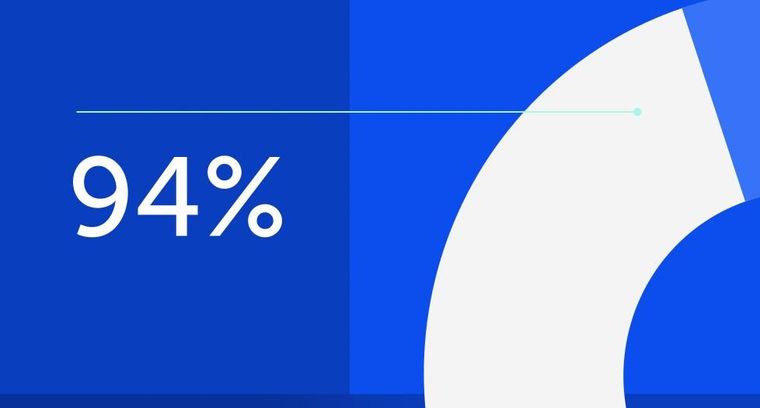
94% of researchers rate our articles as excellent or good
Learn more about the work of our research integrity team to safeguard the quality of each article we publish.
Find out more
ORIGINAL RESEARCH article
Front. Oncol., 16 November 2020
Sec. Cancer Imaging and Image-directed Interventions
Volume 10 - 2020 | https://doi.org/10.3389/fonc.2020.523543
This article is part of the Research TopicInnovations in Imaging for Early Diagnosis and Monitoring for Patients with Gastrointestinal CancerView all 29 articles
Objectives: To develop a radiomics signature for predicting surgical portal vein-superior mesenteric vein (PV-SMV) in patients with pancreatic ductal adenocarcinoma (PDAC) and measure the effect of providing the predictions of radiomics signature to radiologists with different diagnostic experiences during imaging interpretation.
Methods: Between February 2008 and June 2020, 146 patients with PDAC in pancreatic head or uncinate process from two institutions were retrospectively included and randomly split into a training (n = 88) and a validation (n =58) cohort. Intraoperative vascular exploration findings were used to identify surgical PV-SMV invasion. Radiomics features were extracted from the portal venous phase CT images. Radiomics signature was built with a linear elastic-net regression model. Area under receiver operating characteristic curve (AUC) of the radiomics signature was calculated. A senior and a junior radiologist independently review CT scans and made the diagnosis for PV-SMV invasion both with and without radiomics score (Radscore) assistance. A 2-sided Pearson’s chi-squared test was conducted to evaluate whether there was a difference in sensitivity, specificity, and accuracy between the radiomics signature and the unassisted radiologists. To assess the incremental value of providing Radscore predictions to the radiologists, we compared the performance between unassisted evaluation and Radscore-assisted evaluation by using the McNemar test.
Results: Numbers of patients identified as presence of surgical PV-SMV invasion were 33 (37.5%) and 19 (32.8%) in the training and validation cohort, respectively. The radiomics signature achieved an AUC of 0.848 (95% confidence interval, 0.724–0.971) in the validation cohort and had a comparable sensitivity, specificity, and accuracy as the senior radiologist in predicting PV-SMV invasion (all p-values > 0.05). Providing predictions of radiomics signature increased both radiologists’ sensitivity in identifying PV-SMV invasion, while only the increase of the junior radiologist was significant (63.2 vs 89.5%, p-value = 0.025) instead of the senior radiologist (73.7 vs 89.5%, p-value = 0.08). Both radiologists’ accuracy had no significant increase when provided radiomics signature assistance (both p-values > 0.05).
Conclusions: The radiomics signature can predict surgical PV-SMV invasion in patients with PDAC and may have incremental value to the diagnostic performance of radiologists during imaging interpretation.
Pancreatic ductal adenocarcinoma (PDAC) is a lethal disease, and the five-year survival rate is lower than 8% (1–3). Although surgery remains the only potential chance for a cure, some patients with localized PDAC are not appropriated for upfront surgery or even unresectable due to the involvement of peripancreatic vessels (3–8). Regarding patients with peripancreatic arterial involvement, upfront surgery is known to be associated with a low resection rate and a deteriorated long-term survival (4, 5). In contrast, for patients with isolated peripancreatic venous [portal vein-superior mesenteric vein (PV-SMV)] involvement, long-term survival after extended pancreaticoduodenectomy (PD) with venous resection may be comparable to that achieved by standard PD without venous resection (6–8). However, the determination of surgical PV-SMV invasion is still based on intraoperative diagnosis (9). Preoperative knowledge of PV-SMV invasion status can promote adequate preoperative preparation, which may mitigate the positive margins associated with unplanned PV-SMV resection, decrease unresectable events due to the inexperience of extended PD, and reduce surgery-related complications (6–8, 10).
CT is commonly used to assess possible vascular involvement and plays a significant role in surgical planning (11, 12). Existing radiological classifications for classifying vascular involvement are based on the presence and degree of tumor contact with the vessel (11–14). Several imaging features for evaluating surgical PV-SMV invasion have been introduced, including encasement (>180°) of the tumor-vein relationship (13), deformation, narrowed or stenotic morphology of PV-SMV (14), and the teardrop sign (15). Unfortunately, such above qualitative imaging findings do not accurately classify vascular involvement, especially in peripancreatic venous involvement (16–18). Also, a recent study showed that agreements in the interpretation of tumor-vascular relationships were low among different observers (19).
Radiomics (20, 21) is a data-centric field that processes radiological imaging data by extracting large amounts of quantitative image features, which are subsequently employed to construct novel imaging biomarkers, namely radiomics signature. Previous radiomics studies on PDAC (22–25) have indicated that quantitative image features were closely related to adverse pathological features, therapeutic response, and prognosis after neoadjuvant therapy. However, radiomics research on distinguishing surgical PV-SMV invasion in patients with PDAC is lacking. Furthermore, it is unknown whether radiomics signature could be used as a supplement to radiological classification for PV-SMV invasion.
Therefore, this study aimed to develop a radiomics signature for classifying surgical PV-SMV invasion and to compare performance of the radiomics signature to that of radiologists. In addition, we evaluate changes in diagnostic performance of the radiologists when predictions of radiomics signature are provided during interpretation.
This study was approved by the Institutional Review Board of the Affiliated Wuxi No.2 People’s Hospital of Nanjing Medical University and Wuxi No.5 People’s Hospital. The need to obtain informed consent was waived.
From May 2008 to June 2020 in institution 1 (The Affiliated Wuxi No.2 People’s Hospital of Nanjing Medical University) and October 2017 to June 2020 in institution 2 (Wuxi No.5 People’s Hospital), consecutive patients who were treated with surgery and pathologically confirmed PDAC were included. In the institutions, upfront resection, rather than neoadjuvant treatment, was recommended in borderline resectable PDAC with isolated venous involvement. The inclusion criteria were as follows: (1) PDAC in pancreatic head or uncinate process; (2) patients who had a complete intraoperative peripancreatic vessel exploration record; and (3) no artery invasion or distant metastases in intraoperative exploration. The exclusion criteria were as follows: (1) no enhanced CT images or poor image quality; (2) preoperative enhanced CT examination performed more than 4 weeks before the surgery; (3) tumors not visible on CT image; and (4) patients had undergone neoadjuvant therapy before surgery. Ultimately, 146 patients with PDAC were included in this study (Figure 1). The patient’s numbers of institutions 1 and 2 were 125 and 21, respectively. Patients were randomly split to a training (n = 88) and a validation cohort (n = 58) according to a ratio of 3:2 using stratified random sampling.
In this study, surgical PV-SMV invasion status in PDAC was determined by the findings of intraoperative exploration. Intraoperative appearances of the interface between tumor and vascular were classified as the following types (8, 9): (1) no adherence; (2) adhering but separable; and (3) inseparable. Type 1 or 2 was defined as absence of surgical PV-SMV invasion; type 3 was defined as presence of surgical PV-SMV invasion. All the above procedures were performed by a team of surgeons with at least 10 years of experience (approximately 50 pancreatectomy surgeries annually per surgeon).
Multiphasic CT was performed by following a pancreatic protocol included unenhanced and contrast-enhanced dual-phasic imaging of the pancreatic parenchymal phase (40–50s) and portal venous phases (65–70s). Images were reconstructed at submillimeter (0.5–1.0mm) thickness in the axial for pancreatic parenchymal and portal venous phase images. Multi-planar reformation and maximal intensity projection reconstructed images of vascular structures were routinely created by radiology technologists and were sent to the Picture Archiving and Communication System (PACS) for interpretation. The pancreatic parenchymal phase produces optimal visual contrast differences between the enhanced pancreatic parenchyma and the tumor; the portal venous phase allows for better evaluation of PV-SMV since the portomesenteric venous system is well enhanced. CT scanners and detailed CT parameters are provided in the Appendix E1.
Tumor segmentation was performed using the ITK-SNAP 3.8.0 (http://www.itksnap.org). A radiologist (FM Chen, 12-year experience in abdominal imaging) selected the slice with the maximum tumor-vein contact on the portal venous phase images and delineated the tumor. The pancreatic parenchymal phase was used to aid determination of tumor boundaries. Another radiologist (B Li, 13-year experience in abdominal imaging) delineated the tumor on a randomly selected cohort of 50 patients following the same procedure.
Image preprocessing and feature extraction were performed using pyradiomics (Version 2.1, https://pyradiomics.readthedocs.io/en/latest/index.html) (21). Images were resampled to a pixel spacing of 1×1 mm2. Intensities were discretized with a fixed bin-width of 25 Hounsfield units. Features in 3 categories were extracted from the original images, included 9 shape-based features, 18 first-order features, and 86 grey-level-matrix-based features. The first-order features and grey-level-matrix-based features were additionally extracted from different image transformations, including four wavelet decompositions and five Laplacian of Gaussian filters (sigma = 1.0, 2.0, 3.0, 4.0, and 5.0 mm). A total of 869 features were extracted (detailed in Appendix E2 and Table S1). Each feature value was normalized by the z-score method, which consisted of subtracting the mean value of feature and dividing by the standard deviation of the feature.
The radiomics features were calculated for each radiologist’s delineation, and the intraclass correlation coefficient (ICC) of each feature was calculated to test the inter-observer reproducibility. The features with an ICC≥0.8 were proceeded to subsequent analyses. The linear elastic-net regression was used for feature selection and radiomics signature building. In the hyper-parameter tuning of linear elastic-net regression (26), the α penalty was set to 0.5 following a grid search with the penalty parameter λ determined by 5-fold cross-validation. The built radiomics signature provides a mathematical formula that predicts PV-SMV invasion by using the selected radiomics features with the equation:
In which is the radiomics score (Radscore), b is the intercept, βi is the coefficient of the feature i, and Xi is the value of the feature i.
There were two readings performed in this study. First, blinded to clinical information, surgical findings, and the Radscore but knowing patients were diagnosed as PDAC, two radiologists (L Zhang, a senior radiologist with 18-year experience in abdominal imaging, and SL. Zhang, a junior radiologist with 5-year experience in abdominal imaging) independently reviewed the CT scans of all patients. Then, after a washout period of 2 weeks, the two radiologists independently reviewed the CT scans of patients in the validation cohort with Radscore assistance but still blinded to the clinical information and surgical findings. For each reading, radiologists were asked to document the following three imaging features:
(1) Tumor was in contact with the PV-SMV for more than 180° (13); (2) PV-SMV blood vessel morphology was deformed, narrowed, or stenotic (14); and (3) PV-SMV was deformed, demonstrating a teardrop shape on axial image (15).
In the first reading, PV-SMV invasion was determined as presence when any one of the above imaging features was present.
In the second reading, the radiologists had been informed of the radiomics-signature-predicted probability of PV-SMV invasion before documenting the imaging features; PV-SMV invasion was also determined as presence when any one of the above imaging features was present. Flowchart of the study is shown in Figure 2.
Figure 2 Flowchart of the study. (A) Radiomics workflow, including ROI segmentation, feature extraction, radiomics signature construction, and validation. (B) Radiologists assessment. Performance of each radiologist between unassisted evaluation and Radscore-assisted evaluation was compared in the validation cohort. Radscore, radiomics score.
In statistical tests of clinical features, the Mann-Whitney test was used for continuous variables, and Fisher’s exact test was used for categorical variables. The performance of the radiomics signature was assessed using area under receiver operating characteristic curve (AUC). The optimal cutoff value of Radscore was selected by maximizing the Youden index (sensitivity + specificity − 1). The sensitivity, specificity, and accuracy of the radiomics signature and the radiologists (with or without Radscore assistance) were also reported. A two-sided Pearson’s chi-squared test was used to compare the performance of the Radscore to that of the radiologists. To assess the incremental value of providing Radscore predictions to the radiologists, we compared the performance measures between unassisted evaluation and Radscore-assisted evaluation by using the McNemar test. All comparisons were performed in the validation cohort. Interobserver agreement between the senior and the junior radiologists (with and without Radscore assistance) was evaluated using the Kappa (κ) test: κ-value of 0.2 to 0.4, fair agreement; κ-value of 0.4 to 0.6, moderate agreement; κ-value of 0.6 to 0.8, substantial agreement; κ-value greater than 0.8, almost perfect agreement.
R (version 3.5.1) statistical software was used for statistical analysis in this study. Glmnet R package was used to perform the linear elastic-net regression. Bilateral p-value < 0.05 was considered statistically significant.
The characteristics of patients are summarized in Table 1. No significant differences were found in clinical and surgical factors between the training and validation cohorts. A total of 33 (37.5%) patients in the training cohort and 19 (32.8%) patients in the validation cohort were confirmed PV-SMV invasion in surgical exploration. For these patients, extended surgery was performed when reconstructable PV-SMV involvement can be achieved, otherwise, palliative surgery was performed.
Among the 869 extracted radiomics features, 751 features with high stability (ICC≥0.8) were identified. The radiomics signature for PV-SMV invasion was developed using the elastic net model (α=0.5, λ=0.174) and retained 10 features, including one morphological feature and 9 texture features (Table 2). The ability of the radiomics signature to discriminate PV-SMV invasion was shown to have an AUC of 0.871 [95% confidence interval (CI) 0.795–0.946] in the training cohort and 0.848 (95% CI 0.724–0.971) in the validation cohort (Figure 3A). Values of Radscores per patient in the training and validation cohorts are plotted in Figure 3B.
Figure 3 Performance of the radiomics signature with operating points of unassisted and assisted radiologists and the value of Radscores per patient. (A) ROC curves of the radiomics signature in the training and validation cohorts. Individual unassisted radiologist (specificity, sensitivity) points are also plotted, where the purple point represents unassisted senior radiologist, and the blue points represents unassisted junior radiologist. In the validation cohort, individual assisted radiologist (specificity, sensitivity) points are also plotted, where the purple circle represents Radscore-assisted senior radiologist, and the blue circle represents Radscore-assisted junior radiologist. (B) Radscore (subtraction of the cut-off determined by maximizing the Youden index) per patient in the training and validation cohorts. ROC, Receiver operating characteristic; Radscore, radiomics score.
The optimal cutoff value of the Radscore was determined at the level of −0.608. Accordingly, sensitivity, specificity, and accuracy of the radiomics signature for differentiating PV-SMV invasion were determined. There were no significant differences in the performance metrics of the radiomics signature and each radiologist (Table 3). The radiomics signature sensitivity (78.9%) for PV-SMV invasion was slightly higher than the radiologists (73.7% of the senior radiologist and 63.2% of the junior radiologist). The Radscore achieved a specificity of 74.4% and an accuracy of 75.9%, while the senior radiologist and the junior radiologist achieved a specificity of 84.6% and 82.1%, and an accuracy of 81.0% and 75.9%, respectively.
Comparison of unassisted and Radscore-assisted performance of each radiologist is illustrated in Figure 3A (right), with numerical values presented in Table 4. To show the changes of diagnosis after the assistance, confusion matrices of each radiologist are shown in Figure 4. When provided Radscore assistance, there was an increase in sensitivity in identifying PV-SMV invasion; for both the junior radiologist (63.2 vs 89.5%, p-value = 0.025) and the senior radiologist (73.7 vs 89.5%, p-value = 0.08). Even though the radiologist’ specificity was slightly decreased (84.6 vs 82.1% for the senior radiologist and 82.1 vs 79.5% for the junior radiologist) when provided Radscore assistance, the accuracy was slightly increased (81.0 vs 84.5% for the senior radiologist and 75.9 vs 85.8% for the junior radiologist), but neither was significant (all p-values > 0.05, Table 4). With Radscore assistance, κ-value of inter-rater reliability increased from 0.571 to 0.757 (both p-values <0.001). Representative cases which were reclassified after Radscore assistance are shown in Figure 5.
Table 4 Comparison of unassisted and assisted performance in each radiologist on the validation set.
Figure 4 Confusion matrices comparing the true labels and the diagnostic labels. Each plot illustrates performance on the validation cohort. left, the senior radiologist’s unassisted evaluation and Radscore-assisted evaluation; right, the junior radiologist’ unassisted evaluation and Radscore-assisted evaluation. Radscore, radiomics score.
Figure 5 Representative cases which were reclassified after Radscore assistance. (A) Axial portal venous phase CT image in a 73-year-old man with PDAC in the pancreatic head. The vein was suspiciously deformed (*). The Radscore prediction gave a high probability of PV-SMV invasion. The interpretation of deformity of the vein between unassisted and Radscore-assisted was discrepant for both radiologists, they assigned PV-SMV invasion category with Radscore assistance. Intraoperative exploration confirmed the diagnosis of PV-SMV invasion. (B) Axial portal venous phase CT image in a 66-year-old female with PDAC in the pancreatic head. The vein was interpreted as teardrop shape by the junior radiologist (*) in first the reading. The Radscore prediction gave a low probability of PV-SMV invasion. The vein was interpreted as absence of teardrop shape by the junior radiologist (*) in the second reading with Radscore assistance, and PV-SMV non-invasion category was assigned. Intraoperative exploration confirmed the diagnosis of PV-SMV non-invasion.
In this study, we developed a Radscore on presurgical pancreatic enhanced CT in classifying surgical PV-SMV invasion of PDAC in the pancreatic head or uncinate process. In addition, we compared performance between unassisted and Radscore-assisted reviews of radiologists with different diagnostic experiences. Our results demonstrated that the Radscore achieved an AUC 0.848 (95% CI 0.724–0.971) for discriminating PV-SMV involvement and had a comparable diagnostic performance as the senior radiologist. We also found that providing predictions of Radscore to the junior radiologist as a diagnostic aid led to significant improvement in sensitivity for identifying surgical PV-SMV invasion.
Accurate estimation of surgical PV-SMV invasion plays a vital role in the perioperative management of patients with PDAC (11, 12). In this study, prediction-related features consisting of the radiomics signature included one shape-based feature (sphericity) and nine texture features. Among them, sphericity is a measure of roundness of the shape of the tumor region and was an important component for predicting PV-SMV invasion. A previous study (27) reported that unfavorable tumor morphology was highly associated with the presence of peripancreatic vessels involvement. Features related to shape were associated with morphology of the tumor region adjacent to the vein on CT images. Moreover, shape-based features are independent of imaging acquisition parameters and imaging preprocessing techniques, and thus may be highly reproducible. In addition, recent studies (22–25) have suggested that texture features indicating inhomogeneity in imaging are associated with increased intra-tumor heterogeneity of PDAC. The results of this study indicated that some texture features may be closely related to adverse tumor biology in PV-SMV involvement.
In this study, the radiomics signature provided individualized predictions of PV-SMV invasion and the delineation of ROI was easy. Several reasons were explaining why we used 2D ROI instead of 3D ROI. First, the focus of our studies was tumor invasion of PV-SMV, which most likely takes place in the tumor-PV-SMV contact region. Second, delineating 3D ROI slice by slice on the submillimeter-thick images was labor-intensive and may decrease reproducibility. We evaluated inter-reader agreement in delineating 2D ROI and found most features (86.5%) were highly stable. We also compared diagnostic performance of radiomics signature and two radiologists with different diagnostic experiences; even though the radiomics signature did not significantly outperform the junior radiologist, it achieved comparable performance as the senior radiologist. Hence, the proposed radiomics signature combing both morphological and texture parameters was a valuable marker for surgical PV-SMV invasion.
Interestingly, though the interobserver agreement between the radiologists was moderate, their sensitivities were both unsatisfactory. The diagnostic performance of the radiologists was concordant with that of similar studies on CT (16–19); about 20% of cases were false negative according to the qualitative imaging features. Though a recent study reported that endoscopic ultrasound elastography could improve diagnostic performance of vascular invasion in PDAC, the elastography technique was not commonly used for all patients (28). As we knew, image interpretation for PDAC peripancreatic vascular invasion was based on visual assessment of tumor-vein relationship. Vascular morphologic changes on visual assessment, such as vascular deformation, narrowing, or teardrop sign, sometimes were difficult to judge the presence of invasion, this could result in low sensitivity of radiologists in detecting PV-SMV invasion. Concordance to the previous study, pancreatitis-related changes usually blur the contact region between the solid tumor and adjacent vessels; this led to discrepancy in the interpretation of the degree of tumor-vascular contact (abutment vs encasement, <180° vs >180°, respectively) and may have caused the interobserver variability (19).
To examine the effect of the Radscore may have on the interpretation performance of radiologists with different experiences, our study compared unassisted and Radscore-assisted performance of each radiologist in the validation cohort. We found a statistically significant improvement in sensitivity of the junior radiologist (p-value=0.025) for discriminating PV-SMV involvement with Radscore assistance and, though no statistically significant increase in accuracy. This was because qualitative features in some cases were relabeled by radiologists with Radscore assistance; as visual assessment has limited capabilities to discern subtle changes. Besides, Radscore assistance also resulted in a higher level of interobserver agreement. Similar findings have been reported in recent deep-learning-assisted diagnosis studies (29, 30). Our results implied that the proposed radiomics signature could be a new imaging marker providing surrogate information for PV-SMV invasion and help to overcome the limitations of subjective visual assessment. To our knowledge, this is the first study to explore providing predictions of the Radscore to assist radiologists in image interpretation of discriminating PV-SMV invasion.
Our study had several limitations. First, as it was a retrospective study, there was an unavoidable selection bias: the study only included patients who had undergone surgical treatment. Second, the study sample was relatively small. Third, we did not examine the relationships between radiological findings and histopathologic vein invasion. As we knew, histopathologic vein invasion is a significant prognostic factor; but the focus of the study is surgical PV-SMV invasion, which may contribute to elaborate preoperative planning of PD, with or without PV-SMV resection and reconstruction. Last, we excluded patients who received neoadjuvant therapy before surgery since several studies have suggested that conventional cross-sectional imaging often failed to identify the extent of the remaining viable tumor (31, 32).
In conclusion, we developed a radiomics signature that achieved comparable performance to radiologists for identifying surgical PV-SMV invasion in patients with PDAC. The radiomics signature could be a new imaging maker and demonstrated incremental value to radiologists in diagnosing surgical PV-SMV invasion.
All datasets generated for this study are included in the manuscript/Supplementary Material.
The studies involving human participants were reviewed and approved by the Ethics Review Committee of the Affiliated Wuxi No. 2 People’s Hospital of Nanjing Medical University and Wuxi No. 5 People’s Hospital. Written informed consent for participation was not required for this study in accordance with the national legislation and the institutional requirements.
FC and LZ designed the study. FC, YZ, and WX conducted the experiments. FC, LZ, YZ, and XQ analyzed the data. RZ and XG advised the study and revised the draft. FC wrote the draft. All authors contributed to the article and approved the submitted version.
This study is supported by the foundation of Wuxi Municipal Bureau on Science and Technology (CN) (N20192023), the Youth Science Project of Wuxi Health Committee (CN) (Q201727), and the Key Project of Science and Technology Development Foundation of Nanjing Medical University (CN) (2017NJMUZD116).
The authors declare that the research was conducted in the absence of any commercial or financial relationships that could be construed as a potential conflict of interest.
We would like to thank Bin Li MD and Shuanglin Zhang MD for their contribution to the patients’ data collection and imaging analysis.
The Supplementary Material for this article can be found online at: https://www.frontiersin.org/articles/10.3389/fonc.2020.523543/full#supplementary-material
PDAC, Pancreatic ductal adenocarcinoma; PV-SMV, portal vein-superior mesenteric vein; PD, pancreaticoduodenectomy; ICC, intraclass correlation coefficient; Radscore, radiomics score; AUC, area under receiver operating characteristic curve.
1. Siegel RL, Miller KD, Jemal A. Cancer statistics, 2020. CA Cancer J Clin (2020) 70(1):7–30. doi: 10.3322/caac.21590
2. Allemani C, Matsuda T, Di Carlo V, Harewood R, Matz M, Nikšić M, et al. Global surveillance of trends in cancer survival 2000–14 (CONCORD-3): analysis of individual records for 37 513 025 patients diagnosed with one of 18 cancers from 322 population-based registries in 71 countries. Lancet (2018) 391(10125):1023–75. doi: 10.1016/s0140-6736(17)33326-3
3. Shin DW, Lee JC, Kim J, Woo SM, Lee WJ, Han SS, et al. Validation of the American Joint Committee on Cancer 8th edition staging system for the pancreatic ductal adenocarcinoma. Eur J Surg Oncol (2019) 45(11):2159–65. doi: 10.1016/j.ejso.2019.06.002
4. Takahashi H, Akita H, Tomokuni A, Kobayashi S, Ohigashi H, Fijiwara Y, et al. Preoperative gemcitabine-based chemoradiation therapy for borderline resectable pancreatic cancer: impact of venous and arterial involvement status on surgical outcome and pattern of recurrence. Ann Surg (2016) 264(6):1091–7. doi: 10.1097/SLA.0000000000001547
5. Murakami Y, Uemura K, Hashimoto Y, Kondo N, Nakagawa N, Takahashi S, et al. Survival effects of adjuvant gemcitabine plus S-1 chemotherapy on pancreatic carcinoma stratified by preoperative resectability status. J Surg Oncol (2016) 113(4):405–12. doi: 10.1002/jso.24156
6. Giovinazzo F, Turri G, Katz MH, Heaton N, Ahmed I. Meta-analysis of benefits of portal-superior mesenteric vein resection in pancreatic resection for ductal adenocarcinoma. Br J Surg (2016) 103(3):179–91. doi: 10.1002/bjs.9969
7. Wang X, Demir IE, Schorn S, Jager C, Scheufele F, Friess H, et al. Venous resection during pancreatectomy for pancreatic cancer: a systematic review. Transl Gastroenterol Hepatol (2019) 4:46. doi: 10.21037/tgh.2019.06.01
8. Delpero JR, Sauvanet A. Vascular resection for pancreatic cancer: 2019 French recommendations based on a literature review from 2008 to 6-2019. Front Oncol (2020) 10:40:40. doi: 10.3389/fonc.2020.00040
9. Bockhorn M, Uzunoglu FG, Adham M, Imrie C, Milicevic M, Sandberg AA, et al. Borderline resectable pancreatic cancer: A consensus statement by the International Study Group of Pancreatic Surgery (ISGPS). Surgery (2014) 155(6):977–88. doi: 10.1016/j.surg.2014.02.001
10. Kim PT, Wei AC, Atenafu EG, Cavallucci D, Cleary SP, Moulton CA, et al. Planned versus unplanned portal vein resections during pancreaticoduodenectomy for adenocarcinoma. Br J Surg (2013) 100(10):1349–56. doi: 10.1002/bjs.9222
11. Al-Hawary MM, Francis IR, Chari ST, Fishman EK, Hough DM, Lu DS, et al. Pancreatic ductal adenocarcinoma radiology reporting template: consensus statement of the Society of Abdominal Radiology and the American Pancreatic Association. Radiology (2014) 270(1):248–60. doi: 10.1148/radiol.13131184
12. Zaky AM, Wolfgang CL, Weiss MJ, Javed AA, Fishman EK, Zaheer A. Tumor-vessel relationships in pancreatic ductal adenocarcinoma at multidetector CT: different classification systems and their influence on treatment planning. Radiographics Rev Publ Radiol Soc North America Inc (2017) 37(1):93–112. doi: 10.1148/rg.2017160054
13. Lu DS, Reber HA, Krasny RM, Kadell BM, Sayre J. Local staging of pancreatic cancer: criteria for unresectability of major vessels as revealed by pancreatic-phase, thin-section helical CT. AJR Am J Roentgenol (1997) 168(6):1439–43. doi: 10.2214/ajr.168.6.9168704
14. Loyer EM, David CL, Dubrow RA, Evans DB, Charnsangavej C. Vascular involvement in pancreatic adenocarcinoma: reassessment by thin-section CT. Abdominal Imaging (1996) 21(3):202–6. doi: 10.1007/s002619900046
15. Hough TJ, Raptopoulos V, Siewert B, Matthews JB. Teardrop superior mesenteric vein: CT sign for unresectable carcinoma of the pancreas. AJR Am J Roentgenol (1999) 173(6):1509–12. doi: 10.2214/ajr.173.6.10584793
16. Garces-Descovich A, Beker K, Jaramillo-Cardoso A, James Moser A, Mortele KJ. Applicability of current NCCN Guidelines for pancreatic adenocarcinoma resectability: analysis and pitfalls. Abdominal Radiol (2018) 43(2):314–22. doi: 10.1007/s00261-018-1459-6
17. Noda Y, Goshima S, Kawada H, Kawai N, Miyoshi T, Matsuo M, et al. Modified national comprehensive cancer network criteria for assessing resectability of pancreatic ductal adenocarcinoma. AJR Am J Roentgenol (2018) 210(6):1252–8. doi: 10.2214/AJR.17.18595
18. Hong SB, Lee SS, Kim JH, Kim HJ, Byun JH, Hong SM, et al. Pancreatic cancer CT: prediction of resectability according to NCCN criteria. Radiology (2018) 289(3):710–8. doi: 10.1148/radiol.2018180628
19. Joo I, Lee JM, Lee ES, Son J-Y, Lee DH, Ahn SJ, et al. Preoperative CT classification of the resectability of pancreatic cancer: interobserver agreement. Radiology (2019) 293(2):343–9. doi: 10.1148/radiol.2019190422
20. Lambin P, Leijenaar RTH, Deist TM, Peerlings J, de Jong EEC, van Timmeren J, et al. Radiomics: the bridge between medical imaging and personalized medicine. Nat Rev Clin Oncol (2017) 14(12):749–62. doi: 10.1038/nrclinonc.2017.141
21. van Griethuysen JJM, Fedorov A, Parmar C, Hosny A, Aucoin N, Narayan V, et al. Computational radiomics system to decode the radiographic phenotype. Cancer Res (2017) 77(21):e104–e7. doi: 10.1158/0008-5472.CAN-17-0339
22. Attiyeh MA, Chakraborty J, Doussot A, Langdon-Embry L, Mainarich S, Gönen M, et al. Survival prediction in pancreatic ductal adenocarcinoma by quantitative computed tomography image analysis. Ann Surg Oncol (2018) 25(4):1034–42. doi: 10.1245/s10434-017-6323-3
23. Sandrasegaran K, Lin Y, Asare-Sawiri M, Taiyini T, Tann M. CT texture analysis of pancreatic cancer. Eur Radiol (2019) 29(3):1067–73. doi: 10.1007/s00330-018-5662-1
24. Kim BR, Kim JH, Ahn SJ, Joo I, Choi SY, Park SJ, et al. CT prediction of resectability and prognosis in patients with pancreatic ductal adenocarcinoma after neoadjuvant treatment using image findings and texture analysis. Eur Radiol (2019) 29(1):362–72. doi: 10.1007/s00330-018-5574-0
25. De Robertis R, Beleù A, Cardobi N, Frigerio I, Ortolani S, Gobbo S, et al. Correlation of MR features and histogram-derived parameters with aggressiveness and outcomes after resection in pancreatic ductal adenocarcinoma. Abdominal Radiol (New York) (2020) 45(11):3809–18. doi: 10.1007/s00261-020-02509-3
26. Zou H, Hastie T. Regularization and variable selection via the elastic net (vol B 672005). J R Stat Soc B (2005) 67:768–:pg 301. doi: 10.1111/j.1467-9868.2005.00527.x
27. Rehders A, Stoecklein NH, Güray A, Riediger R, Alexander A, Knoefel WT. Vascular invasion in pancreatic cancer: tumor biology or tumor topography? Surgery (2012) 152(3 Suppl 1):S143–51. doi: 10.1016/j.surg.2012.05.012
28. Yamada K, Kawashima H, Ohno E, Ishikawa T, Tanaka H, Nakamura M, et al. Diagnosis of vascular invasion in pancreatic ductal adenocarcinoma using endoscopic ultrasound elastography. BMC Gastroenterol (2020) 20(1):81. doi: 10.1186/s12876-020-01228-9
29. Bien N, Rajpurkar P, Ball RL, Irvin J, Park A, Jones E, et al. Deep-learning-assisted diagnosis for knee magnetic resonance imaging: Development and retrospective validation of MRNet. PloS Med (2018) 15(11):e1002699. doi: 10.1371/journal.pmed.1002699
30. Sim Y, Chung MJ, Kotter E, Yune S, Kim M, Do S, et al. Deep convolutional neural network-based software improves radiologist detection of malignant lung nodules on chest radiographs. Radiology (2020) 294(1):199–209. doi: 10.1148/radiol.2019182465
31. Zins M, Matos C, Cassinotto C. Pancreatic adenocarcinoma staging in the era of preoperative chemotherapy and radiation therapy. Radiology (2018) 287(2):374–90. doi: 10.1148/radiol.2018171670
Keywords: pancreatic ductal adenocarcinomas, tomography, X-ray computed, radiomics, neoplasm invasion, presurgical evaluation
Citation: Chen F, Zhou Y, Qi X, Zhang R, Gao X, Xia W and Zhang L (2020) Radiomics-Assisted Presurgical Prediction for Surgical Portal Vein-Superior Mesenteric Vein Invasion in Pancreatic Ductal Adenocarcinoma. Front. Oncol. 10:523543. doi: 10.3389/fonc.2020.523543
Received: 30 December 2019; Accepted: 20 October 2020;
Published: 16 November 2020.
Edited by:
Temel Tirkes, Indiana University, United StatesReviewed by:
Xiao Chen, Affiliated Hospital of Nanjing University of Chinese Medicine, ChinaCopyright © 2020 Chen, Zhou, Qi, Zhang, Gao, Xia and Zhang. This is an open-access article distributed under the terms of the Creative Commons Attribution License (CC BY). The use, distribution or reproduction in other forums is permitted, provided the original author(s) and the copyright owner(s) are credited and that the original publication in this journal is cited, in accordance with accepted academic practice. No use, distribution or reproduction is permitted which does not comply with these terms.
*Correspondence: Lei Zhang, bGVvbjMxODNAMTYzLmNvbQ==; Wei Xia, eGlhdjE5OTBAMTYzLmNvbQ==
†These authors have contributed equally to this work and share first authorship
Disclaimer: All claims expressed in this article are solely those of the authors and do not necessarily represent those of their affiliated organizations, or those of the publisher, the editors and the reviewers. Any product that may be evaluated in this article or claim that may be made by its manufacturer is not guaranteed or endorsed by the publisher.
Research integrity at Frontiers
Learn more about the work of our research integrity team to safeguard the quality of each article we publish.