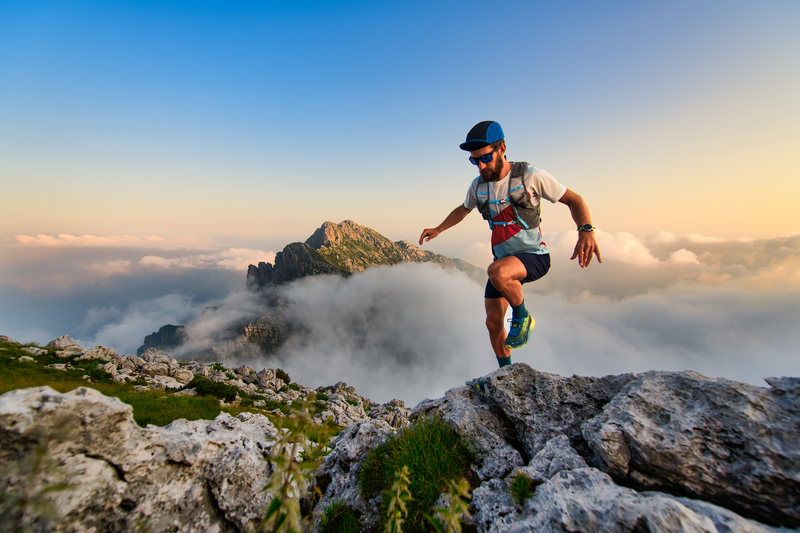
94% of researchers rate our articles as excellent or good
Learn more about the work of our research integrity team to safeguard the quality of each article we publish.
Find out more
ORIGINAL RESEARCH article
Front. Oncol. , 14 October 2020
Sec. Cancer Immunity and Immunotherapy
Volume 10 - 2020 | https://doi.org/10.3389/fonc.2020.560779
This article is part of the Research Topic Genetic and Epigenetic Control on Immune Responses Regulating Molecules in Cancer Development, Progression, and Treatment View all 23 articles
Background: Recent research has shown that immune-related lncRNA plays a crucial part in the tumor immune microenvironment. This study tried to identify immune-related lncRNAs and construct a robust prediction model to increase the predicted value of lung adenocarcinoma (LUAD).
Methods: RNA expression data of LUAD were download from the Cancer Genome Atlas (TCGA) database. Immune genes were acquired from the Molecular Signatures Database (MSigDB). The immune gene related lncRNAs were acquired by the “limma R” package and Cytoscape3.7.1. Cox regression analysis was applied to construct this forecast model. The prognostic model was validated by the testing cohort which was acquired by the bootstrap method.
Results: A total of 551 lncRNA expression profiles including 497 LUAD tissues and 54 non-LUAD tissues were obtained. A total of 331 immune genes were acquired. The result of the Cox regression analysis showed that seven lncRNAs (AC022784-1, NKILA, AC026355-1, AC068338-3, LINC01843, SYNPR-AS1, and AC123595-1) can be performed to construct the prediction model to forecast the prognosis of LUAD. Kaplan–Meier curves indicated that our prediction model can distribute LUAD patients into two different risk groups (high and low) with significant statistical significance (P = 1.484e-07). Cox analysis and independent analysis illustrated that the seven-lncRNAs prediction model was an isolated factor by comparing it with other clinical variables. We validated the accuracy of our model in the testing dataset. Furthermore, the prognostic model also showed higher predictive efficiency than three other published prognostic models. The two different survival groups represented diverse immune features according to principal components analysis. GSEA analysis (gene set enrichment analysis) indicated that seven-lncRNAs signatures may be involved in the progression of tumorigenesis.
Conclusions: We have established a seven immune-related lncRNAs prediction model. This prognostic model had significant clinical significance that increased the predicted value and guided the personalized treatment for LUAD patients.
Lung cancer belongs to the malignant tumor group and has becoming the primary killer in tumor-related disease (1, 2). Lung cancer is separated into two important categories including small cell lung cancer (SCLC) and non-small cell lung cancer (NSCLC) (3, 4). Lung adenocarcinoma (LUAD) is the major type of NSCLC and the morbidity of LUAD has surpassed lung squamous carcinoma in recent years (5). Due to the deficiency of tumor diagnosis using the traditional bronchoscopy and computed tomography techniques, patients in the early stage of cancer are difficult to be detected (6, 7). Therefore, it is imperative to find prognostic biomarkers that instruct the treatment of lung cancer. Currently, a lot of attention has been given to the field of immune therapies that could regulate the tumor microenvironment (8–10). For example, a study shows the relationship between the lncRNA signature of tumor-infiltrating B lymphocytes and the immune therapies of bladder cancer (11). Additionally, lncRNAs have several significant biological functions. For example, they participate in high-order chromosomal dynamics and mediate epigenetic changes (12, 13). Furthermore, lncRNA has an important value in evaluating the immune infiltrate of the tumor (14). Recently, Song et al. found that a gene signature including 30 immune-related genes could predict prognosis and reveal the relationship between the tumor and the immune microenvironment (15). Li et al. found that the clinical immune signature can act as a conspicuous marker to evaluate the overall survival rate in NSCLC and patients in the early phase (16). Prognostic biomarkers based on the immune-related lncRNA model for LUAD is still lacking.
In this study, our efforts concentrated on obtaining lncRNA expression profiles and immune genes to establish a prediction model to enhance the prognosis ability of LUAD. Then we identified whether our prognostic model was connected with the survival time of LUAD patients and independent of other clinical variables. Finally, we aimed to show the possible biological pathway of the prediction model.
The RNA-seq FPKM (reads per kilobase per million) data of 551 LUAD patients (including 497 LUAD tissues and 54 non-LUAD tissues) were download from The Cancer Genome Atlas (TCGA) database.1 Non-LUAD tissue refers to adjacent normal material. There were 445 LUAD patients, including their clinical follow-up information, who were taken into consideration. We chose patients whose survival data >30 days in order to improve the accuracy of our study (17). Patients whose survival data <30 days may die of other diseases rather than LUAD.
The immune genes were download from the Molecular Signatures Database (MSigDB).2 The “limma R” package was used to detect immune lncRNAs. The immune-related lncRNAs were identified by the correlation analysis between the immune genes and lncRNA expression levels in the LUAD samples. We used the function of cor. test () to calculate the correlation coefficient. We set the coefficient of the cor-Filter >0.6 and P value < 0.001.
Cox regression analysis was used to build a prognosis signature of survival using the “survival R” package. lncRNAs with prominently statistical significance in univariable Cox regression were chosen and put into multivariable Cox regression. The risk score of every LUAD patient was computed based on the expression quantity of the model lncRNAs and their coefficient. The risk score was calculated as: risk score = βgene1 × Expressiongene1 + βgene2 × Expressiongene2 + βgene3 × Expressiongene3 + … + βgenen × Expressiongenen (18). Then we divided the LUAD patients into high and low risk groups according to the median risk score.
A Kaplan–Meier survival curve were performed to identify the difference of overall survival in the two different risk groups in the training set. We used the “survival R” package and “survminer R” package to make the K–M survival curve. Then independent analysis was applied to verify the independence of our prediction model by comparing age, gender, stage, and TNM (pathological T stage, pathological N stage, and pathological M stage) pathological stage.
In order to validate the validity of the prognosis model, the testing dataset was acquired by a bootstrap method based on resampling of 1000 times (19). The original dataset acted as a training set. In the testing set, we calculated the risk score based on the expression quantity of the model lncRNA and their coefficient according to the training set. Additionally, we compared our prognostic model with existing gene prognostic models by receiver operating curve (ROC) and C-index analysis. The R package of “survival ROC” and the R package of “survcomp” were used to make the ROC curve and calculate the C-index respectively.
Principal components analysis (PCA) was used to show the different immune statuses of LUAD patients based on the whole gene expression profiles and the prediction model. The “limma R” package and “scatterplot3d R” package were used to complete the PCA analysis. Gene set enrichment analysis (GSEA4.0.3) was used to identify the biological function of the prediction model.
Cox regression analysis, survival analysis, and PCA analysis were achieved in the R software (version 3.6.0). Kaplan–Meier survival analyses were performed by the “survival R” package and “survminer R” package in R software. We verified the prediction model with the “survival R” package, “survminer R” package, “survival ROC R” package, “pheatmap R” package, and the “ggpubr R” package. Gene set enrichment analysis analysis results whose NOM-P value < 0.05 was thought to be statistically significant.
Figure 1 presents the flow diagram of this research. A total of 14,144 lncRNAs sequencing data were obtained from TCGA database and 331 immune genes were detected from MSigDB (20). Immune-related lncRNAs were received by building the immune lncRNAs co-expression network through the “limma package” in R studio and Cytoscape3.7.1 (Figure 2A). The co-expression network refers to the relationship between the immune genes and the lncRNAs. Finally, 554 lncRNAs were identified (P ≤ 0.001).
Figure 2. Co-expression network and the result of Cox regression analysis. (A) Co-expression network between immune genes and lncRNAs. (B) The forest plot of univariate cox regression identified twelve immune-related lncRNAs associated with OS. (C) The forest plot of multivariate Cox regression analysis of seven immune-related lncRNAs associated with OS.
Univariate cox regression analysis was first applied to recognize the predictive model. A total of 12 immune-related lncRNAs had a conspicuous connection with the overall survival rate (P < 0.001). The forest plot of the univariate cox regression is shown in Figure 2B. Then, 12 immune-related lncRNAs were selected and put into the multivariate Cox regression analysis. The result revealed that seven immune-related lncRNAs can act as independent prognostic factors for LUAD. The forest plot of the multivariate cox regression is shown in Figure 2C. Finally, the seven immune-related lncRNAs were selected as our model to predict LUAD’s prognosis (Table 1). The risk score of each patient was computed according to the following formula. Risk score = 0.167 × expression quantity of AC022784-1 + 0.274 × expression quantity of NKILA + (−0.401) × AC026355-1 + (−0.487) × expression quantity of AC068338-3 + 0.171 × expression quantity of LINC01843 + (−0.168) × expression quantity of SYNPR-AS1 + (−0.472) × expression quantity of AC123595-1. We obtained a high-risk group (n = 222) and a low-risk group (n = 223) according to the median risk scores. The overall survival (OS) of patients in the high-risk group are shorter than in the other group (P-value = 1.484e-7; Figure 3A). In our survival analysis, the survival rate of the LUAD patients in the low-risk group was 66% after 3 years, 46% after 6 years, however, the survival rate in the high-risk group was only 48% after 3 years, 17% after 6 years, respectively. The risk score curve and survival status data of these two different groups are shown in Figure 3B. The abscissa axis of the risk score curve and survival status data were ranked by the risk score value. This result showed that the mortality of LUAD patients in the high-risk group was much higher than patients in the other group. To show the expression difference of our model lncRNAs, we used a heat-map plot (Figure 3C). The heat-map showed that the expression of lncRNAs (NKILA, AC022784-1, LINC01843) were obviously up-regulated in patients in the high-risk group, whereas the model lncRNAs (SYNPR-AS1, AC026355-1, AC068338-3, AC123595-1) were down-regulated. In the low-risk group, the expression of NKILA, AC022784-1, and LINC01843 were correspondingly decreased. The accuracy of the prognostic model was shown in the ROC curve. The area under the curve (AUC) values of the ROC curve of the 1-, 3-, and 5-year OS were 0.747, 0.678, and 0.702, respectively (Figure 3D). Then, the prognostic model was verified in the testing dataset, the OS in the high-risk group was significantly worse than that in the low-risk group (P-value = 9.853e-10; Figure 3E). The risk score curve and survival status data of the two different groups in the testing dataset are shown in Figure 3F. The heat-map plot of the testing dataset is shown in Figure 3G. Similarly, the AUC values of the 1-, 3-, and 5-year OS in the testing dataset were 0.847, 0.696, and 0.747, respectively (Figure 3H). The C-index for OS predictions in the training dataset and testing dataset were 0.687 (95% CI, 0.639–0.735) and 0.749 (95% CI, 0.707–0.791) (Figure 4).
Figure 3. Kaplan–Meier curve survival analysis, risk score analysis, heatmap, Time–ROC curve analysis. (A) Kaplan–Meier curve, (B) risk score, (C) heatmap, (D) time–ROC curve of the immune-related lncRNA signature in the training cohort. (E) Kaplan–Meier curve, (F) risk score, (G) heatmap, and (H) time–ROC curve of the immune-related lncRNA signature in the testing cohort.
Figure 4. C-index of the immune-related lncRNA signature in the training cohort and the testing cohort.
In order to evaluate whether the survival prognosis of these lncRNAs were independent of other clinical factors. We used cox regression analysis to analysis LUAD clinical characteristics based on the predictive model. Univariate independent prognostic analysis illustrated that the risk score, stage, pathological T stage, and pathological N stage have statistical difference when connected with overall survival (P < 0.001) (Figure 5A). Multivariate independent prognostic analysis illustrated that stage and risk score can be considered as independent predicted factors of LUAD (P < 0.05) (Figure 5B). In conclusion, univariate and multivariate independent prognostic analysis illustrated that the prediction model was an independent predicted factor (P < 0.05) (Table 2). Furthermore, we used stratification analysis to identify the independence of the prediction model (Figures 5C,D). For stage, AC068338-3 was significantly up-regulated in early stage LUAD (P < 0.001). AC022784-1 was determined to be over expressed in patients of later stage LUAD (P < 0.05). SYNPR-AS1 was significantly up-regulated in early stage LUAD (P < 0.05). AC068338-3 had obviously statistical significance comparing with the pathological T stage (P < 0.001). The expression of AC068338-3 was down-regulated with the development of the pathological T stage.
Figure 5. Stratification analyses of clinicopathological characteristics. (A) Univariate independent prognostic analysis forest map of the prognostic model and LUAD clinicopathological characteristics. (B) Multivariate independent prognostic analysis forest map of prognostic model and LUAD clinicopathological characteristics. (C) Stratification analyses of all patients adjusted to stage using the signature of seven immune-related lncRNAs. (D) Stratification analyses of all patients adjusted to T stage using the signature of seven immune-related lncRNAs.
We compared our prognostic model with other published prognostic signatures (21–23). In Figure 6A, the AUC of ROC for 5-year OS in our seven immune-related lncRNA model was 0.702. The C-index is 0.687. Comparing with other prognostic models, our model has superior predictive sensitivity and specificity. The AUC of the eight-lncRNA signature of Miao, seven-lncRNA signature of Lin, and five lncRNA signature of Zeng are 0.627, 0.542, and 0.542, respectively. The C-index of the eight-lncRNA signature of Miao, seven-lncRNA signature of Lin, and five lncRNA signature of Zeng are 0.613, 0.531, and 0.530, respectively (Figure 6B).
Figure 6. Comparison of the receiver operating characteristic (ROC) curve (A) and c-index (B) for different prognostic signatures.
In order to identify the discrepancy between the two risk groups based on our model lncRNAs and the total gene expression, PCA analysis was applied to our progression (Figure 7). The result illustrated that patients in these two groups were spread in different directions. However, the model lncRNAs divided LUAD patients into two specific sections, showing that the immune status of LUAD patients were quite different in the two groups. To identify the unknown function of the seven-lncRNAs model, we used GSEA analysis to find possible biological functions of the seven-lncRNA model of LUAD (Figure 8). The GSEA analysis showed that five tumor gene sets (“P53_SIGNALING_PATHWAY,” “DNA_REPLICATION,” “CELL_CYCLE,” “SMALL_CELL_LUNG_CANCER,” “PATHWAYS_IN_CANCER”) were obviously enriched in the high-risk group. The result indicated that these associated biological pathways can significantly affect the tumorigenesis of LUAD.
Figure 7. Low- and high-risk groups displayed different immune status. (A) Principal components analysis between low- and high-risk groups based on whole gene expression profiles. (B) Principal components analysis between low- and high-risk groups based on the signature of seven immune-related lncRNAs.
Figure 8. Gene set enrichment analysis (GSEA) between high and low immune risk groups. (A) CELL_CYCLE; (B) SMALL_CELL_LUNG_CANCER; (C) P53_SIGNALING_PATHWAY; (D) DNA_REPLICATION; (E) PATHWAYS_IN_CANCER.
Lung adenocarcinoma is the most common pathological subtype and it has been widely detected over the years via unique genetic changes and various prognostic factors (24–26). Due to unknown pathogenesis, the mortality of LUAD patients is still high and the treatment outcome is also unsatisfactory (27). In recent years, lncRNAs have played a more significant role in the development of tumors and the disorder of lncRNA may be considered as a crucial factor in the activities of tumors (28, 29). We have found that lncRNA participated in 33 kinds of cancers. Moreover, the importance of immune-related genes in tumor progression and immunotherapies has become apparent (30–32). Immune-related lncRNA helps to prioritize cancer-related lncRNA and distinguish cancer subtypes based on specific immunological characteristics. In addition, in recent years, prognostic biomarkers combined with the tumor immune microenvironment have already emerged (33–36). The value of immune-related lncRNA has been shown in many cancers such as hepatocellular carcinoma (HCC), anaplastic glioma (37), glioblastoma multiforme (38), diffuse large B cell lymphoma (39), and breast cancer. In the research of HCC, Zhang et al. identified that the immune-related lncRNA signature not only had a significant effect on survival prognosis but also had the possible role of evaluating the response to ICB (immune checkpoint blockade) immunotherapy (40). Shen et al. discovered the 11-lncRNA signature of breast cancer and analyzed the correlation between the lncRNA prognostic signature and the infiltration of immune cell subtypes (41). This novel field can improve patient treatment in the era of immunotherapy. Although the preciseness of survival prediction has still not reached its potential and many limitations remain. Therefore, it is necessary to identify accurate markers and to choose suitable immune therapies to enhance the survival rate of LUAD.
Recently, several studies have revealed prognostic markers of the tumor immune microenvironment that are capable of predicting the prognosis of the tumor. Shen et al. found that an immune gene model can act as a possible marker to predict the prognosis of clear renal clear cell cancer (42). Yang et al. revealed that a prediction immune model can forecast the survival outcome of cervical cancer (43). Chen et al. reflected that a nine immune gene model has prognostic value for HCC (44). Nowadays, prognostic biomarkers related to tumor immunity in lung cancer are still lacking. In our research, we tried to detect the prognostic model of LUAD based on immune-related lncRNA.
In our study, we demonstrated that a seven immune-related lncRNA can improve prognosis prediction in LUAD. Gene set enrichment analysis analysis revealed that these lncRNAs were enriched in the pathways of “P53_SIGNALING_PATHWAY,” “DNA_REPLICATION,” “CELL_CYCLE,” “SMALL_CELL_LUNG_CANCER,” and “PATHWAYS_IN_CANCER,” A recent study illustrated that an immune regulatory protein can induce apoptosis of lung carcinoma cells through the P53 signaling pathway (45). The P53 signature pathway is closely connected to the progression of lung carcinoma. César Muñoz-Fontela et al. indicated that the p53 signaling pathway has an extensive impact on immune responses (46). For example, it can regulate the immune signal to participate in the immune reaction and effect autoimmunity by restraining inappropriate response of inflammatory cytokines (47–49). DNA replication is a significant molecular mechanism of tumorigenesis. Macheret et al. indicated that the enhancement of DNA replication is an important symbol of cancer progression (50). A recent study revealed that ailanthone can suppress NSCLC growth by restraining DNA replication through reducing RPA1 (replication protein A1) (51). In our research, the prediction signature was not only connected with the immune response but also with tumorigenesis. Compared with previous studies, our study first constructed the prognostic model based on immune-related lncRNAs.
In this study, the immune-related lncRNAs prediction model was established by univariate and multivariate Cox regression analysis. In order to verify the efficiency of our model, we used a K–M survival curve to illustrate the survival time of the two groups. The P value of the K–M survival curve was 1.484e-7, which indicated that our predicated model had a strong correlation with the survival outcomes of LUAD patients. Furthermore, we validated our prognostic signature in the testing set. The AUC of ROC of 5 years were 0.702 and 0.747, respectively in the training dataset and the testing dataset, which showed that this model had superior accuracy. Our prognostic model was also more superior than other prognostic signatures by comparison. Principal components analysis indicated that the prediction model was equipped to separate LUAD patients into different groups according to their immune status. Finally, we used GSEA analysis to detect the biological functions of our prediction model. The result powerfully identified that these lncRNAs participated in the progression of the tumors.
In conclusion, in this study, we constructed an immune-related lncRNAs model of LUAD. The finding illustrated that the seven immune-related lncRNAs prognosis model was efficient in predicting the clinical prognosis. Furthermore, studies based on the immune response and lncRNA not only enhanced the diagnosis rate but also gave us a new direction for immune therapy.
RNAseq FPKM data was downloaded from the TCGA GDC data portal (https://portal.gdc.cancer.gov/) under the project ID of TCGA-LUAD. Immune genes were downloaded from The Molecular Signatures Database (https://www.gsea-msigdb.org/gsea/msigdb/) under the accession numbers of Immune system process M13664 and Immune response M19817.
Y-QQ and J-PL designed the study. RL and XL collected the literature. CH, T-TL, and JY analyzed the data. J-PL drafted the manuscript. Y-QQ modified the manuscript. All authors read and approved the final manuscript.
This work was supported by grants from the Major Scientific and Technological Innovation Project of Shandong Province (2018CXGC1212), the Science and Technology Foundation of Shandong Province (2014GSF118084), the CSCO-Qilu Cancer Research Fund (Y-Q201802-014), the Medical and Health Technology Innovation Plan of Jinan City (201805002), and the National Natural Science Foundation of China (81372333).
The authors declare that the research was conducted in the absence of any commercial or financial relationships that could be construed as a potential conflict of interest.
LUAD, lung adenocarcinoma; TCGA, The Cancer Genome Atlas; MSigDB, Molecular Signatures Database; SCLC, small cell lung cancer; NSCLC, non-small cell lung cancer; OS, overall survival; ROC, receiver operating curve; AUC, area under ROC curve; PCA, principal components analysis; GSEA, gene set enrichment analysis.
1. Schabath MB, DiGiovanni J. Introduction to special issue: recent advances in mechanisms, prevention and treatment of lung cancer. Mol Carcinog. (2015) 54(Suppl. 1):vi. doi: 10.1002/mc.22337
2. Bray F, Ferlay J, Soerjomataram I, Siegel RL, Torre LA, Jemal A. Global cancer statistics 2018: GLOBOCAN estimates of incidence and mortality worldwide for 36 cancers in 185 countries. CA Cancer J Clin. (2018) 68:394–424. doi: 10.3322/caac.21492
3. Liu J, Cho SN, Akkanti B, Jin N, Mao J, Long W, et al. ErbB2 pathway activation upon Smad4 loss promotes lung tumor growth and metastasis. Cell Rep. (2015) 10:1599–613. doi: 10.1016/j.celrep.2015.02.014
4. Rodriguez-Canales J, Parra-Cuentas E, Wistuba II. Diagnosis and molecular classification of lung cancer. Cancer Treat Res. (2016) 170:25–46. doi: 10.1007/978-3-319-40389-2_2
5. Tong L, Liu J, Yan W, Cao W, Shen S, Li K, et al. RDM1 plays an oncogenic role in human lung adenocarcinoma cells. Sci Rep. (2018) 8:11525. doi: 10.1038/s41598-018-30071-y
6. Sun S, Schiller JH, Gazdar AF. Lung cancer in never smokers–a different disease. Nat Rev Cancer. (2007) 7:778–90. doi: 10.1038/nrc2190
7. Yerukala Sathipati S, Ho SY. Identifying the miRNA signature associated with survival time in patients with lung adenocarcinoma using miRNA expression profiles. Sci Rep. (2017) 7:7507. doi: 10.1038/s41598-017-07739-y
8. Steven A, Fisher SA, Robinson BW. Immunotherapy for lung cancer. Respirology. (2016) 21:821–33. doi: 10.1111/resp.12789
9. Madureira P, de Mello RA, de Vasconcelos A, Zhang Y. Immunotherapy for lung cancer: for whom the bell tolls? Tumour Biol. (2015) 36:1411–22. doi: 10.1007/s13277-015-3285-6
10. Raez LE, Cardona AF, Santos ES, Catoe H, Rolfo C, Lopes G, et al. The burden of lung cancer in Latin-America and challenges in the access to genomic profiling, immunotherapy and targeted treatments. Lung Cancer. (2018) 119:7–13. doi: 10.1016/j.lungcan.2018.02.014
11. Zhou M, Zhang Z, Bao S, Hou P, Yan C, Su J, et al. Computational recognition of lncRNA signature of tumor-infiltrating B lymphocytes with potential implications in prognosis and immunotherapy of bladder cancer. Brief Bioinform. (2020) 1–13. doi: 10.1093/bib/bbaa047
12. Mercer TR, Dinger ME, Mattick JS. Long non-coding RNAs: insights into functions. Nat Rev Genet. (2009) 10:155–9. doi: 10.1038/nrg2521
13. Fang Y, Fullwood MJ. Roles, functions, and mechanisms of long non-coding RNAs in cancer. Genomics Proteomics Bioinformatics. (2016) 14:42–54. doi: 10.1016/j.gpb.2015.09.006
14. Sun J, Zhang Z, Bao S, Yan C, Hou P, Wu N, et al. Identification of tumor immune infiltration-associated lncRNAs for improving prognosis and immunotherapy response of patients with non-small cell lung cancer. J Immunother Cancer. (2020) 8:e000110. doi: 10.1136/jitc-2019-000110
15. Song Q, Shang J, Yang Z, Zhang L, Zhang C, Chen J, et al. Identification of an immune signature predicting prognosis risk of patients in lung adenocarcinoma. J Transl Med. (2019) 17:70. doi: 10.1186/s12967-019-1824-4
16. Li B, Cui Y, Diehn M, Li R. Development and validation of an individualized immune prognostic signature in early-stage nonsquamous non-small cell lung cancer. JAMA Oncol. (2017) 3:1529–37. doi: 10.1001/jamaoncol.2017.1609
17. Xia Z, Ou-Yang W, Hu T, Du K. Prognostic significance of CDC25C in lung adenocarcinoma: an analysis of TCGA data. Cancer Genet. (2019) 233–4:67–74. doi: 10.1016/j.cancergen.2019.04.001
18. Li X, Meng Y. Survival analysis of immune-related lncRNA in low-grade glioma. BMC Cancer. (2019) 19:813. doi: 10.1186/s12885-019-6032-3
19. Wang J, Li Y, Fu W, Zhang Y, Jiang J, Zhang Y, et al. Prognostic nomogram based on immune scores for breast cancer patients. Cancer Med. (2019) 8:5214–22. doi: 10.1002/cam4.2428
20. Subramanian A, Tamayo P, Mootha VK, Mukherjee S, Ebert BL, Gillette MA, et al. Gene set enrichment analysis: a knowledge-based approach for interpreting genome-wide expression profiles. Proc Natl Acad Sci USA. (2005) 102:15545–50. doi: 10.1073/pnas.0506580102
21. Miao R, Ge C, Zhang X, He Y, Ma X, Xiang X, et al. Combined eight-long noncoding RNA signature: a new risk score predicting prognosis in elderly non-small cell lung cancer patients. Aging. (2019) 11:467–79. doi: 10.18632/aging.101752
22. Lin T, Fu YN, Zhang X, Gu JX, Ma XH, Miao RC, et al. A seven-long noncoding RNA signature predicts overall survival for patients with early stage non-small cell lung cancer. Aging. (2018) 10:2356–66. doi: 10.18632/aging.101550
23. Zeng L, Wang W, Chen Y, Lv X, Yuan J, Sun R, et al. A five-long non-coding RNA signature with the ability to predict overall survival of patients with lung adenocarcinoma. Exp Ther Med. (2019) 18:4852–64. doi: 10.3892/etm.2019.8138
24. Wu Q, Wang L, Wei H, Li B, Yang J, Wang Z, et al. Integration of multiple key molecules in lung adenocarcinoma identifies prognostic and immunotherapeutic relevant gene signatures. Int Immunopharmacol. (2020) 83:106477. doi: 10.1016/j.intimp.2020.106477
25. Zhao Y, Wang R, Shen X, Pan Y, Cheng C, Li Y, et al. Minor components of micropapillary and solid subtypes in lung adenocarcinoma are predictors of lymph node metastasis and poor prognosis. Ann Surg Oncol. (2016) 23:2099–105. doi: 10.1245/s10434-015-5043-9
26. Fujimoto J, Nunomura-Nakamura S, Liu Y, Lang W, McDowell T, Jakubek Y, et al. Development of Kras mutant lung adenocarcinoma in mice with knockout of the airway lineage-specific gene Gprc5a. Int J Cancer. (2017) 141:1589–99. doi: 10.1002/ijc.30851
27. Wang J, Wang Y, Tong M, Pan H, Li D. Research progress of the clinicopathologic features of lung adenosquamous carcinoma. Onco Targets Ther. (2018) 11:7011–7. doi: 10.2147/OTT.S179904
28. Mohebi M, Ghafouri-Fard S, Modarressi MH, Dashti S, Zekri A, Kholghi-Oskooei V, et al. Expression analysis of vimentin and the related lncRNA network in breast cancer. Exp Mol Pathol. (2020) 115:104439. doi: 10.1016/j.yexmp.2020.104439
29. Guan H, Zhu T, Wu S, Liu S, Liu B, Wu J, et al. Long noncoding RNA LINC00673-v4 promotes aggressiveness of lung adenocarcinoma via activating WNT/beta-catenin signaling. Proc Natl Acad Sci USA. (2019) 116:14019–28. doi: 10.1073/pnas.1900997116
30. Ko EC, Raben D, Formenti SC. the integration of radiotherapy with immunotherapy for the treatment of non-small cell lung cancer. Clin Cancer Res. (2018) 24:5792–806. doi: 10.1158/1078-0432.CCR-17-3620
31. Li Y, Jiang T, Zhou W, Li J, Li X, Wang Q, et al. Pan-cancer characterization of immune-related lncRNAs identifies potential oncogenic biomarkers. Nat Commun. (2020) 11:1000. doi: 10.1038/s41467-020-14802-2
32. Tomasi TB, Magner WJ, Khan ANH. Epigenetic regulation of immune escape genes in cancer. Cancer Immunol Immunother. (2006) 55:1159–84. doi: 10.1007/s00262-006-0164-4
33. Taube JM, Galon J, Sholl LM, Rodig SJ, Cottrell TR, Giraldo NA, et al. Implications of the tumor immune microenvironment for staging and therapeutics. Mod Pathol. (2018) 31:214–34. doi: 10.1038/modpathol.2017.156
34. Kirilovsky A, Marliot F, El Sissy C, Haicheur N, Galon J, Pages F. Rational bases for the use of the immunoscore in routine clinical settings as a prognostic and predictive biomarker in cancer patients. Int Immunol. (2016) 28:373–82. doi: 10.1093/intimm/dxw021
35. Galon J, Pages F, Marincola FM, Thurin M, Trinchieri G, Fox BA, et al. The immune score as a new possible approach for the classification of cancer. J Transl Med. (2012) 10:1. doi: 10.1186/1479-5876-10-1
36. Galon J, Bindea G, Mlecnik B, Angell H, Lagorce C, Todosi AM, et al. [Intratumoral immune microenvironment and survival: the immunoscore]. Med Sci. (2014) 30:439–44. doi: 10.1051/medsci/20143004020
37. Wang W, Zhao Z, Yang F, Wang H, Wu F, Liang T, et al. An immune-related lncRNA signature for patients with anaplastic gliomas. J Neurooncol. (2018) 136:263–71. doi: 10.1007/s11060-017-2667-6
38. Zhou M, Zhang Z, Zhao H, Bao S, Cheng L, Sun J. An immune-related six-lncRNA signature to improve prognosis prediction of glioblastoma multiforme. Mol Neurobiol. (2018) 55:3684–97. doi: 10.1007/s12035-017-0572-9
39. Zhou M, Zhao H, Xu W, Bao S, Cheng L, Sun J. Discovery and validation of immune-associated long non-coding RNA biomarkers associated with clinically molecular subtype and prognosis in diffuse large B cell lymphoma. Mol Cancer. (2017) 16:16. doi: 10.1186/s12943-017-0580-4
40. Zhang Y, Zhang L, Xu Y, Wu X, Zhou Y, Mo J. Immune-related long noncoding RNA signature for predicting survival and immune checkpoint blockade in hepatocellular carcinoma. J Cell Physiol. (2020) 235, 9304–9316. doi: 10.1002/jcp.29730
41. Shen Y, Peng X, Shen C. Identification and validation of immune-related lncRNA prognostic signature for breast cancer. Genomics. (2020) 112:2640–6. doi: 10.1016/j.ygeno.2020.02.015
42. Shen C, Liu J, Wang J, Zhong X, Dong D, Yang X, et al. Development and validation of a prognostic immune-associated gene signature in clear cell renal cell carcinoma. Int Immunopharmacol. (2020) 81:106274. doi: 10.1016/j.intimp.2020.106274
43. Yang S, Wu Y, Deng Y, Zhou L, Yang P, Zheng Y, et al. Identification of a prognostic immune signature for cervical cancer to predict survival and response to immune checkpoint inhibitors. Oncoimmunology. (2019) 8:e1659094. doi: 10.1080/2162402X.2019.1659094
44. Chen W, Ou M, Tang D, Dai Y, Du W. Identification and validation of immune-related gene prognostic signature for hepatocellular carcinoma. J Immunol Res. (2020) 2020:5494858. doi: 10.1155/2020/5494858
45. Wang JJ, Wang Y, Hou L, Xin F, Fan B, Lu C, et al. . Immunomodulatory protein from nectria haematococca induces apoptosis in lung cancer cells via the P53 pathway. Int J Mol Sci. (2019) 20:5348. doi: 10.3390/ijms20215348
46. Munoz-Fontela C, Mandinova A, Aaronson SA, Lee SW. Emerging roles of p53 and other tumour-suppressor genes in immune regulation. Nat Rev Immunol. (2016) 16:741–50. doi: 10.1038/nri.2016.99
47. Watanabe M, Moon KD, Vacchio MS, Hathcock KS, Hodes RJ. Downmodulation of tumor suppressor p53 by T cell receptor signaling is critical for antigen-specific CD4(+) T cell responses. Immunity. (2014) 40:681–91. doi: 10.1016/j.immuni.2014.04.006
48. Menendez D, Shatz M, Resnick MA. Interactions between the tumor suppressor p53 and immune responses. Curr Opin Oncol. (2013) 25:85–92. doi: 10.1097/CCO.0b013e32835b6386
49. Takatori H, Kawashima H, Suzuki K, Nakajima H. Role of p53 in systemic autoimmune diseases. Crit Rev Immunol. (2014) 34:509–16. doi: 10.1615/critrevimmunol.2014012193
50. Macheret M, Halazonetis TD. DNA replication stress as a hallmark of cancer. Annu Rev Pathol. (2015) 10:425–48. doi: 10.1146/annurev-pathol-012414-040424
Keywords: lung adenocarcinoma, immune-related lncRNAs, GSEA analysis, prognosis, predicted model
Citation: Li J-P, Li R, Liu X, Huo C, Liu T-T, Yao J and Qu Y-Q (2020) A Seven Immune-Related lncRNAs Model to Increase the Predicted Value of Lung Adenocarcinoma. Front. Oncol. 10:560779. doi: 10.3389/fonc.2020.560779
Received: 10 May 2020; Accepted: 13 August 2020;
Published: 14 October 2020.
Edited by:
Alejandro Villagra, George Washington University, United StatesReviewed by:
Meng Zhou, Wenzhou Medical University, ChinaCopyright © 2020 Li, Li, Liu, Huo, Liu, Yao and Qu. This is an open-access article distributed under the terms of the Creative Commons Attribution License (CC BY). The use, distribution or reproduction in other forums is permitted, provided the original author(s) and the copyright owner(s) are credited and that the original publication in this journal is cited, in accordance with accepted academic practice. No use, distribution or reproduction is permitted which does not comply with these terms.
*Correspondence: Yi-Qing Qu, cXV5aXFpbmdAc2R1LmVkdS5jbg==
Disclaimer: All claims expressed in this article are solely those of the authors and do not necessarily represent those of their affiliated organizations, or those of the publisher, the editors and the reviewers. Any product that may be evaluated in this article or claim that may be made by its manufacturer is not guaranteed or endorsed by the publisher.
Research integrity at Frontiers
Learn more about the work of our research integrity team to safeguard the quality of each article we publish.