2):363–73. doi: 10.1016/j.jhep.2016.09.021
50. Utsunomiya T, Shimada M, Kudo M, Ichida T, Matsui O, Izumi N, et al. A comparison of the surgical outcomes among patients with HBV-positive, HCV-positive, and non-B non-C hepatocellular carcinoma: a nationwide study of 11,950 patients. Ann Surg (2015) 261(3):513–20. doi: 10.1097/SLA.0000000000000821
Keywords: hepatocellular carcinoma, recurrence, machine learning, modeling, prognosis
Citation: Huang Y, Chen H, Zeng Y, Liu Z, Ma H and Liu J (2021) Development and Validation of a Machine Learning Prognostic Model for Hepatocellular Carcinoma Recurrence After Surgical Resection. Front. Oncol. 10:593741. doi: 10.3389/fonc.2020.593741
Received: 13 August 2020; Accepted: 17 December 2020;
Published: 01 February 2021.
Edited by:
Francesco Giovinazzo, Catholic University of the Sacred Heart, ItalyReviewed by:
Alfonso Recordare, Ospedale dell’Angelo, ItalyHan Wu, Eastern Hepatobiliary Surgery Hospital, China
Copyright © 2021 Huang, Chen, Zeng, Liu, Ma and Liu. This is an open-access article distributed under the terms of the Creative Commons Attribution License (CC BY). The use, distribution or reproduction in other forums is permitted, provided the original author(s) and the copyright owner(s) are credited and that the original publication in this journal is cited, in accordance with accepted academic practice. No use, distribution or reproduction is permitted which does not comply with these terms.
*Correspondence: Jingfeng Liu, drjingfeng@126.com
†These authors have contributed equally to this work and share first authorship