- 1The First Clinical Medical College of Zhejiang Chinese Medical University, Hangzhou, China
- 2Department of Radiology, The First Affiliated Hospital of Zhejiang Chinese Medical University, Hangzhou, China
- 3School of Mathematical Sciences, Zhejiang University, Hangzhou, China
Background: The detection rate for pulmonary nodules, particularly subsolid nodules (SSNs), has been significantly improved. The purpose of this review is to summarize the relationship between quantitative features of initial CT imaging and the subsequent natural growth of SSNs to explore potential reasons for these findings.
Methods: Relevant studies were collected from a literature search of PubMed, Embase, Web of Science, and Cochrane. Data extraction was performed on the patients' basic information, CT methods, and acquisition methods, including quantitative CT features, and statistical methods.
Results: A total of 10 relevant articles were included in our review, which included 850 patients with 1,026 SSNs. Overall, the results were variable, and the key findings were as follows. Seven studies looked at the relationship between the diameter and growth of SSNs, showing that SSNs with larger diameters were associated with increased growth. An additional three studies which focused on the relationship between CT attenuation and the growth of SSNs showed that SSNs with a high CT attenuation were associated with increased growth.
Conclusion: CT attenuation may be useful in predicting the natural growth of SSNs, and mean CT attenuation may be more useful in predicting the natural growth of pure ground glass nodules (GGNs) than part-solid GGNs. While evaluation by diameter did have some limitations, it demonstrates value in predicting the growth of SSNs.
Introduction
Lung cancer is one of the most common malignancies in the world, with a high mortality rate (1). Thanks to the advent of low-dose CT scanning, early detection of lung cancer has become increasingly accurate and the detection rate of pulmonary nodules has been significantly improved, particularly the subsolid nodules (SSNs) (2, 3).
The SSN is defined as a hazy, hyperdense nodule on lung windows without obscurity to bronchovesicular structures (4), which includes pure ground glass nodules (GGNs) and part-solid GGNs. The pathophysiology of SSNs is derived from thickening of the alveolar walls and septal interstitium or accumulation of fluid, cells, or amorphous material in the alveoli itself (5). Many lesions can be defined as SSNs, such as benign and malignant tumors, inflammatory lesions, and interstitial lung disease. However, persistent SSNs mainly consist of atypical adenomatous hyperplasia (AAH), adenocarcinoma in situ (AIS), minimally invasive adenocarcinoma (MIA), and invasive adenocarcinoma (IAC). These lesions are difficult to distinguish in CT imaging (6, 7). Moreover, their malignant transformation rate from preinvasive lesions to invasive lesions may reach up to 34%. Yet the potential biological basis for this transformation is not fully understood (8). The performance of current CT imaging modalities is also insufficient to differentiate these lesions. Additionally, most SSNs are small with low metabolic rates, leading to a high false-negative rate using PET/CT (9).
In predicting the course of lung disease, the growth of SSNs is commonly used as one of the key prognostic indicators for disease severity. Moreover, in 2017, the Fleischner Society released new guidelines for management of SSNs, suggesting that most nodules do require subsequent follow-up (10). While radiological observation of SSNs in follow-up CT examinations is easily doable, the changes of SSNs occur on a slow timescale, making it difficult to ascertain any discernible difference between short follow-up periods (11). This daunting waiting game is a significant cause of stress and anxiety for patients and their families. Therefore, the purpose of this review is to summarize the relationship between the quantitative features of SSN CT imaging with the future growth of SSNs. Furthermore, we seek to explore potential diagnostic indicators which can accurately predict the course and history of SSNs for further study (Figure 1).
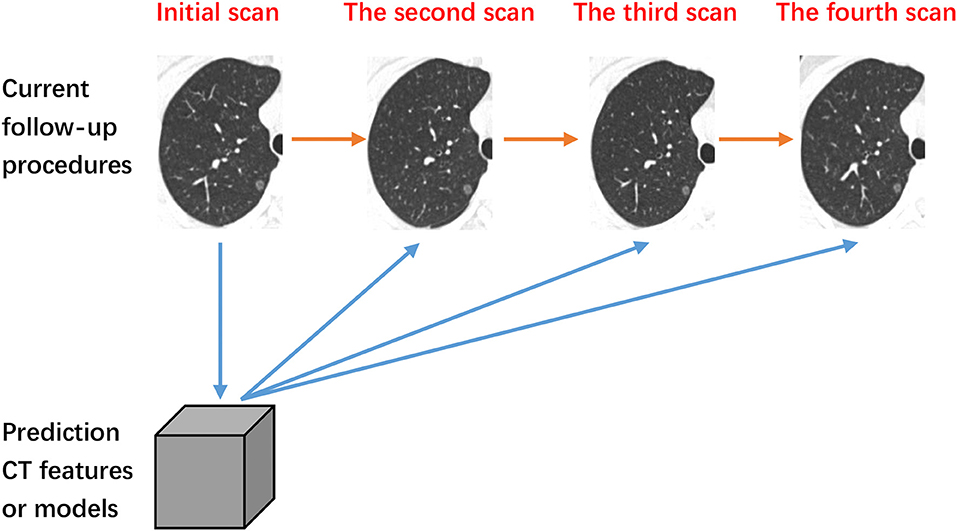
Figure 1. The process of follow-up. The orange line denotes current follow-up procedures. The blue lines denote our methodology of predicating the change of subsolid nodules based on initial quantitative CT features.
Methods
We conducted systematic literature searches using PubMed, Embase, Web of Science, and Cochrane from inception to October 2018. Search strategies are shown in the Supplemental Materials. No year and language restrictions were applied. These reference literatures from the selected articles were also included in additional studies to review, which were relevant to our topic. The protocol of this review was registered on PROSPERO (http://www.crd.york.ac.uk/PROSPERO; CRD42019124138).
After duplicates were eliminated, articles were selected which met the following criteria based on their title and abstract: (1) relate to the SSNs; (2) relate to the growth of primary lung cancers, lung nodules, or SSNs; and (3) study quantitative CT features associated with growth of SSNs. Some studies were excluded, including artificial nodule studies, those undergoing intervention before follow-up or during the follow-up, animal research studies, reviews, case reports, conference abstracts, comments, editorials, letters, and guidelines. The selected articles were then analyzed in full text. The title and abstract of studies retrieved using the search strategy and those from additional sources will be screened independently by two review authors to identify studies that potentially meet the inclusion criteria outlined above. The full text of these potentially eligible studies will be retrieved and independently assessed for eligibility by two review team members. Any disagreement between them over the eligibility of particular studies will be resolved through discussion with a third reviewer.
Data analysis included the patient population, year, data inclusion criteria, the number of nodules, types of nodules, CT scanner, reconstruction algorithm, reconstruction slice thickness, respiratory control, acquisition of CT quantitative features, statistical models, outcome features, assessment of SSNs, CT quantitative features, results, and key findings. Two team members independently extracted and organized data, and the remaining team members were consulted in order to resolve discrepancies in the data synthesis process.
Results
After searching databases using the aforementioned strategy, a total of 2,157 records were found. Twenty-six studies from the reference of 2,157 selected articles were also included in additional studies. Then, 717 duplicate references were excluded, and 1,466 articles were analyzed using their titles and abstracts. The process was described in Figure 2. A total of 98 studies were examined in full text. Finally, 10 relevant articles were included in this review (Tables 1,2).
Subjects
For these 10 studies, a total of 850 patients with 1,026 SSNs were recruited. Eight of ten studies examined a sex ratio of patients, and the other two (13, 21) examined a sex ratio of nodules (Table 1). The number of nodules was equal to the number of patients in two of the studies (14, 18). In the study conducted by Kobayashi et al. (20), the number of nodules is 120, which is about twice the number of patients. Four studies (12–15) only included pure GGNs, and the other six studies (16–21) included both pure GGNs and part-solid GGNs. The results of five studies (16–20) were based on all types of SSNs, while one study (21) was based on each type of SSNs.
CT Parameters
Three studies (12, 13, 18) used a single CT scan, five studies (15–17, 20, 21) used two different CT scans, and two studies (14, 19) used three different CT scans (Table 1). Only one study (12) showed all reconstruction algorithms using a bone algorithm. Three studies (13, 14, 16) only used a single reconstruction thickness, which was 2, 1.25, and 1 mm, for the respective studies. Three studies (15, 16, 18) conducted CT scans at the end inspiration, and one study (13) conducted the CT scan at mid-inspiration during one breath hold.
Classification of SSNs
One study (15) defined pure ground glass opacities (GGOs) or mixed GGO based on the tumor shadow disappearance rate (TDR). Nine studies (12–14, 16–21) used visual assessments in the definitions of SSNs (Table 2).
Definitions of SSN Changes
Three studies (15, 19, 20) defined a change in SSNs as an increase in diameter ≥2 mm. Three different studies (12–14) defined a change in SSNs using the parameter above and/or the presence of an emerging solid portion. Two more studies (18, 21) defined a change in SSNs as the two parameters mentioned above and/or an increase in the solid parts' size. Two studies (16, 17) used volume doubling time (VDT) and/or mass doubling time (MDT) to define changes of SSNs.
Features of Quantitative CT Imaging
Acquisition
Three studies (12, 16, 17) first used a segmented region of interest (ROI), followed by extraction of quantitative CT features (Table 1). Four studies (13, 18, 20, 21) acquired quantitative features using manual measurement without segmentation of ROI. Two studies (15, 19) did not mention their acquisition methods.
Diameter
All 10 articles studied the relationship between diameter and the growth or growth rate of SSNs. Four out of the 10 articles only included diameter as their quantitative feature (Table 2). One article (12) used the diameter of the GGO component and the diameter of the solid component in place of the diameter of the whole SSNs. The results of six studies (14, 15, 18–21) showed that SSNs with large diameters were associated with increased growth. On the contrary, two studies (12, 13) pointed to no significant relationship between the diameter of SSNs and their growth, and the results of two other studies (16, 17) showed that there was no significant relationship between the diameter of SSNs and their growth rate.
CT Attenuation
Six articles (12–14, 16–18) studied the relationship between CT attenuation and the growth or growth rate of SSNs (Table 2). Five articles (13, 14, 16–18) used mean CT attenuation as their measure. However, one study (12) solely relied on the histogram of CT attenuation without mean CT attenuation. The results of two articles (13, 14) showed that SSNs with large mean CT attenuation was associated with increased growth. The results of study 17 showed that SSNs with large mean CT attenuation were associated with low VDT. However, study 18 showed no significant relationship between the mean CT attenuation of initial SSNs and their subsequent growth. Study 16 showed no significant relationship between the mean CT attenuation of initial SSNs and their growth rate.
Volume
Two studies (12, 16) used volume as a signature to predict the growth or growth rate of SSNs (Table 2). Studies 12 and 16 pointed to no significant relationship between the initial volume and growth or growth rate of SSNs.
Mass
Two articles (12, 16) studied the relationship between mass and the growth or growth rate of SSNs and indicated no significant relationship (Table 2).
Results of Different Types of SSNs
In studies (16–20) in which the results were based on all types of SSNs (Table 2), three articles (18–20) studied the relationship between the diameter and the growth of SSNs. The results of three articles (18–20) showed significant differences in diameter between rapidly growing and non-growing SSNs. And in studies (12–15, 21) in which the results were based on pure GGNs (Table 2), all articles studied the relationship between diameter and the growth of pure GGNs. However, only three articles (14, 15, 21) showed significant differences in diameter between rapidly growing and non-growing pure GGNs.
In studies (12–14) whose results were based on pure GGNs (Table 2), all articles showed significant differences in CT attenuation between rapidly growing and non-growing GGNs.
Discussion
In this study, we reviewed the relationship between quantitative features of initial CT imaging and the growth of SSNs. Quantitative features, such as diameter, CT attenuation, volume, and mass, were investigated in 10 articles. The majority of these articles pointed to at least one significant correlation between a quantitative feature and the growth of SSNs.
In five studies (16–20), three articles (18–20) studied the relationship between diameter and the growth of SSNs, and two articles (16, 17) studied the relationship between diameter and the growth rate of SSNs. The results of three articles (18–20) all showed significant differences in diameter between rapidly growing and non-growing SSNs. However, the results of the other two articles (16, 17) showed there is no significant correlation between diameter and VDT. In another five studies (12–15, 21), all articles noted the relationship between diameter and the growth of pure GGNs. However, only three of these articles (14, 15, 21) showed there were significant differences in diameter between rapidly growing and non-growing pure GGNs. The reason is as follows: firstly, as a two-dimensional parameter, the diameter does have some limitations. For example, most nodules are asymmetrical (22), making diameter an inaccurate representation of the entire SSNs. Secondly, this may be attributable to the small sample size and/or lead time bias from varying lengths of follow-up (23, 24). VDT on the other hand included information about the change of volume and the length of follow-up. Many studies used VDT to estimate the growth of a nodule, to classify tumors, and to evaluate malignancy (17, 25). Moreover, the growth pattern of malignant nodules is exponential (26), and SSNs with large diameters will grow faster than their smaller counterparts. Thus, we believe that measurement parameters such as diameter or volume will have value in predicting the growth of SSNs.
Most AISs/MIAs were displayed as GGO, and IACs were also found to be solid nodules (27–30). CT attenuation of GGNs was related with the physical cell density (31). The results of Barletta et al. (32) suggested that the solid pattern of SSNs was associated with cytologic atypia and tumor growth. Following this train of thought, SSNs with greater CT attenuation are more likely to be rapidly growing or malignant. And the results of three selected articles supported this view (12–14). In these studies (12–14) in which the results were based on pure GGNs, all articles showed significant differences in CT attenuation between rapidly growing and non-growing SSNs. However, two studies (13, 14) included mean CT attenuation into their analyses, and one study (12) included a histogram of CT attenuation. The mean CT attenuation only represented the overall density of nodules while other indicators, such as max CT attenuation, standard deviation CT attenuation, and a histogram of CT attenuation, represented information about the heterogeneity of SSNs. It was found that SSNs with a high degree of heterogeneity was malignant and invasive (33–35). Therefore, SSNs with large mean CT attenuations and great heterogeneity will grow even more rapidly, indicating a poor prognosis. Thus, mean CT attenuation, max CT attenuation, standard deviation of CT attenuation, and a histogram of CT attenuation may be useful in predicting the growth of SSNs.
Interestingly, in two studies (16, 17) in which the results were based on all types of SSNs, the two articles also studied the relationship between mean CT attenuation and the growth rate of SSNs. Only one study (17) showed that SSNs with greater CT attenuation are more likely to be rapidly growing. This may be the cause in the different rates of two types of SSNs. In the study (16), they included seven pure GGNs and 15 part-solid GGNs. And in another study (17), they included 28 pure GGNs and 19 part-solid GGNs. In study (16), they mentioned that some part-solid GGNs showed a reduction in mean CT attenuation during the follow-up. If the growth of the GGO component in part-solid GGNs is greater than the growth of the solid component, it might reduce mean CT attenuation. Thus, mean CT attenuation may be more useful in predicting the growth of pure GGNs than part-solid GGNs.
There are several limitations to the current study. Firstly, few cases were included in selected studies. The small sample size negatively impacts the credibility of those studies (23, 24). Among the reasons may be that inclusion criteria are strict and follow-up is longer. Another reason may be that patients with SSNs are simply too anxious to endure long periods of follow-up and will request biopsy or surgery for definitive diagnosis. Another limitation is regarding the variable inclusion criteria among articles. Some articles only included SSNs with confirmed pathology while some also included patients with a history of cancer. Thirdly, the length of follow-up was variable. If the follow-up time is insufficient, the growth of SSNs may be attributed to a measurement error. Fourthly, studies used different reconstruction methods or scanning machines, which may affect the value of measured CT features (36). Lastly, while these quantitative CT imaging features may predict the growth of SSNs, the underlying causes and natural history of SSNs have not yet been fully understood.
Several challenges are worth noting. The segmentation of SSNs was used in most studies, including manual, semiautomatic, and automatic segmentation. At present, manual segmentation is considered the gold standard. However, manual segmentation is time-consuming and suffers from interobserver variation (37, 38). Physicians pay more attention to semiautomatic segmentation as the new standard. LungCARE software, for instance, uses two algorithms (sharp, B60F; medium sharp, B50F), to segment pure GGNs. The ratios of successful segmentation were a staggering 98.3 and 97.8% with sharp and medium sharp reconstruction algorithms, respectively (39). However, the boundary between SSNs and the surrounding lung parenchyma is hazy, and the size and CT attenuation of SSNs are susceptible to changes in respiration (40, 41). Thus, the performance of said software models needs to be verified in further studies, and the segmentation technology definitely could benefit from further development. Secondly, quantitative CT features such as diameter, volume, and CT attenuation are common and visible. With the development of computer technology, many radiologists attach importance to the radiomic features which are quantitatively extracted from medical imaging, such as the gray-level co-occurrence matrix (GLCM) and run-length matrix (RLM) (42). A study by Wang et al. (43) retrospectively illustrated the potential predictive power of quantitative features to differentiate malignant from benign lung nodules. One hundred fifty radiomic features were extracted from 593 patients' CT imaging and shown to poise predictive power using a support vector machine. For their training group and testing group, the sensitivity and specificity of diagnosis were 82.5% sens. (165 of 200), 89.5% spec. (179 of 200), and 74.6% sens. (91 of 122), 78.9% spec. (56 of 71), respectively. Radiomic features may be of value in improving the predictive model and growth model of SSNs.
Conclusion
This study examined the predictive power of quantitative CT imaging features for predicting the growth of SSNs across several studies. While using the diameter of SSNs as a prognostic indicator did have some limitation, it demonstrated value in predicting the growth of SSNs. CT attenuation may be useful in predicting the growth of SSNs, and mean CT attenuation may be more useful in predicting the growth of pure GGNs than part-solid GGNs. Accurate and timely detection of SSNs and their plausible growth can offer great value in directing appropriate treatment options and lowering patient anxiety. Further studies are needed to explore the predictive potential of quantitative CT imaging and radiomic features to understand the natural history of SSNs.
Data Availability Statement
The datasets analyzed in this article are not publicly available. Requests to access the datasets should be directed to Maosheng Xu, xums166@zcmu.edu.cn.
Author Contributions
CG: manuscript draft, search strategy, literature review, study selection, data extraction, review of final draft. JL: literature review, study selection, data extraction. LW: literature review, study selection, data extraction. DK: review of final draft. CZ: conception and design of the study, search strategy, review of final draft. MX: conception and design of the study, search strategy, and review of final draft.
Funding
This work was supported by the Zhejiang Provincial Natural Science Foundation of China (LSY19H180003) and the Medical Health Science and Technology Project of Zhejiang Provincial Health Commission (2019KY117).
Conflict of Interest
The authors declare that the research was conducted in the absence of any commercial or financial relationships that could be construed as a potential conflict of interest.
Acknowledgments
The authors thank Michael C. Lin for language editing.
Supplementary Material
The Supplementary Material for this article can be found online at: https://www.frontiersin.org/articles/10.3389/fonc.2020.00318/full#supplementary-material
References
1. Siegel RL, Miller KD, Jemal A. Cancer statistics 2017. CA Cancer J Clin. (2017) 67:7–30. doi: 10.3322/caac.21387
2. Kakinuma R, Ohmatsu H, Kaneko M, Kusumoto M, Yoshida J, Nagai K, et al. Progression of focal pure ground glass opacity detected by low-dose helical computed tomography screening for lung cancer. J Comput Assist Tomogr. (2004) 28:17–23. doi: 10.1097/00004728-200401000-00003
3. Kovalchik SA, Tammemagi M, Berg CD, Caporaso NE, Riley TL, Korch M, et al. Targeting of low-dose CT screening according to the risk of lung-cancer death. N Engl J Med. (2013) 369:245–54. doi: 10.1056/NEJMoa1301851
4. Collins J, Stern EJ. Ground glass opacity at CT: the ABCs. AJR Am J Roentgenol. (1997) 169:355–67. doi: 10.2214/ajr.169.2.9242736
5. Engeler CE, Tashjian JH, Trenkner SW, Walsh JW. Ground glass opacity of the lung parenchyma: a guide to analysis with high-resolution CT. AJR Am J Roentgenol. (1993) 160:249–51. doi: 10.2214/ajr.160.2.8424326
6. Kim HY, Shim YM, Lee KS, Han J, Yi CA, Kim YK. Persistent pulmonary nodular ground glass opacity at thin-section CT: histopathologic comparisons. Radiology. (2007) 245:267–75. doi: 10.1148/radiol.2451061682
7. Diederich S. Pulmonary nodules: do we need a separate algorithm for non-solid lesions? Cancer Imaging. (2009) 9:S126–8. doi: 10.1102/1470-7330.2009.9046
8. Henschke CI, Yankelevitz DF, Mirtcheva R, McGuinness G, McCauley D, Miettinen OS. CT screening for lung cancer: frequency and significance of part-solid and nonsolid nodules. AJR Am J Roentgenol. (2002) 178:1053–7. doi: 10.2214/ajr.178.5.1781053
9. Kim TJ, Park CM, Goo JM, Lee KW. Is there a role for FDG PET in the management of lung cancer manifesting predominantly as ground glass opacity? AJR Am J Roentgenol. (2012) 198:83–8. doi: 10.2214/AJR.11.6862
10. MacMahon H, Naidich DP, Goo JM, Lee KS, Leung ANC, Mayo JR, et al. Guidelines for management of incidental pulmonary nodules detected on CT images: from the fleischner society 2017. Radiology. (2017) 284:228–43. doi: 10.1148/radiol.2017161659
11. Lee HJ, Goo JM, Lee CH, Yoo CG, Kim YT, Im JG. Nodular ground glass opacities on thin-section CT: size change during follow-up and pathological results. Korean J Radiol. (2007) 8:22–31. doi: 10.3348/kjr.2007.8.1.22
12. Bak SH, Lee HY, Kim JH, Um SW, Kwon OJ, Han J, et al. Quantitative CT scanning analysis of pure ground glass opacity nodules predicts further CT scanning change. Chest. (2016) 149:180–91. doi: 10.1378/chest.15-0034
13. Tamura M, Shimizu Y, Yamamoto T, Yoshikawa J, Hashizume Y. Predictive value of one-dimensional mean computed tomography value of ground glass opacity on high-resolution images for the possibility of future change. J Thorac Oncol. (2014) 9:469–72. doi: 10.1097/JTO.0000000000000117
14. Eguchi T, Kondo R, Kawakami S, Matsushita M, Yoshizawa A, Hara D, et al. Computed tomography attenuation predicts the growth of pure ground glass nodules. Lung Cancer. (2014) 84:242–7. doi: 10.1016/j.lungcan.2014.03.009
15. Chang B, Hwang JH, Choi YH, Chung MP, Kim H, Kwon OJ, et al. Natural history of pure ground glass opacity lung nodules detected by low-dose CT scan. Chest. (2013) 143:172–8. doi: 10.1378/chest.11-2501
16. Borghesi A, Farina D, Michelini S, Ferrari M, Benetti D, Fisogni S, et al. Pulmonary adenocarcinomas presenting as ground glass opacities on multidetector CT: three-dimensional computer-assisted analysis of growth pattern and doubling time. Diagn Interv Radiol. (2016) 22:525–33. doi: 10.5152/dir.2016.16110
17. Oda S, Awai K, Murao K, Ozawa A, Utsunomiya D, Yanaga Y, et al. Volume-doubling time of pulmonary nodules with ground glass opacity at multidetector CT: assessment with computer-aided three-dimensional volumetry. Acad Radiol. (2011) 18:63–9. doi: 10.1016/j.acra.2010.08.022
18. Hiramatsu M, Inagaki T, Inagaki T, Matsui Y, Satoh Y, Okumura S, et al. Pulmonary ground glass opacity (GGO) lesions-large size and a history of lung cancer are risk factors for growth. J Thorac Oncol. (2008) 3:1245–50. doi: 10.1097/JTO.0b013e318189f526
19. Lee SW, Leem CS, Kim TJ, Lee KW, Chung JH, Jheon S, et al. The long-term course of ground glass opacities detected on thin-section computed tomography. Respir Med. (2013) 107:904–10. doi: 10.1016/j.rmed.2013.02.014
20. Kobayashi Y, Sakao Y, Deshpande GA, Fukui T, Mizuno T, Kuroda H, et al. The association between baseline clinical-radiological characteristics and growth of pulmonary nodules with ground glass opacity. Lung Cancer. (2014) 83:61–6. doi: 10.1016/j.lungcan.2013.10.017
21. Matsuguma H, Mori K, Nakahara R, Suzuki H, Kasai T, Kamiyama Y, et al. Characteristics of subsolid pulmonary nodules showing growth during follow-up with CT scanning. Chest. (2013) 143:436–43. doi: 10.1378/chest.11-3306
22. Yanagawa M, Tanaka Y, Kusumoto M, Watanabe S, Tsuchiya R, Honda O, et al. Automated assessment of malignant degree of small peripheral adenocarcinomas using volumetric CT data: correlation with pathologic prognostic factors. Lung Cancer. (2010) 70:286–94. doi: 10.1016/j.lungcan.2010.03.009
23. Figueroa RL, Zeng-treitler Q. Predicting sample size required for classification performance. BMC Med Inform Decis Mak. (2012) 12:8. doi: 10.1186/1472-6947-12-8
24. Tam VH, Kabbara S, Yeh RF, Leary RH. Impact of sample size on the performance of multiple-model pharmacokinetic simulations. Antimicrob Agents Chemother. (2006) 50:3950–2. doi: 10.1128/AAC.00337-06
25. Song YS, Park CM, Park SJ, Lee SM, Jeon YK, Goo JM. Volume and mass doubling times of persistent pulmonary subsolid nodules detected in patients without known malignancy. Radiology. (2014) 273:276–84. doi: 10.1148/radiol.14132324
26. Scharcanski J, da Silva LS, Koff D, Wong A. Interactive modeling and evaluation of tumor growth. J Digit Imaging. (2010) 23:755–68. doi: 10.1007/s10278-009-9234-4
27. Aoki T, Tomoda Y, Watanabe H, Nakata H, Kasai T, Hashimoto H, et al. Peripheral lung adenocarcinoma: correlation of thin-section CT findings with histologic prognostic factors and survival. Radiology. (2001) 220:803–9. doi: 10.1148/radiol.2203001701
28. Ikeda K, Awai K, Mori T, Kawanaka K, Yamashita Y, Nomori H. Differential diagnosis of ground glass opacity nodules: CT number analysis by three-dimensional computerized quantification. Chest. (2007) 132:984–90. doi: 10.1378/chest.07-0793
29. Kodama K, Higashiyama M, Yokouchi H, Takami K, Kuriyama K, Kusunoki Y, et al. Natural history of pure ground glass opacity after long-term follow-up of more than 2 years. Ann Thorac Surg. (2002) 73:383–6. doi: 10.1016/S0003-4975(01)03410-5
30. Travis WD, Garg K, Franklin WA, Wistuba II, Sabloff B, Noguchi M, et al. Evolving concepts in the pathology and computed tomography imaging of lung adenocarcinoma and bronchioloalveolar carcinoma. J Clin Oncol. (2005) 23:3279–87. doi: 10.1200/JCO.2005.15.776
31. Mull RT. Mass estimates by computed tomography: physical density from CT numbers. AJR Am J Roentgenol. (1984) 143:1101–4. doi: 10.2214/ajr.143.5.1101
32. Barletta JA, Yeap BY, Chirieac LR. Prognostic significance of grading in lung adenocarcinoma. Cancer. (2010) 116:659–69. doi: 10.1002/cncr.24831
33. Burrell RA, McGranahan N, Bartek J, Swanton C. The causes and consequences of genetic heterogeneity in cancer evolution. Nature. (2013) 501:338–45. doi: 10.1038/nature12625
34. Swanton C. Intratumor heterogeneity: evolution through space and time. Cancer Res. (2012) 72:4875–82. doi: 10.1158/0008-5472.CAN-12-2217
35. Lee HY, Jeong JY, Lee KS, Kim HJ, Han J, Kim BT, et al. Solitary pulmonary nodular lung adenocarcinoma: correlation of histopathologic scoring and patient survival with imaging biomarkers. Radiology. (2012) 264:884–93. doi: 10.1148/radiol.12111793
36. Ko JP, Rusinek H, Jacobs EL, Babb JS, Betke M, McGuinness G, et al. Small pulmonary nodules: volume measurement at chest CT–phantom study. Radiology. (2003) 228:864–70. doi: 10.1148/radiol.2283020059
37. Thawani R, McLane M, Beig N, Ghose S, Prasanna P, Velcheti V, et al. Radiomics and radiogenomics in lung cancer: a review for the clinician. Lung Cancer. (2018) 115:34–41. doi: 10.1016/j.lungcan.2017.10.015
38. Kumar V, Gu Y, Basu S, Berglund A, Eschrich SA, Schabath MB, et al. Radiomics: the process and the challenges. Magn Reson Imaging. (2012) 30:1234–48. doi: 10.1016/j.mri.2012.06.010
39. Park CM, Goo JM, Lee HJ, Kim KG, Kang MJ, Shin YH. Persistent pure ground glass nodules in the lung: interscan variability of semiautomated volume and attenuation measurements. AJR Am J Roentgenol. (2010) 195:W408–14. doi: 10.2214/AJR.09.4157
40. de Hoop B, Gietema H, van de Vorst S, Murphy K, van Klaveren RJ, Prokop M. Pulmonary ground glass nodules: increase in mass as an early indicator of growth. Radiology. (2010) 255:199–206. doi: 10.1148/radiol.09090571
41. Goo JM, Kim KG, Gierada DS, Castro M, Bae KT. Volumetric measurements of lung nodules with multi-detector row CT: effect of changes in lung volume. Korean J Radiol. (2006) 7:243–8. doi: 10.3348/kjr.2006.7.4.243
42. Gillies RJ, Kinahan PE, Hricak H. Radiomics: images are more than pictures, they are data. Radiology. (2016) 278:563–77. doi: 10.1148/radiol.2015151169
Keywords: subsolid nodule, ground glass nodule, natural growth, quantitative, CT features, systematic review
Citation: Gao C, Li J, Wu L, Kong D, Xu M and Zhou C (2020) The Natural Growth of Subsolid Nodules Predicted by Quantitative Initial CT Features: A Systematic Review. Front. Oncol. 10:318. doi: 10.3389/fonc.2020.00318
Received: 22 November 2019; Accepted: 21 February 2020;
Published: 27 March 2020.
Edited by:
Mei Lan Tan, University of Science Malaysia, MalaysiaReviewed by:
David Naidich, New York University, United StatesChen Chen, Central South University, China
Copyright © 2020 Gao, Li, Wu, Kong, Xu and Zhou. This is an open-access article distributed under the terms of the Creative Commons Attribution License (CC BY). The use, distribution or reproduction in other forums is permitted, provided the original author(s) and the copyright owner(s) are credited and that the original publication in this journal is cited, in accordance with accepted academic practice. No use, distribution or reproduction is permitted which does not comply with these terms.
*Correspondence: Maosheng Xu, eHVtczE2NiYjeDAwMDQwO3pjbXUuZWR1LmNu; Changyu Zhou, dG9waG9yaXpvbiYjeDAwMDQwO3pjbXUuZWR1LmNu