- 1Hwa Mei Hospital, University of Chinese Academy of Science, Ningbo, China
- 2Zhejiang Key Laboratory of Pathophysiology, Department of Preventative Medicine, Medical School of Ningbo University, Ningbo, China
- 3Ningbo Institute of Life and Health Industry, University of Chinese Academy of Sciences, Ningbo, China
The CRISPR/Cas system has stood in the center of attention in the last few years as a revolutionary gene editing tool with a wide application to investigate gene functions. However, the labor-intensive workflow requires a sophisticated pre-experimental and post-experimental analysis, thus becoming one of the hindrances for the further popularization of practical applications. Recently, the increasing emergence and advancement of the in silico methods play a formidable role to support and boost experimental work. However, various tools based on distinctive design principles and frameworks harbor unique characteristics that are likely to confuse users about how to choose the most appropriate one for their purpose. In this review, we will present a comprehensive overview and comparisons on the in silico methods from the aspects of CRISPR/Cas system identification, guide RNA design, and post-experimental assistance. Furthermore, we establish the hypotheses in light of the new trends around the technical optimization and hope to provide significant clues for future tools development.
Introduction
The mysterious veil of the genome and transcriptome in diverse organisms is being uncovered owing to contributive sequencing efforts. Even so, the functions of most genes remain unknown (1). The toughest challenge has been to associate phenotype changes to alterations on genetic layers. The state-of-the-art CRISPR/Cas system for genetic manipulation is an emerging tool to solve this nerve-wracking problem (2). CRISPR/Cas system is developed from a prokaryotic adaptive immune defense mechanism against the exogenous nucleic acids in archaea and bacteria (3), which follows a base-pairing rule between target and guide RNA (gRNA). The role of gRNA is to steer Cas enzyme to the custom positions in the presence of a protospacer adjacent motif (PAM) or protospacer flanking sequence (PFS) (4). PAM/PFS is a recognizable component following the target sites that enables precise cleavages on exogenous nucleic acids complementary to gRNA. In different types of CRISPR/Cas systems, gRNA could be the CRISPR RNA (crRNA), a kind of short non-coding RNAs derived from CRISPR arrays, or the synthetic formed by crRNA and trans-activating crRNA (tracrRNA). Besides, the category of CRISPR/Cas systems can be divided into two classes and subdivided into six types and 30 subtypes by different kinds of Cas effector module organizations, the position of the CRISPR array and acquisition module (5). As shown in Figure 1A, type I, III, and IV CRISPR/Cas systems have multi-subunit effector complexes and thereby collectively belong to class 1, while class 2 containing type II, V, and VI systems has a simpler architecture composed of only one protein effector (6–8).
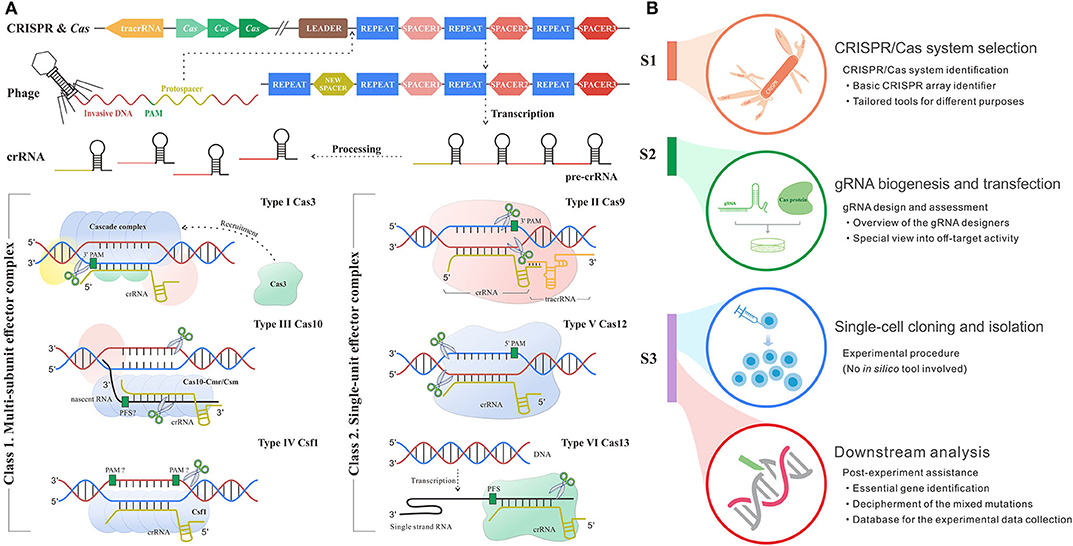
Figure 1. Schematic diagram shows the mechanism and workflow of CRISPR/Cas adaptive immune system. (A) The mechanism of CRISPR/Cas system. S1: Adaptation stage. The invasive DNA sequence produced by phage is cleaved and incorporated into the start of a CRISPR array comprised of a string of spacers flanked by repeats, forming a new spacer downstream leader. S2: CRISPR RNA (crRNA) biogenesis stage. The precursor of crRNA transcribed from CRISPR array is further processed into mature crRNA, which carries the genetic information from spacer. S3: Interference stage across six main types of systems. In type I system (signature protein: Cas3), the multimeric effector, Cascade, binds to target DNA complementary to crRNA and then recruits Cas3 to generate the single-strand nick. Type II system (signature protein: Cas9) encodes tracRNA to hybridize with crRNA and form a dual tracRNA:crRNA complex, which guides Cas9 enzyme to the target and thus generates blunt double-strand breaks (DSBs). In type III system (signature protein: Cas10), Cas10-Cmr/Csm complex recognizes the nascent target RNA following by the new enzymatic activity for complementary DNA cleavage. Type IV system (signature protein: Csf1) remains mostly unknown, although current research had demonstrated the crRNA maturation and proved its evolutionary connection with type I system (15). Type V system (signature protein: Cas12) solely relies on the formation of a binary complex between crRNA and Cas12 enzyme to identify target sequence and triggers staggered DSBs. In type VI system (signature protein: Cas13), crRNA binds to single-strand RNA through the protospacer flanking sequence (PFS) reorganization and guides Cas13 to realize the cleavage. (B) The workflow of CRISPR/Cas-mediated gene editing includes CRISPR/Cas system selection, guide RNA (gRNA) biogenesis and transfection, single-cell cloning and isolation, and downstream analysis. The subheadings under the main title represent the processes where in silico methods are involved. The flow linking the left and right panels represents the correspondence. For example, red flow shows that the implement of downstream analysis corresponds to the stage after CRISPR inference.
Up to now, CRISPR/Cas system has been extensively applied in fundamental studies (9) as well as clinical practices across multiple diseases (10, 11). Of note, the discovery and implementation of CRISPR/Cas system require an intricate workflow (Figure 1B) including CRISPR/Cas system identification and selection, gRNA design, transfection, single-cell clone establishment, clone screening, and systematic mutation analysis (12–14). Each step expends considerable time, money, and manpower. Fortunately, the advance in computer science creates scope for remedying the deficiency and fueling the overall procedure (Figure 1B). In silico methods based on different algorithms and frameworks harbor different merits and are appropriate for diverse applications. Even though a variety of in silico tools goes on a growth spurt over recent years, there is a lack of a comprehensive summary for their roles in the overall procedure from system identification to application, so that many biological researchers are likely lost in the selection of suitable tools for their given intention. Therefore, it is necessary and urgent to make an explicit review of the existing tools.
In this review, we aim to summarize the released in silico methods from three major aspects (CRISPR/Cas system identification, gRNA design, and post-experimental assistance), discuss the relative merits, expound their applicability for various purposes, and put forward the possible assumptions for further improvements. We believe that our review is capable of elaborating on the roles of in silico toolkits in CRISPR/Cas system to formulate meaningful guidance for biological researchers and even provide significant clues for future tools development.
CRISPR/Cas System Identification
At the phase of adaptation, bacteria copy a DNA segment (protospacer) from the invasive phages or plasmids and paste it to the start of the CRISPR array downstream of the leader sequence as a new spacer (Figure 1A) (3, 16, 17). CRISPR arrays are then transcribed and processed into crRNAs that possess partial genetic information of the invasive DNA and thus are able to form gRNA or directly guide Cas protein to the planned position (6). Since crRNAs and Cas protein, respectively, take full control of the specificity and editing efficiency of CRISPR/Cas systems, identification and classification of CRISPR/Cas system composed of different types of crRNAs and Cas proteins must be the most fundamental prerequisite for the downstream application.
Recognition of CRISPR Arrays That Generate crRNAs
The most important component of CRISPR/Cas system, crRNA, is generated from CRISPR arrays (Figure 1A). Therefore, recognition of efficient CRISPR arrays largely determines the engineering specificity in the application. Until now, a variety of computational methods have been proposed to recognize CRISPR arrays using sequence information. One of the earliest tools, PatScan (18), was developed long before CRISPR/Cas system was applied in gene editing, which searches for the fragments homologous to the predefined pattern. However, PatScan was designed to detect general repeat not specific for CRISPRs, causing the inability of distinguishing the spacers and repeats in the whole CRISPR array. Later, several specific CRISPR identifiers came along, such as CRISPRFinder (19), PILER-CR (20), and CRT (21). The principle of CRISPRFinder (19) is using the suffix tree-based algorithm to find the maximal repeats that are clamped by the non-repeating sequences with similar length. Besides, PILER-CR (20) based on the alignment matrix identifies putative CRISPR arrays through searching local hits of the query genome to itself and uses sequence similarity, conservation, and length distribution to refine them. Different from CRISPRFinder and PILER-CR, CRT (21) does not rely on any central data structure but adopts the strategy of simple sequential scanning, which enables a high execution speed independent of the number of repeats in the given genome. Afterward, CRISPRDetect (22) based on k-mer and extension strategy was proposed and labeled itself with the improvement of utilizing the features of CRISPR loci especially mutations. CRISPRDetect (22) is more sensitive to short and degenerated repeats by scanning for the variant repeats under a low identity threshold in long spacers, but it incidentally brings the possibility of wrong segmentation of the large integral CRISPRs. The comparison of the advantages and disadvantages of the abovementioned basic CRISPRs identifiers was demonstrated in Table 1.
Along with the diversity of research demand, there are some tools derived from the basic identifiers and tailored for different purposes (Table 2). One of the most popular purposes now is to explore the CRISPR diversity from metagenomic data and classify the CRISPR/Cas system. Due to the repetitive nature and population heterogeneity, it is hard to assemble CRISPRs from metagenomes using basic tools. Therefore, MinCED (23), MetaCRAST (24), Crass (25), and metaCRT (26) were developed. MinCED, Crass, and MetaCRT are all based on CRT (21) tool and implement the de novo detection. Moreover, MinCED and Crass have no need for prior knowledge of CRISPR arrays of which MinCED only detects spacers in reads without assembly and Crass assembles the reads into arrays. In contrast, metaCRT (26) integrates the reference-based and de novo detection. Besides, MetaCRAST (24), another reference-based method, searches for repeats pairing with the user-defined templates that could be identified by either other tools like CRISPRFinder, PILER-CR, and CRF or taxonomy, whereas its performance is inferior to Crass and MinCED for the poor taxonomic diversity. In addition, there are also some tools tailored for other purposes. For instance, if users want to compare the CRISPR arrays from different species, CRISPRcompar (27) comprising CRISPRcomparison and CRISPRtionary and basically derived from CRISPRFinder must be the best choice. Besides, CRF (28) based on CRT added random forest algorithm to make an extra filtration for invalid CRISPR arrays, but this learning-based tool may partially lose the ability to discover new CRISPRs. Beyond that, three representative tools are designed for CRISPR strand prediction using the characteristics of leader and repeat that include CRISPRstrand (29), CRISPRleader (31), and CRISPRDirection (37).
Incorporation With Cas Protein Detector
Other than the abovementioned tools only focusing on CRISPR arrays, recent tools integrate Cas protein detector to improve the classification capacity and enable the automated CRISPR/Cas system discovery. These tools determine the putative Cas protein by using the homologous sequence searcher such as BLAST (38) and HMMER (39), which compare the query Cas protein with the sequences in a known protein database. For example, CRISPRmap (34) is composed of CRT and CRISPRFinder for CRISPR array identification and HMMER for Cas protein annotation. CRISPRdisco (32) incorporates MinCED and BLAST to realize similar functions. Besides, CRISPRCasFinder based on CRISPRFinder for CRISPR array identification integrates the function of Cas protein detection by using a dedicated tool MacSyFinder (40), which is in essence HMMER. Except for the predictors, there are some databases collecting the predicted CRISPRs and Cas proteins such as CRISPRBank (30), CRISPRone (35), and CRISPRCasdb (CRISPRdb) (36).
Although much effort had been invested in the CRISPR/Cas system identification and classification, there are still some unsolved limitations. On one hand, identifying CRISPR arrays especially short arrays based only on pattern alignment or along with limited sequence information is not enough to accurately eliminate noises. It is an imperative trend, as the progression from basic tools to tailored tools, to excavate and incorporate more significant architectural and functional features such as the transcriptional polarity within CRISPRs (41) and regulatory relationships with endogenous genes in a bacterial host (42) to improve the prediction performance. On the other hand, current tools for Cas protein detection are majorly based on the annotation propagation by searching for homologous sequences, which narrows the possibility of discovering novel Cas proteins.
Guide RNA Design and Assessment
As a key component of CRISPR/Cas system, gRNA specifies the target of Cas enzymes through PAM recognition. The quality of gRNA largely determines the efficacy and specificity of CRISPR/Cas-mediated editing. To date, there have been several types of RNAs found to play guiding roles via various mechanisms in different CRISPR/Cas systems (Figure 1), such as the mature crRNA in CRISPR/Cas12a (formerly Cpf1) system (43) and the hybrid of crRNA and tracRNA in CRISPR/Cas9 system (44). In this section, these RNAs with guiding functions have a joint name, gRNA.
With the wider applications of the CRISPR/Cas system, an increasing number of studies expressed their apprehensions over the incidental off-target effects, which may trigger the mis-editing at other loci and lead to unforeseeable phenotypic alterations (45, 46). Thereupon, designing an efficient and functional gRNA with both high on-target efficacy and low off-target mutations becomes the focus of much attention. Recent computational efforts have taken a massive step toward high-quality gRNA design. In what follows, we will set forth the usages and contributions of gRNA designers from two subsections, Overview of the gRNA Designers and Special View Into Off-Target Activity.
Overview of the gRNA Designers
Owing to the simple architecture and superior operability, class 2 CRISPR/Cas systems (Figure 1) gain much wider applications. Consequently, almost all current in silico gRNA designers are developed for class 2 systems. The following description is also confined to the class 2 CRISPR systems.
By different inner principles, we divided the gRNA designers into three major genres (Figure 2). The characteristics of the representative tools in each genre were shown in Table 3.
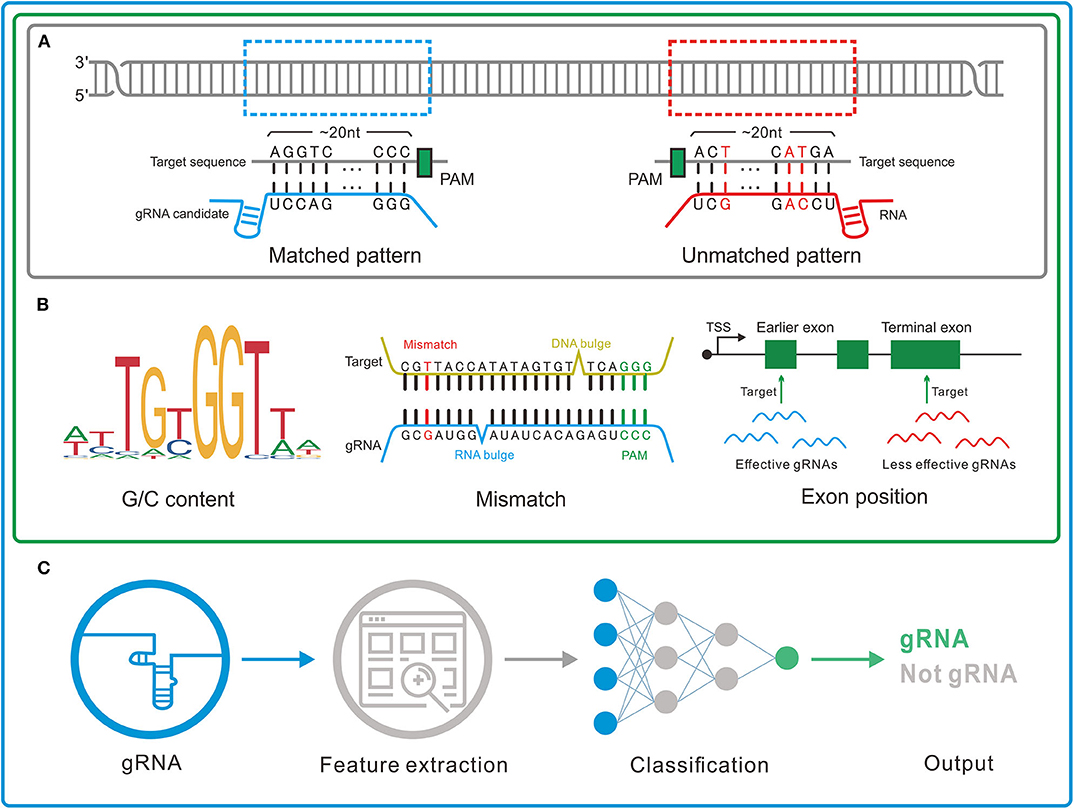
Figure 2. Three genres of guide RNA (gRNA) designers. (A) Pattern recognition genre. The tools in this genre depend on the base-pairing rule to determine the gRNAs. (B) Feature rule genre. A set of features such as G/C content, mismatch, and gRNA transcription method is used to filter out the unreliable or unconcerned gRNAs obtained by pattern recognition. (C) Machine learning genre. In this genre, machine learning algorithms are applied to integrate the effects of the features and thus more precisely identify the gRNAs.
1) Pattern recognition genre (Figure 2A) relying on base-pairing principle. In this category, tools search for a piece of sequence comprising a short PAM and around 20-bp candidate gRNA complementary to the query sequence in a specified genome. The fewer mismatches the candidate gRNA has, the greater on-target possibility it likely produces. Besides, the specific PAM should be predefined for its diversity in different CRISPR/Cas systems. Another factor influencing gRNA pattern is the transcription methods, in which U6 and T7 promoters, respectively, require G and GG at 5'end of gRNA (87, 88). Some tools such as CRISPRseek (49) and flyCRISPR (50) take it into account while others such as SSFinder (48) and GT-Scan (51) do not. Besides, for individual studies, Crisflash (54) is able to improve the accuracy by incorporating user-supplied somatic mutation data into pattern matching.
2) Feature rule genre (Figure 2B). The subsequent finding that editing activities vary across different target sites indicates the inherent disparity of some targets in the sensitivity to cleavage (89–92) and thus ushers a series of explorations to seek out the key features that influence the targeting efficacy (93, 94). These features include G/C content of gRNAs (high or low G/C content indicates less activity) (95), frequency of frameshift mutations (negative with CRISPR efficacy) (96), poly-T sequences (a typical terminator for gRNA transcription) (97, 98), compositions of nucleobases involved in Cas binding preference (the presence of PAM-preceding G and the absence of pyrimidines in the last 4nt of gRNA spacers are preferred) (63), exon position (lower efficacy when gRNAs targeting the terminal coding exon rather than the earlier exons) (99), the status of the motif- and feature-enriched ~10–12 nt proximal to PAM in spacer sequences dubbed seed region (associated with pairing process) (100, 101), and so on. Tools in this genre always integrate several measurable features with the basic pattern recognition approach to provide more information about candidate gRNAs and target sites. According to feature indexes and the corresponding thresholds, users can lay down their own rules to filter out the gRNAs with poor reliability or of no interest. For instance, Cas-Designer (56) lists putative gRNAs along with G/C proportions and out-of-frame scores that indicate the frequency of in-frame mutations. Besides, CRISPR-ERA (60) constructs a simple scoring rule by arbitrarily quantifying and weighting the information of G/C content, poly-T motifs and target locations.
Tools affiliated to this genre provide separate assessment or arbitrary combinations for multiple features rather than perform an integrative analysis on their interactive contributions, which may perplex users about how to balance the probably discordant results of multiple features. Machine learning algorithms found an exit for this dilemma.
3) Machine learning genre (Figure 2C). Given that the weights of multiple features remain uncertain, researchers resort to mathematical algorithms that systematically integrate features for refining optimal gRNA. These models always differ in algorithms and information in training data. For example, Doench et al. (95) (Rule set 1) observed the depletion rates of gRNAs targeting cell surface markers in mouse and human cells and attributed them to the intrinsic nucleotide composition of target sequences, which then acted as training data to construct the logistic regression classifier for gRNA activity prediction. Moreover, combining the changes in expression of cell surface markers (Rule set 1) (95) and drug resistance pathways (Rule set 2), Azimuth (102) trained by the information of not only nucleotide composition but also secondary structure of gRNAs and the relative location of target sites to the transcription start site (TSS) shows improved performance. Unlike above methods using phenotypic changes to measure activity, some others relying on mutations detected by sequencing were proposed. CRISPRscan (75), a linear regression model, investigated the effect of nucleotide composition on CRISPR/Cas9 efficacy by taking the gRNA-induced mutation rates of target sequences in zebrafish embryos as the signal of activity. In addition, sgRNA Scorers v2.0 (76) based on the support vector machine used similar training data from sequencing (mutation rates of the targets in human HEK293T cells). Likewise, TUSCAN (77) reanalyzed the published data and improved the prediction performance by adding the features of flanking target regions and replacing the algorithm with random forest. For fear of the potential biases caused by the manual selection of features in abovementioned tools based on the conventional machine learning algorithm, up-to-date tools (80, 81, 86) based on deep learning algorithm minimize the biases by automating feature extraction of which DeepCRISPR (86) is particularly noteworthy for unifying both on-target and off-target predictions into one framework and additionally allowing for epigenetic features despite using phenotype-driven data.
Phenotype-driven models are largely influenced by the target positions, some of which far from TSS less likely trigger phenotypic change and would be misclassified into the negative. In contrast, sequencing-based models implement more direct measurement of genetic mutations and have consequently superior generalizability (77). In a word, phenotype-driven models get the upper hand when users are more interested in the functional outcome of gRNA-induced mutations, while sequencing-based models occupy wider application fields if only genotype alterations are focused.
Even though in silico gRNA designers experience a positive evolution, the performances of machine learning-based tools remain difficult to maintain due to the varying features across different species and Cas enzymes requiring an exclusive loading process. Therefore, users were recommended to use the tools based on feature rules if their data are not eligible for the machine learning algorithm. Except for the abovementioned categorical characteristic, gRNA designers also have other distinguishable specialties such as the one-step customization of paired gRNA (pgRNA) for large fragment deletion [e.g., CRISPETa (70), pgRNAFinder (73), and GuideScan (82)], special consideration for CRISPR activation or interference (CRISPRa/i) (103) [e.g., SSC (63), CRISPR-ERA (60), and CHOPCHOP v3.0 (71)], application platform, off-target prediction, and so on. These specialties endow the tools with distinctive ability in particular fields and thus give users more choices for their specific purpose. Moreover, some commercial tools should also be helpful for their visual interface, online consultation, and one-stop ordering service, such as Synthego (https://www.synthego.com/products/bioinformatics/crispr-design-tool) based on the Azimuth algorithm (102) and IDT (https://www.idtdna.com/site/order/designtool/index/CRISPR_CUSTOM) based on their own evaluation algorithm, but most of the commercial tools were designed for the most popular CRISPR/Cas9 system and provided less support for other types of CRISPR systems. Table 3 recording the detailed comparison of some commonly used gRNA designers provides a more brief reference. Since no tool can be omnipotent, the pre-conditions and anticipated purpose should be fully thought before the gRNA designer selection.
Special View Into Off-Target Activity
Off-target activity leading to mis-editing on the unintended regions had been widely reported, which can trigger unpredictably adverse outcomes (104, 105). Undoubtedly, experimental methods including whole-genome sequencing [e.g., CIRCLE-seq (106), GUIDE-seq (107), DISCOVER-seq (108), Digenome-seq (109), BLESS (110), and HTGTS (111)] and the improved VIVO strategy (112) are relatively robust and accurate for off-target identification. Nonetheless, the labor- and cost-intensive sequencing methods are not affordable for every researcher and sometimes unnecessary, thus urging the coming and progress of in silico methods.
The most typical and convenient in silico strategy for off-target risk evaluation is to align the short gRNA sequences sometimes with PAMs to reference genome to detect mismatch number and position by repurposing the alignment tools [e.g., Bowtie (113), PatMaN (114), and BWA (115)], which is exemplified by GT-Scan (51), CRISPR-RT (61), E-CRISP (65), and so on. However, short read aligners likely induce a large proportion of false-negative errors due to their maximum allowable mismatches. When mismatch number exceeds 2 in a certain read, the accuracy of aligners gets a drastic decline (116). The comparison between the gold standard GUIDE-seq (107) and the alignment strategy revealed that numerous high-mismatch off-targets and even one-mismatch off-targets cannot be detected by only alignment (107). On the other hand, the limited mismatches are hard to represent the authentic off-targets and may cause false-positives. This is supported by an experiment based on SITE-seq, which found that the alignment-based off-targets largely outnumbered the validated off-targets by up to 10-fold (117).
Aiming to narrow both types of errors and realize the quantitative evaluation on off-target possibility, some features and scoring systems are incorporated into the prediction programs (Figure 3). For example, CCTop (67) and CROP-IT (59), respectively, incorporate seed region and DNase-sensitive region with mismatch number to grade the potential off-target sites using handcraft rules. Furthermore, mismatches with a few extra bases (DNA bulge) or missing bases (RNA bulge) in target sequences were once reported to be tolerable (118). COSMID (119) lists the number of bulges rather than incorporates it into the scoring rule for the lack of experimentally validated data. Despite the additional features in the above tools, the off-target searching method they used still relies on alignment strategy, which is not as reliable as the sequencing-based off-target source used in following tools. By introducing the mutated gRNAs into cells and measuring the gRNA abundance to quantify the off-target activities, CFD (102) exhibited more dominant power and has been widely repurposed in other tools such as CRISPR-Local (84), GuideScan (82), and GPP sgRNA designer (78). In contrast with the discontinued MIT-Broad algorithm (120) whose scans area confines to 20-bp sequences, CFD (102) covers PAM as it found non-canonical PAMs tend to induce potential off-target events (102). Subsequently, researchers proved CFD's superior performance by comparison with experimental data (121). However, it should be noted that CFD only aggregates the off-targets within a certain gene rather than a genome-wide scale.
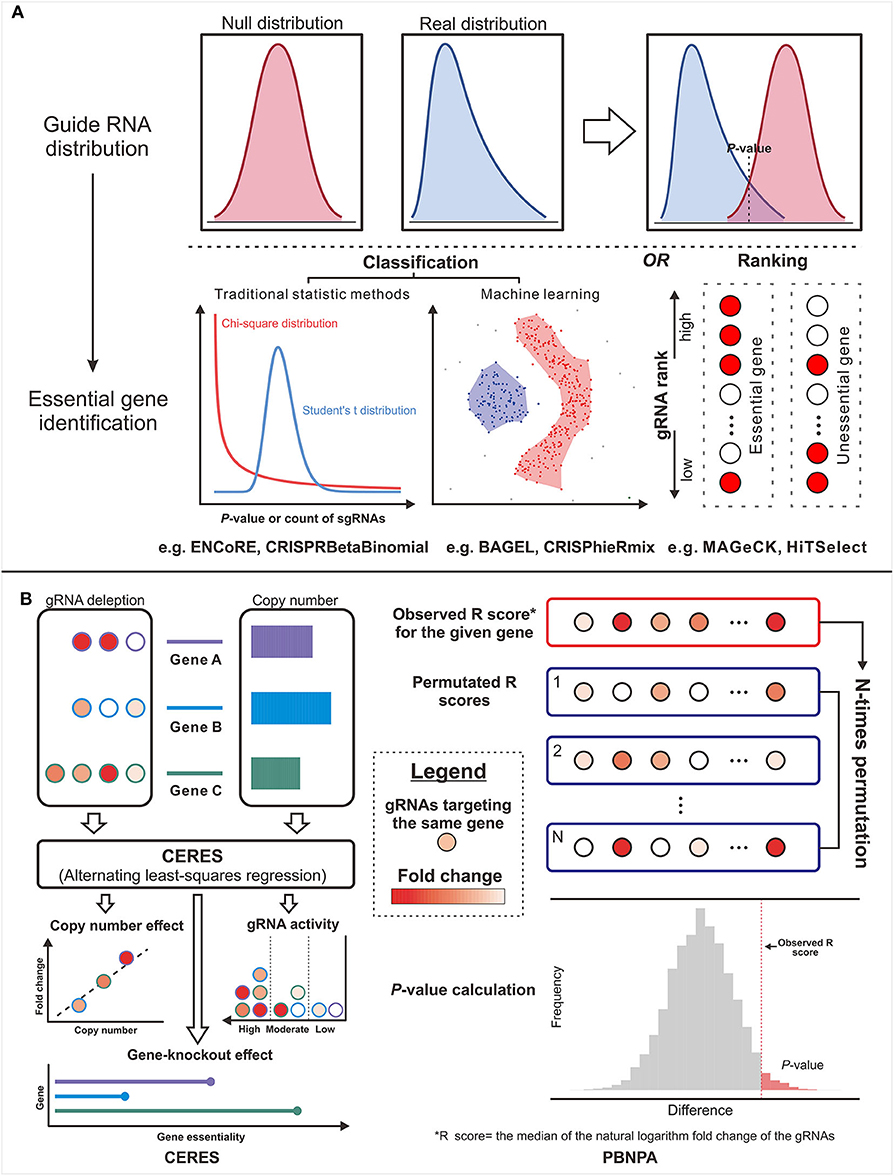
Figure 3. The design concepts of the gene essentiality evaluators. (A) The typical genre is from the guide RNA (gRNA) distribution comparison to essential gene identification. (B) Two methods in untypical ways: The left panel illustrates the workflow of CERES, which corrects the copy number effect based on the alternating least-squares regression. The right panel illustrates the workflow of PBNPA based on the permutation test.
To overcome the drawbacks of handcraft rules and extend the aggregation scale, recent developers are more inclined to machine learning algorithm (Figure 3). CRISTA (122) constructed a random forest model based on the enlarged feature set covering mismatch types (wobble and bulge), chromatin accessibility, DNA enthalpy, and DNA geometry. Regrettably, the complex feature set creates a double-edge sword, which indeed enhances the prediction performance but also restricts the application scope. Using simpler features, Elevation (123), a genome-wide aggregation model based on Naive Bayes, provides a more systematic assessment for multi-loci off-target detection. Besides, the state-of-the-art deep learning algorithm was also applied using only sequencing data and achieved a relatively better result (124). Deep learning takes more full advantage of experimental datasets, whereas the lack of aggregation function and the narrow feature set remain an intractable limitation. The evolution of the original off-target scoring systems is illustrated in Figure 3.
In conclusion, an optimal gRNA should possess not only maximum on-target efficacy but also minimum off-target activity, which requires in silico designers equipped with both high accuracy and robustness. Moreover, the incorporation of more functional features is a key to improve prediction performance. As genetic researches are stepping forward, some additional factors such as histone modification (93, 125) and Cas protein variants (126) were found to exert significant influences on editing efficacy and specificity. Besides, what wins the most attention recently must be individual variance that was reported to be discriminately associated with the genesis or destruction of the potential off-target activity (127–129). Therefore, the applications of CRISPR/Cas system especially for clinical purposes would better be specified into the individual scale to control the risk of deleterious side effects.
Post-Experimental Assistance
CRISPR/Cas-mediated high-throughput screening has become a main force to impute phenotypic changes to large-scale genetic or epigenetic alterations. In screening, the pooled gRNA library is amplified, packaged, and transfected into the host cells (130, 131). The transfected cells are screened for a phenotype of interest, of which the survived would be sequenced to measure gRNA abundance. After that, the major challenges turn to be how to precisely transform the differential gRNA abundances after selection to the gene essentiality evaluation and how to systematically enumerate and visualize the CRISPR/Cas-induced mutations. Bioinformaticians have provided innovative solutions using computational methods to boost the experimental procedure as shown in Figure 1B. Hereinafter, in silico methods are introduced in three parts: Essential Gene Identification, Decipherment of the CRISPR-Induced Mutations, and Database for Experimental Data Collection.
Essential Gene Identification
Since CRISPR/Cas-mediated screening strategy was proposed, several sorts of approaches have been put forward to estimate gene essentiality. At the early stage, some off-the-shelf tools for RNA-seq expression analysis [e.g., edgeR (132), baySeq (133), and DEseq2 (134)] served as makeshifts for CRISPR studies. The algorithms designed for RNA interference (RNAi) screens [e.g., RIGER (135) and RSA (136)] were also regarded as substitutes. However, these algorithms cannot exactly achieve satisfying suitability for CRISPR screens due to various deficiencies including the lack of quality control, unrobustness to variable gRNA coverage per gene, and the weak power in controlling the bias toward small sample size or gRNAs with small read count. To fill the gaps, some dedicated methods have been emerging constantly (Figure 4, Table 4). The typical strategy (Figure 4) is to compare the read count distribution of gRNA with control and then aggregate the variances of multiple gRNAs with the same target into an estimate of gene-level effect.
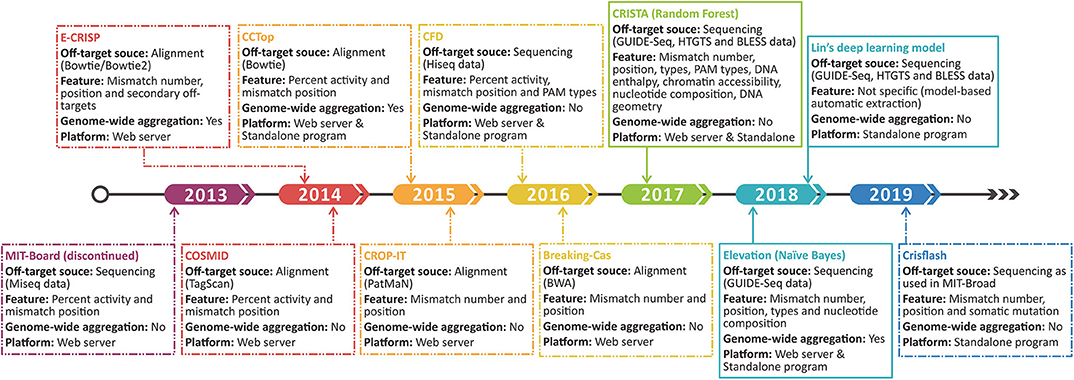
Figure 4. Time line shows the development progress of the original off-target scoring system. The dashed and sealed boxes represent the handcraft and machine learning-based scoring systems, respectively.
MAGeCK-RRA (147) based on the negative binomial model and robust rank aggregation (RRA) is the first tool customized for prioritizing gRNAs, performing gene-level ranking and identifying the enriched pathways. To extend the functions, MAGeCK-RRA (147) was further updated to scMAGeCK (148) for single-cell CRISPR screening (a novel technique combining pooled CRISPR screening with single-cell RNA-seq, which enables the identification of gRNAs at single-cell resolution from sequencing by modifying the lentiviral vector) and MAGeCKFlute (137) with optional ranking algorithm (maximum likelihood estimation) (149), gRNA outlier removal by network essentiality scoring tool (150), and various accessory functions including upstream quality control and downstream visualization. For some novices without programming expertise, command-line programs are hard to tame and the graphical workflow, ENCoRE (141), seems more user-friendly, whereas the rough processing of gene ranking may induce unreliable results. Likewise, a universal analyzer, HiTSelect (138), is designed for both RNAi and CRISPR screens, whereas Poisson distribution used to fit the active gRNA abundance is not applicable because the mean and variance of gRNA count are always not equal. Considering that the variance of gRNA count can be either smaller or greater than the mean, Jeong et al. (146) developed CRISPRBetaBinomial based on beta-binomial distribution model and gained the superior sensitivity as well as lower false-negative rate as expected. Totally different in gene-level statistic, BAGEL (140) and JACKs (143) used the reference sets composed of the identified essential and non-essential genes to analyze the query data. Even though these prior knowledge-based methods reward excellent performance, the required compatibility between reference and query sets and the prohibitive update of the pre-set data remain the critical handicaps for popularization. Allowing for the varying effects of gRNAs targeting the same gene especially in CRISPRa/i screens, CRISPhieRmix (145) took a hierarchical mixture model to deconvolute the gRNA distribution and calculate a posterior probability for genes, in which sufficient gRNAs per gene are required to ensure the full discovery of essential genes.
Other than the above methods affiliated to typical strategy, the methods in other ways provide more options for particular problems. For example, CERES (144) incorporated copy number effect and thus realized improved specificity in the realm of cancer cells (the left panel of Figure 4). Furthermore, PBNPA (142) (the right panel of Figure 4) permuted gRNA labels to compute gene-level p-values, which may outperform the competitors when encountering the small amounts of gRNAs per gene or low sequencing depth. Similarly, ScreenBEAM (139) is another skillful solution for low-quality data owing to the direct estimation on the gene level. The characteristics of existing essentiality evaluators are listed in Table 4.
In general, despite leaving copy number effect out of consideration, MAGeCK (137) remains the most widely used tool in various biological fields such as identifying cancer drivers (151), drug targets (152), and pathway components (153). Its prominent advantages over other tools are the all-around service covering both upstream and downstream analyses, relative ease of use, and the excellent ranking criteria that deal well with variable gRNA efficacies. Meanwhile, there are still positions for other tools when facing the cases they are adept at. ScreenBEAM (139) for low-quality data and ENCoRE (141) for novice users are two representative examples.
Decipherment of the CRISPR-Induced Mutations
Owing to the outstanding feasibility and versatility, type II CRISPR/Cas9 and type V CRISPR/Cas12a occupy the most dominant position in practical use. Double-strand breaks (DSBs) created by Cas9 or Cas12a cleavage can be repaired via several kinds of pathways, which induce the mixed mutations. The repair pathways mainly include (1) non-homologous end joining (NHEJ) (154), which is an error-prone repair pathway and may induce random insertions and deletions (INDELs); (2) homology-directed repair (HDR) (155), which relies on a donor template homologous to the sequence around DSB site to realize the precise editing or correction; and (3) microhomology-mediated end-joining (MMEJ) (156), where the single-stranded overhangs generated by the nuclease are annealed at the microhomologies (typically 5–25 bp) existing both upstream and downstream of DSB. Then, two major methods were used to dissect the mutational outcome. First, some machine learning-based tools, such as in Delphi (157), FORECasT (158), and Lindel (159), used the characteristic of sequence context to achieve a great prediction on the distribution of mutations. However, as similar as other learning-based tools, the application of these tools was largely subject to the training set and cannot be spread across different CRISPR systems and species. Secondly, next-generation sequencing (NGS) can not only detect the mutations but also classify the mutation types and mutagenesis efficiency. Nonetheless, transforming millions of sequencing signals to quantitative and comparable data remains challenging and needs mathematical aids from in silico tools. The fundamental workflow of these tools is similar to the standard high-throughput sequencing analysis including quality control, trimming adaptor, alignment, and quantification. The main difference in the existing tools will be demonstrated as follows.
1) Alignment strategy. The existing tools adopt either local alignment to the reference amplicons [e.g., CRIS.py (160), CRISPR-DAV (161), and CRISPR-GA (162)] or global alignment to an entire reference genome [e.g., CrispRVariants (163) and AmpliconDIVider (164)]. The local strategy is apt to miscount the candidate off-target reads, while global strategy makes it difficult to quantitatively deconvolute the mixed outcomes of gene editing. Besides, some tools [e.g., CRISPResso2 (165) and BATCH-GE (166)] combine both strategies by predefining cut sites. Collectively, choosing an alignment strategy depends on what kind of information users prefer.
2) Deconvolution of the mixed mutations. As mentioned above, three major pathways (NHEJ, MMEJ, and HDR) jointly participate in DSB repair. In contrast to the unpredictable mutations generated by NHEJ, precise modifications generated by HDR and MMEJ are preferred for purposive gene editing. Therefore, classifying the modified alleles is essential for determining the mutant sites and mutagenesis efficiency. The tools adopting local strategy [e.g., CRISPResso2 (165), CRIS.py (160), CRISPR-DAV (161), and CRISPR-GA (162)] align reads to the expected HDR amplicon and the reference amplicon and then identify the modification status by the comparisons of alignment rates and sequence identities. Moreover, some tools [e.g., ampliconDIVider (164), CRIS.py (160), and CRISPResso2 (165)] enable the quantification of in-frame occurrences and potential splice sites according to mutation location and sequence length. The mutations located in the coding region with relatively conserved length are always regarded as in-frame, while the others are frame-shift. Yet regrettably, the tool for distinguishing MMEJ-induced mutations remains unavailable.
3) Applicability for base editors. For fear of the random introduction of INDELs in canonical CRISPR/Cas experiments, base editors, the fusions composed of a catalytically impaired Cas enzyme to a base deaminase that operates on single strand, can directly install point mutations by mediating base conversion without DSB generation (167, 168). Conventional tools only for INDEL quantification cannot detect the varying combinations of base conversion induced by the base editor. Interestingly, CRIS.py (160) and CRISPResso2 (165) compensate for this vacancy through searching the pre-set nucleotide substitution rule.
Additionally, whether the tools are equipped with visualization and the execution platform is worth considering. The detailed information of existing CRISPR NGS data analyzers is listed in Table 5.
Database for Experimental Data Collection
The applications of CRISPR/Cas screening massively expand in gene function exploration, so does the need for the open databases for validated data collection where researchers can easily get access to raw or processed data. To satisfy the urgent need, several repositories had been built (Table 6). Of note, compared with the databases only recording results but without any comparisons of screening results among different researches [e.g., CRISPRz (171), CrisprGE (172), CRISPRlnc (151), and BioGRID ORCS (176)], GenomeCRISPR (173) based on 84 high-throughput screens additionally provides the intuitive comparisons of gRNA efficacies as well as perturbation phenotypes under specific conditions. Instead of collecting the gRNA information, PICKLES (174) reanalyzed the raw screening data and compared the essentiality of a certain gene across multiple experiments, tissues, or cells. Another two independent databases tailored for human cancer research are Sanger DepMap (175) and Broad DepMap (144), which record the information of gene dependencies in cancer cell lines through analyzing the CRISPR/Cas9 screening data.
Furthermore, there are some databases [e.g., Anti-CRISPRdb (177) and CRISPRminer (178)] recording the anti-CRISPR proteins in phage that had been experimentally validated to inhibit the activity of CRISPR/Cas system and reduce off-target events (179).
Conclusion and Perspective
CRISPR/Cas systems have navigated researchers to traverse through the dark where they are left flat-footed by the complex functional annotation. However, the advances in experimental techniques still cannot promise CRISPR/Cas system an effortless and expedite manner, which, therefore, needs essential assistance from in silico methods. Our study makes a comprehensive summary and comparisons on the released tools from two perspectives: pre-experimental guidance (CRISPR/Cas system identification and gRNA design) and post-experimental analysis (gene essentiality evaluation, decipherment of the experimental outcome, and data collection). The characteristics of tools based on different design principles and frameworks had been elucidated hereinbefore, which hopefully guide users to make more reasonable choices for their specific data and purposes.
Unfortunately, CRISPR/Cas system cannot yet reach a satisfying achievement in practical use. Current strategies for technical improvement mainly probe into two aspects. On one hand, the most reliable and effective approach is to optimize the experimental technique, which is well-exemplified by the fusion of catalytically impaired Cas enzymes to other engineered proteins for constructing the riskless systems such as CRISPRa/i (103), base editor (167), and prime editor (180) and enhancing the efficiency of precise repair (181). Yet experimental improvement cannot cover all facets, let alone guarantee affordable cost. At that time, in silico tools, the second aspect, are of importance even if there is still a long way ahead such as how chromatin environment affects the on-target and off-target activities, whether the effects are fixed or varying across tissue and organisms, how to solve the disparity of training set in machine learning-based tools that may cause the poor versatility, and how to combine the individual information into the personalized gRNA design. To the best of our knowledge, the hypotheses of tool optimization are: (1) For CRISPR/Cas system identification, precisely distinguishing CRISPR arrays from other similar repeats requires the incorporation of more distinct features such as the interactions with other genes in the host (42) and the intra-genus conservation (41); (2) For gRNA design, except feature expansion and algorithm optimization, the individual variance associated with on-target and off-target activities (127–129) should be taken into account. Current tools such as Crisflash (54) and CRISPR-Local (84) considering only somatic mutation are far from satisfactory. It is envisioned that in silico tools covering more individual characteristics such as chromatin environment, accessibility, and exon expression promise more reliable prediction, especially for the clinical purpose; (3) For gene essentiality evaluation, existing tools are not as all-powerful as we expected, which misinterpret the uncertain relationships between the mean and variance of gRNA count, neglect the copy number effect, or lack accessory functions; (4) For the deconvolution of mutations, combination of microhomology predictor and local alignment to reference may pave a new way for quantifying the MMEJ-induced mutations.
The urgent demand for optimizing in silico methods cannot mask the truth that they have made tremendous contributions to biological researches. It is increasingly expected that the progress in computational methods will push CRISPR/Cas system into a higher stage and even assist in an earlier realization of clinical popularization.
Author Contributions
All authors listed have made a substantial, direct and intellectual contribution to the work, and approved it for publication.
Funding
This work was supported by the National Natural Science Foundation of China (Grant Number 31970630), the Fundamental Research Funds for the Provincial Universities of Zhejiang (Grant Number SJLZ2021001), Zhejiang Provincial Natural Science Foundation of China (Grant Number LY19H160011), Key Laboratory of Diagnosis and Treatment of Digestive System Tumors of Zhejiang Province (Grant Number 2019E10020), Ningbo Clinical Research Center for Digestive System Tumors (Grant Number 2019A21003), Ningbo Health Branding Subject Fund (Grant Number PPXK2018-05), Natural Science Foundation of Ningbo (Grant Number 2017A610154), Zhejiang Key Laboratory of Pathophysiology (Grant Number 201812), The Scientific Innovation Team Project of Ningbo (Grant Numbers 2016C51001 and 2017C110019), the Fundamental Research Funds for the Provincial Universities of Zhejiang, and K.C. Wong Magna Fund in Ningbo University.
Conflict of Interest
The authors declare that the research was conducted in the absence of any commercial or financial relationships that could be construed as a potential conflict of interest.
Acknowledgments
We would like to appreciate Xi Hu from the Department of Chemical Pathology, The Chinese University of Hong Kong, Prince of Wales Hospital, for her contribution to the figure beautification.
References
1. Gupta SK, Shukla P. Gene editing for cell engineering: trends and applications. Crit Rev Biotechnol. (2017) 37:672–84. doi: 10.1080/07388551.2016.1214557
2. Knott GJ, Doudna JA. CRISPR-Cas guides the future of genetic engineering. Science. (2018) 361:866–9. doi: 10.1126/science.aat5011
3. Barrangou R, Fremaux C, Deveau H, Richards M, Boyaval P, Moineau S, et al. CRISPR provides acquired resistance against viruses in prokaryotes. Science. (2007) 315:1709–12. doi: 10.1126/science.1138140
4. Leenay RT, Beisel CL. Deciphering, communicating, and engineering the CRISPR PAM. J Mol Biol. (2017) 429:177–91. doi: 10.1016/j.jmb.2016.11.024
5. Pickar-Oliver A, Gersbach CA. The next generation of CRISPR-Cas technologies and applications. Nat Rev Mol Cell Biol. (2019) 20:490–507. doi: 10.1038/s41580-019-0131-5
6. Koonin EV, Makarova KS, Zhang F. Diversity, classification and evolution of CRISPR-Cas systems. Curr Opin Microbiol. (2017) 37:67–78. doi: 10.1016/j.mib.2017.05.008
7. Shmakov S, Smargon A, Scott D, Cox D, Pyzocha N, Yan W, et al. Diversity and evolution of class 2 CRISPR-Cas systems. Nat Rev Microbiol. (2017) 15:169–82. doi: 10.1038/nrmicro.2016.184
8. Tang Y, Fu Y. Class 2 CRISPR/Cas: an expanding biotechnology toolbox for and beyond genome editing. Cell Biosci. (2018) 8:59. doi: 10.1186/s13578-018-0255-x
9. Zhu S, Li W, Liu J, Chen CH, Liao Q, Xu P, et al. Genome-scale deletion screening of human long non-coding RNAs using a paired-guide RNA CRISPR-Cas9 library. Nat Biotechnol. (2016) 34:1279–86. doi: 10.1038/nbt.3715
10. Xu L, Wang J, Liu Y, Xie L, Su B, Mou D, et al. CRISPR-edited stem cells in a patient with HIV and acute lymphocytic leukemia. N Engl J Med. (2019) 381:1240–7. doi: 10.1056/NEJMoa1817426
11. Yin H, Xue W, Anderson DG. CRISPR-Cas: a tool for cancer research and therapeutics. Nat Rev Clin Oncol. (2019) 16:281–95. doi: 10.1038/s41571-019-0166-8
12. Ran FA, Hsu PD, Wright J, Agarwala V, Scott DA, Zhang F. Genome engineering using the CRISPR-Cas9 system. Nat Protoc. (2013) 8:2281–308. doi: 10.1038/nprot.2013.143
13. Li B, Zeng C, Dong Y. Design and assessment of engineered CRISPR-Cpf1 and its use for genome editing. Nat Protoc. (2018) 13:899–914. doi: 10.1038/nprot.2018.004
14. Strecker J, Jones S, Koopal B, Schmid-Burgk J, Zetsche B, Gao L, et al. Engineering of CRISPR-Cas12b for human genome editing. Nat Commun. (2019) 10:212. doi: 10.1038/s41467-018-08224-4
15. Ozcan A, Pausch P, Linden A, Wulf A, Schuhle K, Heider J, et al. Type IV CRISPR RNA processing and effector complex formation in aromatoleum aromaticum. Nat Microbiol. (2019) 4:89–96. doi: 10.1038/s41564-018-0274-8
16. Brouns SJ, Jore MM, Lundgren M, Westra ER, Slijkhuis RJ, Snijders AP, et al. Small CRISPR RNAs guide antiviral defense in prokaryotes. Science. (2008) 321:960–4. doi: 10.1126/science.1159689
17. Marraffini LA, Sontheimer EJ. CRISPR interference limits horizontal gene transfer in staphylococci by targeting DNA. Science. (2008) 322:1843–5. doi: 10.1126/science.1165771
18. Dsouza M, Larsen N, Overbeek R. Searching for patterns in genomic data. Trends Genet. (1997) 13:497–8. doi: 10.1016/S0168-9525(97)01347-4
19. Grissa I, Vergnaud G, Pourcel C. CRISPRFinder: a web tool to identify clustered regularly interspaced short palindromic repeats. Nucleic Acids Res. (2007) 35:W52–57. doi: 10.1093/nar/gkm360
20. Edgar RC. PILER-CR: fast and accurate identification of CRISPR repeats. BMC Bioinformatics. (2007) 8:18. doi: 10.1186/1471-2105-8-18
21. Bland C, Ramsey TL, Sabree F, Lowe M, Brown K, Kyrpides NC, et al. CRISPR recognition tool (CRT): a tool for automatic detection of clustered regularly interspaced palindromic repeats. BMC Bioinformatics. (2007) 8:209. doi: 10.1186/1471-2105-8-209
22. Biswas A, Staals RH, Morales SE, Fineran PC, Brown CM. CRISPRDetect: a flexible algorithm to define CRISPR arrays. BMC Genomics. (2016) 17:356. doi: 10.1186/s12864-016-2627-0
23. Skennerton C. T. (2016). MinCED: Mining CRISPRs in Environmental Datasets. Available online at: https://github.com/ctSkennerton/minced/tree/master (accessed September 16, 2020).
24. Moller AG, Liang C. MetaCRAST: reference-guided extraction of CRISPR spacers from unassembled metagenomes. PeerJ. (2017) 5:e3788. doi: 10.7717/peerj.3788
25. Skennerton CT, Imelfort M, Tyson GW. Crass: identification and reconstruction of CRISPR from unassembled metagenomic data. Nucleic Acids Res. (2013) 41:e105. doi: 10.1093/nar/gkt183
26. Rho M, Wu YW, Tang H, Doak TG, Ye Y. Diverse CRISPRs evolving in human microbiomes. PLoS Genet. (2012) 8:e1002441. doi: 10.1371/journal.pgen.1002441
27. Grissa I, Vergnaud G, Pourcel C. CRISPRcompar: a website to compare clustered regularly interspaced short palindromic repeats. Nucleic Acids Res. (2008) 36:W145–48. doi: 10.1093/nar/gkn228
28. Wang K, Liang C. CRF: detection of CRISPR arrays using random forest. PeerJ. (2017) 5:e3219. doi: 10.7717/peerj.3219
29. Alkhnbashi OS, Costa F, Shah SA, Garrett RA, Saunders SJ, Backofen R. CRISPRstrand: predicting repeat orientations to determine the crRNA-encoding strand at CRISPR loci. Bioinformatics. (2014) 30:i489–96. doi: 10.1093/bioinformatics/btu459
30. Naito Y, Hino K, Bono H, Ui-Tei K. CRISPRdirect: software for designing CRISPR/Cas guide RNA with reduced off-target sites. Bioinformatics. (2015) 31:1120–3. doi: 10.1093/bioinformatics/btu743
31. Alkhnbashi OS, Shah SA, Garrett RA, Saunders SJ, Costa F, Backofen R. Characterizing leader sequences of CRISPR loci. Bioinformatics. (2016) 32:i576–85. doi: 10.1093/bioinformatics/btw454
32. Crawley AB, Henriksen JR, Barrangou R. CRISPRdisco: an automated pipeline for the discovery and analysis of CRISPR-Cas systems. CRISPR J. (2018) 1:171–81. doi: 10.1089/crispr.2017.0022
33. Couvin D, Bernheim A, Toffano-Nioche C, Touchon M, Michalik J, Neron B, et al. CRISPRCasFinder, an update of CRISRFinder, includes a portable version, enhanced performance and integrates search for Cas proteins. Nucleic Acids Res. (2018) 46:W246–51. doi: 10.1093/nar/gky425
34. Lange SJ, Alkhnbashi OS, Rose D, Will S, Backofen R. CRISPRmap: an automated classification of repeat conservation in prokaryotic adaptive immune systems. Nucleic Acids Res. (2013) 41:8034–44. doi: 10.1093/nar/gkt606
35. Zhang Q, Ye Y. Not all predicted CRISPR-Cas systems are equal: isolated cas genes and classes of CRISPR like elements. BMC Bioinformatics. (2017) 18:92. doi: 10.1186/s12859-017-1512-4
36. Pourcel C, Touchon M, Villeriot N, Vernadet JP, Couvin D, Toffano-Nioche C, et al. CRISPRCasdb a successor of CRISPRdb containing CRISPR arrays and cas genes from complete genome sequences, and tools to download and query lists of repeats and spacers. Nucleic Acids Res. (2019) 48:D535–44. doi: 10.1093/nar/gkz915
37. Biswas A, Fineran PC, Brown CM. Accurate computational prediction of the transcribed strand of CRISPR non-coding RNAs. Bioinformatics. (2014) 30:1805–13. doi: 10.1093/bioinformatics/btu114
38. Camacho C, Coulouris G, Avagyan V, Ma N, Papadopoulos J, Bealer K, et al. BLAST+: architecture and applications. BMC Bioinformatics. (2009) 10:421. doi: 10.1186/1471-2105-10-421
39. Eddy SR. Accelerated profile HMM searches. PLoS Comput Biol. (2011) 7:e1002195. doi: 10.1371/journal.pcbi.1002195
40. Abby SS, Neron B, Menager H, Touchon M, Rocha EP. MacSyFinder: a program to mine genomes for molecular systems with an application to CRISPR-Cas systems. PLoS ONE. (2014) 9:e110726. doi: 10.1371/journal.pone.0110726
41. Bernick DL, Cox CL, Dennis PP, Lowe TM. Comparative genomic and transcriptional analyses of CRISPR systems across the genus pyrobaculum. Front Microbiol. (2012) 3:251. doi: 10.3389/fmicb.2012.00251
42. Chen J, Li T, Zhou X, Cheng L, Huo Y, Zou J, et al. Characterization of the clustered regularly interspaced short palindromic repeats sites in Streptococcus mutans isolated from early childhood caries patients. Arch Oral Biol. (2017) 83:174–80. doi: 10.1016/j.archoralbio.2017.07.023
43. Zetsche B, Gootenberg JS, Abudayyeh OO, Slaymaker IM, Makarova KS, Essletzbichler P, et al. Cpf1 is a single RNA-guided endonuclease of a class 2 CRISPR-Cas system. Cell. (2015) 163:759–71. doi: 10.1016/j.cell.2015.09.038
44. Karvelis T, Gasiunas G, Miksys A, Barrangou R, Horvath P, Siksnys V. crRNA and tracrRNA guide Cas9-mediated DNA interference in Streptococcus thermophilus. RNA Biol. (2013) 10:841–51. doi: 10.4161/rna.24203
45. Schaefer KA, Wu WH, Colgan DF, Tsang SH, Bassuk AG, Mahajan VB. Unexpected mutations after CRISPR-Cas9 editing in vivo. Nat Methods. (2017) 14:547–8. doi: 10.1038/nmeth.4293
46. Anderson KR, Haeussler M, Watanabe C, Janakiraman V, Lund J, Modrusan Z, et al. CRISPR off-target analysis in genetically engineered rats and mice. Nat Methods. (2018) 15:512–4. doi: 10.1038/s41592-018-0011-5
47. Bae S, Park J, Kim JS. Cas-OFFinder: a fast and versatile algorithm that searches for potential off-target sites of Cas9 RNA-guided endonucleases. Bioinformatics. (2014) 30:1473–5. doi: 10.1093/bioinformatics/btu048
48. Upadhyay SK, Sharma S. SSFinder: high throughput CRISPR-Cas target sites prediction tool. Biomed Res Int. (2014) 2014:742482. doi: 10.1155/2014/742482
49. Zhu LJ, Holmes BR, Aronin N, Brodsky MH. CRISPRseek: a bioconductor package to identify target-specific guide RNAs for CRISPR-Cas9 genome-editing systems. PLoS ONE. (2014) 9:e108424. doi: 10.1371/journal.pone.0108424
50. Gratz SJ, Cummings AM, Nguyen JN, Hamm DC, Donohue LK, Harrison MM, et al. Genome engineering of Drosophila with the CRISPR RNA-guided Cas9 nuclease. Genetics. (2013) 194:1029–35. doi: 10.1534/genetics.113.152710
51. O'brien A, Bailey TL. GT-scan: identifying unique genomic targets. Bioinformatics. (2014) 30:2673–5. doi: 10.1093/bioinformatics/btu354
52. Aach J, Mali P, Church GM. CasFinder: flexible algorithm for identifying specific Cas9 targets in genomes. bioRxiv. [Preprint]. (2014) 005074. doi: 10.1101/005074
53. Oliveros JC, Franch M, Tabas-Madrid D, San-Leon D, Montoliu L, Cubas P, et al. Breaking-Cas-interactive design of guide RNAs for CRISPR-Cas experiments for ENSEMBL genomes. Nucleic Acids Res. (2016) 44:W267–71. doi: 10.1093/nar/gkw407
54. Jacquin ALS, Odom DT, Lukk M. Crisflash: open-source software to generate CRISPR guide RNAs against genomes annotated with individual variation. Bioinformatics. (2019) 35:3146–7. doi: 10.1093/bioinformatics/btz019
55. Xie S, Shen B, Zhang C, Huang X, Zhang Y. sgRNAcas9: a software package for designing CRISPR sgRNA and evaluating potential off-target cleavage sites. PLoS ONE. (2014) 9:e100448. doi: 10.1371/journal.pone.0100448
56. Park J, Bae S, Kim JS. Cas-Designer: a web-based tool for choice of CRISPR-Cas9 target sites. Bioinformatics. (2015) 31:4014–6. doi: 10.1093/bioinformatics/btv537
57. Zhu H, Misel L, Graham M, Robinson ML, Liang C. CT-Finder: a web service for CRISPR optimal target prediction and visualization. Sci Rep. (2016) 6:25516. doi: 10.1038/srep25516
58. Brazelton VA Jr, Zarecor S, Wright DA, Wang Y, Liu J, Chen K, et al. A quick guide to CRISPR sgRNA design tools. GM Crops Food. (2015) 6:266–76. doi: 10.1080/21645698.2015.1137690
59. Singh R, Kuscu C, Quinlan A, Qi Y, Adli M. Cas9-chromatin binding information enables more accurate CRISPR off-target prediction. Nucleic Acids Res. (2015) 43:e118. doi: 10.1093/nar/gkv575
60. Liu H, Wei Z, Dominguez A, Li Y, Wang X, Qi LS. CRISPR-ERA: a comprehensive design tool for CRISPR-mediated gene editing, repression and activation. Bioinformatics. (2015) 31:3676–8. doi: 10.1093/bioinformatics/btv423
61. Zhu H, Richmond E, Liang C. CRISPR-RT: a web application for designing CRISPR-C2c2 crRNA with improved target specificity. Bioinformatics. (2018) 34:117–9. doi: 10.1093/bioinformatics/btx580
62. Prykhozhij SV, Rajan V, Gaston D, Berman JN. CRISPR multitargeter: a web tool to find common and unique CRISPR single guide RNA targets in a set of similar sequences. PLoS ONE. (2015) 10:e0119372. doi: 10.1371/journal.pone.0119372
63. Xu H, Xiao T, Chen CH, Li W, Meyer CA, Wu Q, et al. Sequence determinants of improved CRISPR sgRNA design. Genome Res. (2015) 25:1147–57. doi: 10.1101/gr.191452.115
64. Peng D, Tarleton R. EuPaGDT: a web tool tailored to design CRISPR guide RNAs for eukaryotic pathogens. Microb Genom. (2015) 1:e000033. doi: 10.1099/mgen.0.000033
65. Heigwer F, Kerr G, Boutros M. E-CRISP: fast CRISPR target site identification. Nat Methods. (2014) 11:122–3. doi: 10.1038/nmeth.2812
66. Liu H, Ding Y, Zhou Y, Jin W, Xie K, Chen LL. CRISPR-P 2.0: an improved CRISPR-Cas9 tool for genome editing in plants. Mol Plant. (2017) 10:530–32. doi: 10.1016/j.molp.2017.01.003
67. Stemmer M, Thumberger T, Del Sol Keyer M, Wittbrodt J, Mateo JL. CCTop: an intuitive, flexible and reliable CRISPR/Cas9 target prediction tool. PLoS ONE. (2015) 10:e0124633. doi: 10.1371/journal.pone.0124633
68. Labuhn M, Adams FF, Ng M, Knoess S, Schambach A, Charpentier EM, et al. Refined sgRNA efficacy prediction improves large- and small-scale CRISPR-Cas9 applications. Nucleic Acids Res. (2018) 46:1375–85. doi: 10.1093/nar/gkx1268
69. Zhao C, Zheng X, Qu W, Li G, Li X, Miao YL, et al. CRISPR-offinder: a CRISPR guide RNA design and off-target searching tool for user-defined protospacer adjacent motif. Int J Biol Sci. (2017) 13:1470–8. doi: 10.7150/ijbs.21312
70. Pulido-Quetglas C, Aparicio-Prat E, Arnan C, Polidori T, Hermoso T, Palumbo E, et al. Scalable design of paired CRISPR guide RNAs for genomic deletion. PLoS Comput Biol. (2017) 13:e1005341. doi: 10.1371/journal.pcbi.1005341
71. Labun K, Montague TG, Krause M, Torres Cleuren YN, Tjeldnes H, Valen E. CHOPCHOP v3: expanding the CRISPR web toolbox beyond genome editing. Nucleic Acids Res. (2019) 47:W171–4. doi: 10.1093/nar/gkz365
72. Zhu H, Liang C. CRISPR-DT: designing gRNAs for the CRISPR-Cpf1 system with improved target efficiency and specificity. Bioinformatics. (2019) 35:2783–9. doi: 10.1093/bioinformatics/bty1061
73. Xiong Y, Xie X, Wang Y, Ma W, Liang P, Songyang Z, et al. pgRNAFinder: a web-based tool to design distance independent paired-gRNA. Bioinformatics. (2017) 33:3642–4. doi: 10.1093/bioinformatics/btx472
74. Wong N, Liu W, Wang X. WU-CRISPR: characteristics of functional guide RNAs for the CRISPR/Cas9 system. Genome Biol. (2015) 16:218. doi: 10.1186/s13059-015-0784-0
75. Moreno-Mateos MA, Vejnar CE, Beaudoin JD, Fernandez JP, Mis EK, Khokha MK, et al. CRISPRscan: designing highly efficient sgRNAs for CRISPR-Cas9 targeting in vivo. Nat Methods. (2015) 12:982–8. doi: 10.1038/nmeth.3543
76. Chari R, Yeo NC, Chavez A, Church GM. sgRNA Scorer 2.0: a species-independent model to predict CRISPR/Cas9 activity. ACS Synth Biol. (2017) 6:902–4. doi: 10.1021/acssynbio.6b00343
77. Wilson LOW, Reti D, O'brien AR, Dunne RA, Bauer DC. High activity target-site identification using phenotypic independent CRISPR-Cas9 core functionality. CRISPR J. (2018) 1:182–190. doi: 10.1089/crispr.2017.0021
78. Sanson KR, Hanna RE, Hegde M, Donovan KF, Strand C, Sullender ME, et al. Optimized libraries for CRISPR-Cas9 genetic screens with multiple modalities. Nat Commun. (2018) 9:5416. doi: 10.1038/s41467-018-07901-8
79. Concordet JP, Haeussler M. CRISPOR: intuitive guide selection for CRISPR/Cas9 genome editing experiments and screens. Nucleic Acids Res. (2018) 46:W242–5. doi: 10.1093/nar/gky354
80. Kim HK, Min S, Song M, Jung S, Choi JW, Kim Y, et al. Deep learning improves prediction of CRISPR-Cpf1 guide RNA activity. Nat Biotechnol. (2018) 36:239–41. doi: 10.1038/nbt.4061
81. Kim HK, Kim Y, Lee S, Min S, Bae JY, Choi JW, et al. SpCas9 activity prediction by DeepCas9, a deep learning-based model with unparalleled generalization performance. bioRxiv. [Preprint]. (2019) 636472. doi: 10.1101/636472
82. Perez AR, Pritykin Y, Vidigal JA, Chhangawala S, Zamparo L, Leslie CS, et al. GuideScan software for improved single and paired CRISPR guide RNA design. Nat Biotechnol. (2017) 35:347–9. doi: 10.1038/nbt.3804
83. Heigwer F, Zhan T, Breinig M, Winter J, Brugemann D, Leible S, et al. CRISPR library designer (CLD): software for multispecies design of single guide RNA libraries. Genome Biol. (2016) 17:55. doi: 10.1186/s13059-016-0915-2
84. Sun J, Liu H, Liu J, Cheng S, Peng Y, Zhang Q, et al. CRISPR-Local: a local single-guide RNA (sgRNA) design tool for non-reference plant genomes. Bioinformatics. (2019) 35:2501–3. doi: 10.1093/bioinformatics/bty970
85. Schaefer M, Clevert DA, Weiss B, Steffen A. PAVOOC: designing CRISPR sgRNAs using 3D protein structures and functional domain annotations. Bioinformatics. (2019) 35:2309–10. doi: 10.1093/bioinformatics/bty935
86. Chuai G, Ma H, Yan J, Chen M, Hong N, Xue D, et al. DeepCRISPR: optimized CRISPR guide RNA design by deep learning. Genome Biol. (2018) 19:80. doi: 10.1186/s13059-018-1459-4
87. Sander JD, Joung JK. CRISPR-Cas systems for editing, regulating and targeting genomes. Nat Biotechnol. (2014) 32:347–55. doi: 10.1038/nbt.2842
88. Zhang T, Gao Y, Wang R, Zhao Y. Production of guide RNAs in vitro and in vivo for CRISPR using ribozymes and RNA polymerase II promoters. Bio Protoc. (2017) 7:e2148. doi: 10.21769/BioProtoc.2148
89. Fu Y, Foden JA, Khayter C, Maeder ML, Reyon D, Joung JK, et al. High-frequency off-target mutagenesis induced by CRISPR-Cas nucleases in human cells. Nat Biotechnol. (2013) 31:822–6. doi: 10.1038/nbt.2623
90. Fu Y, Sander JD, Reyon D, Cascio VM, Joung JK. Improving CRISPR-Cas nuclease specificity using truncated guide RNAs. Nat Biotechnol. (2014) 32:279–84. doi: 10.1038/nbt.2808
91. Koike-Yusa H, Li Y, Tan EP, Velasco-Herrera Mdel C, Yusa K. Genome-wide recessive genetic screening in mammalian cells with a lentiviral CRISPR-guide RNA library. Nat Biotechnol. (2014) 32:267–73. doi: 10.1038/nbt.2800
92. Shalem O, Sanjana NE, Hartenian E, Shi X, Scott DA, Mikkelson T, et al. Genome-scale CRISPR-Cas9 knockout screening in human cells. Science. (2014) 343:84–7. doi: 10.1126/science.1247005
93. Chari R, Mali P, Moosburner M, Church GM. Unraveling CRISPR-Cas9 genome engineering parameters via a library-on-library approach. Nat Methods. (2015) 12:823–6. doi: 10.1038/nmeth.3473
94. Horlbeck MA, Witkowsky LB, Guglielmi B, Replogle JM, Gilbert LA, Villalta JE, et al. Nucleosomes impede Cas9 access to DNA in vivo and in vitro. Elife. (2016) 5:e12677. doi: 10.7554/eLife.12677.022
95. Doench JG, Hartenian E, Graham DB, Tothova Z, Hegde M, Smith I, et al. Rational design of highly active sgRNAs for CRISPR-Cas9-mediated gene inactivation. Nat Biotechnol. (2014) 32:1262–7. doi: 10.1038/nbt.3026
96. Bae S, Kweon J, Kim HS, Kim JS. Microhomology-based choice of Cas9 nuclease target sites. Nat Methods. (2014) 11:705–6. doi: 10.1038/nmeth.3015
97. Billon P, Bryant EE, Joseph SA, Nambiar TS, Hayward SB, Rothstein R, et al. CRISPR-mediated base editing enables efficient disruption of eukaryotic genes through induction of STOP codons. Mol Cell. (2017) 67:1068–79.e1064. doi: 10.1016/j.molcel.2017.08.008
98. Tong Y, Whitford CM, Robertsen HL, Blin K, Jorgensen TS, Klitgaard AK, et al. Highly efficient DSB-free base editing for streptomycetes with CRISPR-BEST. Proc Natl Acad Sci USA. (2019) 116:20366–75. doi: 10.1073/pnas.1913493116
99. Wang T, Wei JJ, Sabatini DM, Lander ES. Genetic screens in human cells using the CRISPR-Cas9 system. Science. (2014) 343:80–4. doi: 10.1126/science.1246981
100. Jiang F, Doudna JA. CRISPR-Cas9 structures and mechanisms. Annu Rev Biophys. (2017) 46:505–29. doi: 10.1146/annurev-biophys-062215-010822
101. Graf R, Li X, Chu VT, Rajewsky K. sgRNA sequence motifs blocking efficient CRISPR/Cas9-mediated gene editing. Cell Rep. (2019) 26:1098–103.e1093. doi: 10.1016/j.celrep.2019.01.024
102. Doench JG, Fusi N, Sullender M, Hegde M, Vaimberg EW, Donovan KF, et al. Optimized sgRNA design to maximize activity and minimize off-target effects of CRISPR-Cas9. Nat Biotechnol. (2016) 34:184–91. doi: 10.1038/nbt.3437
103. Gilbert LA, Horlbeck MA, Adamson B, Villalta JE, Chen Y, Whitehead EH, et al. Genome-scale CRISPR-mediated control of gene repression and activation. Cell. (2014) 159:647–61. doi: 10.1016/j.cell.2014.09.029
104. Alkan F, Wenzel A, Anthon C, Havgaard JH, Gorodkin J. CRISPR-Cas9 off-targeting assessment with nucleic acid duplex energy parameters. Genome Biol. (2018) 19:177. doi: 10.1186/s13059-018-1534-x
105. Hajiahmadi Z, Movahedi A, Wei H, Li D, Orooji Y, Ruan H, et al. Strategies to increase on-target and reduce off-target effects of the CRISPR/Cas9 system in plants. Int J Mol Sci. (2019) 20:3719. doi: 10.3390/ijms20153719
106. Tsai SQ, Nguyen NT, Malagon-Lopez J, Topkar VV, Aryee MJ, Joung JK. CIRCLE-seq: a highly sensitive in vitro screen for genome-wide CRISPR-Cas9 nuclease off-targets. Nat Methods. (2017) 14:607–14. doi: 10.1038/nmeth.4278
107. Tsai SQ, Zheng Z, Nguyen NT, Liebers M, Topkar VV, Thapar V, et al. GUIDE-seq enables genome-wide profiling of off-target cleavage by CRISPR-Cas nucleases. Nat Biotechnol. (2015) 33:187–97. doi: 10.1038/nbt.3117
108. Wienert B, Wyman SK, Richardson CD, Yeh CD, Akcakaya P, Porritt MJ, et al. Unbiased detection of CRISPR off-targets in vivo using DISCOVER-Seq. Science. (2019) 364:286–9. doi: 10.1101/469635
109. Kim D, Bae S, Park J, Kim E, Kim S, Yu HR, et al. Digenome-seq: genome-wide profiling of CRISPR-Cas9 off-target effects in human cells. Nat Methods. (2015) 12:237–43. doi: 10.1038/nmeth.3284
110. Ran FA, Cong L, Yan WX, Scott DA, Gootenberg JS, Kriz AJ, et al. In vivo genome editing using Staphylococcus aureus Cas9. Nature. (2015) 520:186–91. doi: 10.1038/nature14299
111. Frock RL, Hu J, Meyers RM, Ho YJ, Kii E, Alt FW. Genome-wide detection of DNA double-stranded breaks induced by engineered nucleases. Nat Biotechnol. (2015) 33:179–86. doi: 10.1038/nbt.3101
112. Akcakaya P, Bobbin ML, Guo JA, Malagon-Lopez J, Clement K, Garcia SP, et al. In vivo CRISPR editing with no detectable genome-wide off-target mutations. Nature. (2018) 561:416–9. doi: 10.1038/s41586-018-0500-9
113. Langmead B, Trapnell C, Pop M, Salzberg SL. Ultrafast and memory-efficient alignment of short DNA sequences to the human genome. Genome Biol. (2009) 10:R25. doi: 10.1186/gb-2009-10-3-r25
114. Prufer K, Stenzel U, Dannemann M, Green RE, Lachmann M, Kelso J. PatMaN: rapid alignment of short sequences to large databases. Bioinformatics. (2008) 24:1530–1. doi: 10.1093/bioinformatics/btn223
115. Li H, Durbin R. Fast and accurate short read alignment with burrows-wheeler transform. Bioinformatics. (2009) 25:1754–60. doi: 10.1093/bioinformatics/btp324
116. Hoffmann S, Otto C, Kurtz S, Sharma CM, Khaitovich P, Vogel J, et al. Fast mapping of short sequences with mismatches, insertions and deletions using index structures. PLoS Comput Biol. (2009) 5:e1000502. doi: 10.1371/journal.pcbi.1000502
117. Cameron P, Fuller CK, Donohoue PD, Jones BN, Thompson MS, Carter MM, et al. Mapping the genomic landscape of CRISPR-Cas9 cleavage. Nat Methods. (2017) 14:600–6. doi: 10.1038/nmeth.4284
118. Lin Y, Cradick TJ, Brown MT, Deshmukh H, Ranjan P, Sarode N, et al. CRISPR/Cas9 systems have off-target activity with insertions or deletions between target DNA and guide RNA sequences. Nucleic Acids Res. (2014) 42:7473–85. doi: 10.1093/nar/gku402
119. Cradick TJ, Qiu P, Lee CM, Fine EJ, Bao G. COSMID: a web-based tool for identifying and validating CRISPR/cas off-target sites. Mol Ther Nucleic Acids. (2014) 3:e214. doi: 10.1038/mtna.2014.64
120. Hsu PD, Scott DA, Weinstein JA, Ran FA, Konermann S, Agarwala V, et al. DNA targeting specificity of RNA-guided Cas9 nucleases. Nat Biotechnol. (2013) 31:827–32. doi: 10.1038/nbt.2647
121. Haeussler M, Schonig K, Eckert H, Eschstruth A, Mianne J, Renaud JB, et al. Evaluation of off-target and on-target scoring algorithms and integration into the guide RNA selection tool CRISPOR. Genome Biol. (2016) 17:148. doi: 10.1186/s13059-016-1012-2
122. Abadi S, Yan WX, Amar D, Mayrose I. A machine learning approach for predicting CRISPR-Cas9 cleavage efficiencies and patterns underlying its mechanism of action. PLoS Comput Biol. (2017) 13:e1005807. doi: 10.1371/journal.pcbi.1005807
123. Listgarten J, Weinstein M, Kleinstiver BP, Sousa AA, Joung JK, Crawford J, et al. Prediction of off-target activities for the end-to-end design of CRISPR guide RNAs. Nat Biomed Eng. (2018) 2:38–47. doi: 10.1038/s41551-017-0178-6
124. Lin J, Wong KC. Off-target predictions in CRISPR-Cas9 gene editing using deep learning. Bioinformatics. (2018) 34:i656–63. doi: 10.1093/bioinformatics/bty554
125. Uusi-Makela MIE, Barker HR, Bauerlein CA, Hakkinen T, Nykter M, Ramet M. Chromatin accessibility is associated with CRISPR-Cas9 efficiency in the zebrafish (Danio rerio). PLoS ONE. (2018) 13:e0196238. doi: 10.1371/journal.pone.0196238
126. Choi GCG, Zhou P, Yuen CTL, Chan BKC, Xu F, Bao S, et al. Combinatorial mutagenesis en masse optimizes the genome editing activities of SpCas9. Nat Methods. (2019) 16:722–30. doi: 10.1038/s41592-019-0473-0
127. Canver MC, Lessard S, Pinello L, Wu Y, Ilboudo Y, Stern EN, et al. Variant-aware saturating mutagenesis using multiple Cas9 nucleases identifies regulatory elements at trait-associated loci. Nat Genet. (2017) 49:625–34. doi: 10.1038/ng.3793
128. Lessard S, Francioli L, Alfoldi J, Tardif JC, Ellinor PT, Macarthur DG, et al. Human genetic variation alters CRISPR-Cas9 on- and off-targeting specificity at therapeutically implicated loci. Proc Natl Acad Sci USA. (2017) 114:E11257–66. doi: 10.1073/pnas.1714640114
129. Liu G, Yin K, Zhang Q, Gao C, Qiu JL. Modulating chromatin accessibility by transactivation and targeting proximal dsgRNAs enhances Cas9 editing efficiency in vivo. Genome Biol. (2019) 20:145. doi: 10.1186/s13059-019-1762-8
130. Shang W, Wang F, Fan G, Wang H. Key elements for designing and performing a CRISPR/Cas9-based genetic screen. J Genet Genomics. (2017) 44:439–49. doi: 10.1016/j.jgg.2017.09.005
131. Ford K, Mcdonald D, Mali P. Functional genomics via CRISPR-Cas. J Mol Biol. (2019) 431:48–65. doi: 10.1016/j.jmb.2018.06.034
132. Robinson MD, Mccarthy DJ, Smyth GK. edgeR: a bioconductor package for differential expression analysis of digital gene expression data. Bioinformatics. (2010) 26:139–40. doi: 10.1093/bioinformatics/btp616
133. Hardcastle TJ, Kelly KA. baySeq: empirical bayesian methods for identifying differential expression in sequence count data. BMC Bioinformatics. (2010) 11:422. doi: 10.1186/1471-2105-11-422
134. Love MI, Huber W, Anders S. Moderated estimation of fold change and dispersion for RNA-seq data with DESeq2. Genome Biol. (2014) 15:550. doi: 10.1186/s13059-014-0550-8
135. Luo B, Cheung HW, Subramanian A, Sharifnia T, Okamoto M, Yang X, et al. Highly parallel identification of essential genes in cancer cells. Proc Natl Acad Sci USA. (2008) 105:20380–5. doi: 10.1073/pnas.0810485105
136. Konig R, Chiang CY, Tu BP, Yan SF, Dejesus PD, Romero A, et al. A probability-based approach for the analysis of large-scale RNAi screens. Nat Methods. (2007) 4:847–9. doi: 10.1038/nmeth1089
137. Wang B, Wang M, Zhang W, Xiao T, Chen CH, Wu A, et al. Integrative analysis of pooled CRISPR genetic screens using MAGeCKFlute. Nat Protoc. (2019) 14:756–80. doi: 10.1038/s41596-018-0113-7
138. Diaz AA, Qin H, Ramalho-Santos M, Song JS. HiTSelect: a comprehensive tool for high-complexity-pooled screen analysis. Nucleic Acids Res. (2015) 43:e16. doi: 10.1093/nar/gku1197
139. Yu J, Silva J, Califano A. ScreenBEAM: a novel meta-analysis algorithm for functional genomics screens via bayesian hierarchical modeling. Bioinformatics. (2016) 32:260–7. doi: 10.1093/bioinformatics/btv556
140. Hart T, Moffat J. BAGEL: a computational framework for identifying essential genes from pooled library screens. BMC Bioinformatics. (2016) 17:164. doi: 10.1186/s12859-016-1015-8
141. Trumbach D, Pfeiffer S, Poppe M, Scherb H, Doll S, Wurst W, et al. ENCoRE: an efficient software for CRISPR screens identifies new players in extrinsic apoptosis. BMC Genomics. (2017) 18:905. doi: 10.1186/s12864-017-4285-2
142. Jia G, Wang X, Xiao G. A permutation-based non-parametric analysis of CRISPR screen data. BMC Genomics. (2017) 18:545. doi: 10.1186/s12864-017-3938-5
143. Allen F, Behan F, Khodak A, Iorio F, Yusa K, Garnett M, et al. JACKS: joint analysis of CRISPR/Cas9 knockout screens. Genome Res. (2019) 29:464–71. doi: 10.1101/gr.238923.118
144. Tsherniak A, Vazquez F, Montgomery PG, Weir BA, Kryukov G, Cowley GS, et al. Defining a cancer dependency map. Cell. (2017) 170:564–76.e516. doi: 10.1016/j.cell.2017.06.010
145. Daley TP, Lin Z, Lin X, Liu Y, Wong WH, Qi LS. CRISPhieRmix: a hierarchical mixture model for CRISPR pooled screens. Genome Biol. (2018) 19:159. doi: 10.1186/s13059-018-1538-6
146. Jeong HH, Kim SY, Rousseaux MWC, Zoghbi HY, Liu Z. Beta-binomial modeling of CRISPR pooled screen data identifies target genes with greater sensitivity and fewer false negatives. Genome Res. (2019) 29:999–1008. doi: 10.1101/gr.245571.118
147. Li W, Xu H, Xiao T, Cong L, Love MI, Zhang F, et al. MAGeCK enables robust identification of essential genes from genome-scale CRISPR/Cas9 knockout screens. Genome Biol. (2014) 15:554. doi: 10.1186/s13059-014-0554-4
148. Yang L, Zhu Y, Yu H, Cheng X, Chen S, Chu Y, et al. scMAGeCK links genotypes with multiple phenotypes in single-cell CRISPR screens. Genome Biol. (2020) 21:19. doi: 10.1186/s13059-020-1928-4
149. Li W, Koster J, Xu H, Chen CH, Xiao T, Liu JS, et al. Quality control, modeling, and visualization of CRISPR screens with MAGeCK-VISPR. Genome Biol. (2015) 16:281. doi: 10.1186/s13059-015-0843-6
150. Chen CH, Xiao T, Xu H, Jiang P, Meyer CA, Li W, et al. Improved design and analysis of CRISPR knockout screens. Bioinformatics. (2018) 34:4095–101. doi: 10.1093/bioinformatics/bty450
151. Chen W, Zhang G, Li J, Zhang X, Huang S, Xiang S, et al. CRISPRlnc: a manually curated database of validated sgRNAs for lncRNAs. Nucleic Acids Res. (2019) 47:D63–8. doi: 10.1093/nar/gky904
152. Szlachta K, Kuscu C, Tufan T, Adair SJ, Shang S, Michaels AD, et al. CRISPR knockout screening identifies combinatorial drug targets in pancreatic cancer and models cellular drug response. Nat Commun. (2018) 9:4275. doi: 10.1038/s41467-018-06676-2
153. Arroyo JD, Jourdain AA, Calvo SE, Ballarano CA, Doench JG, Root DE, et al. A genome-wide CRISPR death screen identifies genes essential for oxidative phosphorylation. Cell Metab. (2016) 24:875–85. doi: 10.1016/j.cmet.2016.08.017
154. Ghezraoui H, Piganeau M, Renouf B, Renaud JB, Sallmyr A, Ruis B, et al. Chromosomal translocations in human cells are generated by canonical nonhomologous end-joining. Mol Cell. (2014) 55:829–42. doi: 10.1016/j.molcel.2014.08.002
155. Ye L, Wang C, Hong L, Sun N, Chen D, Chen S, et al. Programmable DNA repair with CRISPRa/i enhanced homology-directed repair efficiency with a single Cas9. Cell Discov. (2018) 4:46. doi: 10.1038/s41421-018-0049-7
156. Sakuma T, Nakade S, Sakane Y, Suzuki KT, Yamamoto T. MMEJ-assisted gene knock-in using TALENs and CRISPR-Cas9 with the PITCh systems. Nat Protoc. (2016) 11:118–33. doi: 10.1038/nprot.2015.140
157. Shen MW, Arbab M, Hsu JY, Worstell D, Culbertson SJ, Krabbe O, et al. Predictable and precise template-free CRISPR editing of pathogenic variants. Nature. (2018) 563:646–51. doi: 10.1038/s41586-018-0686-x
158. Allen F, Crepaldi L, Alsinet C, Strong AJ, Kleshchevnikov V, De Angeli P, et al. Predicting the mutations generated by repair of Cas9-induced double-strand breaks. Nat Biotechnol. (2018) 37:64–72. doi: 10.1038/nbt.4317
159. Chen W, Mckenna A, Schreiber J, Haeussler M, Yin Y, Agarwal V, et al. Massively parallel profiling and predictive modeling of the outcomes of CRISPR/Cas9-mediated double-strand break repair. Nucleic Acids Res. (2019) 47:7989–8003. doi: 10.1093/nar/gkz487
160. Connelly JP, Pruett-Miller SM. CRIS.py: a versatile and high-throughput analysis program for CRISPR-based genome editing. Sci Rep. (2019) 9:4194. doi: 10.1038/s41598-019-40896-w
161. Wang X, Tilford C, Neuhaus I, Mintier G, Guo Q, Feder JN, et al. CRISPR-DAV: CRISPR NGS data analysis and visualization pipeline. Bioinformatics. (2017) 33:3811–2. doi: 10.1093/bioinformatics/btx518
162. Guell M, Yang L, Church GM. Genome editing assessment using CRISPR genome analyzer (CRISPR-GA). Bioinformatics. (2014) 30:2968–70. doi: 10.1093/bioinformatics/btu427
163. Lindsay H, Burger A, Biyong B, Felker A, Hess C, Zaugg J, et al. CrispRVariants charts the mutation spectrum of genome engineering experiments. Nat Biotechnol. (2016) 34:701–2. doi: 10.1038/nbt.3628
164. Varshney GK, Pei W, Lafave MC, Idol J, Xu L, Gallardo V, et al. High-throughput gene targeting and phenotyping in zebrafish using CRISPR/Cas9. Genome Res. (2015) 25:1030–42. doi: 10.1101/gr.186379.114
165. Clement K, Rees H, Canver MC, Gehrke JM, Farouni R, Hsu JY, et al. CRISPResso2 provides accurate and rapid genome editing sequence analysis. Nat Biotechnol. (2019) 37:224–6. doi: 10.1038/s41587-019-0032-3
166. Boel A, Steyaert W, De Rocker N, Menten B, Callewaert B, De Paepe A, et al. BATCH-GE: batch analysis of next-generation sequencing data for genome editing assessment. Sci Rep. (2016) 6:30330. doi: 10.1038/srep30330
167. Komor AC, Kim YB, Packer MS, Zuris JA, Liu DR. Programmable editing of a target base in genomic DNA without double-stranded DNA cleavage. Nature. (2016) 533:420–4. doi: 10.1038/nature17946
168. Rees HA, Liu DR. Base editing: precision chemistry on the genome and transcriptome of living cells. Nat Rev Genet. (2018) 19:770–88. doi: 10.1038/s41576-018-0059-1
169. Park J, Lim K, Kim JS, Bae S. Cas-analyzer: an online tool for assessing genome editing results using NGS data. Bioinformatics. (2017) 33:286–8. doi: 10.1093/bioinformatics/btw561
170. You Q, Zhong Z, Ren Q, Hassan F, Zhang Y, Zhang T. CRISPRMatch: an automatic calculation and visualization tool for high-throughput CRISPR genome-editing data analysis. Int J Biol Sci. (2018) 14:858–62. doi: 10.7150/ijbs.24581
171. Varshney GK, Zhang S, Pei W, Adomako-Ankomah A, Fohtung J, Schaffer K, et al. CRISPRz: a database of zebrafish validated sgRNAs. Nucleic Acids Res. (2016) 44:D822–6. doi: 10.1093/nar/gkv998
172. Kaur K, Tandon H, Gupta AK, Kumar M. CrisprGE: a central hub of CRISPR/Cas-based genome editing. Database. (2015) 2015:bav055. doi: 10.1093/database/bav055
173. Rauscher B, Heigwer F, Breinig M, Winter J, Boutros M. GenomeCRISPR - a database for high-throughput CRISPR/Cas9 screens. Nucleic Acids Res. (2017) 45:D679–86. doi: 10.1093/nar/gkw997
174. Lenoir WF, Lim TL, Hart T. PICKLES: the database of pooled in-vitro CRISPR knockout library essentiality screens. Nucleic Acids Res. (2018) 46:D776–80. doi: 10.1093/nar/gkx993
175. Behan FM, Iorio F, Picco G, Goncalves E, Beaver CM, Migliardi G, et al. Prioritization of cancer therapeutic targets using CRISPR-Cas9 screens. Nature. (2019) 568:511–6. doi: 10.1038/s41586-019-1103-9
176. Oughtred R, Stark C, Breitkreutz BJ, Rust J, Boucher L, Chang C, et al. The BioGRID interaction database: 2019 update. Nucleic Acids Res. (2019) 47:D529–41. doi: 10.1093/nar/gky1079
177. Dong C, Hao GF, Hua HL, Liu S, Labena AA, Chai G, et al. Anti-CRISPRdb: a comprehensive online resource for anti-CRISPR proteins. Nucleic Acids Res. (2018) 46:D393–8. doi: 10.1093/nar/gkx835
178. Zhang F, Zhao S, Ren C, Zhu Y, Zhou H, Lai Y, et al. CRISPRminer is a knowledge base for exploring CRISPR-Cas systems in microbe and phage interactions. Commun Biol. (2018) 1:180. doi: 10.1038/s42003-018-0184-6
179. Shin J, Jiang F, Liu JJ, Bray NL, Rauch BJ, Baik SH, et al. Disabling Cas9 by an anti-CRISPR DNA mimic. Sci Adv. (2017) 3:e1701620. doi: 10.1126/sciadv.1701620
180. Anzalone AV, Randolph PB, Davis JR, Sousa AA, Koblan LW, Levy JM, et al. Search-and-replace genome editing without double-strand breaks or donor DNA. Nature. (2019) 576:149–57. doi: 10.1038/s41586-019-1711-4
Keywords: CRISPR/Cas system, In silico methods, CRISPR/Cas system identification, guide RNA design, post-experimental assistance
Citation: Zhang Y, Zhao G, Ahmed FYH, Yi T, Hu S, Cai T and Liao Q (2020) In silico Method in CRISPR/Cas System: An Expedite and Powerful Booster. Front. Oncol. 10:584404. doi: 10.3389/fonc.2020.584404
Received: 17 July 2020; Accepted: 24 August 2020;
Published: 02 October 2020.
Edited by:
Meng Zhou, Wenzhou Medical University, ChinaReviewed by:
Feizhen Wu, Fudan University, ChinaWei Li, Children's National Hospital, United States
Copyright © 2020 Zhang, Zhao, Ahmed, Yi, Hu, Cai and Liao. This is an open-access article distributed under the terms of the Creative Commons Attribution License (CC BY). The use, distribution or reproduction in other forums is permitted, provided the original author(s) and the copyright owner(s) are credited and that the original publication in this journal is cited, in accordance with accepted academic practice. No use, distribution or reproduction is permitted which does not comply with these terms.
*Correspondence: Ting Cai, Y2FpdGluZyYjeDAwMDQwO3VjYXMuYWMuY24=; Qi Liao, bGlhb3FpJiN4MDAwNDA7bmJ1LmVkdS5jbg==