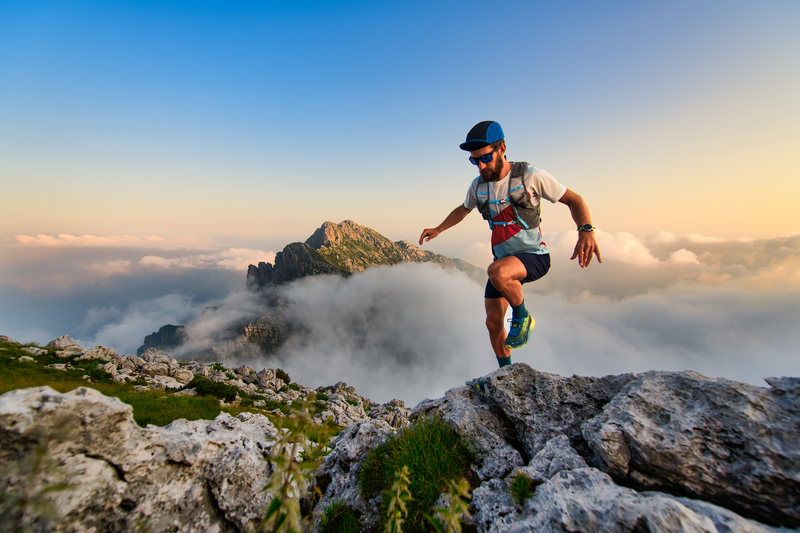
94% of researchers rate our articles as excellent or good
Learn more about the work of our research integrity team to safeguard the quality of each article we publish.
Find out more
ORIGINAL RESEARCH article
Front. Oncol. , 14 October 2020
Sec. Surgical Oncology
Volume 10 - 2020 | https://doi.org/10.3389/fonc.2020.551668
This article is part of the Research Topic Novel Insights into the Treatment of HCC and Liver Tumors View all 41 articles
Background: Surgery is a potential cure for hepatocellular carcinoma (HCC), but its postoperative recurrence rate is high, its prognosis is poor, and reliable predictive indicators are lacking. This study was conducted to develop a simple, practical, and effective predictive model.
Materials and Methods: Preoperative clinical and postoperative pathological data on patients with HCC undergoing partial hepatectomies at the Third Affiliated Hospital of Soochow University from January 2010 to December 2015 were retrospectively analyzed, and a nomogram was constructed. The model performance was evaluated using C-indexes, receiver operating characteristic curves, and calibration curves. The results were verified from validation cohort data collected at the same center from January 2016 to January 2017 and compared with the traditional staging systems.
Results: Three hundred three patients were enrolled in this study: 238 in the training cohort and 65 in the validation cohort. From the univariate and multivariate Cox regression analyses in the training cohort, six independent risk factors, i.e., age, alpha-fetoprotein (AFP), tumor size, satellite nodules, systemic immune inflammation index (SII), and prognostic nutritional index (PNI), were filtered and included in the nomogram. The C-index was 0.701 [95% confidence interval (CI): 0.654–0.748] in the training cohort and 0.705 (95% CI: 0.619–0.791) in the validation cohort. The areas under the curve for the 1- and 3-year recurrence-free survival were 0.706 and 0.716 in the training cohort and 0.686 and 0.743 in the validation cohort, respectively. The calibration curves showed good agreement. Compared with traditional American Joint Committee on Cancer 8th edition (AJCC8th) and Barcelona Clinic Liver Cancer (BCLC) staging systems, our nomogram showed better predictive ability.
Conclusion: Our nomogram is simple, practical, and reliable. According to our nomogram, predicting the risk of recurrence and stratifying HCC patient management will yield the greatest survival benefit for patients.
Hepatocellular carcinoma (HCC) is the sixth most common cancer and the fourth leading cause of cancer-related death worldwide. Approximately 841,000 new cases and 782,000 deaths occur annually, presenting a public health burden (1). Surgery is a potential cure for HCC. However, the recurrence rate can reach 70% at 5 years postsurgery, and two-thirds of recurrences occur within 2 years (2). Traditional staging systems, such as the American Joint Committee on Cancer 8th edition (AJCC8th) and the Barcelona Clinic Liver Cancer (BCLC) staging systems, cannot satisfactorily predict postoperative prognosis (3). As a new clinical prognostic model, nomograms have been explored in several carcinomas (4–6). However, scholars have not reached a unified standard or consensus for a clinical prognosis model of an HCC nomogram. Therefore, a pragmatic and powerful standardized nomogram based on objective measures is needed to predict HCC prognoses.
Nomograms are based on multiple independent risk factors. Previous studies on tumor prognosis have been based primarily on demographic and clinicopathological data. Increasing attention is being given to the relationship between conventional serological indicators and tumor prognosis, including indicators of serum inflammation, serum nutrition, liver function, and coagulation function. Gan et al. (7) reported that fibrinogen and C-reactive protein scores were good prognostic indicators for postoperative patients with HCC. Ho et al. (8) showed that the albumin-bilirubin grade could predict HCC recurrence in patients after surgery.
Here, we constructed a practical and effective nomogram based on conventional prognostic indicators combined with multiple serological indicators to predict the postoperative recurrence of HCC.
We retrospectively collected and analyzed preoperative clinical and postoperative pathological data for patients diagnosed with HCC who underwent partial hepatectomies at the Third Affiliated Hospital of Soochow University between January 2010 and January 2017. All data were collected in our hospital, and all serum indicators were obtained within 1 week before surgery. The inclusion criteria were (I) aged 20–85 years; (II) histopathologically proven HCC; and (III) initial diagnosis rather than recurrent tumors. Exclusion criteria were (I) acute tumor rupture with hemoperitoneum; (II) distant metastasis; (III) positive surgical margins; (IV) mixed cholangiocarcinoma; (V) other concomitant malignant diseases; (VI) perioperative death (A death occurred within 90 days after surgery) or death from other diseases during follow-up; (VII) incomplete data; and (VIII) lost to follow-up. Patients who were included from January 2010 to December 2015 were defined as the training cohort; patients who were included from January 2016 to January 2017 were defined as the validation cohort. The institutional review board of the Third Affiliated Hospital of Soochow University approved the study.
The model indices were calculated as follows. Body mass index was calculated by dividing the weight by the height squared (kg/m2). The neutrophil-to-lymphocyte ratio (NLR) was calculated by dividing the neutrophil count by the lymphocyte count. The lymphocyte-to-monocyte ratio was calculated by dividing the lymphocyte count by the monocyte count. The platelet-to-lymphocyte ratio (PLR) was calculated by dividing the platelet count by the lymphocyte count. The systemic immune inflammation index (SII) was calculated as platelet count × neutrophil count/lymphocyte count (109/L). The systemic inflammatory response index (SIRI) was calculated as monocyte count × neutrophil count/lymphocyte count (109/L). The prognostic nutritional index (PNI) was the sum of serum albumin (g/L) and 5 × lymphocyte count (109/L).
All patients were advised to receive regular follow-up according to clinical guidelines after surgery. The outpatient review was conducted every 3 months for the first 2 postoperative years, then every 6 months thereafter if no recurrence or metastasis occurred. Serum AFP, liver function, routine blood, and abdominal ultrasound examinations were performed at each follow-up visit. If signs of recurrence were noted, further computed tomography (CT) examinations were conducted; otherwise, CT examinations were performed once every 6 months. The event endpoint was tumor recurrence. All tumor recurrences were diagnosed via CT images. Recurrence-free survival (RFS) was defined as the time interval between the date of surgery and the date that recurrence was diagnosed. The follow-up deadline was January 31, 2020.
Statistical analysis was performed using SPSS 19.0 (IBM Corp). Continuous variables were compared using the Mann–Whitney U-test; categorical variables were compared using chi-square tests. Continuous variables tested in the laboratory were divided into binary variables according to critical values. The cutoff values for age and model indices were obtained via receiver operating characteristic (ROC) curve analysis, and then these continuous variables were converted into binary variables according to cutoff values. Univariate and multivariate Cox regression analyses were used to identify independent risk factors for HCC recurrence in the training cohort. P < 0.05 was considered statistically significant.
A nomogram was constructed based on the multivariate Cox regression analysis results. The concordance index (C-index), ROC curves, and calibration curves were constructed to evaluate the model performance in the training cohort and verify it in the validation cohort. The risk score for recurrence was determined from the nomogram. The median score of the patients in the training cohort was defined as the cutoff value. Patients with values below the cutoff value were considered the low-risk group; patients with values above the cutoff value were considered the high-risk group. RFS was analyzed using the Kaplan–Meier method. The above steps were analyzed in R, version 3.6.0. The main R packages used included “rms,” “foreign,” “survival,” and “survivalROC.”
Three hundred three patients were enrolled in this study: 238 in the training cohort and 65 in the validation cohort. Table 1 lists the detailed demographics, serum indices, pathological characteristics, AJCC8th stage, and BCLC stage of the patients in the two cohorts.
In the training cohort, the average age of the patients was (59.1 ± 11.3) years, 195 (81.9%) cases were male patients, most of them were infected with hepatitis B virus (182 cases, account for 76.5%), the average diameter of the tumors was (5.0 ± 3.0) cm, and 225 (94.5%) patients had a single tumor. Pathology showed that 93.3% of the tumors were low and middle differentiation, 21.4% of them had vascular invasion, and 11.3% of them had satellite nodules. According to the AJCC8th and BCLC stage, early-stage patients accounted for 94.1% (stage I and II) and 53.8% (stage 0 and A), respectively.
In the validation cohort, the average age of the patients was (60.4 ± 11.6) years, and 56 (86.2%) cases were male patients. Similarly, 73.8% of patients were infected with the hepatitis B virus. The average diameter of the tumors was (5.6 ± 3.7) cm, and 61 (93.8%) patients had a single tumor. Pathology showed that 96.9% of the tumors were low and middle differentiation, 13.8% of them had vascular invasion, and 24.6% of them had satellite nodules. According to the AJCC8th and BCLC stage, early-stage patients accounted for 92.3 and 58.5%, respectively.
Compared with the validation cohort, the serum bilirubin (11.4 ± 5.0 vs.14.1 ± 7.7 μmol/L, P = 0.009), albumin (38.3 ± 5.1 vs. 40.2 ± 4.3 g/L, P = 0.008), neutrophil counts (3.5 ± 1.8 vs. 4.1 ± 2.2 × 109/L, P = 0.014), and tumor satellite nodules (11.3% vs. 24.6%, P = 0.007) in the training cohort were significantly lower. There was no significant difference in age, sex, BMI, hepatitis B virus infection, serum AFP, alanine aminotransferase (ALT), leukocyte count, lymphocyte count, monocyte count, platelet count, and the size, number, differentiation, vascular invasion, AJCC8th, and BCLC stage of tumors between the two groups.
In the training cohort, the median follow-up time was 36.9 months (range, 0.9–120.8 months), and the postoperative 1- and 3-year RFS were 70.6 and 50.8%, respectively. In the validation cohort, the median follow-up time was 36.4 months (range, 1.3–48.6 months), and the postoperative 1- and 3-year RFS were 66.2 and 53.8%, respectively. Figure 1 shows the RFS curves for both groups.
Figure 1. Kaplan–Meier estimate of the postoperative RFS of patients with HCC in the training cohort (A) and validation cohort (B).
In the training cohort, the optimal cutoff values for age, NLR, MLR, PLR, SII, SIRI, PNI, and tumor size were determined to be 67 years and 2.71, 0.26, 78.24, 279.29, 0.93, 48.05, and 6.5 cm, respectively, as per the ROC curves. All variables were then divided into categorical variables and analyzed via univariate and multivariate Cox regression analysis. Table 2 shows the univariate and multivariate Cox regression analysis results. Univariate analyses revealed that age (P = 0.006), AFP (P = 0.002), SII (P = 0.03), PNI (P = 0.015), tumor size (P = 0.003), and satellite nodules (P = 0.003) were identified as significant prognostic factors for HCC recurrence. In multivariate analysis, age [hazard ratio (HR): 1.710; 95% confidence interval (CI): 1.083–2.702; P = 0.021], AFP (HR: 1.498; 95% CI: 1.041–2.156; P = 0.03), SII (HR: 1.456; 95% CI: 1.034–2.051; P = 0.031), PNI (HR: 1.503; 95% CI: 1.016–2.223; P = 0.041), tumor size (HR: 1.621; 95% CI: 1.109–2.369; P = 0.013), and satellite nodules (HR: 1.829; 95% CI: 1.140–2.933; P = 0.012) were identified as independent risk factors for HCC recurrence.
From the multivariate Cox regression analysis results, age, AFP, tumor size, satellite nodules, SII, and PNI were integrated and used to construct the nomogram (Figure 2), which showed that satellite nodules had the greatest impact on HCC recurrence, followed by age, tumor size, PNI, AFP, and SII. The total scores of these six prognostic factors can be used to determine the probabilities of 1- and 3-year RFS.
Figure 2. Nomogram predicting 1- and 3-year RFS probabilities of patients with HCC. Each risk factor was assigned a point according to the nomogram. The exact values of each factor are age (0, 89 points), AFP (0, 67 points), tumor size (0, 80 points), satellite nodules (0, 100 points), SII (0, 62 points), and PNI (0, 68 points). The total points were obtained by adding the points of all risk factors. Then, the 1- and 3-year RFS could be predicted based on the total points.
The performance of the nomogram in the training cohort was evaluated using the C-index, ROC curves, and calibration curves. The C-index for RFS prediction was 0.701 (95% CI: 0.654–0.748). The areas under the ROC curve (AUCs) for the 1- and 3-year RFS were 0.706 and 0.716, respectively (Figures 3A,B). The calibration curves for the probability of 1- and 3-year RFS after surgery showed good probability consistencies between the nomogram prediction and actual observation (Figures 4A,B).
Figure 3. ROC curve evaluating predictive performance of the nomogram for 1-year RFS (A) and 3-year RFS (B) in the training cohort and 1-year RFS (C) and 3-year RFS (D) in the validation cohort.
Figure 4. Calibration curve for predicting 1-year RFS (A) and 3-year RFS (B) in the training cohort and 1-year RFS (C) and 3-year RFS (D) in the validation cohort. The nomogram-predicted probability of RFS is plotted on the x-axis; the actual RFS is plotted on the y-axis.
Using the same method, we verified the predictive ability of the nomogram in the validation cohort. The results showed that the C-index was 0.705 (95% CI: 0.619–0.791). The AUCs of the 1- and 3-year RFS were 0.686 and 0.743, respectively (Figures 3C,D). The calibration curves also had good prediction consistency (Figures 4C,D).
First, we divided the patients into the low-risk and high-risk groups based on the median nomogram score (218.5 points) for the training cohort. The RFS was then analyzed using the Kaplan–Meier method. The RFS survival probability of the high-risk group was significantly lower than that of the low-risk group in both the training and validation cohorts (Figures 5A,D). RFS analysis was performed using the same method based on the AJCC8th and BCLC staging systems. RFS survival probability differed significantly between stages according to the AJCC8th and BCLC staging systems in the validation cohort (Figures 5E,F) but not in the training cohort (Figures 5B,C). Their predictive abilities were compared by calculating the C-index (Table 3). In the training cohort, the C-index of the nomogram (0.701; 95% CI: 0.654–0.748) was higher than that of the AJCC8th (0.533) and BCLC (0.548) staging systems. In the validation cohort, the nomogram performance (C-index: 0.705; 95% CI: 0.619–0.791) was also superior to the AJCC8th (0.672) and BCLC (0.684) staging systems. Therefore, our nomogram better predicted the RFS for HCC patients.
Figure 5. Kaplan–Meier survival curves of RFS for different models. (A–C) Training cohort: (A) nomogram, (B) AJCC8th staging system, and (C) BCLC staging system. (D–F) Validation cohort: (D) nomogram, (E) AJCC8th staging system, and (F) BCLC staging system.
For resectable HCC, surgery is the best treatment option. However, high recurrence rates after hepatectomies greatly reduce patients’ long-term survival benefit. In the training cohort of this study, the 1- and 3-year HCC recurrence rates were 29.4 and 49.2%, respectively. Therefore, the risk of postoperative HCC recurrence must be predicted and stratified to allow early intervention for high-risk patients. At present, the internationally recognized and widely used systems include the AJCC, BCLC, Italian Liver Cancer Program, and Okuda staging systems. However, these traditional liver cancer staging systems have not achieved satisfactory results in predicting the prognosis (3, 9). The nomogram is a prognostic prediction map based on multiple independent risk factors. It has been researched in a variety of tumors, and several studies have reported that its prediction performance is better than that of traditional liver cancer staging systems (10, 11).
In this study, we established a nomogram based on SII and PNI. The nomogram showed good performance for predicting prognoses as per C-index, ROC curve, calibration curve, and internal cohort verification. The nomogram also exhibited better prognostic predictive ability compared with the traditional AJCC and BCLC staging systems.
Studies have shown that systemic immune inflammatory responses are significantly correlated with cancers (12, 13). Neutrophils in the peripheral blood or tumor microenvironment can produce angiogenic factors that stimulate tumor development and progression (14). Lymphocytes reflect the host immunity status and can inhibit tumor progression (15). Therefore, cancer-related inflammation is considered the seventh hallmark of cancer (16). Recent studies have mainly focused on the inflammation index converted from multiple serum inflammatory indicators, such as the NLR, MLR, PLR, SII, and SIRI, modified Glasgow prognostic score (mGPS), and C-reactive protein/albumin ratio (CAR). Nomogram clinical models based on inflammatory indicators such as NLR, PLR, hypersensitive C-reactive protein (hs-CRP), and FC-score composed of fibrinogen and C-reactive protein are reported to have good predictive prognostic performances for HCC (7, 17–19). In this study, several easily available inflammatory indicators were analyzed, including NLR, PLR, MLR, SIRI, and SII. Although the prognostic ability of CAR in HCC is reportedly better than that of other inflammatory indexes (10), C-reactive protein is not used in routine preoperative examinations in our center; thus, it was excluded from the study, as was the mGPS. Our results indicated that only the inflammatory index SII was an independent risk factor for postoperative HCC recurrence. Fu et al. (20) reported that preoperative SII was a powerful prognostic biomarker in HCC patients who undergo liver transplantation, and it was superior to PLR, NLR, and MLR for prediction of overall survival. Jomrich et al. (21) also showed that SII was superior to PLR and NLR for predicting overall survival in pancreatic ductal adenocarcinoma patients. Therefore, SII was a convenient, low-cost, and effective inflammatory indicator to predict the prognosis of tumors. Previous studies have only reported the use of SII in nomograms for gastric and tongue cancer (22, 23). Here, we report for the first time that nomograms based on SII can effectively predict postoperative HCC recurrence.
Relationships between nutritional status and cancer prognosis have gained attention from researchers over the last decade. Studies have shown that malnutrition often leads to poor tumor prognosis (24). In studying the HCC clinical nomogram model, albumin-based nutritional indexes, including the albumin-bilirubin grade and lactic dehydrogenase/albumin ratio, showed good prognostic predictive performance (25, 26). In this study, we explored the albumin-based PNI. The PNI was originally designed to assess the nutritional status of patients undergoing gastrointestinal surgery (27). Pinato et al. (28) found for the first time that the PNI independently predicted overall survival of HCC patients. Our results also indicated that low PNI (≤48.05) was an independent risk factor for postoperative HCC recurrence, and our PNI-based nomogram showed good prediction performance. Cancer cachexia is reportedly driven by a sustained inflammatory response (29). Therefore, we believe that inflammation and malnutrition jointly promote tumor progression; thus, our nomogram comprehensively includes both inflammation and nutrition indexes.
Our nomogram also included four common factors: age, serum AFP, tumor size, and satellite nodules; for these, we included demographic characteristics, serum indicators, and clinicopathological data to optimize the overall predictive ability.
At present, there was no uniform standard for predicting the prognosis of HCC. The AJCC and BCLC staging systems were the most common staging systems for liver cancer and were primarily meant to formulate patient’s treatment plans in oncology settings. In recent studies, Kee and Chun et al. (9, 30) reported that the AJCC staging system had a predictive ability for the prognosis of HCC. Grieco et al. (31) reported that the BCLC staging system gave a better prediction of prognosis in patients with HCC at a very early stage. Kim et al. (32) also found that the BCLC staging system was the best long-term prognostic model for treatment-naive HCC in a large scale Korean cohort. These reports indicated that AJCC and BCLC staging systems had predictive value for the prognosis of HCC. So, we compared the AJCC8th and BCLC staging systems with our nomogram model in our study. Combining the above six independent risk factors, our nomogram model showed a better predictive ability for postoperative recurrence of HCC than the AJCC8th and BCLC staging systems.
According to our nomogram, we can assess the risk of recurrence of HCC after surgery and conduct early individualized interventions for high-risk patients. We think that early interventions mainly include the following aspects: First, etiological interventions are critical, such as anti-hepatitis B virus, alcohol withdrawal, etc. Second, early postoperative transarterial chemoembolization (TACE) may play a role in preventing tumor recurrence, especially for patients with large and multiple lesions. Third, early postoperative immunoregulatory therapy may be a strategy, such as programmed cell death protein 1 (PD-1) inhibitor, thymosin, interferon, etc. Fourth, the detection of gene targets should be conducted after surgery, and targeted therapy may bring survival benefits to suitable patients. The early implementation of these methods may reduce the risk of tumor recurrence to a certain extent.
This study also had several limitations. First, this was a retrospective study. Second, our nomogram performance was verified with an internal cohort, without external or multicenter verification. Third, the number of patients enrolled in the model was relatively small. Therefore, further large-sample, multicenter, and prospective verification studies are needed. Besides, the exclusion of patients who were lost to follow-up was the main bias in this study.
In conclusion, our nomogram based on SII and PNI is simple, practical, and reliable. It has a good predictive ability for postoperative HCC recurrence. Our nomogram suggests that predicting the risk of recurrence and stratifying the management of HCC patients will yield the greatest survival benefit for patients.
The raw data supporting the conclusions of this article will be made available by the authors, without undue reservation.
The studies involving human participants were reviewed and approved by the institutional review board of the Third Affiliated Hospital of Soochow University. Written informed consent for participation was not required for this study in accordance with the national legislation and the institutional requirements.
DS conceived and designed the study. JY collected the data and wrote the manuscript. YB analyzed the data. YD and WC modified the manuscript. All co-authors have reviewed and approved this version of the manuscript. All authors contributed to the article and approved the submitted version.
This study was supported by the National Natural Science Foundation of China (Grant Number 81602054).
The authors declare that the research was conducted in the absence of any commercial or financial relationships that could be construed as a potential conflict of interest.
All authors are grateful to all the patients who contributed data to this study.
1. Bray F, Ferlay J, Soerjomataram I, Siegel RL, Torre LA, Jemal A. Global cancer statistics 2018: GLOBOCAN estimates of incidence and mortality worldwide for 36 cancers in 185 countries. CA Cancer J Clin. (2018) 68:394–424. doi: 10.3322/caac.21492
2. Kulik L, El-Serag HB. Epidemiology and management of hepatocellular carcinoma. Gastroenterology. (2019) 156:477–491.e471. doi: 10.1053/j.gastro.2018.08.065
3. Huitzil-Melendez FD, Capanu M, O’reilly EM, Duffy A, Gansukh B, Saltz LL, et al. Advanced hepatocellular carcinoma: which staging systems best predict prognosis? J Clin Oncol. (2010) 28:2889–95. doi: 10.1200/JCO.2009.25.9895
4. Chen SH, Wan QS, Zhou D, Wang T, Hu J, He YT, et al. A simple-to-use nomogram for predicting the survival of early hepatocellular carcinoma patients. Front Oncol. (2019) 9:584. doi: 10.3389/fonc.2019.00584
5. Chen M, Cao J, Zhang B, Pan L, Cai X. A nomogram for prediction of overall survival in patients with node-negative gallbladder cancer. J Cancer. (2019) 10:3246–52. doi: 10.7150/jca.30046
6. Yu C, Zhang Y. Development and validation of prognostic nomogram for young patients with gastric cancer. Ann Transl Med. (2019) 7:641. doi: 10.21037/atm.2019.10.77
7. Gan W, Yi Y, Fu Y, Huang J, Lu Z, Jing C, et al. Fibrinogen and C-reactive protein score is a prognostic index for patients with hepatocellular carcinoma undergoing curative resection: a prognostic nomogram study. J Cancer. (2018) 9:148–56. doi: 10.7150/jca.22246
8. Ho SY, Hsu CY, Liu PH, Hsia CY, Su CW, Huang YH, et al. Albumin-bilirubin (ALBI) grade-based nomogram to predict tumor recurrence in patients with hepatocellular carcinoma. Eur J Surg Oncol. (2019) 45:776–81. doi: 10.1016/j.ejso.2018.10.541
9. Chun YH, Kim SU, Park JY, Kim DY, Han KH, Chon CY, et al. Prognostic value of the 7th edition of the AJCC staging system as a clinical staging system in patients with hepatocellular carcinoma. Eur J Cancer. (2011) 47:2568–75. doi: 10.1016/j.ejca.2011.07.002
10. Chen J, Fang A, Chen M, Tuoheti Y, Zhou Z, Xu L, et al. A novel inflammation-based nomogram system to predict survival of patients with hepatocellular carcinoma. Cancer Med. (2018) 7:5027–35. doi: 10.1002/cam4.1787
11. Xu L, Peng ZW, Chen MS, Shi M, Zhang YJ, Guo RP, et al. Prognostic nomogram for patients with unresectable hepatocellular carcinoma after transcatheter arterial chemoembolization. J Hepatol. (2015) 63:122–30. doi: 10.1016/j.jhep.2015.02.034
12. Ringelhan M, Pfister D, O’connor T, Pikarsky E, Heikenwalder M. The immunology of hepatocellular carcinoma. Nat Immunol. (2018) 19:222–32. doi: 10.1038/s41590-018-0044-z
13. Diakos CI, Charles KA, Mcmillan DC, Clarke SJ. Cancer-related inflammation and treatment effectiveness. Lancet Oncol. (2014) 15:e493–503. doi: 10.1016/S1470-2045(14)70263-3
14. Dings RP, Nesmelova I, Griffioen AW, Mayo KH. Discovery and development of anti-angiogenic peptides: a structural link. Angiogenesis. (2003) 6:83–91. doi: 10.1023/B:AGEN.0000011730.94233.06
15. Dunn GP, Old LJ, Schreiber RD. The immunobiology of cancer immunosurveillance and immunoediting. Immunity. (2004) 21:137–48. doi: 10.1016/j.immuni.2004.07.017
16. Hanahan D, Weinberg RA. Hallmarks of cancer: the next generation. Cell. (2011) 144:646–74. doi: 10.1016/j.cell.2011.02.013
17. Chon YE, Park H, Hyun HK, Ha Y, Kim MN, Kim BK, et al. Development of a new nomogram including neutrophil-to-lymphocyte ratio to predict survival in patients with hepatocellular carcinoma undergoing transarterial chemoembolization. Cancers (Basel). (2019) 11:509. doi: 10.3390/cancers11040509
18. Li X, Huang H, Yu X, Chen P, Ouyang J, Huang B. A novel prognostic nomogram based on microvascular invasion and hematological biomarkers to predict survival outcome for hepatocellular carcinoma patients. Surg Oncol. (2020) 33:51–7. doi: 10.1016/j.suronc.2020.01.006
19. Jing CY, Fu YP, Zheng SS, Yi Y, Shen HJ, Huang JL, et al. Prognostic nomogram for patients with hepatocellular carcinoma underwent adjuvant transarterial chemoembolization following curative resection. Medicine (Baltimore). (2017) 96:e6140. doi: 10.1097/MD.0000000000006140
20. Fu H, Zheng J, Cai J, Zeng K, Yao J, Chen L, et al. Systemic immune-inflammation index (SII) is useful to predict survival outcomes in patients after liver transplantation for hepatocellular carcinoma within hangzhou criteria. Cell Physiol Biochem. (2018) 47:293–301. doi: 10.1159/000489807
21. Jomrich G, Gruber E, Winkler D, Hollenstein M, Gnant M, Sahora K, et al. Systemic immune-inflammation index (SII) predicts poor survival in pancreatic cancer patients undergoing resection. J Gastroint Surgery. (2020) 24:610–8. doi: 10.1007/s11605-019-04187-z
22. Shi H, Jiang Y, Cao H, Zhu H, Chen B, Ji W. Nomogram based on systemic immune-inflammation index to predict overall survival in gastric cancer patients. Dis Markers. (2018) 2018:1787424. doi: 10.1155/2018/1787424
23. Lu Z, Yan W, Liang J, Yu M, Liu J, Hao J, et al. Nomogram based on systemic immune-inflammation index to predict survival of tongue cancer patients who underwent cervical dissection. Front Oncol. (2020) 10:341. doi: 10.3389/fonc.2020.00341
24. Wu N, Chen G, Hu H, Pang L, Chen Z. Low pretherapeutic serum albumin as a risk factor for poor outcome in esophageal squamous cell carcinomas. Nutr Cancer. (2015) 67:481–5. doi: 10.1080/01635581.2015.1004726
25. He W, Peng B, Tang Y, Yang J, Zheng Y, Qiu J, et al. Nomogram to predict survival of patients with recurrence of hepatocellular carcinoma after surgery. Clin Gastroenterol Hepatol. (2018) 16:756–64. doi: 10.1016/j.cgh.2017.12.002
26. Gan W, Zhang MX, Wang JX, Fu YP, Huang JL, Yi Y, et al. Prognostic impact of lactic dehydrogenase to albumin ratio in hepatocellular carcinoma patients with Child-Pugh I who underwent curative resection: a prognostic nomogram study. Cancer Manag Res. (2018) 10:5383–94. doi: 10.2147/CMAR.S176317
27. Nozoe T, Kimura Y, Ishida M, Saeki H, Korenaga D, Sugimachi K. Correlation of pre-operative nutritional condition with post-operative complications in surgical treatment for oesophageal carcinoma. Eur J Surg Oncol. (2002) 28:396–400. doi: 10.1053/ejso.2002.1257
28. Pinato DJ, North BV, Sharma R. A novel, externally validated inflammation-based prognostic algorithm in hepatocellular carcinoma: the prognostic nutritional index (PNI). Br J Cancer. (2012) 106:1439–45. doi: 10.1038/bjc.2012.92
29. Esper DH, Harb WA. The cancer cachexia syndrome: a review of metabolic and clinical manifestations. Nutr Clin Pract. (2005) 20:369–76. doi: 10.1177/0115426505020004369
30. Kee K, Wang J, Lee C, Chen C, Changchien C, Hu T, et al. Validation of clinical AJCC/UICC TNM staging system for hepatocellular carcinoma: analysis of 5,613 cases from a medical center in southern Taiwan. Int J Cancer. (2007) 120:2650–5. doi: 10.1002/ijc.22616
31. Grieco A, Pompili M, Caminiti G, Miele L, Covino M, Alfei B, et al. Prognostic factors for survival in patients with early-intermediate hepatocellular carcinoma undergoing non-surgical therapy: comparison of Okuda, CLIP, and BCLC staging systems in a single Italian centre. Gut. (2005) 54:411–8. doi: 10.1136/gut.2004.048124
32. Kim B, Kim S, Park J, Kim D, Ahn S, Park M, et al. Applicability of BCLC stage for prognostic stratification in comparison with other staging systems: single centre experience from long-term clinical outcomes of 1717 treatment-naïve patients with hepatocellular carcinoma. Liver Int. (2012) 32:1120–7. doi: 10.1111/j.1478-3231.2012.02811.x
Keywords: hepatocellular carcinoma, recurrence, nomogram, SII, PNI
Citation: Yang J, Bao Y, Chen W, Duan Y and Sun D (2020) Nomogram Based on Systemic Immune Inflammation Index and Prognostic Nutrition Index Predicts Recurrence of Hepatocellular Carcinoma After Surgery. Front. Oncol. 10:551668. doi: 10.3389/fonc.2020.551668
Received: 16 April 2020; Accepted: 21 September 2020;
Published: 14 October 2020.
Edited by:
Gianluca Vanni, University of Rome Tor Vergata, ItalyReviewed by:
Domenico Tamburrino, San Raffaele Hospital (IRCCS), ItalyCopyright © 2020 Yang, Bao, Chen, Duan and Sun. This is an open-access article distributed under the terms of the Creative Commons Attribution License (CC BY). The use, distribution or reproduction in other forums is permitted, provided the original author(s) and the copyright owner(s) are credited and that the original publication in this journal is cited, in accordance with accepted academic practice. No use, distribution or reproduction is permitted which does not comply with these terms.
*Correspondence: Donglin Sun, Y3p5eXNkbEAxNjMuY29t
Disclaimer: All claims expressed in this article are solely those of the authors and do not necessarily represent those of their affiliated organizations, or those of the publisher, the editors and the reviewers. Any product that may be evaluated in this article or claim that may be made by its manufacturer is not guaranteed or endorsed by the publisher.
Research integrity at Frontiers
Learn more about the work of our research integrity team to safeguard the quality of each article we publish.