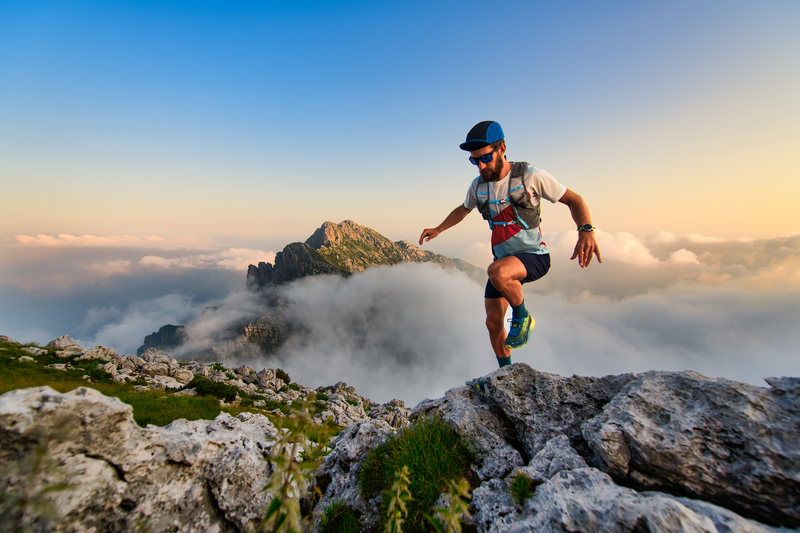
94% of researchers rate our articles as excellent or good
Learn more about the work of our research integrity team to safeguard the quality of each article we publish.
Find out more
ORIGINAL RESEARCH article
Front. Oncol. , 10 November 2020
Sec. Cancer Epidemiology and Prevention
Volume 10 - 2020 | https://doi.org/10.3389/fonc.2020.591110
This article is part of the Research Topic Pathophysiological Interrelationship between Obesity, Metabolic Diseases and Cancer View all 9 articles
Background: The association between obesity and lung cancer (LC) remains poorly understood. However, other indices of obesity on the basis of body shape instead of body size have not been examined yet. The aim of this study was to evaluate the association between different indices of body size and body shape and the risk of LC. In particular, this study examined the association between A Body Shape Index, a more precise indicator of abdominal fat than traditional anthropometric measures, and the risk of LC.
Methods: In the prospective cohort the Rotterdam Study, we analysed data of 9,689 participants. LC diagnoses were based on medical records and anthropometric measurements were assessed at baseline. Cox-regression analyses with corresponding Hazard Ratios were used to examine the association between the anthropometric measurements and the risk of LC with adjustment for potential confounders. Potential non-linear associations were explored with cubic splines using the Likelihood ratio (LR) test.
Results: During follow-up, 319 participants developed LC. Body mass Index (BMI) was inversely associated with the risk of lung cancer (HR 0.94, 95% CI: 0.91–0.97) and persisted after excluding lung cancer cases during the first 10 years of follow-up. There was evidence for a non-linear association between BMI and the risk of lung cancer (0,04, df = 1), which indicated that the inverse association between BMI and lung cancer was mainly present in non-obese participants. Waist circumference (WC) (HR 1.03 95% CI: 1.01–1.05), Waist-to-Hip Ratio (WHR) (HR 1.23 95% CI: 1.09–1.38) and ABSI (A Body Shape Index) (HR 1.17 95% CI: 1.05–1.30) were positively and linearly associated with the risk of lung cancer.
Conclusions: Body shape rather than body size may be an important risk indicator of LC. Future research should focus on the role of visceral fat and the risk of LC as well as the underlying mechanisms.
Lung cancer is currently the main cause of cancer mortality worldwide (1). In 2018, the number of incident lung cancer cases was approximately 2.9 million worldwide, accompanied by 1.75 million cases of death by lung cancer (2, 3). Apart from smoking, emerging evidence shows that obesity may play a role in the development of cancer. Although obesity is associated with 13 types of cancer (4), the association between obesity and lung cancer remains controversial and the underlying mechanisms are poorly understood.
Several indices of nutritional status exist. The most commonly used method is Body Mass Index (BMI) due to its simplicity of measuring and low cost (5). Several meta-analyses have shown an inverse association between BMI and the risk of lung cancer (6–9). A first explanation may come from residual confounding by smoking. Multiple studies that assessed the role of residual confounding by restricting the association between BMI and the risk of lung cancer to never smokers were not able to fully explain the inverse association between BMI and lung cancer (9–13). A second explanation for this inverse association may be due to weight loss as a result of preclinical lung cancer or an impaired lung function e.g. by chronic obstructive lung disease (14). It has been suggested that the association of BMI and lung cancer should increase to a more positive association after excluding lung cancer cases during the first years of the follow-up which may include imminent cases of lung cancer. However, several studies that excluded lung cancer cases in the first years of follow-up have found inconsistent results (9, 15–20).
In contrast to overall obesity and body size, as measured by BMI, specifically abdominal obesity may have a more important role in lung cancer development (9, 21–23). Abdominal obesity as a measure of body shape can be reflected by an increased Waist-to-Hip Ratio (WHR) and Waist circumference (WC), independent of BMI (24, 25). A meta-analysis, including seven prospective cohorts, has showed that WC and WHR were positively associated with the risk of lung cancer (21). However, this meta-analysis consisted of mainly women and measurements were mostly self-reported and/or self-measured. Meanwhile, more recent studies with larger sample sizes have shown more controversial results; a pooled analysis of 12 cohorts has concluded that both WC and WHR were positively associated with the risk of lung cancer (9), whereas another prospective cohort study has found an inverse association for WC and the risk of lung cancer in Chinese participants (23), and a study among 162.679 American adults has found no association between BMI and WC with the risk of lung cancer (22).
A newly developed anthropometric measurement is A Body Shape Index (ABSI), combining WC to BMI and weight into one formula (26). Studies have shown that ABSI is an independent predictor of the fat and fat free mass ratio (27), indicating that ABSI may be a more precise indicator of abdominal fat. Moreover, a meta-analysis has shown that ABSI outperformed BMI and WC in predicting all-cause mortality including cancer (28). However, to the best of our knowledge, the association between ABSI and the risk of lung cancer has not been examined yet.
The objective of this study was to evaluate the different indices of body size (i.e. BMI) and body shape (i.e. WC, WHR, and ABSI) and the risk of lung cancer in a population-based prospective cohort study.
Data for this prospective cohort study was derived from three sub cohorts from the ongoing prospective Rotterdam Study (29). The Rotterdam study started its first cohort in 1990 (RS-I) and has expanded with two additional cohorts in 2000 (RS-II) and 2006 (RS-III), consisting of participants living in the district of Ommoord in the city of Rotterdam. At the start of each cohort and subsequently every 3 to 4 year, all participants were examined in detail. Participants were interviewed at their home (2 h) and afterwards, trained staff exposed these participants to multiple measurements in a special research facility centre (5 h). An overview of the various cohorts with the various follow-up measurements and starting dates were described elsewhere (29). A written Informed consent was signed by all participants. The institutional review board of the Erasmus MC as well as the review board of The Netherlands Ministry of Health Welfare and Sports approved the Rotterdam Study (29).
The population in this study consisted of participants from the first three cohorts that started in 1990 (RS-I), 2000 (RS-II), and in 2006 (RS-III). By 2008, the total number of participants in the Rotterdam Study at baseline consisted of 14,926 participants aged 45 or over. In this study we excluded participants without all investigated anthropometric or lifestyle measurements at baseline from the initial cohort (N= 5,237). Therefore, the number of participants at baseline was equal to 9,689. All participating women were postmenopausal.
The primary outcome of interest was incident lung cancer. Two research physicians independently assessed the diagnosis of cancer based on medical records obtained through general practitioners and hospital discharge letters. Additional information was collected through linkage with the Dutch Hospital Data, National Cancer Registry, and Dutch pathology database (PALGA). Only cases confirmed by pathology were used. Cases of mesothelioma were not included in the definition of cancer for these analyses. Lung cancer was classified according to the International Classification of Diseases tenth edition (C34.2, C34.3). In case of discrepancy, consensus was obtained through consultation with a cancer epidemiologist. Follow-up of cancer registration was completed up to January 1, 2014.
The anthropometric measurements Body Mass Index (BMI), Waist circumference (WC), Waist-to-Hip Ratio (WHR), and A Body Shape Index (ABSI) were measured in the research centre by trained staff during regular on site visits every 3–4 years.
BMI (kg/m2) was calculated by dividing the weight (kg) by the squared height (m2). Height and weight were measured without shoes and heavy outerwear, using calibrated SECA-measurement scales. BMI was categorised as normal, overweight, and obese with the corresponding cut offs <25, 25–30, and >30 kg/m², respectively (24). Since the underweight class (<18.5 kg/m²) contained only five lung cancer cases, we merged this class with the normal weight class to warrant the sample size.
WC (cm) was measured with a flexible measuring tape at the level halfway between the lower rib margin and the iliac crest in standing position, without heavy outerwear, empty pockets, and calm exhaling. According to recommendations of the WHO (World Health Organization), WC was categorised as normal, increased, and substantially increased with the corresponding cut offs <80, 80–88, and >88 cm, respectively, in females and <94, 94–102, and >102 cm, respectively, in males (24).
WHR was defined as the WC (in cm) divided by the Hip Circumference (HC). HC (in cm) was measured as the maximum circumference over the nates. According to recommendations of the WHO, WHR was categorised as normal, increased, and substantially increased with the corresponding cut offs <0.80, 0.80–0.85, and >0.85 respectively, in females and <0.95, 0.95–1.00 and >1.00, respectively, in males (24). We standardised the continuous WHR values into age (continuous) and sex adjusted z-scores, specific to the sample of analysis.
ABSI (A Body Shape Index) was calculated as WC/(BMI2/3×height1/2) (26). To compare low ABSI-values with higher ABSI-values, we divided ABSI into tertiles. As with WHR, we standardised the continuous ABSI values into age (continuous) and sex adjusted z-scores, specific to the sample of analysis.
Covariates of interest were derived from the existing literature and measured at baseline. We considered the following covariates as important due to their relation with the anthropometric measurements and the risk of lung cancer: age; employment; highest educational level; marital status; sex; ever diagnosed for cancer; smoking status; pack years; physical activity; WCRF/AICR (World Cancer research Fund/American Institute for Cancer Research) adherence score; alcohol consumption.
Paid employment (yes/no), educational level (high: higher general education or university/low: primary education or intermediate vocational), marital status, (partner/no partner) and sex (men/women) ever diagnosed for cancer (yes/no) and smoking status (never, ever, current), together with the corresponding cigarettes in pack years (continuously), were self-reported during the home interviews. Physical activity (30, 31) was measured in MET-hours and assessed with an adapted version of the Zutphen Physical Activity questionnaire (32). For the current analyses, we standardised the continuous MET hours per week values into z-scores, specific to the sample of analysis. The WCRF/AICR adherence score contained five recommendations for cancer prevention including: avoiding energy-dense foods, sugar-containing drinks, red and processed meat, and stimulating fruit and vegetables, dietary fiber, and vitamin supplementation. A minimum sum score of 0 points indicated that none of the recommendations was met and a maximum sum score of 5 indicated that all recommendations were fully met. Dietary intake items as well as alcohol consumption (grams of ethanol per day) were measured by using the validated food frequency questionnaire (FFQ) (33).
We used Cox proportional hazards regression to analyse the association between BMI, WC, WHR, and ABSI values with the risk of lung cancer. Both continuous and categorical analyses were performed. For all categorical variables, we used the lowest category as reference category. Results of the Cox-regression analyses were presented as hazard ratios (HRs) with the corresponding 95% confidence intervals (CI). The underlying time axis of the Cox-regression model was the follow-up time, starting at baseline until the first diagnosis of lung cancer, death, or end of the study period, whichever came first. Results of the Cox-regression analysis were presented in a crude model (model 1) which included the association between the main determinant and the outcome variable, adjusted for sex and age, as well as a multivariable model, adjusting the association for all (potential) confounders at baseline (model 2). Furthermore, we used the Wald-test to calculate the overall p-for trend for the categorical variables. We added an interaction term between the covariates and the underlying follow-up time to test for the proportional hazard assumption. In addition to the baseline measurements, we analysed the mean differences between baseline and follow-up of BMI, WC, WHR, and ABSI in relation to the risk of lung cancer to examine whether the association might change over time. Within these associations, the baseline measurement of each anthropometric measurement was added to account for potential regression to the mean (34). These mean differences were calculated by using a paired sample t-test.
We tested for effect modification by sex (men/women), smoking status (never, ever current), and pack years (continuous). We added an interaction term between the main determinant(s) and sex (determinant*sex) in an unadjusted Cox-regression model. Whenever the interaction term was significant, the results were presented categorized by sex, smoking status or stratified by the median of pack years.
In this study, we performed two sensitivity analyses. First, we systemically excluded lung cancer cases within the first 5, 7.5, and 10 years of follow-up to examine whether reverse causation could influence the association between BMI at baseline and the risk of lung cancer. Second, to assess the role of residual confounding by smoking, the association between BMI and the risk of lung cancer was stratified for never, ever and, current smokers.
The possibility of non-linear associations between the anthropometric measurements BMI, WC, WHR and, ABSI with the risk of lung cancer were assessed by using data driven natural cubic splines as suggested by Durrleman et al. (34). Degrees of freedom were determined on the basis of the lowest Akaike’s information criterion (AIC) value. Likelihood ratio tests (LRTs) were performed to ascertain whether a non-linear model suited the data better than a linear model. Whenever the LRTs showed a significant result, the effect estimates were separated for the different intervals. The cut-offs of the interval were then based on the knots in the cubic spline.
All missing values, except for the outcome variable, were imputed by using the Markov chain Monte Carlo (MCMC) method. After the imputation procedure, the resulting ten datasets were pooled and an average of the imputed data was calculated. The pooled data was used to conduct all analyses in this study. In this study, p-values less than 0.05 (two-sided) were considered as statistically significant. For the non-linear Likelihood-ratio (LR) test we used R-statistics version 4.4.3. In all further statistical analyses we used Statistical Product and Service Solutions (SPSS) version 24.0.
Table 1 shows the main baseline characteristics of the study population (n= 9,689). Within a mean follow-up of 13.2 years, 319 participants developed lung cancer. Most participants developed lung cancer in cohort 1 (88.7%) within a mean follow-up of 16.0 years. According to the guidelines of the WHO, most of the participants showed a substantially increased WC in all cohorts. Similarly, this trend was also observed for WHR, except for RS-III. Participants in RS-III showed the lowest age, WHR, ABSI, but the highest BMI on average.
As shown in Table 2, an 1 kg/m2 increase of BMI was associated with a reduced risk of lung cancer, after adjustment for sex and age (HR 0.95, 95% CI: 0.92–0.98) and after full adjustment for sex, age, cohort, physical activity, alcohol intake, pack-years, education, WCRF adherence score, and diabetes mellitus (HR 0.94, 95% CI: 0.91–0.97). This corresponding inverse trend was also observed for the normal BMI group (reference group, BMI<25) compared with the high and obese group, after adjustment for sex and age (High: HR 0.76 95% CI: 0.60–0.96) (Obese: HR 0.68 95% CI: 0.47–0.99) and in the fully adjusted model (High: HR 0.72 95% CI: 0.56–0.91) (Obese: HR 0.61 95% CI: 0.41–0.89). Furthermore, 1 cm of WC (HR 1.03 95% CI: 1.01–1.06) and one standard deviation increase of WHR (HR 1.23 95% CI: 1.09–1.38) and ABSI (HR 1.17 95% CI: 1.05–1.30) were positively associated with a 3%, 23%, and 17% higher risk of lung cancer respectively, after full adjustment for confounders. Similar results were observed when WHR (HR 1.66 95% CI: 1.21–2.26, P-for trend = 0.06) and ABSI (HR 1.50 95% CI: 1.05–2.12, P-for trend = <0.001) were analyzed categorically.
Table 2 Hazard ratio’s (95% CI) for the association between BMI (kg/m2), WC (cm), WHR, and ABSI with the risk of lung cancer.
Table 3 shows that the inverse association between BMI and the risk of lung cancer persisted after excluding lung cancer cases in the first 5, 7.5, and 10 years of follow-up, before and after full adjustment for sex, age, cohort, physical activity, alcohol intake, pack-years, education, WCRF adherence score, and diabetes mellitus. Moreover, in both models, the inverse association remained stable as the number of excluded follow-up years increased. Table 4 shows the associations between BMI and the risk of lung cancer, stratified for never, ever, and current smokers. Before and after full adjustment for confounders, BMI was not associated with the risk of lung cancer in never, ever, and current smokers.
Table 3 Hazard ratio’s (95% CI) for BMI (kg/m2) with the risk of lung cancer after excluding lung cancer cases during the first 5, 7.5, and 10 years of follow-up after baseline measurement.
Table 4 Hazard ratio’s (95% CI) for BMI (kg/m2) and the risk of lung cancer in never, ever and current smokers.
Within a mean follow-up of 11.6 years from baseline until the next follow up measurement, in which 173 lung cancer cases were identified, the mean BMI (-0.58 kg/m2, 95% CI: -0.62 – -0.53), WC (-2.79 cm, 95% CI: -3.00 – -2.58), and WHR (-0.016 95% CI: -0.019 – -0.014) decreased, whereas the ABSI mean (0.0350 95% CI: 0.0348 – 0.0352) increased. Table 5 provides the hazard ratios for the associations between the mean differences of BMI, WC, WHR, and ABSI at baseline and follow-up with the risk of lung cancer. None of the associations between the mean differences at baseline and follow-up for BMI, WC, WHR, and ABSI with the risk of lung cancer were significant.
Table 5 Hazard ratio’s (95% CI) for the mean differences of BMI (kg/m2), WC (cm), WHR, and ABSI between baseline and follow-up with the risk of lung cancer.
In all Cox regression models, there was no significant interaction between follow-up time and BMI, WC, WHR, and ABSI. Therefore, the proportional hazard assumption was met for all associations. No significant interactions were observed between BMI, WC, WHR, and ABSI with smoking status and sex (data not shown). Furthermore, no significant interactions were found for BMI, WC, WHR, and ABSI with pack years of smoking (data not shown). Figure 1 shows the relation between 1-unit increase of BMI, WC, WHR, and ABSI with the risk of lung cancer for 9689 participants, including 319 lung cancer cases. None of the Likelihood-ratio (LR) tests between WC (p = 0.99, df =1), WHR (p = 0.09, df =1) or ABSI (p = 0.46, df =1) and the risk of lung cancer suggested a non-linear relationship. In contrast, the LR test between BMI (p = 0.04, df =1) and the risk of lung cancer did suggest a non-linear relationship. More specifically, the inverse association between BMI and lung cancer risk was only linear in non-obese participants whereas the curve flattened in obese participants.
Figure 1 Natural cubic splines in a standard cox-regression model with knots at 22, 27, 34 and 72, 92, 112, and -1.5, 0, 1.5 and -1.5, 0, 1.5 for BMI, WC, WHR, and, ABSI respectively. The blue line represents the hazard ratios for the risk of lung cancer with the corresponding 95% confidence interval. Hazard ratios were adjusted for sex, age, cohort (RS-I/RS-II/RS-III), physical activity (continuous), alcohol intake (continuous), pack-years (continuous), education (high/low), WCRF adherence score (continuous), and diabetes mellitus (yes/no). WC and WHR were additionally adjusted for BMI.
In this prospective cohort study, we found that BMI was inversely associated with the risk of lung cancer. In contrast to BMI, an increased WC, WHR, and ABSI were associated with a higher risk of lung cancer. Additionally, we found evidence regarding a non-linear association between BMI and the risk of lung cancer, which indicated that the inverse association between BMI and lung cancer risk was only present in non-obese participants, whereas an increase in WC, WHR, and, ABSI showed a linearly higher risk of lung cancer.
Our results showed that a higher BMI was associated with a reduced risk of lung cancer which is in line with two meta-analyses (36, 37) and two pooled analyses (9, 38). Although these results seem to be consistent, this inverse association is in conflict with the hypothesis that suggests that obesity is a risk factor for lung cancer (39, 40). Residual confounding by smoking and reverse causation are proposed explanations for this inverse association (41, 42). Interestingly we found that the inverse association persisted after excluding lung cancer cases during the first 5, 7.5, and 10 years of follow-up. Our findings are in agreement with several other studies that attempted to address the role of reverse causation by excluding the lung cancer cases during the first years of follow-up. However, two other studies suggested that the strength of association between BMI and the risk of lung cancer diluted after excluding lung cancer cases during the first years of follow-up, indicating that reverse causation might play a role (10, 11). However, these two studies included mainly women, which can explain the different results because survival and clinical presentation for lung cancer differs among men and women (43). We also assessed the association between change in BMI and the risk of lung cancer between baseline and follow-up. Nonetheless, we did not find a significant association between a difference in BMI and the risk of lung cancer over time, which contradicts the role of reverse causation. Additionally, we assessed whether the relationship between BMI and the risk of lung cancer had a non-linear shape. We found evidence for a non-linear association between BMI and the risk of lung cancer suggesting that the inverse association only applies for participants without obesity. Previous studies showed that obesity is unbeneficial for developing lung cancer (20, 38), which could flattened the BMI-lung cancer curve for this group in our population.
An explanation for the inverse association between BMI and the risk of lung cancer in those without obesity may come from residual confounding by smoking. In the current study we found no association between BMI and the risk of lung cancer among never, ever, or current smokers. Several other studies which stratified the association for smoking found similar results (15–19). However, similar to our study, these studies had a relatively small number of lung cancer cases because lung cancer is quite rare in subjects without a history of smoking. Studies with larger sample sizes showed contrary results: a recent meta-analysis of 19 studies including 15 million never smokers and a pooled analysis of 12 studies containing almost 700,000 never smokers, showed that the inverse association also persisted in never smokers (9, 20). Thus, although the results in this study cannot fully rule out the presence of residual confounding by smoking, on the basis of our results combined with previous meta-analyses, smoking may not fully explain the inverse association between BMI and the risk of lung cancer.
An alternative biological explanation for the inverse association between BMI and the risk of lung cancer can be the storage of common environmental genotoxicants in fat tissue. Lipophilic genotoxicants such as polycyclic aromatic hydrocarbons (PAHs) that are derived from smoking or occupational exposure influence the development of lung cancer through DNA damage (44). Previous work has suggested that an individual’s BMI or fat tissue influences the metabolism of PAHs, in which lower levels of PAH-DNA adducts are found in the circulation of obese individuals (45). This may imply that individuals with lower fat tissue may have a higher exposure to these lipophilic carcinogens.
Our study found that WC and WHR were positively associated with the risk of lung cancer, consistent with other studies (9, 21). However, other studies that investigated the association between WC and the risk of lung cancer found more controversial results. A prospective study among Chinese participants found an inverse association between WC and the risk of lung cancer (23). Unlike our study, this study did not correct the analyses for BMI, which can explain the contradictory results. Another prospective cohort study found no association between WC and the risk of lung cancer (22). Although WHR and WC are both measurements of abdominal obesity, the association with the risk of lung cancer seems only consistent for WHR studies (9, 21). These dissimilar results could be explained by the fact that WHR has a stronger correlation with visceral fat when compared to WC (46). Increased visceral fat is positively associated with the risk of cancer (47), as visceral fat promotes inflammation and insulin resistance and may therefore create a pro-tumorigenic environment (48).
The findings is this study showed that ABSI was positively associated with the risk of lung cancer. Although this is the first study that examined the association between ABSI and the risk of lung cancer, another study among 200 adults with overweight found an inverse association between ABSI and FFMI (Fat Free Mass Index) (27), indicating that a decreased ABSI is associated with a higher FFMI when compared to higher ABSI values. A low FFMI may be an indicator for a higher risk of lung cancer as progressive loss of muscle mass is the main characteristic of lung cancer cachexia (49). However, since we focussed on incident lung cancer and did not find indications of reverse causality, our results on ABSI may not be explained by lung cancer cachexia. Another study among elderly found that in men, ABSI was positively associated with FM (Fat Mass) and thereby negatively associated with FFM (Fat Free Mass) (50). This study suggests that the positive association between ABSI and the risk of lung cancer may be dedicated to an increased FM, and may therefore support the hypothesis of body fatness and in particular abdominal obesity as a risk factor for lung cancer (50). Moreover, ABSI also shows a positive correlation with visceral fat (51). As mentioned earlier, increased visceral fat is associated with cancer progression (47) and may create a pro-tumorigenic environment (48). Therefore, more research is needed to identify the specific roles of visceral adiposity and FM and FFM in the relation to incident lung cancer as the VAT/SAT (Visceral and Subcutaneous Adipose Tissue) ratio is an independent predictor for non-small-cell lung cancer (52).
Although we found associations between baseline BMI, WC, WHR, and ABSI with the risk of lung cancer, we found no association for the mean differences between baseline and follow-up of BMI, WC, WHR, and ABSI with the risk of lung cancer. Another prospective cohort study among 8000 Dutch participants found that a moderate short-term annual decrease (-0.10 – -0.50 kg/m2/year) of BMI, was associated with a reduced risk of lung cancer in women (53). However, due to a lack of available data, this study could not fully correct the association between BMI and the risk of lung cancer for physical activity and alcohol consumption, both important confounders for the association between obesity and the risk of lung cancer. The non-significant results in this study can be explained in multiple ways. First, it should be mentioned that within the current study, anthropometric measures become less reliable during ageing due to shrinking and changes in body composition (i.e. loss of fat free mass) (54) and could have influenced the non-significant results because lung cancer has a long latency period. Secondly, as people become older, it is more likely that low body mass is reflected by a low muscle mass instead of a low fat mass (55, 56). This implies that BMI, WC, WHR, and ABSI may give an underestimation of the (abdominal) fat mass in a person. Consequently, these anthropometric measurements of body size and body shape become less valid. Therefore, future studies including larger sample sizes, longer years of follow-up, data on changes of covariates over time, and measurements that can distinguish fat and muscle mass, are needed to elucidate the role of body size and body shape over time in an appropriate manner.
An important strength of this study is that all anthropometric measurements are performed by trained staff in a special designed research centre according to a consistent protocol. Therefore, the chance of information bias in this study is minimal when compared to other studies (57). A second strength is the follow-up of 25 years. The Rotterdam Study has one of the longest follow-up in years when compared to other studies, which allowed us to assess the role of reverse causation over a longer time period.
This study has also some limitations. First, the number of incident lung cancer cases in this study was relatively low. As a result we could not perform extensive subgroup analysis since the association between obesity and lung cancer may differ for tumour histological type, ethnic origin, and sex (9). For example, non-smokers develop more often adenocarcinomas and different risk factors (e.g. genetic as well as environmental) can play a role (58). Furthermore, we were unable to conduct sensitivity analyses on the basis of COPD at baseline because of the small number of cases in our cohort. Despite the fact that only 19 lung cancer cases had COPD, the results preclude any conclusions regarding specific groups that may be more or less vulnerable to the consequences of (abdominal) obesity on lung cancer. Due to a lack of detailed data, we were also unable to correct our analysis for metabolic dysfunctions at baseline with the exception of diagnosis of type 2 diabetes. As metabolic dysfunctions are associated with central adiposity as well as lung cancer risk (59), the influence of early stage metabolic disturbances cannot be ruled out. Further, we did not have detailed measures on FFM specifically (eg. By DXA) to confirm that the association between ABSI and lung cancer is explained by sarcopenia, which has been found to be a predictor for lung cancer prognosis (60) as well as metabolic dysfunction (61). A second limitation was that the covariates in this study were only measured at baseline. The analysis of the mean differences between baseline and follow-up was therefore only adjusted for baseline covariables. Important covariates such as smoking and physical activity may have changed during follow-up and could have influenced our results. Additionally, these important covariables were self-reported, which usually leads to an overestimation of physical activity (61) and an underestimation of alcohol consumption (62). A third limitation is that the results in this study were mainly based on a population of traditionally Dutch origin. A pooled analysis found that the association between BMI and lung cancer was stronger among blacks when compared to whites (9). Hence, we may have underestimated the effect in our study when compared to studies which contained more diverse ethnic origins.
In conclusion, we found an inverse association between BMI and the risk of lung cancer. In contrast, WC, WHR and ABSI are positively associated. We found evidence that reverse causation may serve as an explanation for the inverse association between BMI and lung cancer. Future research with larger sample sizes should focus on the role of visceral fat and the distinction between fat mass from fat free mass, using more advanced measuring tools to get a better understanding of the underlying biological mechanisms.
The datasets presented in this article are not readily available because sharing of individual participant data was not included in the informed consent of the study, and there is a potential risk of revealing participants' identities. Requests to access the datasets should be directed to data manager Frank J.A. van Rooij (bG4uY21zdW1zYXJlQGppb29ybmF2LmY=).
The studies involving human participants were reviewed and approved by Medical Ethics Committee of the Erasmus MC. The patients/participants provided their written informed consent to participate in this study.
FA: writing of the manuscript, data analyses, revised the comments of the co-authors. RR: data acquisition, reviewing manuscript. MM: data acquisition, reviewing manuscript. LL: data acquisition, reviewing manuscript. BS: participated in setting up the study design, data acquisition, reviewing manuscript. JK-J: participated in setting up the study design, data acquisition, supervision of first author, critically reviewing the manuscript. All authors contributed to the article and approved the submitted version.
The Rotterdam Study is supported by Erasmus University Medical Center and Erasmus University, Rotterdam, the NWO; the Netherlands Organization for Health Research and Development; the Research Institute for Diseases in the Elderly; the Netherlands Genomics Initiative; the Ministry of Education, Culture and Science; the Ministry of Health, Welfare and Sports; the European Commission (DG XII); and the Municipality of Rotterdam. The funders played no role in the study design or in data collection and analysis. The authors had final responsibility for design and conduct of the study, collection, management, analysis, and interpretation of the data, and preparation, review or approval of the manuscript.
The authors declare that the research was conducted in the absence of any commercial or financial relationships that could be construed as a potential conflict of interest.
We gratefully acknowledge the contribution of the participants of the Rotterdam Study, research assistants, the general practitioners, hospitals and pharmacies in Rotterdam. Furthermore we also want to thank Dr. M. M. Goedendorp for her useful comments during the whole process of writing the manuscript.
1. Siegel R, Miller K, Ahmedin J. Cancer statistics, 2016. CA Cancer J Clin (2016) 66(1):7–30. doi: 10.3322/caac.21332
2. Bray F, Ferlay J, Soerjomataram I, Siegel R, Torre L, Jemal A. Global cancer statistics 2018: GLOBOCAN estimates of incidence and mortality worldwide for 36 cancers in 185 countries. CA Cancer J Clin (2018) 68(6):394–424. doi: 10.3322/caac.21492
3. Siegel RL, Miller KD, Jemal A. Cancer statistics, 2019. CA Cancer J Clin (2019) 69(1):7–34. doi: 10.3322/caac.21551
4. Bharkaran K, Douglas I, Forbes H, dos-Santos-Silva I, Leon D, Smeeth L. Body-mass index and risk of 22 specific cancers: a population-based cohort study of 5·24 million UK adults. Lancet (2014) 384(9945):755–65. doi: 10.1016/S0140-6736(14)60892-8
5. Huxley R, Mendis S, Zheleznyakov E, Reddy S, Chan J. Body mass index, waist circumference and waist:hip ratio as predictors of cardiovascular risk–a review of the literature. Eur J Clin Nutr (2010) 64(1):16–22. doi: 10.1038/ejcn.2009.68
6. Gupta A, Majumber K, Arora N, Mayo H, Singh P, Beg M, et al. Premorbid body mass index and mortality in patients with lung cancer: A systematic review and meta-analysis. Lung Cancer (2016) 102:49–59. doi: 10.1016/j.lungcan.2016.10.017
7. Shen N, Ping F, Cui B, Bu C, Bi J. Associations between body mass index and the risk of mortality from lung cancer. Medicine (Baltimore) (2017). 96(34):e7721. doi: 10.1097/MD.0000000000007721
8. Wang J, Xu H, Zhou S, Wang D, Zhu L, Hou J, et al. Body mass index and mortality in lung cancer patients: a systematic review and meta-analysis. Eur J Clin Nutr (2018) 72(1):4–17. doi: 10.1038/ejcn.2017.70
9. Yu D, Zheng W, Johansson M, lan Q, Park Y, White E, et al. Overall and Central Obesity and Risk of Lung Cancer: A Pooled Analysis. J Natl Cancer Institute (2018) p:10. doi: 10.1093/jnci/djx286
10. Kabat GC, Kim M, Hunt J, Chlebowski R, Rohan T. Body Mass Index and Waist Circumference in Relation to Lung Cancer Risk in the Women’s Health Initiative. Am J Epidemiol (2008) 168(2):158–69. doi: 10.1093/aje/kwn109
11. Utami Dewi N, Boshuizen H, Johansson M, Vineis P, Kampman E, Steffen A, et al. Anthropometry and the Risk of Lung Cancer in EPIC. Am J Epidemiol (2016) 184(2):129–39. doi: 10.1093/aje/kwv298
12. Olson JE, Yang P, Schmitz K, Vierkant R, Cerhan J, Sellers T. Differential association of body mass index and fat distribution with three major histologic types of lung cancer: evidence from a cohort of older women. Am J Epidemiol (2002) 156(7):606–15. doi: 10.1093/aje/kwf084
13. Samanic C, Chow W, Gridley G, Jarvholm B, Fraumeni J. Relation of body mass index to cancer risk in 362,552 Swedish men. Cancer Causes Control (2006) 17(7):901–9. doi: 10.1007/s10552-006-0023-9
14. Flegal KM, Graubard B, Williamson D, Cooper R. Reverse causation and illness-related weight loss in observational studies of body weight and mortality. Am J Epidemiol (2011) 173(1):1–9. doi: 10.1093/aje/kwq341
15. Renehan AG, Tyson M, Egger M. Body-mass index and incidence of cancer: A systematic review and meta-analysis of prospective observational studies. Lancet (2008) 371(9612):569–78. doi: 10.1016/S0140-6736(08)60269-X
16. Bhaskaran K, Douglas I, Forbes H. Body-mass index and risk of 22 specific cancers: A population-based cohort study of 5.24 million UK adults. Lancet (2014) 384(9945):755–65. doi: 10.1016/S0140-6736(14)60892-8
17. Smith L, Brinton LA, Spitz MR. 2012, Body mass index and risk of lung cancer among never, former, and current smokers. J Natl Cancer Inst (2012) 104(10):778–89. doi: 10.1093/jnci/djs179
18. Lam TK, Moore SC, Brinton LA, Smith L, Hollenbeck AR, Gierach GL, et al. Anthropometric measures and physical activity and the risk of lung cancer in never smokers: A prospective cohort study. PloS One (2013) 8(8):e70672. doi: 10.1371/journal.pone.0070672
19. Koh WP, Yuan J-M, Wang R, Lee H-P, Yu MC. Body mass index and smoking-related lung cancer risk in the Singapore Chinese Health Study. Br J Cancer (2010) 102(3):610–4. doi: 10.1038/sj.bjc.6605496
20. Zhu H, Zhang S. Body mass index and lung cancer risk in never smokers: a meta-analysis. BMC Cancer (2018) 18:635. doi: 10.1186/s12885-018-4543-y
21. Hidayat K, Du X, Chen G, Shi M, Shi B. Abdominal Obesity and Lung Cancer Risk: Systematic Review and Meta-Analysis of Prospective Studies. Nutrients (2016) 8(12):810. doi: 10.3390/nu8120810
22. Patel AV, Carter B, Stevens V, Gaudet M, Campell P, Gapstur S. The relationship between physical activity, obesity, and lung cancer risk by smoking status in a large prospective cohort of US adults. Cancer Causes Control (2017) 28(12):1357–68. doi: 10.1007/s10552-017-0949-0
23. Xie S, Wang G, Guo L, Chen S, Su K, Li F, et al. Relation between waist circumference and risk of male lung cancer incidence: a prospective cohort study. Zhonghua Liu Xing Bing Xue Za Zhi (2017) 38(2):137–41. doi: 10.3760/cma.j.issn.0254-6450.2017.02.001
25. Molarius A, Seidell JC. Selection of anthropometric indicators for classification of abdominal fatness–a critical review. Int J Obes Relat Metab Disord (1998) 22(8):719–27. doi: 10.1038/sj.ijo.0800660
26. Krakauer NY, Krakauer JC. A new body shape index predicts mortality hazard independently of body mass index. PloS One (2012) p:7 (7). doi: 10.1371/journal.pone.0039504
27. Gomez-Peralta F, Abreu C, Cruz-Bravo M, Alcarria E, Gutierrez-Buey G, Krakauer N, et al. Relationship between “a body shape index (ABSI)” and body composition in obese patients with type 2 diabetes. Diabetol Metab Syndr (2018) 10:21. doi: 10.1186/s13098-018-0323-8
28. Ji M, Zhang S, An R. Effectiveness of A Body Shape Index (ABSI) in predicting chronic diseases and mortality: a systematic review and meta-analysis. Obes Rev (2018) 19(5):737–59. doi: 10.1111/obr.12666
29. Arfan Ikram M, Brussele G, Darwish Murad S, van Duijn C, Franco O, Goedgebure A, et al. The Rotterdam Study: 2018 update on objectives, design and main results. Eur J Epidemiol (2017) 32(9):807–50. doi: 10.1007/s10654-017-0321-4
30. Zhong S, Ma T, Chen L, Chen W, Lv M, Zhang X, et al. Physical Activity and Risk of Lung Cancer: A Meta-analysis. Clin J Sport Med (2016) 26(3):173–81. doi: 10.1097/JSM.0000000000000219
31. Swift DL, Johannsen N, Lavie C, Earnest C, Church T. The Role of Exercise and Physical Activity in Weight Loss and Maintenance. Prog Cardiovasc Dis (2014) 56(4):441–7. doi: 10.1016/j.pcad.2013.09.012
32. Caspersen CJ, Bloemberg B, Saris W, Merrit R, Kromhout D. The prevalence of selected physical activities and their relation with coronary heart disease risk factors in elderly men: the Zutphen Study, 1985. Am J Epidemiol (1991) 133(11):1078–92. doi: 10.1093/oxfordjournals.aje.a115821
33. Klipstein-Grobusch K, den Breeijen J, Goldbohm R, Geleijnse J, Hofman A, Grobbee D, et al. Dietary assessment in the elderly: validation of a semiquantitative food frequency questionnaire. Eur J Clin Nutr (1998) 52(8):588–96. doi: 10.1038/sj.ejcn.1600611
34. Durrleman S, Simon R. Flexible regression models with cubic splines. Stat Med (1989) 8(5):551–61. doi: 10.1002/sim.4780080504
35. Barnett AG, van der Pols JC, Dobson AJ. Regression to the mean: What it is and how to deal with it. Int J Epidemiol (2005) 34(1):215–20. doi: 10.3760/cma.j.issn.0254-6450.2017.02.001
36. Duan P, Chunhui H, Quan C, Yi X, Zhou W, Yuan M, et al. Body mass index and risk of lung cancer: Systematic review and dose-response meta-analysis. Sci Rep (2015) p. 5:16938. doi: 10.1038/srep16938
37. Yang Y, Dong Y, Sun K, Zhao L, Wang L, Jiao Y. Obesity and incidence of lung cancer: a meta-analysis. Int J Cancer (2013) 132(5):1162–9. doi: 10.1002/ijc.27719
38. Sanikini H, Yuan J, Butler L, Koh W, Gao Y, Steffen A, et al. Body mass index and lung cancer risk: a pooled analysis based on nested case-control studies from four cohort studies. BMC Cancer (2018) 18(1):220. p. 23. doi: 10.1186/s12885-018-4124-0
39. Park J, Thomas S, Min K, Deborah J, Philipp E. Obesity and cancer—mechanisms underlying tumour progression and recurrence. Nat Rev Endocrinol (2014) 10(8):455–65. doi: 10.1038/nrendo.2014.94
40. Renehan AG, Zwahlen M, Egger M. Adiposity and cancer risk: new mechanistic insights from epidemiology. Nat Rev Cancer (2015) 15(8):484–98. doi: 10.1038/nrc3967
41. Lung Cancer Alliance. Lung Cancer Alliance. (2017). Available at: https://lungcanceralliance.org; https://lungcanceralliance.org/wp-content/uploads/2017/09/Cachexia_Brochure_2017.pdf.
42. Mineur Y, Abizaid A, Rao Y, Salas R, DiLeone R, Gundisch D, et al. Nicotine decreases food intake through activation of POMC neurons. Science (2011) 332(6035):1330–2. doi: 10.1126/science.1201889
43. Kelsey CR, Fornili M, Ambrogi F, Higgins K, Boyd J, Biganzoli E, et al. Metastasis dynamics for non-small-cell lung cancer: effect of patient and tumor-related factors. Clin Lung Cancer (2013) 14(4):425–32. doi: 10.1016/j.cllc.2013.01.002
44. Kim KH, Jahan S, Kabir E, Brown R. A review of airborne polycyclic aromatic hydrocarbons (PAHs) and their human health effects. Environ Int (2013) 60:71–80. doi: 10.1016/j.envint.2013.07.019
45. Li X, Bai Y, Wang S, Nyamathira S, Zhang X, Zhang W, et al. Association of body mass index with chromosome damage levels and lung cancer risk among males. Sci Rep (2015) 5:9458. doi: 10.1038/srep09458
46. Gadekar M, Dudeja C, Basu L, Vashisht M, Mukherji C. Correlation of visceral body fat with waist–hip ratio, waist circumference and body mass index in healthy adults: A cross sectional study. Med J Armed Forces India (2020) 76(1):41–6. doi: 10.1016/j.mjafi.2017.12.001
47. Murphy R, Bureyko T, Milijkovic I, Cauley J, Satterfield S, Hue T, et al. Association of total adiposity and computed tomographic measures of regional adiposity with incident cancer risk: a prospective population-based study of older adults. Appl Physiol Nutr Metab (2014) 39(6):687–92. doi: 10.1139/apnm-2013-0360
48. Doyle S, Donohoe C, Lysaght J, Reynolds J. Visceral obesity, metabolic syndrome, insulin resistance and cancer. Proc Nutr Soc (2012) 71(1):181–9. doi: 10.1017/S002966511100320X
49. Blauwhoff-Buskermolen S, Langius J, Becker A, Verheul H, de van der Schueren M. The influence of different muscle mass measurements on the diagnosis of cancer cachexia. J Cachexia Sarcopenia Muscle (2017) 8(4):615–22. doi: 10.1002/jcsm.12200
50. Dhana K, Koolhaas C, Schoufour J, Rivadeneira F, Hofman A, Kavousi M, et al. Association of anthropometric measures with fat and fat-free mass in the elderly: The Rotterdam study. Maturitas (2016) 88:96–100. doi: 10.1016/j.maturitas.2016.03.018
51. Bouchi R, Asakawa M, Ohara N, Nakano Y, Takeuchi T, Murakami M. Indirect measure of visceral adiposity ‘A Body Shape Index’ (ABSI) is associated with arterial stiffness in patients with type 2 diabetes. BMJ Open Diabetes Res Care (2016) 4(1):e000188. doi: 10.1136/bmjdrc-2015-000188
52. Blanc-Durand P, Campedel L, Mule S, Jegou S, Luciani A, Pigneur F, et al. Prognostic value of anthropometric measures extracted from whole-body CT using deep learning in patients with non-small-cell lung cancer. Eur Radiol (2020) 17:10. doi: 10.1007/s00330-019-06630-w
53. Taghizadeh N, Boezen H, Schouten J, Schroder C, de Vries E, Vonk J. BMI and Lifetime Changes in BMI and Cancer Mortality Risk. PloS One (2015) 10(4):e0125261. doi: 10.1371/journal.pone.0125261
54. Price G, Uauy R, Breeze E, Bulpitt C, Fletcher A. Weight, Shape, and Mortality Risk in Older Persons: Elevated Waist-Hip Ratio, Not High Body Mass Index, Is Associated With a Greater Risk of Death. Am J Clin Nutr (2006) 84(2):449–60. doi: 10.1093/ajcn/84.2.449
55. O’Neill D. Measuring obesity in the absence of a gold standard. Econ Hum Biol (2015) 17:116–28. doi: 10.1016/j.ehb.2015.02.002
56. Yang L, Zhao M, Xi B. Is BMI accurate to reflect true adiposity? Int J Cardiol (2016) 220:883. doi: 10.1016/j.ijcard.2016.06.310
57. Flegal K, Graubard B, Williamson D, Cooper R. Association of all-cause mortality with overweight and obesity using standard body mass index categories: a systematic review and meta-analysis. JAMA (2013) 309(1):71–82. doi: 10.1001/jama.2012.113905
58. Xufan L, Jia L, Pin W, Liyuan Z, Bingjian L, Kejing Y, et al. Smoker and Non-Smoker Lung Adenocarcinoma Is Characterized by Distinct Tumor Immune Microenvironments. Oncoimmunology (2018) 7(10):e1494677. p. 30. doi: 10.1080/2162402X.2018.1494677
59. Sin S, Lee C-H, Choi S, Han K-D, Lee J. Metabolic Syndrome and Risk of Lung Cancer: An Analysis of Korean National Health Insurance Corporation Database. J Clin Endocrinol Metab (2020) 105(11):dgaa596. doi: 10.1210/clinem/dgaa596
60. Yang M, Shen Y, Tan L, Li W. Prognostic Value of Sarcopenia in Lung Cancer: A Systematic Review and Meta-analysis. Chest (2019) 156(1):101–11. doi: 10.1016/j.chest.2019.04.115
61. Schaller A, Rudolf K, Dejonghe L, Grieben C, Froboese I. Influencing Factors on the Overestimation of Self-Reported Physical Activity: A Cross-Sectional Analysis of Low Back Pain Patients and Healthy Controls. BioMed Res Int (2016) 2016:1497213. doi: 10.1155/2016/1497213
Keywords: waist-to-hip ratio (WHR), waist circumference (WC), body mass index (BMI), A Body Shape Index (ABSI), lung cancer risk, obesity
Citation: Ardesch FH, Ruiter R, Mulder M, Lahousse L, Stricker BHC and Kiefte-de Jong JC (2020) The Obesity Paradox in Lung Cancer: Associations With Body Size Versus Body Shape. Front. Oncol. 10:591110. doi: 10.3389/fonc.2020.591110
Received: 03 August 2020; Accepted: 13 October 2020;
Published: 10 November 2020.
Edited by:
Manuel D. Gahete, Maimonides Biomedical Research Institute of Cordoba (IMIBIC), SpainReviewed by:
Kristy A. Brown, Cornell University, United StatesCopyright © 2020 Ardesch, Ruiter, Mulder, Lahousse, Stricker and Kiefte-de Jong. This is an open-access article distributed under the terms of the Creative Commons Attribution License (CC BY). The use, distribution or reproduction in other forums is permitted, provided the original author(s) and the copyright owner(s) are credited and that the original publication in this journal is cited, in accordance with accepted academic practice. No use, distribution or reproduction is permitted which does not comply with these terms.
*Correspondence: J. C. Kiefte-de Jong, Si5DLktpZWZ0ZUBsdW1jLm5s
Disclaimer: All claims expressed in this article are solely those of the authors and do not necessarily represent those of their affiliated organizations, or those of the publisher, the editors and the reviewers. Any product that may be evaluated in this article or claim that may be made by its manufacturer is not guaranteed or endorsed by the publisher.
Research integrity at Frontiers
Learn more about the work of our research integrity team to safeguard the quality of each article we publish.