- Department of Ultrasound, Chinese Academy of Medical Sciences and Peking Union Medical College Hospital, Beijing, China
Objective: To develop a nomogram for predicting axillary lymph node (ALN) metastases using the breast imaging reporting and data system (BI-RADS) ultrasound lexicon.
Methods: A total of 703 patients from July 2015 to January 2018 were included in this study as a primary cohort for model construction. Moreover, 109 patients including 51 pathologically confirmed N1 patients (TNM staging) and 58 non-metastatic patients were recruited as an external validation cohort from March 2018 to August 2019. Ultrasound images and clinical information of these patients were retrospectively reviewed. The ultrasonic features based on the BI-RADS lexicon were extracted by two radiologists. The features extracted from the primary cohort were used to develop a nomogram using multivariate analysis. Internal and external validations were performed to evaluate the predictive efficacy of the nomogram.
Results: The nomogram was based on two features (size, lesion boundary) and showed an area under the curve of 0.75 (95% confidence interval [CI], 0.70–0.79) in the primary cohort and 0.91 (95% CI, 0.84–0.97) in the external validation cohort; it achieved an 88% sensitivity in N1 patients.
Conclusion: The nomogram based on BI-RADS ultrasonic features can predict breast cancer ALN status with relatively high accuracy. It has potential clinical value in improving the sensitivity and accuracy of the preoperative diagnosis of ALN metastases, especially for N1 patients.
Introduction
Breast cancer, posing a serious threat to women’s health and social economy, has drawn great attention from researchers for years (1). Axillary lymph node (ALN) status plays an essential role in treatment planning for breast cancer (2), being the most significant prognostic indicator for early stage patients (3). Preoperative staging of ALN status can make a way for optimized clinical decision making. While, currently recognized method for identifying ALN status is sentinel lymph node biopsy (SLNB), which is performed during surgery and requires pathological diagnosis. The SLNB-negative patients would be diagnosed as pN0 in TNM staging (4, 5).
In current clinical practice, axillary ultrasound (US) is commonly recommended for all patients with breast cancer to evaluate ALN status preoperatively (6, 7). However, the SLN cannot be identified by grayscale US, and metastases of isolated tumor cells or micro-metastases are not visible on US. As a consequence, it is difficult for conventional US to achieve high accuracy in identifying axillary nodal metastases. It was reported that US has a sensitivity of 45% to 87% in diagnosing ALN metastases and specificity of 55% to 97% (8). Zhang et al. proved that among N1-3 patients, axillary US had the highest false-negative rate in pathologic N1 patients (9). Hence, it is crucial to improve the preoperative diagnostic accuracy of US in identifying ALN metastases, especially for patients with a minimal number of abnormal nodes.
Previous studies have demonstrated that some ultrasonic features of breast lesions, such as tumor size, margin, and location might be associated with breast cancer nodal metastases and thus can help predict ALN status (10–13). However, in those studies, US findings and tumor clinicopathologic characteristics were simultaneously incorporated to predict ALN metastases (11–13), or a risk model was developed for predicting ALN metastases in a subgroup of patients with invasive ductal carcinoma (10, 11, 13). Considering that the clinicopathologic characteristics, such as histological type, histological grade, and molecular subtype, might directly be related to the probability of ALN metastases, it is necessary to explore the independent contributions of breast lesion US features in determining the likelihood of positive lymph nodes in a preoperative patient population. Therefore, we aimed to construct a predictive model for ALN metastases based on breast lesion US features, to investigate the feasibility of using only US features in identifying nodal metastases preoperatively.
In this study, we summarize the ultrasonic features of the malignant lesions using the breast imaging reporting and data system (BI-RADS) lexicon, the widely accepted standard for defining ultrasonic feature of breast lesions (14). We analyzed the correlations of these ultrasonic features with nodal metastases, developed an ALN metastases predictive model based on these features, and presented it as a nomogram. Such a tool is expected to improve preoperative diagnostic efficacy, especially for N1 patients.
Materials and Methods
This study is retrospective and was approved by the Institutional Review Board of Perking Union Medical College Hospital.
Patient Recruitment
A total of 1,024 female patients with breast cancer were enrolled consecutively for model construction and internal validation from July 2015 to January 2018. The clinical data, US images, and pathological results were reviewed. The inclusion and exclusion criteria for establishing the primary and internal validation cohorts were as follows.
Inclusion criteria:
(1) patients pathologically diagnosed as having breast cancer;
(2) ALN status clearly illustrated by pathology after SLNB or ALN dissection (ALND);
(3) breast US scanning performed within one month before surgery;
(4) only a single lesion pathologically identified in each patient, with a diameter less than 5 cm (T1 and T2 stage).
Exclusion criteria:
(1) neoadjuvant chemotherapy or biopsy performed before US scanning;
(2) multiple malignant lesions;
(3) target neoplasms that could not be visualized on US;
(4) incomplete clinical and pathological information.
Finally, a total of 703 consecutive patients were included in this study for model construction and internal validation from July 2015 to January 2018. Then, to validate the efficacy of the prediction model in early breast cancer patients, based on the inclusion and exclusion criteria described above, another 109 patients with pN1/pN0 were recruited at 1:1 ratio as the external validation cohort after primary cohort (From March 2018 to August 2019). Including 51 patients classified as having N1 according to the TNM classification (with one to three metastatic ALN nodes) by postoperative pathology and 58 patients with no ALN metastases (15).
Clinical and Pathological Information Collection
The clinical and pathological features of the patients, including age, pathological results, and ALN status (LN-positive or LN-negative), were extracted from the medical records.
Ultrasound Scanning and Imaging Acquisition
All the included patients underwent US scanning before surgery in our Department. Our study did not specify US equipment. The high-quality US images are acquired by four different commercial US devices, which are RS85A (Samsung), IU22 (Philips), Logic 9 (GE) and RS85A (Samsung) with Linear probes (3–12 MHz, centered at 10 MHz). And do not affect the handcrafted extraction of BI-RADS features. The recorded imaging data of the patients were carefully reviewed and selected for further analysis by one experienced radiologist (QZ, 23-year experience in breast US), blinded to the clinical and pathological results. The grayscale and color-Doppler ultrasonic images of both longitudinal section and cross-section were acquired for feature extraction. The largest diameter of each lesion was measured on the grayscale US images.
BI-RADS-Based US Feature Extraction
Referring to the BI-RADS lexicon and previous researches (16–18), a total of eight ultrasonic features were selected in this study as evaluation indices (Table 1). Image reading and feature extraction were conducted by the two radiologists (CZ, 4-year experience in breast US, and YL, 2-year experience in breast US), who were also blinded to the patient’s clinical and pathological information. As discrepancies occurred, the agreement would be reached through discussion. Before participating in the study, the two radiologists received systematic training on the BI-RADS lexicon. Inter-observer reliability was assessed by comparing the results of the 2 radiologists in 100 randomly chosen lesions. CZ performed the second feature extraction from 100 randomly selected lesions after 1 week with the same procedure. Then by comparing the results of CZ at two different time points evaluated intra-observer reliability. Finally the inter-observer and intra-observer agreement were measured by kappa statistics.
Model Construction and Validation
The prediction model was built based on multivariate logistic regression analysis. Before construction, multicollinearity analysis was performed by calculating the variance inflation factor (VIF) among the features; a VIF value > 10 was considered to indicate multicollinearity, and the corresponding variables were excluded from the model. All the US features were modeled as categorical data with a dummy variable, adding age as continuous variables, to construct models. In multivariate models, a backward stepwise variable selection procedure was used for model selection based on the Akaike information criterion (AIC). The final model thus built was tested for predictive power using both internal and external validation. Internal validation was performed with the bootstrap resampling method by randomly drawing 500 samples from the primary dataset to avoid overoptimism. The developed model underlying the nomogram was used to predict ALN status of the patients in the external validation cohort. The diagnostic performance of the model in the primary and validation cohorts was evaluated by calculating sensitivity, specificity, positive likelihood ratio, negative likelihood ratio, positive predictive value, and negative predictive value. Receiver operating curves (ROC) and the corresponding area under the curve (AUC) values were used to assess the discriminating ability of the nomogram.
Statistical Analysis
Statistical analysis was performed using R (http://www.R-project.org) and EmpowerStats software (X&Y Solutions). The variables were compared using Student’s t-test (continuous data) and the Pearson chi-squared test (categorical data). Continuous variables are expressed as the mean ± SD, categorical variables as percentages (%), and p values < 0.05 were considered statistically significant. The degree of intra-observer and inter-observer agreement between the two readers was measured using the κ value, which was interpreted as follows: κ < 0, poor agreement; 0 < κ < 0.20, slight agreement; 0.20 < κ < 0.40, fair agreement; 0.40 < κ < 0.60, moderate agreement; 0.60 < κ < 0.80, substantial agreement; and 0.80 < κ < 1, perfect agreement. The “glm” function was used for the univariate and multivariate logistic regression analyses. The “Hmisc” package was used to plot the nomogram. The “pROC” package was used to plot the ROC curves and measure the AUCs. The “calibration curve” function was used to plot the calibration curves.
Results
Clinical Characteristics and Ultrasonic Features of the Primary and External Validation Cohorts
Table 2 shows the baseline clinical characteristics and ultrasonic features of the 703 patients in the primary cohort and 109 patients in the external validation cohort. A total of 167 (23.9%) patients with ALN metastases were included in the primary cohort and 51 patients (46.8%) with ALN metastases in the external validation cohort.
The inter-operator agreements for the ultrasonic features ranged from 0.77 to 0.92 (shape: 0.87; orientation: 0.92; margin: 0.91; lesion boundary: 0.77; echo pattern: 0.92; posterior acoustic features: 0.90; calcification: 0.78; vascularity: 0.81). The intra-operator agreements for the ultrasonic features ranged from 0.79 to 0.96 (shape: 0.91; orientation: 0.94; margin: 0.89; lesion boundary: 0.82; echo pattern: 0.96; posterior acoustic features: 0.91; calcification: 0.85; vascularity: 0.90).
Diagnostic Performance of the Nomogram
Using multivariate logistic regression analysis, several multivariate models were generated. And after stepwise model selection, two features showed independent correlation with the risk of ALN metastases (Table 3) and thus were incorporated into the final nomogram, namely, size and lesion boundary. The nomogram is presented in Figure 1.
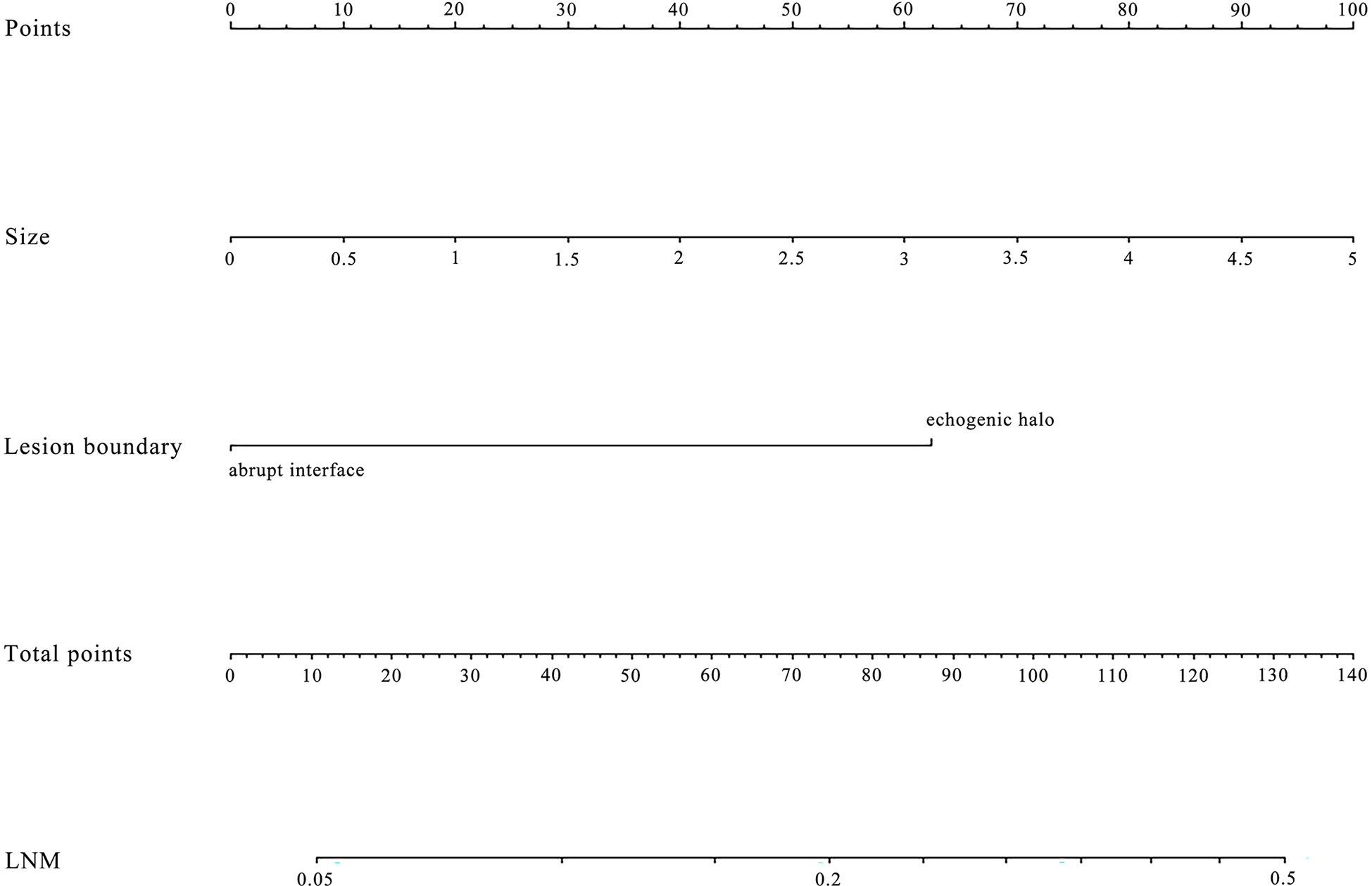
Figure 1 The nomogram was developed in the primary dataset. it included two factors (size, lesion boundary). The nomogram plot provides a visual way to predict the risk of LN metastases for breast cancer patients.
The diagnostic performance of the nomogram in the primary dataset is shown in Table 4. The ROC curve of the nomogram showed good predictive power, with an AUC of 0.75 [95% confidence interval (CI), 0.70–0.79] (Figure 2).
Good calibration was observed for the probability of ALN metastases in the primary cohort (Figure 3).
Nomogram Validation in N1 Patients
An external validation cohort of 109 patients was enrolled using the same criteria used to select the primary cohort and included 51 patients (46.8%) with ALN metastases (the mean number of metastatic ALN nodes was 1.57). The nomogram demonstrated good predictive power (Table 4) with an AUC of 0.91 (95% CI: 0.84–0.97) in these N1 patients (Figure 4).
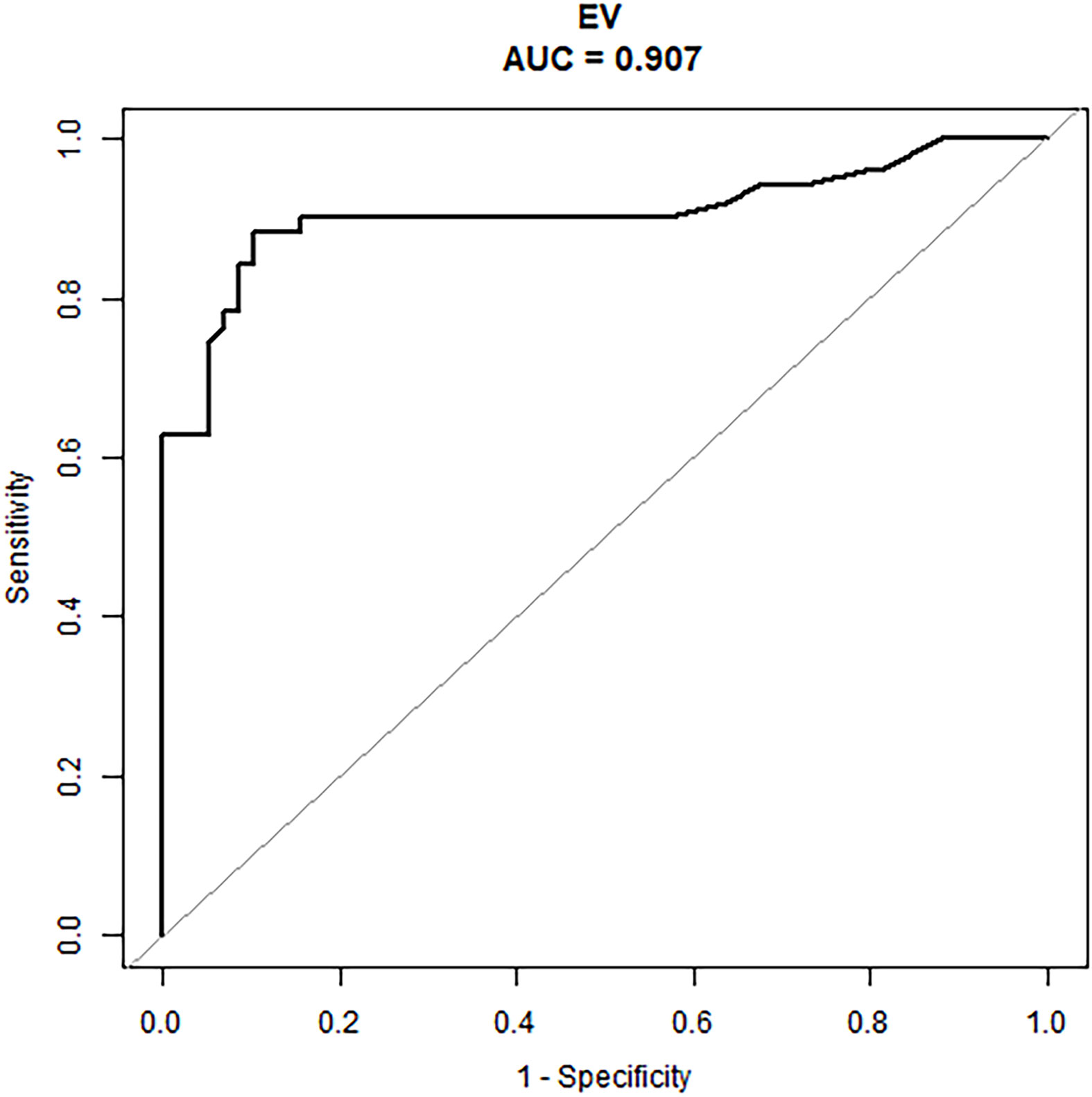
Figure 4 The ROC curve of the prediction model in the N1 patients of the external validation cohort.
Discussion
Axillary imaging plays an essential role in evaluating ALN status. Axillary US is the primary method for evaluation of axillary nodes, especially in the evaluation of early ALN metastasis. Breast MRI can better demonstrate lymph node metastasis on higher stations (19). However, the use of axillary US in evaluating ALN has been limited by its moderate accuracy and considerable discrepancy among the studies. Some studies have shown that malignant lymph nodes detected by US had a higher node burden than those detected by SLNB, implying a disparity between “ultrasound positive” and “SLNB positive” (20, 21). Moreover, according to previous studies, axillary US tends to perform poorly in identifying metastases in pathologic N1 patients, characterized by one to three abnormal nodes (9). Therefore, to improve the US diagnostic performance for ALN metastases, it is important to improve its accuracy and lower its false-negative rate in N1 patients.
In our study, we developed a prediction model based on BI-RADS ultrasonic features to predict the risk of LN metastases, achieving an accuracy of 65.0% in the primary cohort,and 89.0% in the external validation cohort. A nomogram, incorporating two factors among the lesion US features, showed significant discriminating ability in the primary cohort, and also showed high predictive power in an external validation cohort of early-stage breast cancer patients.
Recent studies have investigated the potential value of ultrasonic images of breast lesions in predicting nodal metastases, with reported AUCs ranging from 0.731 to 0.848 (22–25). Some of these studies showed that US features of breast lesion and axillary lymph nodes are correlated with ALN status (22), and in some studies, high-throughput features of ultrasonic images were proved useful for the prediction of ALN metastases (24, 25). Taken together, these results demonstrate that ultrasonic images of breast lesions can potentially be useful in the preoperative diagnosis of ALN metastases. Considering the nonspecific ultrasonic presentations of metastatic ALNs and the disparity in positive rates between US and SLNB, the images of breast lesions are worth exploring, as they might contain helpful information for the prediction of nodal metastases.
In 2003, a standard protocol for breast US was established in the BI-RADS lexicon and received worldwide recognition (18). The definition and description of the ultrasonic features, the lesion classification, and the reporting system were all clearly defined and illustrated in the lexicon, allowing reliable feature identification. Previous studies have validated clinical-pathological factors and US BI-RADS features of masses could predict breast cancer LN metastasis. Zong et al. (26) suggest that US features of breast mass, like margin, microcalcification, and blood flow signals are significantly correlated with ALN metastasis in early breast cancer. Besides, Guo et al. (12) have proven that irregular shape and high color Doppler flow imaging grades are independent impact factors of ALN metastasis. However, both of them incorporated some clinical-pathological factors simultaneously, like immunohistochemical analysis (ER, PR, Ki-67, and so on) and the histologic grade, which are also highly associated with ALN status. To figure out the independent contributions of breast lesion US features in determining the likelihood of ALN metastasis preoperatively, and to develop a simple and practical nomogram based on US features, we adopted the ultrasonic features defined by the BI-RADS lexicon in 2013 to construct our models (17). A total of eight features were included for modeling, which has been commonly used in differentiating benign and malignant breast lesions. Our results show that some features are also related to ALN status. As shown by the nomogram, tumor size and lesion boundary had more significant impacts on total scores than other features. The prediction model displayed a remarkable ability to predict ALN status, especially in N1 patients, yielding an AUC of 0.901. More importantly, it achieved 88% sensitivity for N1 patients, compared with that in previous studies, which presented false-negative rates as high as 46.2% (9). These results indicate the potential value of our model in increasing sensitivity in the identification of abnormal lymph nodes, as well as in decreasing the rate of preoperatively missed diagnoses, thus bringing benefits to early-stage breast cancer patients.
To note, US readers can predict the probability of ALN metastases associated with the lesion using this nomogram, after routinely extracting the standardized features from the breast lesion ultrasonic images. Apart from its high accuracy, compared with some complex models using additional image processing software, the prediction process used by this model is simple and time-saving. We hope that this model will be widely used in clinical practice as a supplementary to conventional breast US, allowing improved accuracy of preoperative diagnosis of nodal metastases.
Our predictive model has several limitations. First, the sample size of the external cohort was relatively small, and increasing the sample size would be necessary to obtain more convincing results. Moreover, the single-center design of the study might lead to an un recognized bias in patient recruitment, imaging acquisition, and image analysis. Adding data from other medical centers would be helpful in further improving the clinical efficacy of the model.
In this study, a nomogram based on ultrasonic features of breast lesions was developed to predict the risk of ALN metastases in breast cancer patients. The model demonstrated clinical potential in providing a non-invasive, effective, and easy-to-use approach to identify ALN metastases preoperatively, which might aid in clinical decision making.
Data Availability Statement
The raw data supporting the conclusions of this article will be made available by the authors, without undue reservation.
Ethics Statement
This retrospective study was approved by the Institutional Review Board of Peking Union Medical College Hospital.
Author Contributions
YJ and QZ conceived and designed the study. WL, JZ, LM, and MX collected the clinical and image data. YL and JQ performed image pre-processing. CZ and YG analyzed the image data and performed the statistical analysis. YL and CZ wrote the manuscript. All authors contributed to the article and approved the submitted version.
Funding
This work was supported by CAMS Innovation Fund for Medical Sciences (2017-I2M-1-006).
Conflict of Interest
The authors declare that the research was conducted in the absence of any commercial or financial relationships that could be construed as a potential conflict of interest.
References
1. Bray F, Ferlay J, Soerjomataram I, Siegel RL, Torre LA, Jemal A. Global cancer statistics 2018: GLOBOCAN estimates of incidence and mortality worldwide for 36 cancers in 185 countries. CA: Cancer J Clin (2018) 68(6):394–424. doi: 10.3322/caac.21492
2. Rao R, Euhus D, Mayo HG, Balch C. Axillary node interventions in breast cancer: a systematic review. JAMA (2013) 310(13):1385–94. doi: 10.1001/jama.2013.277804
3. Cianfrocca M, Goldstein LJ. Prognostic and predictive factors in early-stage breast cancer. Oncol (2004) 9(6):606–16. doi: 10.1634/theoncologist.9-6-606
4. Janni W, Kuhn T, Schwentner L, Kreienberg R, Fehm T, Wockel A. Sentinel node biopsy and axillary dissection in breast cancer: the evidence and its limits. Deutsches Arzteblatt Int (2014) 111(14):244–9. doi: 10.3238/arztebl.2014.0244
5. Lyman GH, Giuliano AE, Somerfield MR, Benson 3rd AB, Bodurka DC, Burstein HJ, et al. American Society of Clinical Oncology guideline recommendations for sentinel lymph node biopsy in early-stage breast cancer. J Clin Oncol (2005) 23(30):7703–20. doi: 10.1200/jco.2005.08.001
6. Surgery tAoB. Consensus statement on management of the malignant axilla in early breast cancer. (2015).
7. Ecanow JS, Abe H, Newstead GM, Ecanow DB, Jeske JM. Axillary staging of breast cancer: what the radiologist should know. Radiographics (2013) 33(6):1589–612. doi: 10.1148/rg.336125060
8. Alvarez S, Anorbe E, Alcorta P, Lopez F, Alonso I, Cortes J. Role of sonography in the diagnosis of axillary lymph node metastases in breast cancer: a systematic review. AJR Am J Roentgenol (2006) 186(5):1342–8. doi: 10.2214/ajr.05.0936
9. Zhang YN, Wang CJ, Xu Y, Zhu QL, Zhou YD, Zhang J, et al. Sensitivity, Specificity and Accuracy of Ultrasound in Diagnosis of Breast Cancer Metastasis to the Axillary Lymph Nodes in Chinese Patients. Ultrasound Med Biol (2015) 41(7):1835–41. doi: 10.1016/j.ultrasmedbio.2015.03.024
10. Li XL, Xu HX, Li DD, He YP, Yue WW, Xu JM, et al. A Risk Model based on Ultrasound, Ultrasound Elastography, and Histologic Parameters for Predicting Axillary Lymph Node Metastasis in Breast Invasive Ductal Carcinoma. Sci Rep (2017) 7(1):3029. doi: 10.1038/s41598-017-03582-3
11. Yun SJ, Sohn YM, Seo M. Risk Stratification For Axillary Lymph Node Metastases in Breast Cancer Patients: What Clinicopathological and Radiological Factors of Primary Breast Cancer Can Predict Preoperatively Axillary Lymph Node Metastases? Ultrasound Q (2017) 33(1):15–22. doi: 10.1097/ruq.0000000000000249
12. Guo Q, Dong Z, Zhang L, Ning C, Li Z, Wang D, et al. Ultrasound Features of Breast Cancer for Predicting Axillary Lymph Node Metastasis. J Ultrasound Med (2018) 37(6):1354–3. doi: 10.1002/jum.14469
13. Tran HT, Pack D, Mylander C, Martino L, Rosman M, Tafra L, et al. Ultrasound-Based Nomogram Identifies Breast Cancer Patients Unlikely to Harbor Axillary Metastasis: Towards Selective Omission of Sentinel Lymph Node Biopsy. Ann Surg Oncol (2020) 27(8):2679–86. doi: 10.1245/s10434-019-08164-3
14. Bromham N, Schmidt-Hansen M, Astin M, Hasler E, Reed MW. Axillary treatment for operable primary breast cancer. Cochrane Database Syst Rev (2017) 1:Cd004561. doi: 10.1002/14651858.CD004561.pub3
15. Moons KG, Altman DG, Reitsma JB, Ioannidis JP, Macaskill P, Steyerberg EW, et al. Transparent Reporting of a multivariable prediction model for Individual Prognosis or Diagnosis (TRIPOD): explanation and elaboration. Ann Intern Med (2015) 162(1):W1–73. doi: 10.7326/m14-0698
16. Yu X, Hao X, Wan J, Wang Y, Yu L, Liu B. Correlation between Ultrasound Appearance of Small Breast Cancer and Axillary Lymph Node Metastasis. Ultrasound Med Biol (2018) 44(2):342–9. doi: 10.1016/j.ultrasmedbio.2017.09.020
17. Radiology ACo. Breast Imaging Reporting and Data System® (BI-RADS®) 5. Reston: American College of Radiology (2013).
18. Radiology ACo. Breast Imaging Reporting and Data System® (BI-RADS®) 4. Reston: American College of Radiology (2003).
19. Chang JM, Leung JWT, Moy L, Ha SM, Moon WK. Axillary Nodal Evaluation in Breast Cancer: State of the Art. Radiology (2020) 295(3):500–15. doi: 10.1148/radiol.2020192534
20. Caudle AS, Kuerer HM, Le-Petross HT, Yang W, Yi M, Bedrosian I, et al. Predicting the extent of nodal disease in early-stage breast cancer. Ann Surg Oncol (2014) 21(11):3440–7. doi: 10.1245/s10434-014-3813-4
21. Verheuvel NC, van den Hoven I, Ooms HW, Voogd AC, Roumen RM. The role of ultrasound-guided lymph node biopsy in axillary staging of invasive breast cancer in the post-ACOSOG Z0011 trial era. Ann Surg Oncol (2015) 22(2):409–15. doi: 10.1245/s10434-014-4071-1
22. Akissue de Camargo Teixeira P, Chala LF, Shimizu C, Filassi JR, Maesaka JY, de Barros N. Axillary Lymph Node Sonographic Features and Breast Tumor Characteristics as Predictors of Malignancy: A Nomogram to Predict Risk. Ultrasound Med Biol (2017) 43(9):1837–45. doi: 10.1016/j.ultrasmedbio.2017.05.003
23. Meretoja TJ, Heikkila PS, Mansfield AS, Cserni G, Ambrozay E, Boross G, et al. A predictive tool to estimate the risk of axillary metastases in breast cancer patients with negative axillary ultrasound. Ann Surg Oncol (2014) 21(7):2229–36. doi: 10.1245/s10434-014-3617-6
24. Moon WK, Chen IL, Yi A, Bae MS, Shin SU, Chang RF. Computer-aided prediction model for axillary lymph node metastasis in breast cancer using tumor morphological and textural features on ultrasound. Comput Methods Programs Biomed (2018) 162:129–37. doi: 10.1016/j.cmpb.2018.05.011
25. Moon WK, Lee YW, Huang YS, Lee SH, Bae MS, Yi A, et al. Computer-aided prediction of axillary lymph node status in breast cancer using tumor surrounding tissue features in ultrasound images. Comput Methods Programs Biomed (2017) 146:143–50. doi: 10.1016/j.cmpb.2017.06.001
Keywords: nomogram, breast cancer, axillary lymph node metastasis, ultrasound, prediction model
Citation: Luo Y, Zhao C, Gao Y, Xiao M, Li W, Zhang J, Ma L, Qin J, Jiang Y and Zhu Q (2020) Predicting Axillary Lymph Node Status With a Nomogram Based on Breast Lesion Ultrasound Features: Performance in N1 Breast Cancer Patients. Front. Oncol. 10:581321. doi: 10.3389/fonc.2020.581321
Received: 08 July 2020; Accepted: 29 September 2020;
Published: 27 October 2020.
Edited by:
Katsutoshi Sugimoto, Tokyo Medical University, JapanReviewed by:
Hirohito Takeuchi, Tokyo Medical University, JapanMutsumi Nishida, Hokkaido University Hospital, Japan
Copyright © 2020 Luo, Zhao, Gao, Xiao, Li, Zhang, Ma, Qin, Jiang and Zhu. This is an open-access article distributed under the terms of the Creative Commons Attribution License (CC BY). The use, distribution or reproduction in other forums is permitted, provided the original author(s) and the copyright owner(s) are credited and that the original publication in this journal is cited, in accordance with accepted academic practice. No use, distribution or reproduction is permitted which does not comply with these terms.
*Correspondence: Yuxin Jiang, jiangyuxinxh@163.com; Qingli Zhu, zqlpumch@126.com
†These authors have contributed equally to this work