- 1Department of Neurosurgery, Peking Union Medical College Hospital, Chinese Academy of Medical Sciences and Peking Union Medical College, Beijing, China
- 2Chinese Pituitary Adenoma Cooperative Group, China Pituitary Disease Registry Center, Beijing, China
Purpose: Alternative splicing (AS) was reported to play a vital role in development and progression of glioblastoma (GBM), the most common and fatal brain tumor. Systematic analysis of survival-associated AS event profiles and prognostic prediction model based on multiple AS events in GBM was needed.
Methods: Genome-wide AS and RNA sequencing profiles were generated in 152 patients with GBM in the cancer genome atlas (TCGA). Prognosis-associated AS events were screened by integrated Cox regression analysis to construct the prognostic risk score model in the training cohort (n = 101). The AS-based signature and clinicopathologic parameters were applied to construct a prognostic nomogram for 0.5-, 1-, and 3-year OS prediction. Finally, the regulatory networks between prognostic AS events and splicing factors (SFs) were constructed.
Results: A total of 1,598 prognosis-related AS events from 1,183 source genes were determined. Eight prognostic risk score model based on integrated AS events and 7 AS types were established, respectively. Concordance index (C-index) and receiver operating characteristic (ROC) curve analysis demonstrated powerful ability in distinguishing patients' outcomes. Only Alternate Donor site (AD) and Exon Skip (ES) signature out of the eight types of AS signature were identified as independent prognostic factors for GBM, which was validated in the internal validation cohort. The nomogram with age, new event, pharmaceutical therapy, radiation therapy, AD signature and ES signature were constructed, with C-index of 0.892 (95% CI, 0.853–0.931; P = 5.13 × 10−15). Calibration plots, ROC, and decision curve analysis suggested excellent predictive performance for the nomogram in both TCGA training cohort and validation cohort. Splicing network indicated distinguished correlations between prognostic AS events and SFs in GBM patients.
Conclusions: AS-based prediction model could serve as a promising prognostic predictor and potential therapeutic target for GBM, facilitating better treatment strategies in clinical practice.
Introduction
Glioma is the most common primary tumor of the central nervous system, accounting for 40–50% of brain tumors in the United States from 2010 to 2014 (1). Glioblastoma (GBM), corresponding to WHO grade IV, is the most commonly occurring and most lethal type of glioma, generally exhibiting a 5-year overall survival (OS) rate of ~5% (1, 2). Despite remarkable advances in the development of managements for GBM, including surgery, chemotherapy, radiotherapy, targeted therapy, and immunotherapy, the optimal treatment strategy remains controversial (3). It has been reported in the literature that age, extent of resection, and various molecular alterations can serve as prognostic factors for GBM (4–6). Numerous clinical and molecular studies of GBM have been reported in recent years (4–6). However, the exact underlying mechanisms that contribute to its development, progression and recurrence have not been clearly elucidated. Hence, exploring the underlying molecular mechanisms and investigating prognostic biomarkers and predictors of therapeutic response are indispensable for the treatment of GBM patients.
Alternative splicing (AS) is a vital posttranscriptional process that modifies >95% of human genes by regulating the translation of mRNA isoforms and encoding splice variants in normal physiology (7, 8). Emerging evidence has demonstrated that aberrant AS events play a vital role in multiple cancers by promoting tumor cell proliferation, immune escape, metastasis, and drug resistance (9, 10). In addition, alterations or changes in the expression of splicing factors (SFs) could contribute to oncogenic AS events by activating oncogenes or cancer-related pathways and deactivating tumor suppressors (11). With the rapid development of large-scale genome-sequencing technologies, the roles of multiple oncogenic AS events in GBM have been investigated in recent years, including the associations between those AS events and GBM tumorigenesis, progression, recurrence and even treatment (12–14). Certain AS events and their cancer-specific mRNA isoforms can serve as promising therapeutic and prognostic biomarkers for GBM. However, systematic analysis of survival-associated AS event profiles and prognostic prediction models based on multiple AS events have not been realized in GBM before.
In this study, by performing a global expression profile assessment, we aimed to identify survival-related AS signatures that could serve as molecular biomarkers for subgroup classification, risk stratification, prognostication, and therapeutic targets in GBM. Moreover, we successfully established a novel, promising prognostic nomogram for GBM based on multiple AS signatures and clinicopathological factors, and we demonstrated its powerful predictive ability. Finally, the regulatory networks between prognostic AS events and SFs were constructed to investigate the underlying regulatory mechanisms.
Materials and Methods
Data Retrieval and Processing
The level 3 RNA sequencing data and clinical information of 156 GBM patients were downloaded from The Cancer Genome Atlas (TCGA, https://portal.gdc.cancer.gov/) database. Patients without prognostic information were excluded. AS events in GBM and their percent-splice-in (PSI) values were obtained from the TCGA SpliceSeq data portal (https://bioinformatics.mdanderson.org/TCGASpliceSeq/). PSI values are expressed on a scale from 0 to 1 and are commonly used to quantify AS events, providing an overview of the AS junction and the proportion of included exons from clinical samples (15). Seven types of AS events, namely, alternate acceptor site (AA), alternate donor site (AD), alternate promoter (AP), alternate terminator (AT), exon skip (ES), mutually exclusive exons (ME), and retained intron (RI) events, were quantified by PSI value (Figure 1A). Ultimately, a total of 152 GBM patients with TCGA SpliceSeq data, RNA sequencing data and clinical data were included our study. Four patients were excluded due to lack of prognostic information. Because the data were obtained from TCGA, our study did not require approval by an ethics committee.
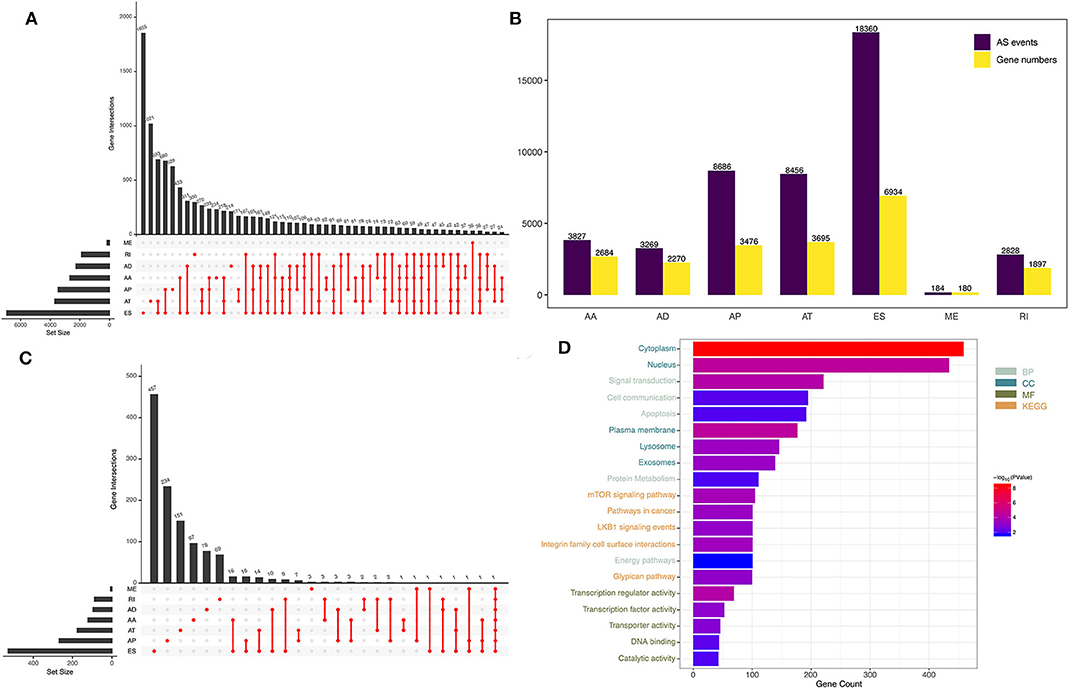
Figure 1. Overview of AS events in GBM patients. (A) UpSet plot of the seven types of AS events. (B) The source genes of the seven types of AS events. (C) The seven types of AS events and their association with survival, shown as an UpSet plot. (D) Functional enrichment analysis of the source genes of prognostic AS events.
Construction of the Prognostic Risk Score Model Based on AS Events
First, all the 152 GBM patients were randomly divided into two groups on a ratio of 2:1, including training cohort (n = 101) and internal validation cohort (n = 51). To identify survival-associated AS events, we performed univariate Cox regression analysis to identify the associations between the PSI values of various AS events and patients' OS using the “survival” package (http://bioconductor.org/packages/survival/) in R 3.5.1 (16). The intersections between AS events as well as the quantitative analysis of these interactive sets were visualized as UpSet plots using the “UpSetR” package (17). Then, the Database for Annotation, Visualization and Integrated Discovery (DAVID, http://david.ncifcrf.gov/) was used to perform functional annotation and pathway enrichment analyses, including Gene Ontology (GO) and Kyoto Encyclopedia of Genes and Genomes (KEGG) pathway analysis, for the source genes of prognostic AS events (18–20). A P-value < 0.05 was considered statistically significant.
Then, prognostic AS events with a P-value < 0.05 in univariate Cox regression were further screened by least absolute shrinkage and selection operator (LASSO) regression. We adopted the optimal value of λ according to 10-fold cross-validation and the Akaike information criterion (AIC) and Bayesian information criterion (BIC) (21, 22). Finally, the optimal prognosis-related AS events were identified by multivariate Cox regression analysis to construct a prognostic risk score model for predicting OS. The prognostic risk score model was established with the following formula: risk score = PSI value of AS event1 × β1 + PSI value of AS event2 × β2 +…+ PSI value of AS eventn × βn, where β represents the regression coefficient calculated by the multivariate Cox regression model (23). Then, the prognostic risk score was generated for each patient. All GBM patients in the training set were divided into high-risk (high risk score) and low-risk (low risk score) groups using the median risk score as the cutoff. Kaplan-Meier (K-M) survival curve analysis using the “survival” package was performed to estimate the prognoses of patients with high and low risk scores, and the survival difference between the high-risk and low-risk groups was assessed by a two-sided log-rank test. The prognostic performance of the AS signature was evaluated by Harrell's concordance index (C-index) and time-dependent receiver operating characteristic (ROC) curve analysis of 0.5-, 1-, 2-, 3-, and 5-year survival with the “survcomp” (http://www.bioconductor.org/packages/survcomp/) and “survivalROC” (https://cran.r-project.org/web/packages/survivalROC/) R packages (24, 25). Both the C-index and the area under the curve (AUC) range from 0.5 to 1, with 1 indicating perfect discrimination and 0.5 indicating no discrimination. Finally, the prognostic model constructed by the TCGA training cohort were further validated by the internal validation cohort in a similar way. In addition, to determine whether the predictive power of the AS signature could be independent of other clinicopathological parameters, we performed univariate and multivariate Cox proportional hazards regression analyses in the training set and validation set.
Construction and Validation of the Nomogram With AS Signatures
Demographics and clinical characteristics of the TCGA GBM patients in the training cohort and validation cohort were shown in Table 1. Clinicopathological parameters [including age, sex, new events, Karnofsky Performance Status (KPS) score, pharmaceutical therapy, radiation therapy, surgery, and IDH mutation status] and AS signatures (including the integrated AS signature as well as the AA, AD, AP, AT, ES, ME, and RI signatures), were entered into the univariate and multivariate Cox regression analysis. All the independent prognostic factors were determined to construct a prognostic nomogram to assess the probability of 0.5-, 1-, and 3-year OS for TCGA GBM patients using the “rms” R package (https://cran.r-project.org/web/packages/rms/) (26). The discrimination performance of the nomogram was quantitatively assessed by the C-index and the area under the ROC curve (24). Calibration plots were also used to graphically evaluate the discriminative ability of the nomogram (25). Additionally, decision curve analysis (DCA) was performed to determine the clinical usefulness of the prognostic nomogram by quantifying the net benefits at different threshold probabilities in GBM patients (27). The best prediction model is typically one that has a high net benefit within a suitable range of threshold probabilities. Finally, the prognostic nomogram was further validated by the internal validation cohort. All analyses were conducted using R version 3.5.1, and a P-value < 0.05 was considered statistically significant. Hazard ratios (HRs) and 95% confidence intervals (CIs) are reported if necessary.
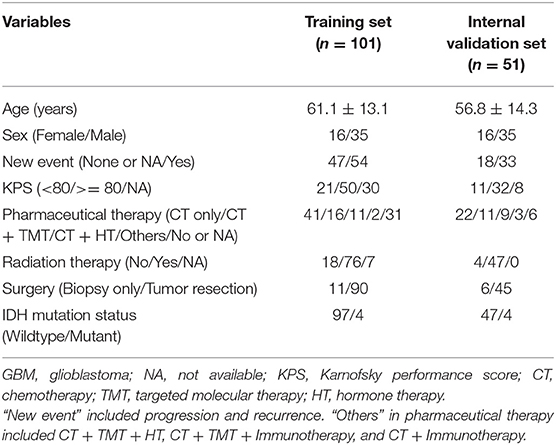
Table 1. Demographics and clinical characteristics of the TCGA GBM patients in the training cohort (n = 101) and internal validation cohort (n = 51).
Correlation Analysis and Regulatory Networks Between Prognostic AS Events and SFs
It has been reported that AS events in the tumor microenvironment can be modified or regulated by SFs (28). Hence, it is vital to explore the correlations between prognostic AS events and SFs in GBM. Correlation analyses between the PSI values of prognostic AS events and the expression levels of the corresponding SF genes were performed using Pearson's correlation test. A P-value < 0.001 combined with a correlation coefficient > 0.6 or < −0.6 was considered to indicate a significant correlation. Then, the regulatory networks between prognostic AS events and SFs were visualized using Cytoscape.
Results
AS Profiles in TCGA GBM Patients
By analyzing SpliceSeq data from 152 GBM patients, we obtained 45,610 mRNA splicing events in 21,136 source genes, including 3,827 AAs in 2,684 genes, 3,269 ADs in 2,270 genes, 8,686 APs in 3,476 genes, 8,456 ATs in 3,695 genes, 18,360 ESs in 6,934 genes, 184 MEs in 180 genes, and 2,828 RIs in 1,897 genes (Figure 1B). ES was the most common type of AS, accounting for 40% of all events, whereas ME was the least common.
Prognostic AS Events and Functional Enrichment Analysis
Through univariate Cox regression analysis, we identified a total of 1,598 prognosis-related AS events in 1,183 source genes, as depicted in Figure 1C. GO analyses, including the biological process (BP), cellular component (CC) and molecular function (MF) categories, were performed on the source genes of prognostic AS events. In the BP category, there was significant gene enrichment in signal transduction, cell communication and apoptosis. In the CC category, genes were significantly enriched in the cytoplasm, nucleus and plasma membrane. In the MF category, genes were significantly enriched in transcription regulator activity, transcription factor activity and transporter activity. In addition, KEGG pathway analysis revealed significant gene enrichment in the mTOR signaling pathway, integrin family cell surface interactions and pathways involved in cancer (Figure 1D).
Using the HR or the z-score, each prognostic AS event was classified as a favorable (HR <1 or z-score <0) or unfavorable (HR > 1 or z-score > 0) prognostic factor. The top 20 most significant AS events of each of the seven types are presented graphically in Figure 2. Interestingly, most of the prognostic AS events were favorable prognostic factors (850 vs. 747).
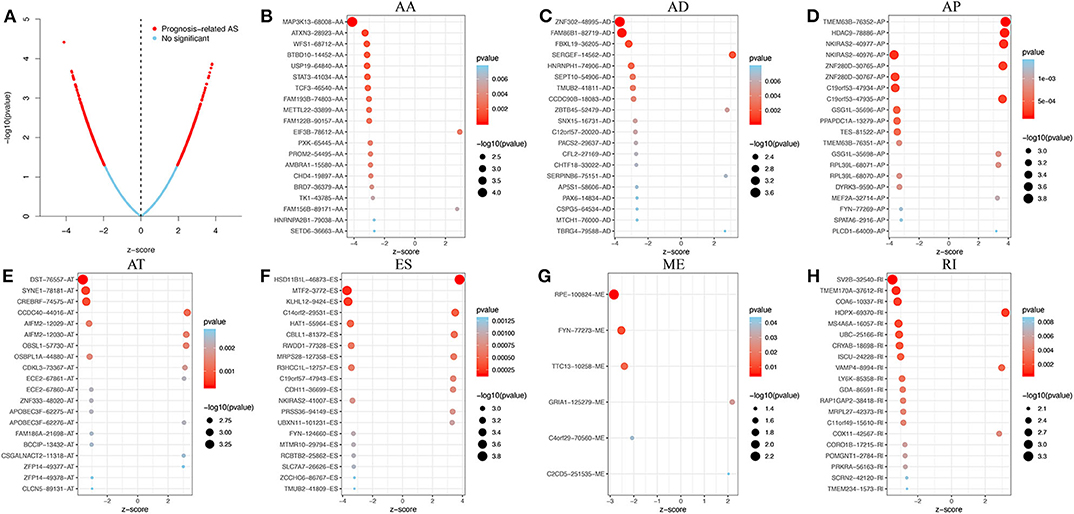
Figure 2. Prognosis-related AS events in GBM. (A) Volcano plot showing an overview of the prognosis-related AS events. (B–H) The top 20 prognosis-related AS events, illustrated by bubble plots, for AA, AD, AP, AT, ES, ME, and RI (z-score > 0, HR > 1; z-score <0, HR <1).
Construction of the AS-Based Prognostic Risk Score Models
Following the univariate Cox regression analysis, LASSO and multivariate Cox regression analysis were applied to all AS types combined and to each of the 7 AS types separately to screen the prognosis-associated AS events (Figure 3, Supplementary Table 1). ROC curves indicated excellent discriminative performances of LASSO analysis (Supplementary Figure 1). Eight prognostic risk score models based on AS events were established by the formulas shown in Table 2. Then, we calculated the prognostic risk score for each patient in the TCGA training cohort. The patients were divided into a high-risk group (high risk score) and a low-risk group (low risk score) using the median risk score as the cutoff (Figure 4). K-M survival curve analysis demonstrated that patients in high-risk groups had significantly poorer OS than patients in low-risk groups as defined by all eight types of AS signatures (log rank P <0.05; Figure 5). The C-indexes of the ES signature and the integrated AS signature for OS prediction were 0.875 (95% CI, 0.836–0.914; P = 6.05 × 10−32) and 0.852 (95% CI, 0.813–0.891; P = 5.99 × 10−26), respectively, demonstrating that these two models had the most favorable predictive value among the eight AS-based risk score models (Table 2). Furthermore, in time-dependent ROC analyses, all eight types of AS signatures showed favorable predictive ability for 0.5-, 1-, 2-, 3-, and 5-year OS, with each signature having an AUC of ~0.9 (Figure 5). Finally, to evaluate that the AS-based prognostic model had similar predictive performances in different populations, we applied it to predict OS in an independent internal validation cohort in a similar way. According to the risk score model, the 51 GBM patients in the validation cohort were divided into high-risk and low-risk groups (Supplementary Figure 2), and the OS of patients with high risk scores was significantly poorer than those with low risk scores in all eight types of AS signatures (logrank P < 0.05; Supplementary Figure 3). All eight types of AS signatures also showed favorable predictive abilities of the 0.5-, 1-, 2, 3-, and 5-year OS rates, with AUC of approximately 0.9, in the validation set (Supplementary Figure 3). These results indicate that all eight types of AS signatures may be robust and reliable prognostic predictors for GBM patients.
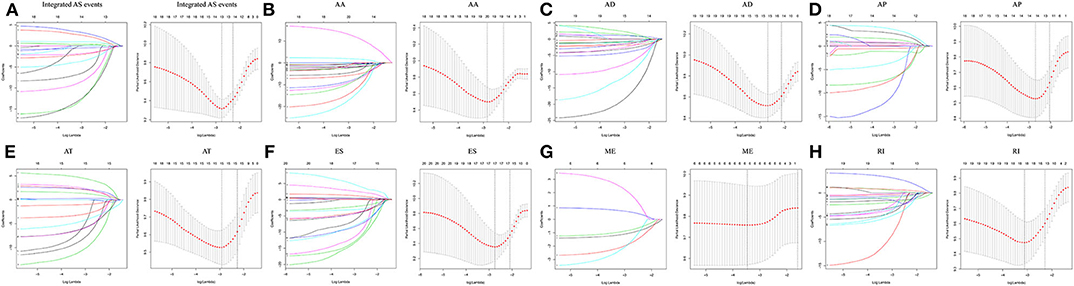
Figure 3. The prognosis-associated AS events were screened by LASSO regression analysis for all AS types combined and for each of the 7 AS types separately. Left panel (A–H) The coefficients are plotted against log(λ). A vertical line is shown at the value selected using 10-fold cross-validation, where the optimal λ results in ten features with nonzero coefficients. Right panel (A–H): Optimal parameter (λ) selection in the LASSO model used 10-fold cross-validation via minimum criteria. The partial likelihood deviance (binomial deviance) curve is plotted vs. log(λ). Dotted vertical lines are shown at the optimal values selected using two different criteria: the minimum and 1 standard error of the minimum.
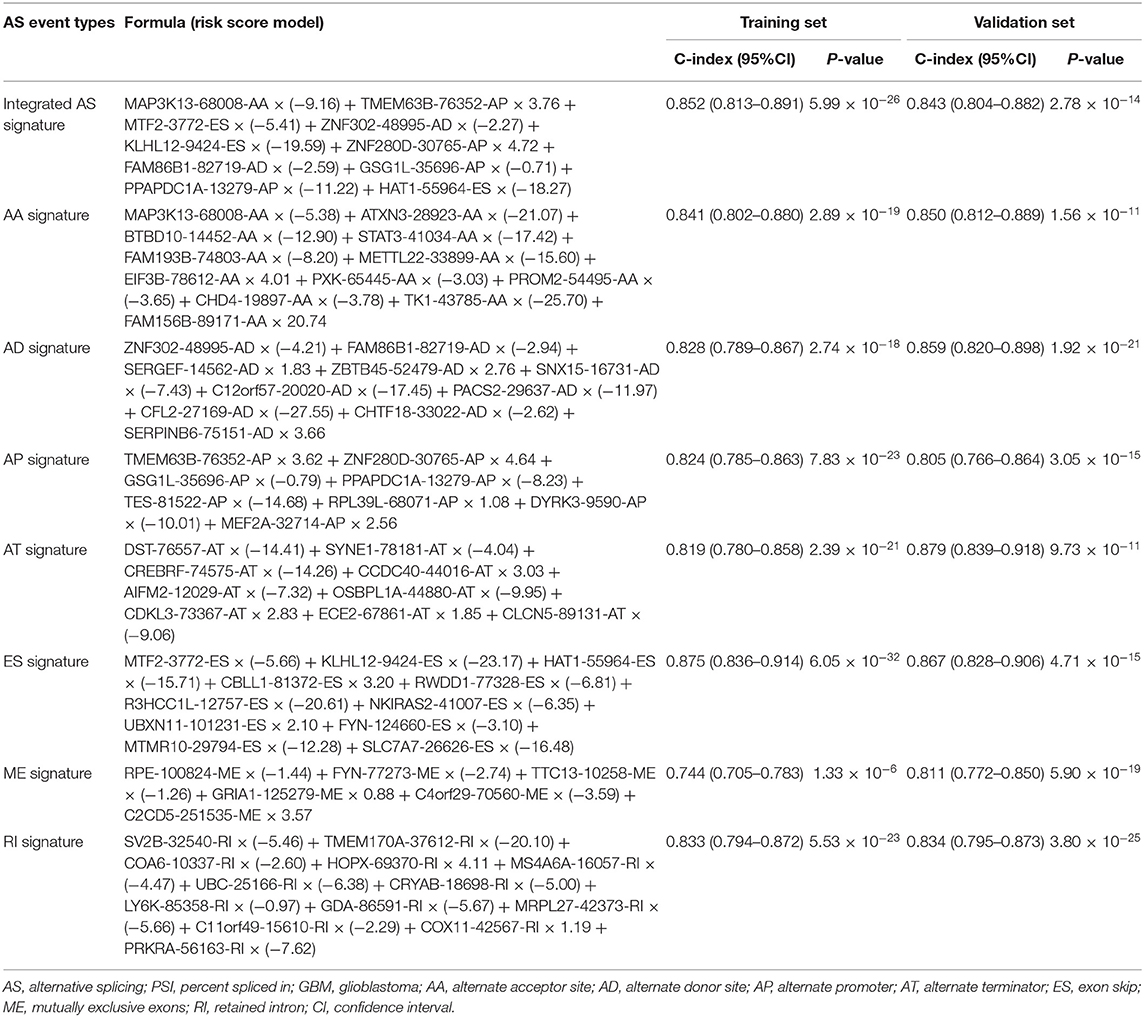
Table 2. The prognostic risk score models based on the PSI values of AS event types of GBM training cohort and C-index of the training and validation cohort.
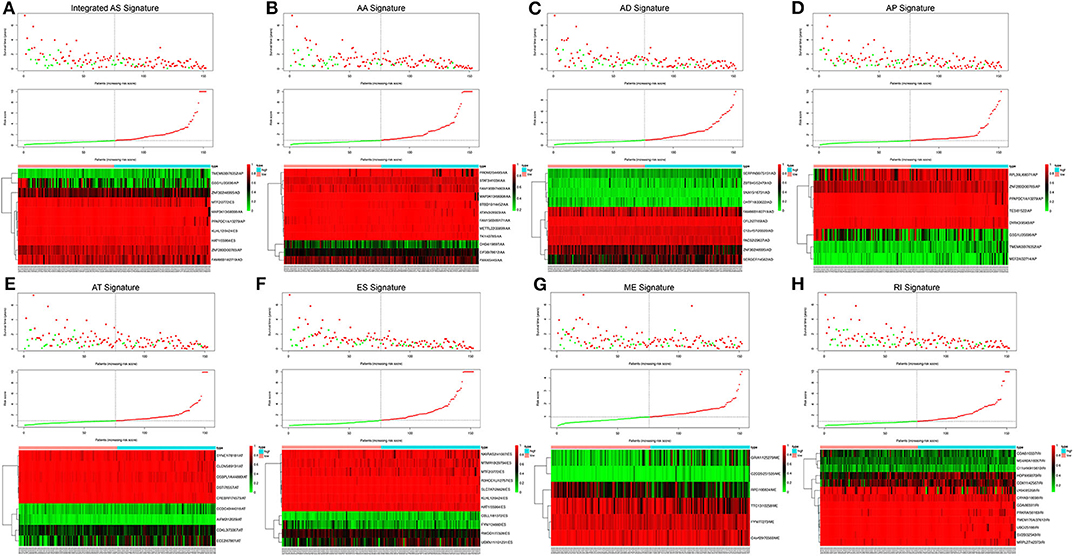
Figure 4. Risk score analysis of the integrated AS signature and the 7 type-specific AS signatures in GBM patients. Upper panel (A–H) Patient survival status and time distributed by risk score. Middle panel (A–H) Risk score curve of the AS signature. Bottom panel (A–H) Heatmaps of prognosis-related AS events. Colors ranging from green to red indicate expression levels ranging from low to high. The dotted line represents the individual inflection point of the risk score curve, by which the patients were categorized into low-risk and high-risk groups.
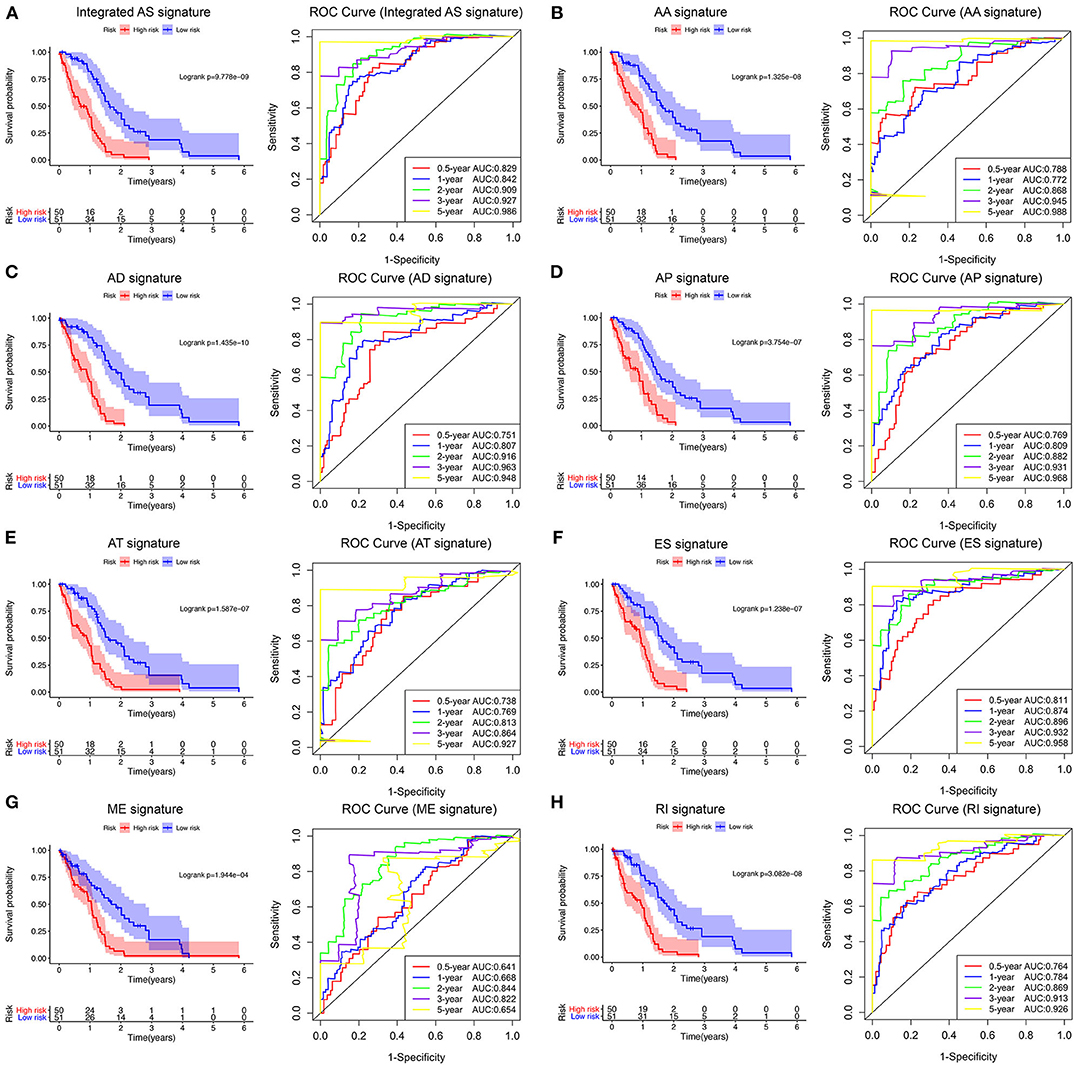
Figure 5. Survival analysis and prognostic performance of the integrated AS signature and the 7 type-specific AS signatures of GBM patients in the TCGA training set. Left panel (A–H) K-M survival curve of the risk score for the OS of GBM patients. The high-risk group had significantly poorer OS rates than the low-risk group. Right panel (A–H) The prognostic performance of the AS-based signature, demonstrated by the time-dependent ROC curves for predicting 0.5-, 1-, 2-, 3-, and 5-year OS in GBM patients.
Correlation analysis of the eight AS signatures indicated that integrated AS, AA, and RI signature was significantly positively correlated with each other in both training (Supplementary Figure 4A) and validation cohort (Supplementary Figure 4B). In addition, the genes within the same signature did not show significant correlations (all correlation coefficient < |0.6|) in both training and validation set, which excluded colinearity among these genes (Supplementary Figures 4C–J).
Evaluation of the Eight Types of AS Signatures as Independent Prognostic Factors
As shown in Table 3, univariate and multivariate Cox regression analyses were performed to evaluate the prognostic significance of the eight types of AS signatures together with various clinicopathological parameters. First, univariate analysis indicated that age (P < 0.001), new events (P = 0.003), pharmaceutical therapy (P < 0.001), radiation therapy (P = 0.001) and IDH mutation status (P = 0.009), integrated AS signature (P < 0.001), AA signature (P < 0.001), AD signature (P < 0.001), AP signature (P < 0.001), AT signature (P < 0.001), ES signature (P < 0.001), ME signature (P < 0.001), and RI signature (P < 0.001) were significantly associated with OS. Then, the multivariate analyses demonstrated that age (P = 0.010), new events (P < 0.001), pharmaceutical therapy (P = 0.011), radiation therapy (P = 0.018), AD signature (P < 0.001), and ES signature (P < 0.001) were significantly correlated with OS. Additionally, following the univariate and multivariate Cox regression analyses in the validation set, AD and ES signature were also proven to be independent prognostic predictors for GBM (Table 2). Interestingly, among the eight types of AS signatures, only AD and ES signatures were identified as independent prognostic factors for GBM.
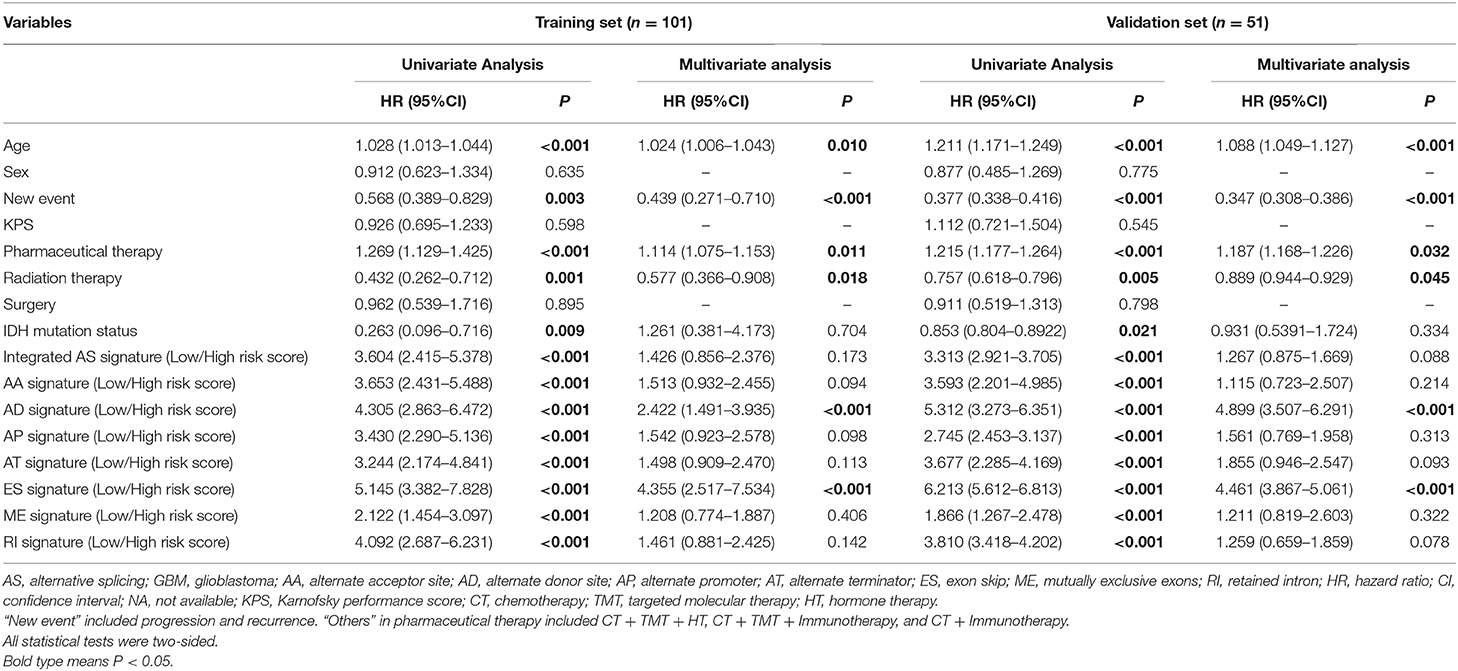
Table 3. Univariate and multivariate cox proportional hazards analysis of clinical parameters and AS event-based risk score model of the TCGA GBM patients in the training cohort (n = 101) and internal validation cohort (n = 55).
Construction and Validation of a Nomogram With AS Signatures
To develop a clinically applicable model for predicting the prognosis of GBM, we constructed a nomogram to predict the probability of 0.5-, 1-, and 3-year survival of GBM patients. Six independent prognostic factors, including age, new events, pharmaceutical therapy, radiation therapy, AD signature and ES signature, were included in the prediction model (Figure 6A). The C-index of the nomogram was 0.892 (95% CI, 0.853–0.931; P = 5.13 ×10−15). The calibration plots (Figures 6B–D) achieved excellent agreement between the predicted and observed probabilities of 0.5-, 1-, and 3-year survival in GBM patients. The nomogram also showed powerful predictive ability for 0.5-, 1-, and 3-year OS, with AUC values of 0.927, 0.928, and 0.912, respectively (Figures 6H–J). As shown in Figures 6H–J, the discrimination performance of the nomogram was significantly higher than that of a prognostic model based on any of the six factors alone (age, new events, pharmaceutical therapy, radiation therapy, AD signature and ES signature). Additionally, DCA curves were applied to determine the clinical usefulness of the prognostic nomogram at 0.5, 1, and 3 years in GBM patients. As shown in Figures 6K–M, the nomogram demonstrated a greater net benefit than any of the single-factor prognostic models. In addition, in the TCGA internal validation cohort, the C-index of the nomogram for predicting survival of 51 GBM patients was 0.795 (95% CI, 0.756–0.834; P = 2.57 ×10−10). The calibration plots also indicated excellent agreements between survival prediction and actual observation in the probabilities of 0.5-, 1-, and 3-year OS in the validation cohort (Figures 3E–G). The nomogram achieved an AUC of 0.835, 0.738, and 0.776 for 0.5-, 1-, and 3-year OS, respectively, in the validation cohort (Supplementary Figure 5A). DCA curves also demonstrated a greater net benefit of the nomogram than other factors (Supplementary Figure 5B). Additionally, as shown in Supplementary Figure 6, the nomogram also achieved excellent predictive performances in both primary and recurrent GBM in the training cohort and validation cohort. These findings suggest that the nomogram is highly reliable in predicting the prognosis of GBM, meaning that it could assist both physicians and patients in performing individualized survival predictions and facilitate better treatment decision making and follow-up scheduling.
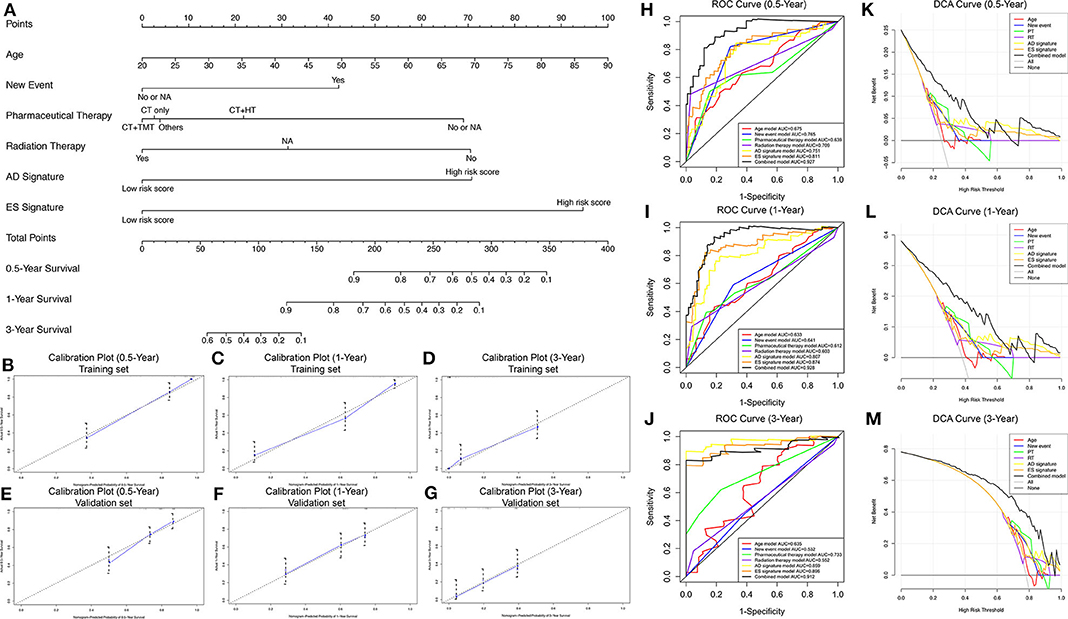
Figure 6. Nomogram to predict the probability of 0.5-, 1-, and 3-year survival in GBM. (A) Prognostic nomogram to predict the survival of GBM patients. (B–D) Calibration plots for the nomogram to predict survival at 0.5, 1, and 3 years in the training set. (E–G) Calibration plots for the nomogram to predict survival at 0.5, 1, and 3 years in the validation set. Actual survival is plotted on the y-axis, and the nomogram-predicted probability is plotted on the x-axis. (H–J) The prognostic performance of the nomogram, demonstrated by the ROC curves for predicting 0.5-, 1-, and 3-year OS, compared with other single factor prognostic models. (K–M) The clinical benefit and the scope of applications of the nomogram, evaluated by the DCA curves at 0.5, 1, and 3 years. The net benefit is plotted on the y-axis, and the threshold probabilities of patients for 1-, 3-, and 5-year survival are plotted on the x-axis.
Regulatory Networks Between Prognostic AS Events and SFs
By performing survival analyses and correlation analyses of the RNA sequencing expression data combined with the AS sequencing data, we identified 47 survival-associated SFs and 52 survival-associated AS events that had significant correlations (Pearson correlation coefficient > 0.6 or < −0.6, P < 0.001; Supplementary Table 2). A total of 151 pairs of SFs-AS events, including 65 with positive correlations and 86 with negative correlations, were included in the regulatory network (Figure 7A). Interestingly, we found two subnetworks with different SF-AS correlations. In the subnetwork centered on HEXA-31540-AT, the majority of unfavorable prognostic AS events were negatively correlated with the expression of SFs, whereas the favorable prognostic AS events were positively correlated with the expression of SFs. In another subnetwork, centered on CELF4, the majority of unfavorable prognostic AS events were positively correlated with the expression of SFs, whereas the favorable prognostic AS events were negatively correlated with the expression of SFs.
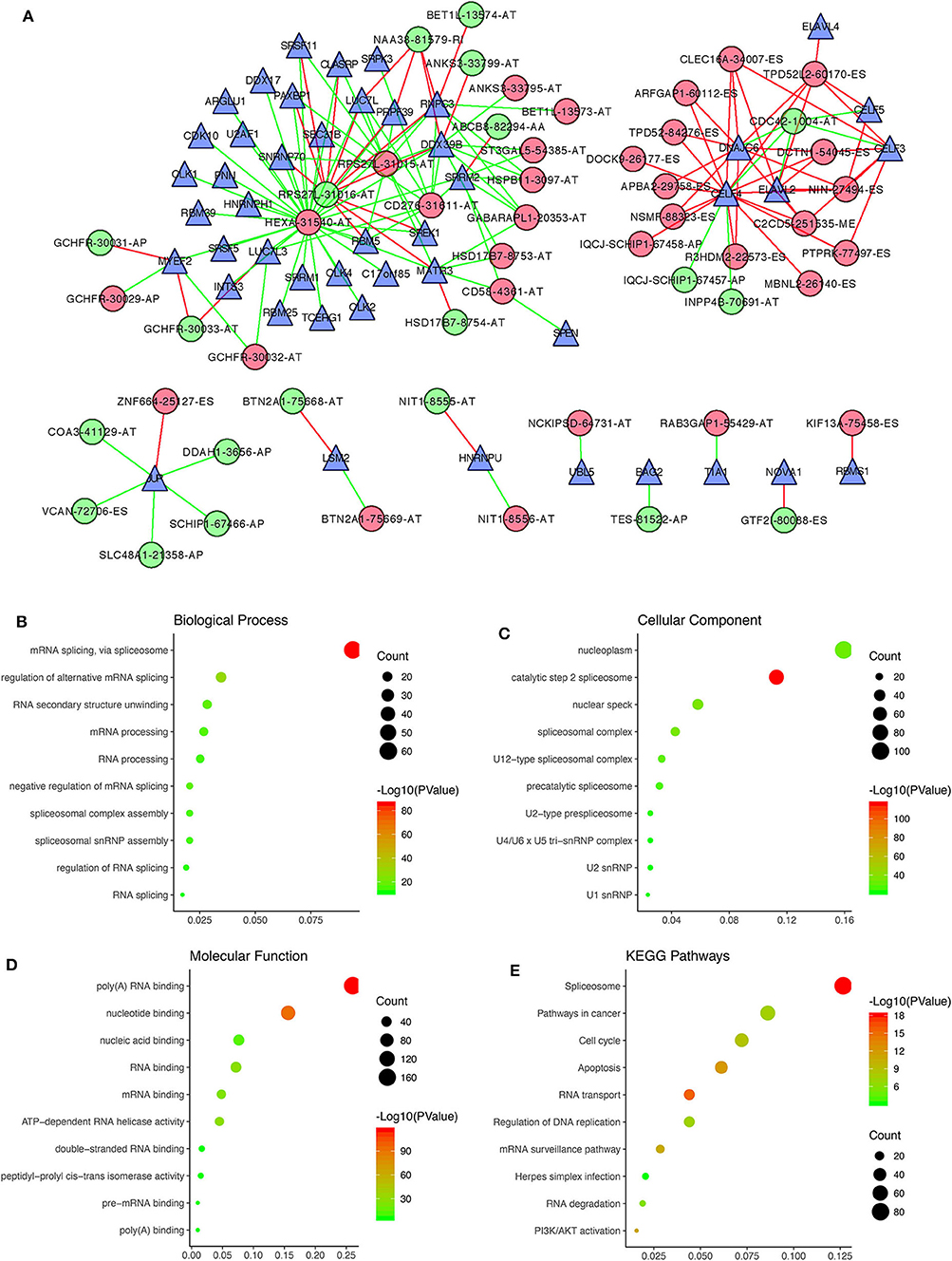
Figure 7. Correlation analysis between prognostic AS events and SFs. (A) The regulatory network between 47 survival-associated SFs and 52 survival-associated AS events, with |Pearson correlation coefficient| > 0.6 and P < 0.001. Green and red dots, respectively, represent favorable and unfavorable prognostic AS events. Green and red lines, respectively, represent negative and positive correlations between AS events and SFs. Blue triangles represent SFs. (B–E) Functional enrichment analysis of the 392 SF genes having |Pearson correlation coefficient| > 0.4 with prognostic AS events.
Furthermore, functional enrichment analyses were performed for the 392 SF genes with |Pearson correlation coefficient| > 0.4. GO analysis, revealing that they were mainly enriched in mRNA splicing and regulation of alternative mRNA splicing within the BP category (Figure 7B), the nucleoplasm and the catalytic step 2 spliceosome within the CC category (Figure 7C), and poly(A) RNA binding and nucleotide binding within the MF category (Figure 7D). As for the KEGG pathways, the 392 SF genes were mainly enriched in spliceosomes, pathways involved in cancer, the cell cycle and apoptosis (Figure 7E).
Discussion
AS is reported to be an important process modifying gene isoforms, which cause cells to produce different mRNA and protein isoforms with various functional properties in normal physiological processes (7, 8). Emerging evidence has demonstrated that dysregulated AS events play a vital role in the origin and progression of multiple cancers, especially GBM (9, 10). Aldave et al. (13) reported that the aberrant splicing regulation of BAF45d contributed to the malignant phenotype of GBM. Ferrarese et al. (29) demonstrated that lineage-specific splicing of a brain-enriched alternative exon promotes GBM progression. In addition, AS can serve as a therapeutic target for GBM. For instance, manipulating AS of the mRNA for the kinase Mnk2 (MKNK2) with splice-switching oligonucleotides (SSOs) was reported as a novel approach to inhibit glioblastoma tumorigenesis (12). In summary, numerous GBM-specific AS events and their mRNA isoforms have been identified, but there is still a lack of systematic analysis of survival-associated AS event profiles and prognostic prediction models based on multiple AS events for GBM.
In this study, we identified prognosis-related AS events and their source genes for the first time by performing univariate Cox regression analysis. A total of 1,598 (3.5%) AS events were associated with the survival of the TCGA GBM patients. Interestingly, more than half of the prognostic AS events were favorable prognostic factors. GO analysis and KEGG pathway enrichment analysis revealed that the prognostic source genes of the above AS events were mainly enriched in the pathways related to cancer and mRNA splicing. Then, following LASSO and multivariate Cox regression analysis, we constructed eight prognostic risk score models based on all AS types combined and each of the 7 AS types separately. All eight AS-based signatures showed excellent performance in distinguishing the survival of GBM patients. However, only two of them, the AD and ES signatures, were ultimately identified as independent prognostic factors for GBM compared with other clinicopathological parameters. Previous studies have investigated the novel prognostic value of various AS events and constructed the corresponding prognostic prediction models based on these AS events in multiple cancers, such as bladder urothelial carcinoma, renal clear cell carcinoma, and lung cancer (30–32). However, prognostic prediction models based on multiple AS events for GBM have not been reported before in the literature. Hence, the novel prognostic signature based on the AS events in our study, mainly AD and ES signatures, can be used for individualized survival predictions for GBM patients.
Nomogram models have been widely used in clinical practice due to its intuitive visual presentation (24). To the best of our knowledge, this is the first prognostic nomogram that incorporates AS-based signatures to predict the survival of GBM patients and was constructed from a large-scale database with long-term follow-up. In this study, we established a nomogram with age, new events, pharmaceutical therapy, radiation therapy, AD signature and ES signature. The calibration plots based on the TCGA GBM cohort demonstrated that the actual survival rates closely corresponded to the predictions, suggesting that the predictive performance of the nomogram was excellent. Following an evaluation of clinical usefulness by DCA, we concluded that our visualized scoring system is a reliable tool to aid physicians in making individualized treatment strategies and survival predictions, which could facilitate better treatment decision-making and follow-up scheduling.
Previous studies have demonstrated that SFs regulate oncogenic AS events by binding to splice-regulatory sequence elements of specific genes (28). In this study, we performed correlation analyses and constructed the regulatory networks between prognostic AS events and SFs to investigate the underlying regulatory mechanisms in GBM. Interestingly, we found two subnetworks with different SF-AS correlations. In the subnetwork centered on HEXA-31540-AT, the majority of unfavorable prognostic AS events were negatively correlated with the expression of SFs, whereas the favorable prognostic AS events were positively correlated with SFs. In another subnetwork, centered on CELF4, the majority of unfavorable prognostic AS events were positively correlated with SFs, whereas the favorable prognostic AS events were negatively correlated with SFs. Our study provides a novel understanding of AS patterns and their correlations with SFs in GBM, which may eventually help to elucidate the underlying roles of oncogenic AS events in the development of GBM.
This study has some limitations. First, the clinicopathological information downloaded from the TCGA GBM database was limited and incomplete. Detailed information on neuroimaging, the extent of resection, radiation therapy and chemotherapy was not included in the Cox regression model. Second, the prediction model was not further validated in the external GBM database containing AS sequencing data. Additional large-scale, multicenter prospective clinical trials are needed in the future.
In conclusion, by performing a global expression profile assessment, we developed a reliable AS-based risk score model for subgroup classification, risk stratification, prognosis prediction, and identification of potential therapeutic targets for GBM. Then, we successfully established a novel, promising prognostic nomogram that uses AS signatures and clinicopathological factors for individualized survival prediction to facilitate better treatment strategy and decision-making. Finally, the regulatory networks between prognostic AS events and SFs were constructed, which may eventually help to elucidate the underlying mechanisms of oncogenic AS events in the development and progression of GBM.
Data Availability Statement
Publicly available datasets were analyzed in this study. This data can be found here: The level 3 RNA sequencing data and clinical information of 156 GBM patients were downloaded from The Cancer Genome Atlas (TCGA, https://portal.gdc.cancer.gov/) database. AS events in GBM and their percent-splice-in (PSI) values were obtained from the TCGA SpliceSeq data portal (https://bioinformatics.mdanderson.org/TCGASpliceSeq/).
Author Contributions
LG, XG, and CF performed the data curation and analysis. KD and WL analyzed and interpreted the results. ZW and BX drafted and reviewed the manuscript. All authors contributed to the article and approved the submitted version.
Funding
This study was supported by the Graduate Innovation Fund of Chinese Academy of Medical Sciences and Peking Union Medical College (2019-1002-73) and the China Postdoctoral Science Foundation (2019M650567).
Conflict of Interest
The authors declare that the research was conducted in the absence of any commercial or financial relationships that could be construed as a potential conflict of interest.
Acknowledgments
ZW is grateful for the invaluable support received from his parents and from BX over the years.
Supplementary Material
The Supplementary Material for this article can be found online at: https://www.frontiersin.org/articles/10.3389/fonc.2020.01257/full#supplementary-material
Abbreviations
Abbreviations: AS, alternative splicing; GBM, glioblastoma; TCGA, the cancer genome atlas; SF, splicing factor; C-index, Harrell's concordance index; ROC, receiver operating characteristic; AD, Alternate Donor site; ES, Exon Skip; OS, overall survival; PSI, Percent-Spliced-In; AP, Alternate Promoter; AT, Alternate Terminator; ME, Mutually Exclusive Exons; RI, Retained Intron; GO, Gene Ontology; KEGG, Kyoto Encyclopedia of Genes and Genomes; LASSO, least absolute shrinkage and selection operator; AIC, Akaike information criterion; BIC, Bayesian information criterion; K-M, Kaplan-Meier; AUC, area under the curve; KPS, Karnofsky performance score; DCA, decision curve analysis; HR, hazard ratio; CI, confidence interval; BP, biological process; CC, cellular component; MF, molecular function; MKNK2, manipulation of the kinase Mnk2; SSO, splice-switching oligonucleotides.
References
1. Ostrom QT, Gittleman H, Liao P, Vecchione-Koval T, Wolinsky Y, Kruchko C, et al. CBTRUS statistical report: primary brain and other central nervous system tumors diagnosed in the United States in 2010-2014. Neuro Oncol. (2017) 19:v1–88. doi: 10.1093/neuonc/nox158
2. Wesseling P, Capper D. WHO 2016 Classification of gliomas. Neuropathol Appl Neurobiol. (2018) 44:139–50. doi: 10.1111/nan.12432
3. Sepulveda-Sanchez JM, Munoz Langa J, Arraez MA, Fuster J, Hernandez Lain A, Reynes G, et al. SEOM clinical guideline of diagnosis and management of low-grade glioma 2017. Clin Transl Oncol. (2018) 20:3–15. doi: 10.1007/s12094-017-1790-3
4. Lacroix M, Abi-Said D, Fourney DR, Gokaslan ZL, Shi W, DeMonte F, et al. A multivariate analysis of 416 patients with glioblastoma multiforme: prognosis, extent of resection, and survival. J Neurosurg. (2001) 95:190–8. doi: 10.3171/jns.2001.95.2.0190
5. Maher EA, Brennan C, Wen PY, Durso L, Ligon KL, Richardson A, et al. Marked genomic differences characterize primary and secondary glioblastoma subtypes and identify two distinct molecular and clinical secondary glioblastoma entities. Cancer Res. (2006) 66:11502–13. doi: 10.1158/0008-5472.CAN-06-2072
6. Parsons DW, Jones S, Zhang X, Lin JC, Leary RJ, Angenendt P, et al. An integrated genomic analysis of human glioblastoma multiforme. Science. (2008) 321:1807–12. doi: 10.1126/science.1164382
7. Nilsen TW, Graveley BR. Expansion of the eukaryotic proteome by alternative splicing. Nature. (2010) 463:457–63. doi: 10.1038/nature08909
8. Ge Y, Porse BT. The functional consequences of intron retention: alternative splicing coupled to NMD as a regulator of gene expression. Bioessays. (2014) 36:236–43. doi: 10.1002/bies.201300156
9. Singh B, Eyras E. The role of alternative splicing in cancer. Transcription. (2017) 8:91–8. doi: 10.1080/21541264.2016.1268245
10. Climente-Gonzalez H, Porta-Pardo E, Godzik A, Eyras E. The functional impact of alternative splicing in cancer. Cell Rep. (2017) 20:2215–26. doi: 10.1016/j.celrep.2017.08.012
11. Dvinge H, Kim E, Abdel-Wahab O, Bradley RK. RNA splicing factors as oncoproteins and tumour suppressors. Nat Rev Cancer. (2016) 16:413–30. doi: 10.1038/nrc.2016.51
12. Mogilevsky M, Shimshon O, Kumar S, Mogilevsky A, Keshet E, Yavin E, et al. Modulation of MKNK2 alternative splicing by splice-switching oligonucleotides as a novel approach for glioblastoma treatment. Nucleic Acids Res. (2018) 46:11396–404. doi: 10.1093/nar/gky921
13. Aldave G, Gonzalez-Huarriz M, Rubio A, Romero JP, Ravi D, Minana B, et al. The aberrant splicing of BAF45d links splicing regulation and transcription in glioblastoma. Neuro Oncol. (2018) 20:930–41. doi: 10.1093/neuonc/noy007
14. Babenko VN, Gubanova NV, Bragin AO, Chadaeva IV, Vasiliev GV, Medvedeva IV, et al. Computer analysis of glioma transcriptome profiling: alternative splicing events. J Integr Bioinform. (2017) 14:20170022. doi: 10.1515/jib-2017-0022
15. Ryan MC, Cleland J, Kim R, Wong WC, Weinstein JN. SpliceSeq: a resource for analysis and visualization of RNA-Seq data on alternative splicing and its functional impacts. Bioinformatics. (2012) 28:2385–7. doi: 10.1093/bioinformatics/bts452
16. Linden A, Yarnold PR. Modeling time-to-event (survival) data using classification tree analysis. J Eval Clin Pract. (2017) 23:1299–308. doi: 10.1111/jep.12779
17. Lex A, Gehlenborg N, Strobelt H, Vuillemot R, Pfister H. UpSet: visualization of intersecting sets. IEEE Trans Vis Comput Graph. (2014) 20:1983–92. doi: 10.1109/TVCG.2014.2346248
18. Huang da W, Sherman BT, Lempicki RA. Systematic and integrative analysis of large gene lists using DAVID bioinformatics resources. Nat Protoc. (2009) 4:44–57. doi: 10.1038/nprot.2008.211
19. Ashburner M, Ball CA, Blake JA, Botstein D, Butler H, Cherry JM, et al. Gene ontology: tool for the unification of biology. The gene ontology consortium. Nat Genet. (2000) 25:25–9. doi: 10.1038/75556
20. Kanehisa M, Furumichi M, Tanabe M, Sato Y, Morishima K. KEGG: new perspectives on genomes, pathways, diseases and drugs. Nucleic Acids Res. (2017) 45:D353–61. doi: 10.1093/nar/gkw1092
21. Goeman JJ. L1 penalized estimation in the Cox proportional hazards model. Biom J. (2010) 52:70–84. doi: 10.1002/bimj.200900028
22. Nagashima K, Sato Y. Information criteria for Firth's penalized partial likelihood approach in Cox regression models. Stat Med. (2017) 36:3422–36. doi: 10.1002/sim.7368
23. Zeng JH, Liang L, He RQ, Tang RX, Cai XY, Chen JQ, et al. Comprehensive investigation of a novel differentially expressed lncRNA expression profile signature to assess the survival of patients with colorectal adenocarcinoma. Oncotarget. (2017) 8:16811–28. doi: 10.18632/oncotarget.15161
24. Harrell FE Jr, Lee KL, Mark DB. Multivariable prognostic models: issues in developing models, evaluating assumptions and adequacy, and measuring and reducing errors. Stat Med. (1996) 15:361–87. doi: 10.1002/(SICI)1097-0258(19960229)15:4<361::AID-SIM168>3.0.CO;2-4
25. Alba AC, Agoritsas T, Walsh M, Hanna S, Iorio A, Devereaux PJ, et al. Discrimination and calibration of clinical prediction models: users' guides to the medical literature. JAMA. (2017) 318:1377–84. doi: 10.1001/jama.2017.12126
26. Qian Z, Li Y, Fan X, Zhang C, Wang Y, Jiang T, et al. Prognostic value of a microRNA signature as a novel biomarker in patients with lower-grade gliomas. J Neurooncol. (2018) 137:127–37. doi: 10.1007/s11060-017-2704-5
27. Vickers AJ, Cronin AM, Elkin EB, Gonen M. Extensions to decision curve analysis, a novel method for evaluating diagnostic tests, prediction models and molecular markers. BMC Med Inform Decis Mak. (2008) 8:53. doi: 10.1186/1472-6947-8-53
28. Brosseau JP, Lucier JF, Nwilati H, Thibault P, Garneau D, Gendron D, et al. Tumor microenvironment-associated modifications of alternative splicing. RNA. (2014) 20:189–201. doi: 10.1261/rna.042168.113
29. Ferrarese R, Harsh GRT, Yadav AK, Bug E, Maticzka D, Reichardt W, et al. Lineage-specific splicing of a brain-enriched alternative exon promotes glioblastoma progression. J Clin Invest. (2014) 124:2861–76. doi: 10.1172/JCI68836
30. He RQ, Zhou XG, Yi QY, Deng CW, Gao JM, Chen G, et al. Prognostic signature of alternative splicing events in bladder urothelial carcinoma based on spliceseq data from 317 cases. Cell Physiol Biochem. (2018) 48:1355–68. doi: 10.1159/000492094
31. Song J, Liu YD, Su J, Yuan D, Sun F, Zhu J. Systematic analysis of alternative splicing signature unveils prognostic predictor for kidney renal clear cell carcinoma. J Cell Physiol. (2019) 234:22753–64. doi: 10.1002/jcp.28840
Keywords: glioblastoma, alternative splicing, splicing factor, prognostic model, TCGA
Citation: Wang Z, Gao L, Guo X, Feng C, Lian W, Deng K and Xing B (2020) Development of a Nomogram With Alternative Splicing Signatures for Predicting the Prognosis of Glioblastoma: A Study Based on Large-Scale Sequencing Data. Front. Oncol. 10:1257. doi: 10.3389/fonc.2020.01257
Received: 11 November 2019; Accepted: 18 June 2020;
Published: 22 July 2020.
Edited by:
Liam Chen, Johns Hopkins University, United StatesReviewed by:
Alireza Mansouri, Pennsylvania State University (PSU), United StatesYuming Jiang, Stanford University, United States
Copyright © 2020 Wang, Gao, Guo, Feng, Lian, Deng and Xing. This is an open-access article distributed under the terms of the Creative Commons Attribution License (CC BY). The use, distribution or reproduction in other forums is permitted, provided the original author(s) and the copyright owner(s) are credited and that the original publication in this journal is cited, in accordance with accepted academic practice. No use, distribution or reproduction is permitted which does not comply with these terms.
*Correspondence: Bing Xing, eGluZ2JpbmdlbWFpbCYjeDAwMDQwO2FsaXl1bi5jb20=