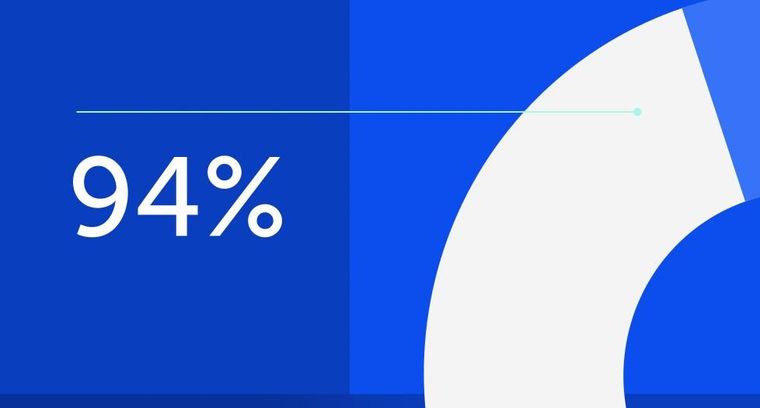
94% of researchers rate our articles as excellent or good
Learn more about the work of our research integrity team to safeguard the quality of each article we publish.
Find out more
ORIGINAL RESEARCH article
Front. Oncol., 04 August 2020
Sec. Cancer Genetics
Volume 10 - 2020 | https://doi.org/10.3389/fonc.2020.01351
Population-specific profiling of mutations in cancer genes is of critical importance for the understanding of cancer biology in general as well as the establishment of optimal diagnostics and treatment guidelines for that particular population. Although genetic analysis of tumor tissue is often used to detect mutations in cancer genes, the invasiveness and limited accessibility hinders its application in large-scale population studies. Here, we used ultra-deep massive parallel sequencing of plasma cell free DNA (cfDNA) to identify the mutation profiles of 265 Vietnamese patients with advanced non-small cell lung cancer (NSCLC). Compared to a cohort of advanced NSCLC patients characterized by sequencing of tissue samples, cfDNA genomic testing, despite lower mutation detection rates, was able to detect major mutations in tested driver genes that reflected similar mutation composition and distribution pattern, as well as major associations between mutation prevalence and clinical features. In conclusion, ultra-deep sequencing of plasma cfDNA represents an alternative approach for population-wide genetic profiling of cancer genes where recruitment of patients is limited to the accessibility of tumor tissue site.
Comprehensive profiling of actionable mutations in non-small cell lung cancer (NSCLC) is vital to precision therapy (1, 2). Therefore, mutation profiling of major driver genes such as EGFR, KRAS, BRAF, NRAS, ALK, and ROS1 has been recommended by the American Society of Clinical Oncology (ASCO) and National Comprehensive Cancer Network (NCCN) for patients with advanced NSCLC (3, 4). Currently, clinical practice guidelines on NSCLC are largely based on results from studies of Caucasian cohorts (4). However, there is a growing body of clinical evidence that NSCLC patients present heterogeneous genetic constitution across different populations, suggesting that large-scale population-specific mutation profiles are of utmost importance to the development of clinical practice guidelines for a particular population (5–7).
Although genetic testing of tumor tissue is the standard method for mutation profiling of NSCLC, the tumor biopsy is invasive and limited by accessibility. In deed, the feasibility of performing tissue biopsy is low in advanced NSCLC patients who are in metastatic stages (8). Consequently, databases built from tissue based testing may under-represent the mutation profiles of advanced NSCLC patients (9). The analysis of cell free DNA (cfDNA) in plasma, known as liquid biopsy, has recently emerged as an alternative and noninvasive approach for exploring tumor genetic landscape (10). This approach involves detecting genetic alterations in circulating tumor DNA (ctDNA), which are 160–200 bp DNA fragments released into the blood circulation by tumor cells undergoing cell death and comprise a fraction of cfDNA (10, 11). The cfDNA based approach allows sample collection for patients at all stages and high-throughput sample processing (12). It is therefore more suitable for population-scale study involving a large number of samples. Additionally, it has been reported that ultra-deep sequencing of plasma cfDNA of NSCLC patients achieved high concordance rates of driver mutation detection compared to sequencing of matched tumor tissues (13–16).
We have previously conducted a study comparing the performance of liquid biopsy and tissue biopsy using matched tissues and plasma samples obtained from the same cohort of patients (15). We observed a high concordance rate of 85% between actionable mutation profiles detected from paired plasma and tissue samples in a cohort of 40 NSCLC patients, consistent with other similar studies (15, 17, 18). However, the major limitation of such studies is that the conclusion was drawn from a relatively small sample size due to the difficulties in obtaining tissue biopsies from NSCLC patients with metastatic cancer. As such, the clinical validation of whether cfDNA sequencing could serve as a non-invasive, alternative approach to tissue biopsy in a larger cohort of patients is needed. However, it has been reported that 20–50% of patients with advanced metastatic NSCLC are not fit for tumor biopsy testing (8, 19), thus making a large-scale comparison between matched plasma and tissue samples a challenge, both logistically and ethically. In this study, we performed liquid biopsy on a large cohort of Vietnamese NSCLC patients to examine whether it can accurately represent the mutation profiles previously obtained by direct analysis of tumor derived DNA (7).
This study compared two cohorts with matched clinical features including tumor stages, treatment status, male to female ratio and age. The first cohort, hereafter called cfDNA cohort, included plasma samples collected from a total of 265 treatment-naïve patients with advanced stage NSCLC (stage III-IV) from four different hospitals in Vietnam. The second cohort, hereafter called ttDNA cohort, comprised of 285 tissue samples from Vietnamese NSCLC selected to match the tumor stages and treatment status of the cfDNA cohort. The mutation profiles of this cohort were retrieved from our previously published study (7). Descriptive statistics of these two cohorts showed that, in addition to tumor stages and treatment status, they were also comparable with respect to male-to-female ratio and median age (Table S1). Furthermore, adenocarcinoma (AC) was the dominant histological subtypes (over 90%) among patients with known histology information in both cohorts (Table S1).
Among 265 patients in the cfDNA cohort, 121 cases (45.7%) had at least one clinically relevant genetic alteration (according to ClinVar) in the six tested genes (Table S2). This detection rate was significantly lower than the detection rate of 67.4% in ttDNA cohort (Figure 1A, p < 0.00001). Likewise, the frequency of cases carrying mutations in two or more tested genes in cfDNA was significantly lower than that of ttDNA (0.4 vs. 4.2%, p < 0.05). Mutation frequencies (MF) in the tested genes were all significantly lower in cfDNA cohort compared to ttDNA cohort. There was no mutations detected in NRAS in cfDNA cohort compared to a low rate of 0.4% in ttDNA cohort.
Figure 1. Large-scale sequencing of plasma cfDNA provides mutational profiles highly correlated to those defined by sequencing tumor tissued derived DNA in Vietnamese patients with non-small cell lung cancer. (A) Correlation analysis of driver mutation frequencies between cfDNA and ttDNA testing. (B,C) Correlated distribution of EGFR (B) and KRAS (C) mutation subtypes between cfDNA and ttDNA cohorts.
There was a significant linear correlation of mutation frequency in each tested gene between the two cohorts (R2 = 0.97, 95% CI: 0.88–0.99) (Figure 1A). Among the tested genes, EGFR and KRAS were identified as the two most frequently mutated driver genes in both cohorts, with more than 50% of cases positive for mutations in either genes (Figure 1A). BRAF mutations, fusion mutations in ALK and ROS1 were detected in both cohorts at lower frequencies than EGFR and KRAS mutations (Figure 1B). These data suggested that mutation analysis using cfDNA, despite its lower detection rate, was able to revealed the relative composition of driver mutations within a cohort as comparable to ttDNA analysis.
We next focused our comparison on the mutation profiles of the two most frequently mutated genes, EGFR and KRAS, between the two cohorts. The frequencies of mutation subtypes in EGFR showed high correlation between the two cohorts (R2 = 0.96, 95% CI 0.95–0.99; Figure 1B). The majority of EGFR mutation subtypes (10/13, 76.9%) detected in ttDNA cohorts were also found in cfDNA cohort (Figure 1B). Of those, Del19 and L858R were identified as the dominant subtypes, accounting for more than 70% of all EGFR mutations in both cohorts (Figure 1B). Three rare EGFR mutation subtypes including S781I, L747S, and del20 with frequencies of 0.9, 0.9, 1.7%, respectively, in ttDNA cohort were not detected in cfDNA cohort. In contrast, certain subtypes including L858Q, R776H, A750P, and G719S were detected in cfDNA cohort but not present in ttDNA cohort (Figure 1B). For KRAS mutation subtypes, their frequencies showed lower correlation between the two cohorts than EGFR mutations (R2 = 0.75, 95% CI 0.68–0.94). Our cfDNA analysis detected 10 out of the 19 subtypes (52.3%) present in ttDNA cohort. Of those subtypes, mutations in exon 2 (G12), accounted for the dominant mutation subtypes in both cohorts (Figure 1C). Among the 9 discordant subtypes, four (Q22L, A59T, R97K, K117T) had frequencies of below 2%, while five subtypes (Q61H, K117N, K117T, G13V, and G13S) were present at greater frequencies than this threshold in ttDNA cohort. There was only 1 subtype A146T detected in cfDNA at 2.5% but not present in ttDNA cohort.
Overall, our data suggested that, despite lower detection rate, cfDNA analysis could estimate the composition and distribution patterns of major mutation subtypes compared to those previously defined by massively parallel sequencing of tumor tissues.
Previous mutation analysis of tumor tissue samples reported significant associations between certain clinical features among Vietnamese NSCLC patients and the prevalence of EGFR and KRAS mutations (7). Here, we sought to examine whether cfDNA testing is able to detect such features. We consistently found in both cohorts that KRAS mutations were more commonly detected in male patients (p < 0.0001, Table 1) while EGFR mutations was more frequently found in female (p < 0.00001, Table 1). Furthermore, the association between smoking status and mutation frequencies of KRAS and EGFR showed similar patterns between cfDNA and ttDNA cohort. Specifically, non-smokers showed significantly higher frequency of EGFR mutation (p < 0.00001, Table 1) but lower frequency of KRAS mutation (p < 0.00001, Table 1) than smokers. Additionally, it was noted that young patients (≤ 62 years) in cfDNA cohort have significant higher frequency of EGFR mutation than older patients (>62 years) (35.9 vs. 21.2%, p < 0.05) and such association was not detected in ttDNA cohort. Taken together cfDNA analysis was able to detect unique associations between mutation prevalence and clinical features.
Table 1. Association between clinical factors and mutation frequencies of NSCLC driver genes in cfDNA and ttDNA cohorts.
Since gender, age and smoking status significantly correlated with EGFR and KRAS mutation frequency in univariate analysis, we selected 252 patients with complete clinical information from the cfDNA cohort for subsequent multivariate analysis to identify significant predictors of mutation frequency. Multivariate logistic regression analysis confirmed gender (p = 0.026, odd ratio: 2.86, 95% CI: 1.09–7.51) and age (p = 0.043, odd ratio: 0.56, 95% CI: 0.31–1.01) but not smoking status (p = 0.47, odd ratio: 0.68, 95% CI: 0.25–1.80) as independent predictors of EGFR mutations, whereas smoking status was the only independent predictor of KRAS mutations (p = 0.025, odd ratio: 7.71, 95% CI: 1.28–46.1) (Table 2). In fact, we did not observed the association between smoking and EGFR mutation status when results were stratified by either gender or age, whereas smoking status was consistently associated with KRAS status regardless of gender or age (Figure S1). Taken together, our data showed that smoking status is the key predictor for KRAS mutations while gender and age together are significant predictors for EGFR mutations.
Table 2. Multivariate logistic regression analysis for EGFR and KRAS mutation status in cfDNA cohort.
Population-specific profiling of mutations in oncogenes is of critical importance for the understanding of cancer biology in general as well as the establishment of optimal diagnostics and treatment guidelines for that particular population (4). It has been reported that in patients with advanced NSCLC, particularly those with metastatic tumor, the feasibility of performing tissue biopsy is low due to its invasiveness and limited access to tissue sites (8, 20). As such, mutation profiles identified by tumor tissue genetic testing might not precisely represent the heterogeneity of the mutation landscape in a cohort of patients with advanced cancer stages (9). Recently, liquid biopsy, genetic testing of plasma cfDNA shed by tumor cells into the blood stream, has been suggested as a promising approach not only to offer noninvasive testing to advanced stage patients but also to provide more comprehensive picture of mutation profiles due to its unbiased sampling of ctDNA in the blood stream (10, 13). In this study, we evaluated the application of liquid biopsy in a large-scale profiling of population-specific mutation profile by comparing the mutation profiles of six driver genes of 265 Vietnamese patients with advance stage NSCLC defined by sequencing of cfDNA to the existing tissue based sequencing data generated from an independent cohort consisting of 285 patients with similar race, histology and treatment status (7).
We found that the overall mutation frequencies in six tested genes were significant lower in cfDNA cohort compared to cfDNA cohort. There were at least two possible explanations for the lower detection rate by cfDNA testing compared to that of tumor tissue testing. Indeed, previous cross-platform comparison between cfDNA testing and digital PCR reported a sensitivity of cfDNA sequencing of 79% for mutation detection in plasma, suggesting that mutations in cfDNA could not be detected in 20% of the cases (15). This was consistent with findings from previous studies that cfDNA based sequencing exhibited lower sensitivity in mutation detection compared to matched tissue sequencing (14, 15, 20–22). Indeed, cfDNA contains DNA from both normal and cancer cells, with the latter being found at lower abundance and in much more degraded fragments, leading to lower sensitivity in detecting tumor specific mutations (23, 24). Thus, the evidence puts more weight toward the lower detection rate of cfDNA sequencing. Additionally, the lower detection rate could be due to the fact that certain tumor clones might not shed DNA into the circulation.
Despite the lower detection rates across tested genes, the mutation profile established by cfDNA analysis showed similar composition and distribution patterns of mutation subtypes compared to the profile previously generated by tumor tissue sequencing of patients in an independent cohort. Specifically, EGFR and KRAS were consistently identified as the two most frequently mutated genes, followed by ALK, ROS1, and BRAF.
We and others have reported high concordance rates between liquid and tissue biopsy testing by comparing mutation profiles of matched plasma and paired tissue samples. However, the major limitation of such studies is that the comparison was mostly carried out in a small cohort of <50 patients due to the difficulties in obtaining sufficient number of matched plasma and tissue biopsies (15, 17, 18). Hence, the analysis was mostly limited to the most commonly mutated driver gene (EGFR or KRAS). In deed, a large number of mutation subtypes in EGFR, KRAS as well as other therapeutically targeted driver mutations such as ALK and ROS1 fusion with low frequencies might be missed and not be taken into account in matched-sample studies. As such, it is essential perform validation in a larger cohort to address whether liquid biopsy can accurately capture the complete mutation profiles of tumor tissues and provide novel insight into the heterogeneity of NSCLC tumor. In fact, we showed that both liquid biopsy and tissue biopsy analysis consistently capture multiple rare subtypes of EGFR and KRAS in addition to the dominant mutation subtypes of EGFR (del19 and L858R) and KRAS (codon 12 exon 2). Of those, mutations including T790M and ins20, known to confer resistance to currently available targeted drugs, were consistently detected in the Vietnamese cohort by both biopsy and tissue testing (25). Thus, these finding are novel and clinically significant as they further supported the potential use of liquid biopsy to identify low abundance primary resistance mutation profiles in a particular community, which is important for providing optimal treatment and diagnostic guidelines. It was noted that certain KRAS subtypes (K117N, Q61H, and G13V) displayed inconsistent results between two analysis approaches. For example, substitution mutation at codon 117 (K117N) was present in ttDNA of eight cases (11% among all KRAS subtypes) but not detected in any case of cfDNA cohort. The most likely explanation for this discrepancy is that tumor cells carrying this type of mutation tend to shed less DNA into the bloodstream, making it below the limit of detection of the current sequencing platform (26, 27). Alternatively, certain tumors were shown to not shed DNA into the circulation (26). On the other hand, there were mutation subtypes of EGFR (A750P, L858Q, R776H, and G719S) and KRAS (A146T) detected by cfDNA but not present in ttDNA cohort. It is possible that those rare subtypes present at low frequencies in cfDNA cohort might be missed by the insufficient of number of tested cases in ttDNA cohort. Alternatively, they may be derived from somatic mutations arising from the clonal hematopoiesis (28). Thus, future studies are required to examine the origin of these genomic alterations.
We also demonstrated that mutation profiling by cfDNA analysis consistently captured major associations between mutation prevalence and clinical features in Vietnamese cohorts. Thus, these findings suggest that cfDNA based sequencing was capable of identifying and charactering major mutations in driver genes of a NSLCL population. Consistent to previous studies (29, 30), in the present study we identified smoking status as being the key predictor for KRAS mutations while gender and age together are significant predictors for EGFR mutations in Vietnamese NSCLC patients.
One major limitation of this study is the lack of complete clinical information. For half of patients in ttDNA cohort, smoking status was not recorded. Additionally, half of the patients in cfDNA cohort lacked histology information. In fact, the lack of histological information of patients in the cfDNA cohort truly reflected the clinical context of our study in that tissue biopsy samples were not obtainable in a large proportion of patients with advanced metastatic cancer due to high risk of complication. Previous studies have reported a range of 20–50% of advanced NSCLC patients without tissue biopsy (8, 19). In addition, previous clinical studies consistently reported that stage IIIB or IV adenocarcinoma is the dominant histology (>80%) among Vietnamese NSCLC patients (30, 31). Thus, despite of the missing histology information, we believed that the patients in both cohorts had comparable histology features with majority had advanced adenocarcinoma, minimizing the confounding effect of histology information to our comparison. In deed, our analysis focused on patients diagnosed with advanced stages (III-IV) and naïve to treatments and may not reflect the mutation profiles of NSCLC patients who are in early cancer stages.
In conclusion, we showed that cfDNA genomic testing was able to detect major mutations in tested driver genes that reflected similar mutation composition and distribution pattern, as well as major associations between mutation prevalence and clinical features when compared to those obtained by the tumor tissue based approach. However, the lower detection rate remains a disadvantage of this method that warrants further development. Given the trade-off between sensitivity and non-invasiveness, cfDNA genomic testing is still a suitable tool for large-scale population study where recruitment of patients is not limited to the accessibility of tumor tissue site.
A total of 265 patients diagnosed with advanced NSCLC (stage IIIB and IV) from Vietnam National Cancer hospital and Ha Noi Oncology hospital, were recruited to this study. Plasma samples were collected from all patients prior to receiving any treatment (cfDNA cohort). Comprehensive patients' clinical information were summarized (Table S1). This study was approved by the Ethic Committee of University of Medicine and Pharmacy at Ho Chi Minh City, Vietnam (Ethic number: 164/HDDD). All patients whose samples were analyzed in this study have provided written consent.
The ttDNA cohort comprised of tissue samples from 285 Vietnamese NSCLC patients selected from our previous study (7). All patients in ttDNA cohort are naïve to treatment and diagnosed with advanced NSCLC at the time of hospital admission.
Ten-milliliter of peripheral blood was drawn in Streck tubes (Cell-free DNA BCT, Streck) and undergone 2 rounds of centrifugation (2,000 × g for 10 min then 16,000 × g for 10 min) to separate plasma from blood cells. The plasma fractions (4–6 mL) were then collected, aliquoted (2 mL per aliquot) and stored at −80°C until cell free DNA extraction.
Cell free DNA was extracted from an aliquot of 2 ml of plasma using the MagMAX Cell-Free DNA Isolation kit (Thermo Fisher, USA) following the manufacturer's instructions.
Cell free DNA was prepared and sequenced as previously described. Briefly, library with unique molecular identifier tagging was prepared from 2 ng of cfDNA using the Accel-NGS 2S Plus DNA library kit (Swift Biosciences, USA) following the manufacturer's instructions. Library concentrations were quantified using QuantiFluor dsDNA system (Promega, USA). Equal amounts of libraries (150 ng per sample) were pooled together and hybridized with xGen Lockdown probes for six targeted genes EGFR, KRAS, NRAS, BRAF, ALK, and ROS1 (IDT DNA, USA). For ALK, and ROS1, customized probes for intron regions were designed and mixed with probes for exon regions at equal concentration. Sequencing was run using NextSeq 500/550 High output kits v2 (150 cycles) on Illumina NextSeq 550 system (Illumina, USA) with minimum target coverage of 10,000 X. A total on-target fraction of 20–40% was detected.
Each sample was barcoded with a single 8-bp index in the P7 primer and each DNA fragment were tagged with a unique identifier consisting of a random 9-bp sequence within the P5 primer. Pair-end (PE) reads and the correspondent unique identifier sequences were generated using bcl2fastq package (Illumina). The reads were aligned to human genome (hg38) using BWA package and then grouped by the unique identifier in order to determine a consensus sequence for each fragment, eliminating sequencing and PCR errors that account for <50% of reads per fragment. The consensus reads were used for final variant calling using Mutect2 (32). A custom pipeline with call to BWA 0.7.1 (33), Picard 2.18.23 (Broad Institute, GitHub Repository), Samtools 1.9 (33) and Fulcrum genomic analysis packages (34) were built to perform the above-mentioned analysis steps. Unique molecular identifier (UMI) grouping was performed by using fgbio 0.8.0 package (Fulcrum Genomics). For detection of ALK and ROS1 rearrangement, fusion variant calling was analyzed using Factera v1.4.4 with default parameters (35).
Differences in demographic features between cfDNA and ttDNA cohort was compared by Kruskal-Wallis and Dunn's multiple comparison test for age and Fisher's exact test for other variables (Table 1). Pearson's chi-squared (χ2) test (sample size > 5) or Fisher's exact test (sample size ≤ 5) was performed on the web page “Social Science Statistics” (http://www.socscistatistics.com) to assess the association between two categorical variables (Table 1). Bonferroni correction was applied when multiple comparisons were performed. Multivariate logistic regression analysis was performed using XLSTAT tool (2020.2.3) was performed to assess the correlation of EGFR and KRAS mutation with age, gender and smoking status.
The datasets in this study have been upload to the NCBI SRA repository (https://www.ncbi.nlm.nih.gov/bioproject). The accession number is PRJNA642002.
This study was approved by the Ethic Committee of University of Medicine and Pharmacy at Ho Chi Minh City, Vietnam (Ethic number: 164/HDDD). The patients/participants provided their written informed consent to participate in this study.
V-UT, BV, A-TD, LTN, T-CN, H-AP, T-TT, and LHN performed experiments. CN, T-HN, HL, M-LN, VL, and V-QB recruited patients and performed pathological analysis. T-TN, K-HN, Y-VV, NN, H-HB, and L-SP collected and analyzed clinical data. Q-TN, T-TD, NL, and KT designed experiments and analyzed data. M-DP revised the manuscript. H-NN supervised the project, analyzed the data, and wrote the manuscript. HG designed experiments and analyzed sequencing data. All authors contributed to the article and approved the submitted version.
This research was funded by Gene Solutions (GS-005). The funder (Gene Solutions) provided support in the form of salaries for authors LT, V-UT, BV, LTN, T-CN, H-AP, T-TT, LHN, T-TN, K-HN, Y-VV, NN, M-DP, and HG but did not have any additional role in the study design, data collection and analysis, decision to publish, or preparation of the manuscript.
LT, V-UT, BV, LTN, T-CN, H-AP, T-TT, LHN, T-TN, K-HN, Y-VV, NN, M-DP, and HG were employed by Gene Solutions.
The remaining authors declare that the research was conducted in the absence of any commercial or financial relationships that could be construed as a potential conflict of interest.
The Supplementary Material for this article can be found online at: https://www.frontiersin.org/articles/10.3389/fonc.2020.01351/full#supplementary-material
1. Absenger G, Terzic J, Bezan A. ASCO update: lung cancer. Memo. (2017) 10:224–7. doi: 10.1007/s12254-017-0373-x
2. Liang H, Huang J, Wang B, Liu Z, He J, Liang W. The role of liquid biopsy in predicting post-operative recurrence of non-small cell lung cancer. J Thorac Dis. (2018) 10:S838–45. doi: 10.21037/jtd.2018.04.08
3. Kalemkerian G, Narula N, Kennedy E. Molecular testing guideline for the selection of lung cancer patients for treatment with TKI. Oncol Pract. (2018) 14:323–7. doi: 10.1200/JOP.18.00035
4. Pennell N, Arcila M, Gandara D, West H. Biomarker testing for patients with advanced non-small cell lung cancer. Am Soc Clin Oncol Educ Book. (2019) 39:531–42. doi: 10.1200/EDBK_237863
5. Bollig-Fischer A, Chen W, Gadgeel SM, Wenzlaff AS, Cote ML, Schwartz AG, et al. Racial diversity of actionable mutations in non-small cell lung cancer. J Thorac Oncol. (2015) 10:250–5. doi: 10.1097/JTO.0000000000000420
6. Lusk CM, Watza D, Dyson G, Craig D, Ratliff V, Wenzlaff AS, et al. Profiling the mutational landscape in known driver genes and novel genes in African American non-small cell lung cancer patients. Clin Cancer Res. (2019) 25:4300–8. doi: 10.1158/1078-0432.CCR-18-2439
7. Dang AH, Tran VU, Tran TT, Thi Pham HA, Le DT, Nguyen L, et al. Actionable mutation profiles of non-small cell lung cancer patients from Vietnamese population. Sci Rep. (2020) 10:2707. doi: 10.1038/s41598-020-59744-3
8. Uozu S, Imaizumi K, Yamaguchi T, Goto Y, Kawada K, Minezawa T, et al. Feasibility of tissue re-biopsy in non-small cell lung cancers resistant to previous epidermal growth factor receptor tyrosine kinase inhibitor therapies. BMC Pulm Med. (2017) 17:175. doi: 10.1186/s12890-017-0514-3
9. Strickler JH, Loree JM, Ahronian LG, Parikh AR, Niedzwiecki D, Pereira AAL, et al. Genomic landscape of cell-free DNA in patients with colorectal cancer. Cancer Discov. (2018) 8:164–73. doi: 10.1158/2159-8290.CD-17-1009
10. Crowley E, Di Nicolantonio F, Loupakis F, Bardelli A. Liquid biopsy: monitoring cancer-genetics in the blood. Nat Rev Clin Oncol. (2013) 10:472–84. doi: 10.1038/nrclinonc.2013.110
11. Maia MC, Salgia M, Pal SK. Harnessing cell-free DNA: plasma circulating tumour DNA for liquid biopsy in genitourinary cancers. Nat Rev Urol. (2020) 17:271–91. doi: 10.1038/s41585-020-0297-9
12. Rosell R, Karachaliou N. Large-scale screening for somatic mutations in lung cancer. Lancet. (2016) 387:1354–6. doi: 10.1016/S0140-6736(15)01125-3
13. Besse B, Remon J, Lacroix L, Mezquita L, Jovelet C, Howarth K, et al. Evaluation of liquid biopsies for molecular profiling in untreated patients with stage III/IV non-small cell lung cancer (NSCLC). J Clin Oncol. (2017) 35:11540. doi: 10.1200/JCO.2017.35.15_suppl.11540
14. Plagnol V, Woodhouse S, Howarth K, Lensing S, Smith M, Epstein M, et al. Analytical validation of a next generation sequencing liquid biopsy assay for high sensitivity broad molecular profiling. PLoS ONE. (2018) 13:e0193802. doi: 10.1371/journal.pone.0193802
15. Tran LS, Pham HT, Tran VU, Tran TT, Dang AH, Le DT, et al. Ultra-deep massively parallel sequencing with unique molecular identifier tagging achieves comparable performance to droplet digital PCR for detection and quantification of circulating tumor DNA from lung cancer patients. PLoS ONE. (2019) 14:e0226193. doi: 10.1371/journal.pone.0226193
16. Jiang J, Adams HP, Yao L, Yaung S, Lal P, Balasubramanyam A, et al. Concordance of genomic alterations by next-generation sequencing in tumor tissue versus cell-free DNA in stage I-IV non-small cell lung cancer. J Mol Diagn. (2020) 22:228–35. doi: 10.1016/j.jmoldx.2019.10.013
17. Schwaederlé MC, Patel SP, Husain H, Ikeda M, Lanman RB, Banks KC, et al. Utility of genomic assessment of blood-derived circulating tumor DNA (ctDNA) in patients with advanced lung adenocarcinoma. Clin Cancer Res. (2017) 23:5101–11. doi: 10.1158/1078-0432.CCR-16-2497
18. Thompson JC, Yee SS, Troxel AB, Savitch SL, Fan R, Balli D, et al. Detection of therapeutically targetable driver and resistance mutations in lung cancer patients by next-generation sequencing of cell-free circulating tumor DNA. Clin Cancer Res. (2016) 22:5772–82. doi: 10.1158/1078-0432.CCR-16-1231
19. Meric-Bernstam F, Brusco L, Shaw K, Horombe C, Kopetz S, Davies MA, et al. Feasibility of large-scale genomic testing to facilitate enrollment onto genomically matched clinical trials. J Clin Oncol. (2015) 33:2753–62. doi: 10.1200/JCO.2014.60.4165
20. Wu Z, Yang Z, Dai Y, Zhu Q, Chen LA. Update on liquid biopsy in clinical management of non-small cell lung cancer. OncoTargets Ther. (2019) 12:5097–109. doi: 10.2147/OTT.S203070
21. Couraud S, Vaca-Paniagua F, Villar S, Oliver J, Schuster T, Blanche H, et al. Noninvasive diagnosis of actionable mutations by deep sequencing of circulating free DNA in lung cancer from never-smokers: a proof-of-concept study from BioCAST/IFCT-1002. Clin Cancer Res. (2014) 20:4613–24. doi: 10.1158/1078-0432.CCR-13-3063
22. Oxnard GR, Paweletz CP, Kuang Y, Mach SL, O'Connell A, Messineo MM, et al. Noninvasive detection of response and resistance in EGFR-mutant lung cancer using quantitative next-generation genotyping of cell-free plasma DNA. Clin Cancer Res. (2014) 20:1698–705. doi: 10.1158/1078-0432.CCR-13-2482
23. Mouliere F, Chandrananda D, Piskorz AM, Moore EK, Morris J, Ahlborn LB, et al. Enhanced detection of circulating tumor DNA by fragment size analysis. Sci Transl Med. (2018) 10:aat4921. doi: 10.1126/scitranslmed.aat4921
24. Underhill HR, Kitzman JO, Hellwig S, Welker NC, Daza R, Baker DN, et al. Fragment length of circulating tumor DNA. PLoS Genet. (2016) 12:e1006162. doi: 10.1371/journal.pgen.1006162
25. Masood A, Kancha RK, Subramanian J. Epidermal growth factor receptor (EGFR) tyrosine kinase inhibitors in non-small cell lung cancer harboring uncommon EGFR mutations: Focus on afatinib. Semin Oncol. (2019) 46:271–83. doi: 10.1053/j.seminoncol.2019.08.004
26. Atkins A, Gupta P, Zhang BM, Tsai WS, Lucas J, Javey M, et al. Detection of circulating tumor DNA with a single-molecule sequencing analysis validated for targeted and immunotherapy selection. Mol Diagn Ther. (2019) 23:521–35. doi: 10.1007/s40291-019-00406-0
27. Komatsubara KM, Sacher AG. Circulating tumor DNA as a liquid biopsy: current clinical applications and future directions. Oncology. (2017) 31:618–27. Available online at: https://europepmc.org/article/med/28812303
28. Yaung SJ, Fuhlbrück F, Wu J, Casey F, Peterson M, Zou W, et al. Evaluation of clonal hematopoiesis in late stage NSCLC using a next-generation sequencing panel targeting cancer genes. J Clin Oncol. (2019) 37:9050. doi: 10.1200/JCO.2019.37.15_suppl.9050
29. Dogan S, Shen R, Ang DC, Johnson ML, D'Angelo SP, Paik PK, et al. Molecular epidemiology of EGFR and KRAS mutations in 3,026 lung adenocarcinomas. Clin Cancer Res. (2012) 18:6169–77. doi: 10.1158/1078-0432.CCR-11-3265
30. Vu HA, Xinh PT, Ha HT, Hanh NT, Bach ND, Thao DT, et al. Spectrum of EGFR gene mutations in Vietnamese patients with non-small cell lung cancer. Asia Pac J Clin Oncol. (2016) 12:86–90. doi: 10.1111/ajco.12448
31. Ha TV, Hoang MV, Vu MQ, Hoang NT, Khuong LQ, Vu AN, et al. Willingness to pay for a quality-adjusted life year among advanced non-small cell lung cancer patients in Viet Nam, 2018. Medicine. (2020) 99:e19379. doi: 10.1097/MD.0000000000019379
32. Cibulskis K, Lawrence MS, Carter SL, Sivachenko A, Jaffe D, Sougnez C, et al. Sensitive detection of somatic point mutations in impure and heterogeneous cancer samples. Nat Biotechnol. (2013) 31:213–9. doi: 10.1038/nbt.2514
33. Li H, Handsaker B, Wysoker A, Fennell T, Ruan J, Homer N, et al. The sequence alignment/map format and SAMtools. Bioinformatics. (2009) 25:2078–9. doi: 10.1093/bioinformatics/btp352
34. Burriesci MS, Lehnert EM, Pringle JR. Fulcrum: condensing redundant reads from high-throughput sequencing studies. Bioinformatics. (2012) 28:1324–7. doi: 10.1093/bioinformatics/bts123
Keywords: liquid biopsy, circulating tumor DNA, non-small cell lung cancer, actionable mutations, ultra-deep sequencing, tissue biopsy, targeted therapy
Citation: Tran LS, Nguyen Q-TT, Nguyen CV, Tran V-U, Nguyen T-HT, Le HT, Nguyen M-LT, Le VT, Pham L-S, Vo BT, Dang A-TH, Nguyen LT, Nguyen T-CV, Pham H-AT, Tran T-T, Nguyen LH, Nguyen T-TT, Nguyen K-HT, Vu Y-V, Nguyen NH, Bui V-Q, Bui H-H, Do T-TT, Lam NV, Truong Dinh K, Phan M-D, Nguyen H-N and Giang H (2020) Ultra-Deep Massive Parallel Sequencing of Plasma Cell-Free DNA Enables Large-Scale Profiling of Driver Mutations in Vietnamese Patients With Advanced Non-Small Cell Lung Cancer. Front. Oncol. 10:1351. doi: 10.3389/fonc.2020.01351
Received: 28 March 2020; Accepted: 26 June 2020;
Published: 04 August 2020.
Edited by:
Xiao Zhu, Guangdong Medical University, ChinaReviewed by:
Yiyang Wu, Vanderbilt University Medical Center, United StatesCopyright © 2020 Tran, Nguyen, Nguyen, Tran, Nguyen, Le, Nguyen, Le, Pham, Vo, Dang, Nguyen, Nguyen, Pham, Tran, Nguyen, Nguyen, Nguyen, Vu, Nguyen, Bui, Bui, Do, Lam, Truong Dinh, Phan, Nguyen and Giang. This is an open-access article distributed under the terms of the Creative Commons Attribution License (CC BY). The use, distribution or reproduction in other forums is permitted, provided the original author(s) and the copyright owner(s) are credited and that the original publication in this journal is cited, in accordance with accepted academic practice. No use, distribution or reproduction is permitted which does not comply with these terms.
*Correspondence: Le Son Tran, bGVzb24xODA4QGdtYWlsLmNvbQ==; Minh-Duy Phan, cG1kdXlAeWFob28uY29t; Hoai-Nghia Nguyen, bmhuZ2hpYTgxQGdtYWlsLmNvbQ==; Hoa Giang, Z2lhbmdob2FAZ21haWwuY29t
Disclaimer: All claims expressed in this article are solely those of the authors and do not necessarily represent those of their affiliated organizations, or those of the publisher, the editors and the reviewers. Any product that may be evaluated in this article or claim that may be made by its manufacturer is not guaranteed or endorsed by the publisher.
Research integrity at Frontiers
Learn more about the work of our research integrity team to safeguard the quality of each article we publish.