- 1Department of Radiology, Second Xiangya Hospital, Central South University, Changsha, China
- 2Department of Radiology, Huadong Hospital Affiliated to Fudan University, Shanghai, China
- 3School of Biomedical Engineering, Capital Medical University, Beijing, China
- 4Diagnosis and Treatment Center of Small Lung Nodules of Huadong Hospital, Shanghai, China
- 5Institute of Functional and Molecular Medical Imaging, Fudan University, Shanghai, China
Purpose: Up to 50% of Asian patients with NSCLC have EGFR gene mutations, indicating that selecting eligible patients for EGFR-TKIs treatments is clinically important. The aim of the study is to develop and validate radiomics-based nomograms, integrating radiomics, CT features and clinical characteristics, to non-invasively predict EGFR mutation status and subtypes.
Materials and Methods: We included 637 patients with lung adenocarcinomas, who performed the EGFR mutations analysis in the current study. The whole dataset was randomly split into a training dataset (n = 322) and validation dataset (n = 315). A sub-dataset of EGFR-mutant lesions (EGFR mutation in exon 19 and in exon 21) was used to explore the capability of radiomic features for predicting EGFR mutation subtypes. Four hundred seventy-five radiomic features were extracted and a radiomics sore (R-score) was constructed by using the least absolute shrinkage and selection operator (LASSO) regression in the training dataset. A radiomics-based nomogram, incorporating clinical characteristics, CT features and R-score was developed in the training dataset and evaluated in the validation dataset.
Results: The constructed R-scores achieved promising performance on predicting EGFR mutation status and subtypes, with AUCs of 0.694 and 0.708 in two validation datasets, respectively. Moreover, the constructed radiomics-based nomograms excelled the R-scores, clinical, CT features alone in terms of predicting EGFR mutation status and subtypes, with AUCs of 0.734 and 0.757 in two validation datasets, respectively.
Conclusions: Radiomics-based nomogram, incorporating clinical characteristics, CT features and radiomic features, can non-invasively and efficiently predict the EGFR mutation status and thus potentially fulfill the ultimate purpose of precision medicine. The methodology is a possible promising strategy to predict EGFR mutation subtypes, providing the support of clinical treatment scenario.
Key points
1. We developed and validated two Radiomics-based nomograms, incorporating clinical characteristics, CT features and radiomic features, to non-invasively predict the EGFR mutation status and subtypes with the aim to potentially fulfill the ultimate purpose of precision medicine.
2. The presented results indicate that radiomics-based nomogram may potentially facilitate scalable precision medicine on identifying eligible patients of lung adenocarcinoma for EGFR-targeted therapy.
Introduction
Lung cancer is the leading cause cancer-related death both in male and female (1). Non-small cell lung cancer (NSCLC) accounts for more than 80% of lung cancers, of which lung adenocarcinoma is the most common histological subtype (2). With the advances of genomics, molecular-targeted therapy like using tyrosine kinase inhibitors (TKIs), which targets the epidermal growth factor receptor (EGFR) mutations, is recommended as first-line system therapy before first-line therapy by National Comprehensive Cancer Network (NCCN) for patients with advanced EGFR-mutant NSCLC (2) and proved to substantially improve the progression-free survival (PFS) compared with conventional chemotherapy (3, 4). Up to 50% of Asian patients with NSCLC have EGFR gene mutations (5), indicating that selecting eligible patients for EGFR-TKIs treatments is clinically important. In patients with NSCLC, the most commonly found EGFR mutations are deletions in exon 19 (45%) and in exon 21 (L858R in 40%) in patients with EGFR mutations (2). Both mutations are associated with sensitivity to the small molecule TKIs as well as erlotinib, gefitinib, afatinib, and osimertinib (2), however, with different survival outcomes in response to both EGFR-TKIs and chemotherapy (6). Therefore, identifying EGFR mutation subtypes, especially those responsive to TKI treatment, seems to be more critically and scientifically important than just predicting EGFR mutation status.
In this context, though more and more research has emerged on the non-invasive prediction of EGFR mutation status in recent years (7–9), no predictors are recommended for selecting patients in clinical decision-making. Moreover, substantial discrepancies are presented to date with regarding to some features, especially semantic features derived from medical images (10). Buoyed by the availability of big data and state-of-art data analysis strategy, such as radiomics and deep learning, decoding tumor phenotype to precisely predict genotype has becoming the point of attention (11). Several studies have investigated the potential ability of radiomics to non-invasively predicting EGFR mutation status and show promising results (12–15). Few results are finally applied in clinical practice yet due to the complicated procedure (e.g., time consuming, poor reproducibility, remaining the operator-dependency that is not biases-free, and so on) of radiomic researches (16). In view of this, models that giving an individual numerical probability of a clinical event (e.g., nomogram) rather than a predicting accuracy, may be more suitable and convenient for clinical application.
In the current study, we aim to build radiomics-based nomograms, integrating radiomics, CT imaging features and clinical characteristics, to non-invasively predict EGFR mutation status and subtypes (exon 19 and 21mutation).
Materials and Methods
Patient Selection and Dataset Preparation
This retrospective study was approved by the institutional review board (No. 20170103), which waived the requirement for patients' informed consent referring to the CIOMS guideline. The flowchart of our study was showed in Figure 1.
A search of Picture Achieving and Communication System (PACS) and pathological system from January 2013 to June 2018 was performed by one author with the following inclusion criteria: (1) Available thin-slice chest CT (<1.5 mm) images before biopsies or surgical treatment; (2) Available detailed histological reports of adenocarcinoma; (3) Available detailed EGFR mutations testing reports; (4) No any prior treatment before EGFR mutations analysis.
Finally, 637 patients were included. Of the 637 lesions, 342 lesions tested positively for an EGFR mutation (EGFR Mut), where 295 lesions were classified as wild-type lung adenocarcinomas (EGFR WT). Note that only one malignant nodule was studied for each patient due to the availability of EGFR testing report. Among the 342 patients with EGFR Mut, 130 patients were detected an EGFR Mut in exon 19, whereas 190 patients were detected an EGFR Mut in exon 21. The clinical and histopathologic variables, including age, sex, smoking status, tumor size, tumor location, histological subtypes etc. were presented in Table 1.
Two tasks were investigated in the current study: task (a), differentiating EGFR Mut from EGFR WT; task (b), differentiating EGFR Mut in exon 19 from in exon 21. Each dataset in two tasks (n = 637 and n = 320) was split into 10 groups (1–10), each subset was randomly selected by choosing 10% of each of the 2 categories. In task (a), groups 1–5 were defined as training dataset, the rest groups were defined as validation dataset. In task (b), considering the insufficient training data, groups 1–6 were defined as training dataset, the rest groups were defined as validation dataset. Note that we only included EGFR mutations in exon 19 and 21 in task b to avoid the sparse training data and the disbalance of data distribution. Mover, constructing a model to predict these two exons in patients with EGFR mutation is clinically reasonable (see Introduction).
CT Acquisition and Imaging Interpretation
All included patients were performed with the following six scanners: GE Discovery CT750 HD, 64-slice LightSpeed VCT, Revolution CT (GE Medical Systems); Somatom Definition flash, Somatom Sensation-16, Somatom Force (Siemens Medical Solutions). The acquisition parameters were as follows: 120 kVp; 100– 200 mAs; pitch, 0.75–1.5; and collimation, 1–1.5 mm, respectively. All imaging data were reconstructed by using a medium sharp reconstruction algorithm with a thickness of 0.75–1.5 mm. CT images were acquired in the supine position at full inspiration for all patients. Only plain CT images were used in the current study. Two radiologists (with 12 and 3 years of experience in chest CT interpretation) independently interpreted the CT images, blinded to clinical and histologic findings. Thirteen CT features (Table 1) were evaluated. The definitions of these features were described in previous study (17, 18). A re-evaluation for achieving a consensus was performed to solve the disagreement between two radiologists.
Segmentation and Radiomic Features Extraction
All nodules were manually delineated slice by slice using a medical image processing and navigation software 3D Slicer (version 4.8.0, Brigham and Women's Hospital) by one author (with 5 years of experience in chest CT interpretation), then the volume of interests (VOIs) were confirmed by another radiologist (with 12 years of experience in chest CT interpretation). Fifty randomly selected nodules were independently segmented by the two authors for feature reproductive analysis. Images and VOIs with NII format were exported for further analysis. Radiomic features from three categories, including 50 gray-level histogram features, 325 gray-level co-occurrence matrix (GLCM) features, and 100 gray-level run lengths matrix (GLRLM) features, were extracted using Matlab 2016b (MathWorks, Natick, USA). The details of extracted radiomic features were presented in Supplementary Data. Radiomic feature extraction methodology was described in our previous study (18).
Features Selection and Radiomic Score Construction
The least absolute shrinkage and selection operator (LASSO) method, which is an accepted algorithm for feature selection in high-dimensional variables (19), was applied to select the features that were most distinguishable and build a logistic regression model in training dataset. Then a radiomic score (R-score) was calculated for each lesion using features selected by LASSO and weighted by the respective coefficients.
Nomogram Construction and Validation
Univariate analysis was firstly to identify the potential predictors among clinical characteristics, CT features and R-score. Factors that associated with EGFR mutation status and subtypes were then included to multivariate analysis to identify the independent predictors. Furthermore, the identified independent factors were selected to construct the final nomogram in the training dataset.
Histologic Evaluation and EGFR Mutation Analysis
The included lung adenocarcinomas were categorized according to the 2011 IASLC/ATS/ERS classification system (20) (drug target-associated). Molecular analysis of mutation status of EGFR exons 18–21 was examined using a PCR-based amplification-refractory mutation system (ARMS) by AmoyDx company.
Statistical Analysis
Statistical analysis was performed using R software (version 3.4.2; http://www.Rproject.org). The mean values and standard deviations were expressed for continuous variables (age, lesions size), and frequency or percentage for categorical variables. The Wilcoxon rank sum test and the x2-test were used to compare medians and proportions between two groups, respectively. Predictive performance was evaluated by the area under the curve (AUC) of the receiver operator characteristic (ROC). DeLong test was used to evaluate the difference of the ROCs between various models (21). Hosmer-Lemeshow test was used to evaluate the goodness-of-fit of the constructed nomogram. ICC analysis was performed using “irr” package, features with an ICC> 075 were consider as robust features. Lasso logistic regression was done using the “glmnet” package. Multivariate logistic regression, nomograms and calibration plots were done with the “rms” package. A P<0.05 indicated a significant difference.
Results
Associations Between Clinical, CT Features and EGFR Mutation Status and Subtypes
Associations between clinical, CT features and EGFR mutation status and subtypes were presented in Tables 2, 3. The incidence of harboring EGFR mutation was significantly higher in female than male in two datasets (P = 0.002, P = 0.013, respectively). Patients with EGFR Mut had a higher age in the current study. Smoking was not a significant factor to differentiate EGFR Mut lesions from EGFR WT lesions. In terms of radiographic features, 9 features, including size, margin, shape, pleural retraction, bronchiole change, lobulation, speculation, peripheral emphysema, peripheral fibrosis were significantly associated with EGFR mutation status. Patients with EGFR mutations in 21 exon had a higher age than those with EGFR mutations in 19 exon in two datasets (P = 0.013, P = 0.003, respectively). No other clinical and CT features were identified as potential factors to predict the EGFR mutation subtypes.
Construction of R-Score and the Association Between R-Score (R-Score*) and EGFR Mutation Status and Subtypes
After performing ICC analysis, 425 of 475 radiomics were identified as robust features. The LASSO logistic regression model was performed to select the most distinguishable features in training dataset, resulting in 11 features left (Figure 2). Subsequently, the 11 potential predictors were consequently conducted into a R-score by using the following formula: R-score = −1.072477 + 0.007008 * mean_10_0 + 0.038891 * Homogeneity 0_90_0 + 1.86E-05 * Contrast 45_45_0 + 8.54E-05 * Contrast 90_135_0 + 6.29E-05 * Contrast 90_135_1 – 0.039584 * skewness_1.5 – 0.254939 * skewness_2 + 1.15E-06 * RLN_90_2.5 + 7.46E-05 * Contrast 90_90_2.5 – 9.69E-05 * Contrast 0_0_2.5 – 6.342383311 * Homogeneity 0_0_2.5. The formula caption was presented in Supplementary Data (Referring to the formula for calculating R-score*).
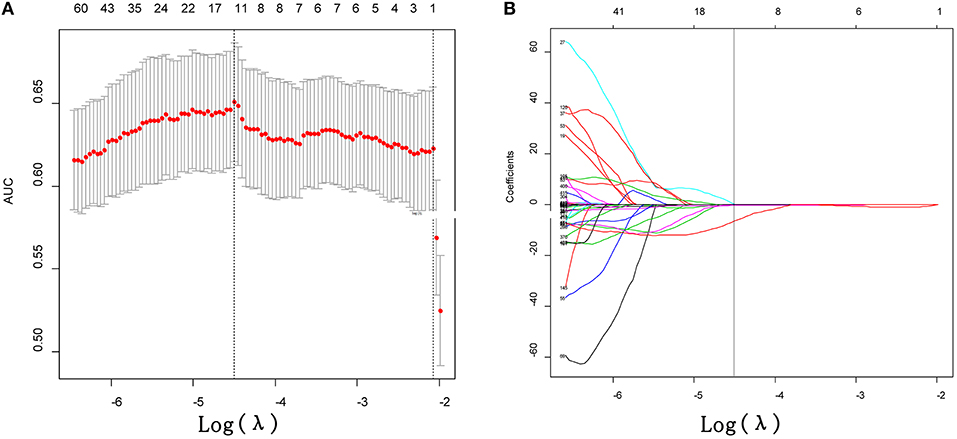
Figure 2. Feature selection using the least absolute shrinkage and selection operator (LASSO) binary logistic regression model. (A) Tuning parameter (λ) selection in the LASSO model used 10-fold cross-validation via minimum criteria. The area under the receiver operating characteristic (AUC) curve was plotted vs. log (λ). Dotted vertical lines were drawn at the optimal values by using the minimum criteria. (B) LASSO coefficient profiles of the 425 texture features. A coefficient profile plot was produced against the log (λ) sequence. Vertical line was drawn at the value selected using 10-fold cross-validation, where optimal λ (−4.497) resulted in 11 non-zero coefficients.
The R-score was calculated for each lesion in two datasets of task a. EGFR-Mut lung adenocarcinomas had a lower R-score than EGFR-WT ones in training dataset (−0.40 ± 0.50 vs. 0.05 ± 0.68, P = 0.000), which was confirmed in validation dataset (−0.37 ± 0.51 vs. 0.01 ± 0.0.58, P = 0.000) (Table 2). The proposed R-score showed a good performance in differentiating EGFR mutation status with AUCs of 0.708, 0.694 in training and validation datasets (Figures 3A,B). The Hosmer-Lemeshow test for R-score yielded a non-significant statistic in the training and validation datasets (P = 0.644, P = 0.657, respectively), indicating that there was no departure from a perfect fit.
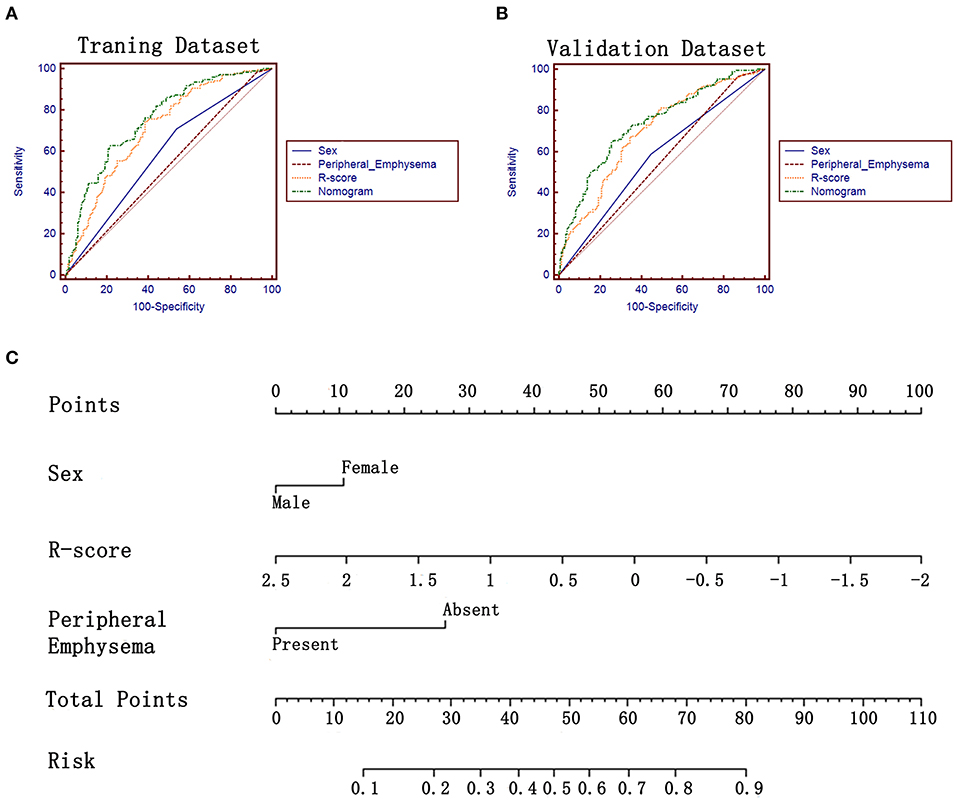
Figure 3. ROC analysis and the constructed nomogram in task a. (A,B) ROC analysis of sex, R-score, peripheral emphysema and the constructed nomogram in training and validation datasets, respectively. (C) The constructed nomogram for predicting EGFR mutation status.
In terms of the task of predicting the EGFR mutation subtypes (task b), 32 features were finally left after performing the LASSO analysis (Supplementary Data). A R-score* was also calculated for each lesion by using the formula presented in the Supplementary Data. Lung adenocarcinomas with EGFR Mut in exon 19 had a lower R-score* than ones with EGFR Mut in exon 21 in training dataset (−0.39 ± 1.35 vs. 0.27 ± 1.12, P = 0.000) and in validation dataset (−0.70 ± 1.25 vs. 0.23 ± 1.12, P = 0.000) (Table 3). The proposed R-score* demonstrated a good performance in differentiating EGFR mutation subtypes with AUCs of 0.684, 0.708 in two datasets (Figures 4A,B). The Hosmer-Lemeshow test for R-score* yielded a non-significant statistic in the training and validation set (P = 0.295, P = 0.242, respectively), which suggested that there was no departure from a perfect fit.
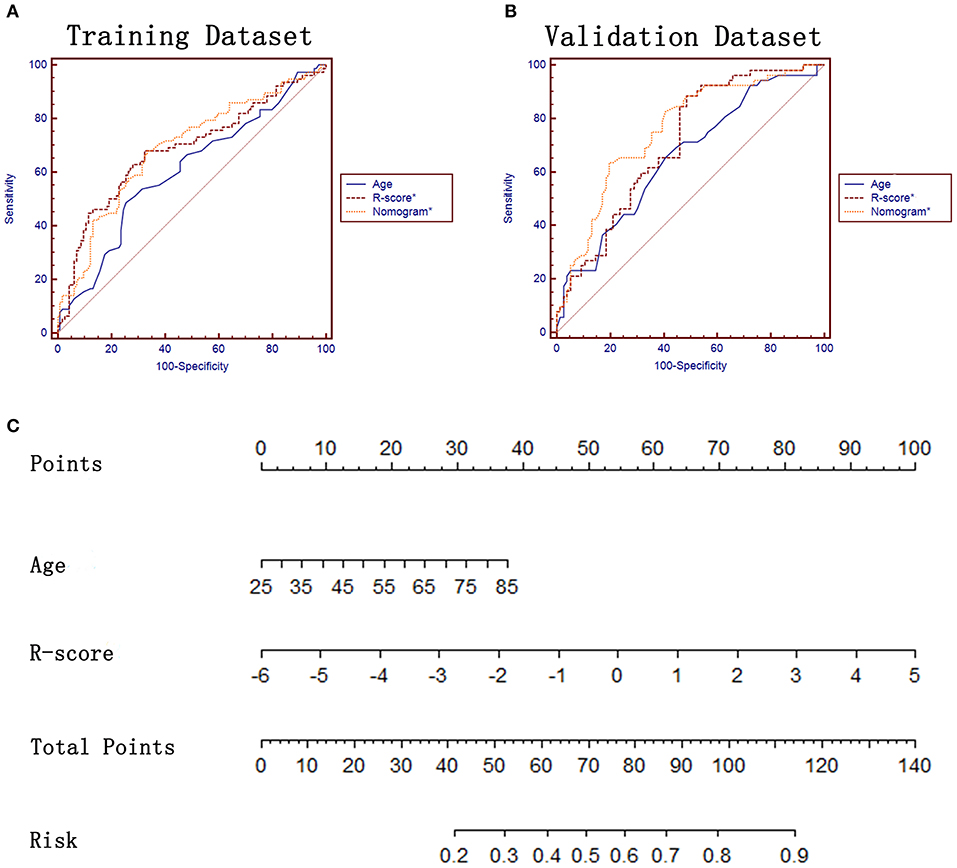
Figure 4. ROC analysis and the constructed nomogram in task b. (A,B) ROC analysis of age, R-score* and the constructed nomogram* in training and validation datasets, respectively. (C) The constructed nomogram* for predicting EGFR mutation subtypes.
Development and Validation of the Radiomics Nomograms for Predicting the EGFR Mutation Status and Subtypes
There was no multicollinearity between the significant factors identified by univariate analysis and R-score. After performing the multivariate analysis, sex, peripheral emphysema and R-score were identified as independent prognostic factors of harboring EGFR mutation (Table 4) and subsequently incorporated to develop the radiomics-based nomogram (Figure 3C). The constructed nomogram obtained a significantly incremental performance for predicting EGFR mutation status compared with that of sex and peripheral emphysema (Table 5). The Hosmer-Lemeshow test for the nomogram yielded a non-significant statistic in the training and validation set (P = 0.313, P = 0.816, respectively), which suggested that there was no departure from a perfect fit. Though the nomogram significantly outperformed R-score in training dataset, the statistically difference was not found in validation dataset (Table 5).
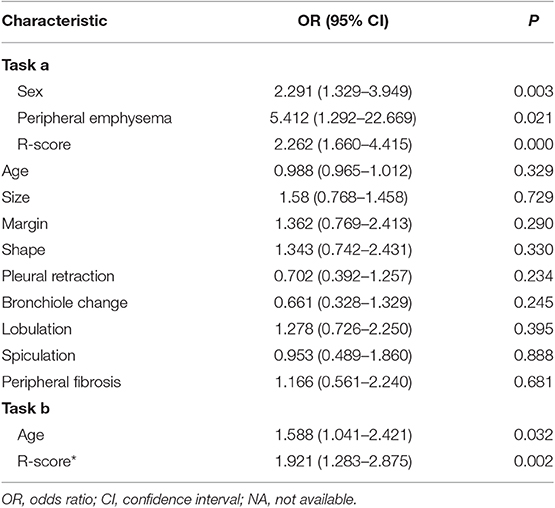
Table 4. Multivariate logistic regression of risk characteristics for predicting the EGFR mutation status and subtypes in training datasets.
In terms of task b, age and R-score* were identified as independent prognostic factors of predicting EGFR mutation subtypes (Table 4) and subsequently incorporated to develop the radiomics-based nomogram* (Figure 4C). The Hosmer-Lemeshow test for the nomogram* yielded a non-significant statistic in the training and validation set (P = 0.760, P = 0.413, respectively), indicating that there was no departure from a perfect fit. Not surprisingly, the constructed nomogram* significantly outperformed age and potentially outperformed R-score* in predicting EGFR mutation subtypes (Table 5).
Discussion
Non-invasively and preoperatively predicting the EGFR mutation status, a field attracted continuous efforts of researchers, can overcome the disadvantages of molecular assays (e.g., high cost, intratumoral heterogeneity, poor sample quality) well and furtherly help clinicians to select the eligible patients for targeted therapy. Moreover, attempting to predict the EGFR mutation subtypes, especially those are sensitivity to small TKIs, may provide important information for making finer treatment scenario. In the current study, we developed and validated two radiomics based nomograms, incorporating clinical characteristics, CT features and radiomics, to predict the EGFR mutation status and subtypes with promising performance (AUC = 0.734, AUC = 0.757, respectively).
It is well-documented that the EGFR Mut rate are significantly higher in female than male (22), which is also confirmed in our study (P = 0.002 and P = 0.013 in two datasets, respectively). Note that smoking status, another potential clinical factor verified by previous studies (8, 14, 17), failed to show significant association with EGFR mutation status in the current study. Note that smoking status is still a contentious risk factor (23) and not recommended to use as the criteria for selecting eligible patients (2). Another reason why the difference between EGFR Mut and WT in terms of smoking status is diminished may be that the incidence of lung adenocarcinomas in female was higher than male (20, 24). Moreover, female may be less likely to be current/former smoker than male. Wu et al. (25) had reported that younger patients (<50 years old) with lung adenocarcinoma had lower EGFR mutation rate, which was not verified in multivariate logistic analysis in the current study. The discrepancy may be contributed to the data bias (ours vs. Wu's: 20.9 vs. 15.5%).
Many prior studies have investigated the role of morphological features in predicting EGFR mutation status. The results remain controversial. On one hand, most radiographic features are non-quantitative subjective features and susceptible to the discrepancy of evaluation caused by different knowledge structure of observers. On the other hand, obvious radiographical features are more frequently presented in advanced tumors instead of early-stage tumors. For example, stage I lung cancers account for 60–70% of detected lung cancers in screening programs (26). In our study, 60.9% of included patients were stage I lung cancers. In this context, the differentiating performance of these semantic features may be compromised. Peripheral emphysema was the only independent risk factors for predicting EGFR mutation status in the current study.
Another disadvantage of semantic features is that they only reflect few tumor information in biological level. By contrast, radiomics method can encode a more comprehensive level of feature abstraction and thus potentially provide better prediction performance. Previous studies have revealed the potential associations between these engineered features and EGFR mutation status (12, 23, 27) and proved that the performance of models can benefit from the integration of radiomics and clinical features (14, 23). These results were also confirmed in our study. Despite the promising results, the complicated process of feature extraction and the inconvenience of formula-based model limit its application in clinical context. Hence, an easy-to-use way for radiomics method is urgently needed. Incorporating multiple radiomic features into a radiomics score can tactfully make multi-marker analyses less complicated to use (18, 28), which is similar to the construction of multi-factor panels. In this study, the LASSO regression model was used to select and compress the radiomic features and thus construct the R-score. The constructed R-score outperformed the clinical and CT features in predicting EGFR mutation status (Table 5). Another strategy to make radiomics method friendly use is presenting the results in a more intuitive way, such as the nomogram (29, 30). Nomogram is a statistic model that can provide an individual numerical probability of a clinical event by integrating multiple variables (31, 32). To comprehensively investigate the predictive performance of non-invasively available variables, including clinical factors, imaging semantic features, imaging radiomic features, we adopted the two above-mentioned strategies to construct a radiomics-based nomogram for predicting the EGFR mutation status. The constructed nomogram in the current study was conveniently used to individualized predict the probability of harboring EGFR mutation by calculation the respective points of three variables (sex, peripheral emphysema, and R-score), with a promising AUC of 0.734. Another promising technique (i.e., liquid biopsy) was consider as an alternative to test EGFR mutations. However, the high false-negative rate (30%) need to be further resolved (33).
In patients with NSCLC, the most commonly found EGFR mutations are deletions in exon 19 (Exon19del in 45%) and in exon 21 (L858R in 40%) (2). Both mutations result in activation of the tyrosine kinase domain, and both are associated with sensitivity to the small molecule TKIs. Nevertheless, patients with exon 19 deletion are associated with longer PFS compared to those with L858R mutation after first-line EGFR-TKIs (34, 35). In addition, the incidence of T790M mutation, which is associated with acquired resistance to reversible EGFR-TKIs (36), might be different between exon 19 deletions and L858R mutations (6), resulting in different treatment scenarios. As a result, furtherly predicting the specific EGFR mutation subtypes may be clinically important. Inspired by the satisfied results of predicting EGFR mutation status, the potential relations between clinical factors, imaging semantic features, imaging radiomic features, and EGFR mutation subtypes were also investigated in our study. Age was the only independent factors that can be used to differentiate different EGFR mutation subtypes. No CT features were identified as potential predictors, which is consistent with previous studies (37, 38), indicating the difficult of predicting EGFR mutation subtypes through semantic features (relatively low-level). This conclusion encouraged us to investigate whether the radiomic features (relatively high-level) might be competent on this task. Surprisingly, the constructed R-score* was identified as an independent predictor and obtained a good performance with an AUC of 0.708. A radiomics-based nomogram*, incorporating age and R-score*, achieved a better performance (AUC = 0.757), indicating the efficiency of radiomic features in different medical tasks.
We may conclude that radiomics outperformed the clinical and semantic features in both tasks (see Table 5). The diagnostic benefits may due to the possession of more presentative and discriminative information of radiomics, which could reflect the tumor spatial heterogeneity, tumor microenvironment, as well as tumor gene patterns. Yet, completely interpreting the association between radiomics and the complex biological processes (EGFR mutation status in the current study) remains an intractable challenge. The interpretability warrants further investigation. Please note that one of the implications of our study was to introduce the nomogram, which was easily used in clinical practice and may facilitate the clinical transformation of radiomics researches.
There are several limitations should be noted. First, although the imaging normalization and reproductive analysis can mitigate the influence of radiomic feature variabilities, it cannot make the current study completely immune to the potential confounding variability caused by different CT scanning parameters (39, 40). Paradoxically, studies with homogeneous images must sacrifice the amount of data. How to balance it well is difficult for each radiomics-based research. Second, this was a single-center study and lacked of external validation, indicating the potential data selection bias. Conducting a multi-center study and validating the constructed model in an independent external dataset may not only improve the generalization and robustness of a model efficiently, the models can also substantially benefit from diversified data from different regions, races and countries. Third, the current study narrowly focused on EGFR mutation status and subtypes. Constructing a nomogram which could cover all clinical relevant EGFR mutation and even other genetic mutations (e.g., ROS-1, ALK) may be interesting and worth investigating in the future work.
Conclusion
Radiomics-based nomogram, incorporating clinical characteristics, CT features and radiomic features, can non-invasively and efficiently predict the EGFR mutation status and thus potentially fulfill the ultimate purpose of precision medicine. The methodology is a possible strategy to predict EGFR mutation subtypes, providing the support of clinical treatment scenario.
Data Availability Statement
The datasets generated for this study are available on request to the corresponding author.
Ethics Statement
This retrospective study was approved by the Institutional Review Board of our institution (No. 20170103), which waived the requirement for patients' informed consent referring to the CIOMS guideline.
Author Contributions
ML, JL, and WZ: conception and design and provision of study materials or patients. ML, JL, YW, and YH: administrative support. ML, WZ, YS, PG, MT, WM, CL, and LJ: collection and assembly of data. ML, JL, WZ, and YX: data analysis and interpretation. All authors: manuscript writing and final approval of manuscript.
Funding
This study was supported by the National Key Research (2017YFC0112905), the Medical Imaging Key Program of Wise Information Technology of 120, Health Commission of Shanghai (Grant No. 2018ZHYL0103), the National Natural Science Foundation of China (Grant Nos. 61976238 and 81671671), the Future Star of famous doctors' training plan of Fudan University, the Shanghai Municipal Commission of Science and Technology (Grant No. 17411963600) and the Key R & D projects in Hunan Province (Grant No. 2019SK2131).
Conflict of Interest
The authors declare that the research was conducted in the absence of any commercial or financial relationships that could be construed as a potential conflict of interest.
Acknowledgments
The authors would like to thank Jianhong Cheng for assisting in data analysis.
Supplementary Material
The Supplementary Material for this article can be found online at: https://www.frontiersin.org/articles/10.3389/fonc.2019.01485/full#supplementary-material
Abbreviations
EGFR, epidermal growth factor receptor; NSCLC, non-small cell lung carcinoma; PFS, progression-free survival; ALK, antigen anaplastic lymphoma kinase; ROS1, c-ros oncogene 1; TKIs, tyrosine kinase inhibitors.
References
1. Bray F, Ferlay J, Soerjomataram I, Siegel RL, Torre LA, Jemal A. Global cancer statistics 2018: GLOBOCAN estimates of incidence and mortality worldwide for 36 cancers in 185 countries. CA Cancer J Clin. (2018) 68:394–424. doi: 10.3322/caac.21492
2. Ettinger DS, Wood DE, Aisner DL, Akerley W, Bauman J, Chirieac LR, et al. Non-small cell lung cancer, Version 5.2017, NCCN clinical practice guidelines in oncology. J Natl Compr Canc Netw. (2017) 15:504–35. doi: 10.6004/jnccn.2017.0050
3. Lee SM, Lewanski CR, Counsell N, Ottensmeier C, Bates A, Patel N, et al. Randomized trial of erlotinib plus whole-brain radiotherapy for NSCLC patients with multiple brain metastases. J Natl Cancer Inst. (2014) 106:151. doi: 10.1093/jnci/dju151
4. Novello S. Epidermal growth factor receptor tyrosine kinase inhibitors as adjuvant therapy in completely resected non-small-cell lung cancer. J Clin Oncol. (2015) 33:3985–6. doi: 10.1200/JCO.2015.63.7587
5. Hirsch FR, Bunn PJ. EGFR testing in lung cancer is ready for prime time. Lancet Oncol. (2009) 10:432–3. doi: 10.1016/S1470-2045(09)70110-X
6. Ke EE, Zhou Q, Zhang QY, Su J, Chen ZH, Zhang XC, et al. A higher proportion of the EGFR T790M mutation may contribute to the better survival of patients with exon 19 deletions compared with those with L858R. J Thorac Oncol. (2017) 12:1368–75. doi: 10.1016/j.jtho.2017.05.018
7. Sacconi B, Anzidei M, Leonardi A, Boni F, Saba L, Scipione R, et al. Analysis of CT features and quantitative texture analysis in patients with lung adenocarcinoma: a correlation with EGFR mutations and survival rates. Clin Radiol. (2017) 72:443–50. doi: 10.1016/j.crad.2017.01.015
8. Hasegawa M, Sakai F, Ishikawa R, Kimura F, Ishida H, Kobayashi K. CT features of epidermal growth factor receptor-mutated adenocarcinoma of the lung: comparison with nonmutated adenocarcinoma. J Thorac Oncol. (2016) 11:819–26. doi: 10.1016/j.jtho.2016.02.010
9. Sabri A, Batool M, Xu Z, Bethune D, Abdolell M, Manos D. Predicting EGFR mutation status in lung cancer: proposal for a scoring model using imaging and demographic characteristics. Eur Radiol. (2016) 26:4141–7. doi: 10.1007/s00330-016-4252-3
10. Cheng Z, Shan F, Yang Y, Shi Y, Zhang Z. CT characteristics of non-small cell lung cancer with epidermal growth factor receptor mutation: a systematic review and meta-analysis. BMC Med Imag. (2017) 17:5. doi: 10.1186/s12880-016-0175-3
11. Aerts HJ, Grossmann P, Tan Y, Oxnard GG, Rizvi N, Schwartz LH, et al. Defining a radiomic response phenotype: a pilot study using targeted therapy in NSCLC. Sci Rep. (2016) 6:33860. doi: 10.1038/srep33860
12. Rios VE, Parmar C, Liu Y, Coroller TP, Cruz G, Stringfeld O, et al. Somatic mutations drive distinct imaging phenotypes in lung cancer. Cancer Res. (2017) 77:3922–30. doi: 10.1158/0008-5472.CAN-17-0122
13. Yip SS, Kim J, Coroller TP, Parmar C, Velazquez ER, Huynh E, et al. Associations between somatic mutations and metabolic imaging phenotypes in non-small cell lung cancer. J Nucl Med. (2017) 58:569–76. doi: 10.2967/jnumed.116.181826
14. Liu Y, Kim J, Balagurunathan Y, Li Q, Garcia AL, Stringfield O, et al. Radiomic features are associated with egfr mutation status in lung adenocarcinomas. Clin Lung Cancer. (2016) 17:441–8.e6. doi: 10.1016/j.cllc.2016.02.001
15. Digumarthy SR, Padole AM, Gullo RL, Sequist LV, Kalra MK. Can CT radiomic analysis in NSCLC predict histology and EGFR mutation status? Medicine. (2019) 98:e13963. doi: 10.1097/MD.0000000000013963
16. Forghani R, Savadjiev P, Chatterjee A, Muthukrishnan N, Reinhold C, Forghani B. Radiomics and artificial intelligence for biomarker and prediction model development in oncology. Comput Struct Biotechnol J. (2019) 17:995–1008. doi: 10.1016/j.csbj.2019.07.001
17. Liu Y, Kim J, Qu F, Liu S, Wang H, Balagurunathan Y, et al. CT features associated with epidermal growth factor receptor mutation status in patients with lung adenocarcinoma. Radiology. (2016) 280:271–80. doi: 10.1148/radiol.2016151455
18. Zhao W, Xu Y, Yang Z, Sun Y, Li C, Jin L, et al. Development and validation of a radiomics nomogram for identifying invasiveness of pulmonary adenocarcinomas appearing as subcentimeter ground-glass opacity nodules. Eur J Radiol. (2019) 112:161–8. doi: 10.1016/j.ejrad.2019.01.021
19. Sauerbrei W, Royston P, Binder H. Selection of important variables and determination of functional form for continuous predictors in multivariable model building. Stat Med. (2007) 26:5512–28. doi: 10.1002/sim.3148
20. Travis WD, Brambilla E, Noguchi M, Nicholson AG, Geisinger KR, Yatabe Y, et al. International association for the study of lung cancer/american thoracic society/european respiratory society international multidisciplinary classification of lung adenocarcinoma. J Thorac Oncol. (2011) 6:244–85. doi: 10.1097/JTO.0b013e318206a221
21. DeLong ER, DeLong DM, Clarke-Pearson DL. Comparing the areas under two or more correlated receiver operating characteristic curves: a nonparametric approach. Biometrics. (1988) 44:837–45. doi: 10.2307/2531595
22. Singal G, Miller PG, Agarwala V, Li G, Kaushik G, Backenroth D, et al. Association of patient characteristics and tumor genomics with clinical outcomes among patients with non-small cell lung cancer using a clinicogenomic database. JAMA. (2019) 321:1391–99. doi: 10.1001/jama.2019.3241
23. Jia TY, Xiong JF, Li XY, Yu W, Xu ZY, Cai XW, et al. Identifying EGFR mutations in lung adenocarcinoma by noninvasive imaging using radiomics features and random forest modeling. Eur Radiol. (2019) 29:4742–50. doi: 10.1007/s00330-019-06024-y
24. Jemal A, Miller KD, Ma J, Siegel RL, Fedewa SA, Islami F, et al. Higher lung cancer incidence in young women than young men in the United States. N Engl J Med. (2018) 378:1999–2009. doi: 10.1056/NEJMoa1715907
25. Wu SG, Chang YL, Yu CJ, Yang PC, Shih JY. Lung adenocarcinoma patients of young age have lower EGFR mutation rate and poorer efficacy of EGFR tyrosine kinase inhibitors. ERJ Open Res. (2017) 3:00092–2016. doi: 10.1183/23120541.00092-2016
26. Rami-Porta R, Bolejack V, Crowley J, Ball D, Kim J, Lyons G, et al. The IASLC lung cancer staging project: proposals for the revisions of the T descriptors in the forthcoming eighth edition of the TNM classification for lung cancer. J Thorac Oncol. (2015) 10:990–1003. doi: 10.1097/JTO.0000000000000559
27. Yang X, Dong X, Wang J, Li W, Gu Z, Gao D, et al. Computed tomography-based radiomics signature: a potential indicator of epidermal growth factor receptor mutation in pulmonary adenocarcinoma appearing as a subsolid nodule. Oncologist. (2019) 24:e1156–64. doi: 10.1634/theoncologist.2018-0706
28. Huang YQ, Liang CH, He L, Tian J, Liang CS, Chen X, et al. Development and validation of a radiomics nomogram for preoperative prediction of lymph node metastasis in colorectal cancer. J Clin Oncol. (2016) 34:2157–64. doi: 10.1200/JCO.2015.65.9128
29. Tan Y, Zhang ST, Wei JW, Dong D, Wang XC, Yang GQ, et al. A radiomics nomogram may improve the prediction of IDH genotype for astrocytoma before surgery. Eur Radiol. (2019) 29:3325–37. doi: 10.1007/s00330-019-06056-4
30. Ji GW, Zhu FP, Zhang YD, Liu XS, Wu FY, Wang K, et al. A radiomics approach to predict lymph node metastasis and clinical outcome of intrahepatic cholangiocarcinoma. Eur Radiol. (2019) 29:3725–35. doi: 10.1007/s00330-019-06142-7
31. Girard N, Sima CS, Jackman DM, Sequist LV, Chen H, Yang JC, et al. Nomogram to predict the presence of EGFR activating mutation in lung adenocarcinoma. Eur Respir J. (2012) 39:366–72. doi: 10.1183/09031936.00010111
32. Balachandran VP, Gonen M, Smith JJ, DeMatteo RP. Nomograms in oncology: more than meets the eye. Lancet Oncol. (2015) 16:e173–80. doi: 10.1016/S1470-2045(14)71116-7
33. Lindeman NI, Cagle PT, Aisner DL, Arcila ME, Beasley MB, Bernicker EH, et al. Updated molecular testing guideline for the selection of lung cancer patients for treatment with targeted tyrosine kinase inhibitors: guideline from the college of American Pathologists, the International Association for the Study of Lung Cancer, and the Association for Molecular Pathology. J Thorac Oncol. (2018) 13:323–58. doi: 10.1016/j.jtho.2017.12.001
34. Lee CK, Wu YL, Ding PN, Lord SJ, Inoue A, Zhou C, et al. Impact of specific epidermal growth factor receptor (EGFR) mutations and clinical characteristics on outcomes after treatment with EGFR tyrosine kinase inhibitors versus chemotherapy in EGFR-mutant lung cancer: a meta-analysis. J Clin Oncol. (2015) 33:1958–65. doi: 10.1200/JCO.2014.58.1736
35. Sheng M, Wang F, Zhao Y, Li S, Wang X, Shou T, et al. Comparison of clinical outcomes of patients with non-small-cell lung cancer harbouring epidermal growth factor receptor exon 19 or exon 21 mutations after tyrosine kinase inhibitors treatment: a meta-analysis. Eur J Clin Pharmacol. (2016) 72:1–11. doi: 10.1007/s00228-015-1966-0
36. Kobayashi S, Boggon TJ, Dayaram T, Janne PA, Kocher O, Meyerson M, et al. EGFR mutation and resistance of non-small-cell lung cancer to gefitinib. N Engl J Med. (2005) 352:786–92. doi: 10.1056/NEJMoa044238
37. Zhao FN, Zhao YQ, Han LZ, Xie YS, Liu Y, Ye ZX. Clinicoradiological features associated with epidermal growth factor receptor exon 19 and 21 mutation in lung adenocarcinoma. Clin Radiol. (2019) 74:80.e7–80.e17. doi: 10.1016/j.crad.2018.10.001
38. Hong SJ, Kim TJ, Choi YW, Park JS, Chung JH, Lee KW. Radiogenomic correlation in lung adenocarcinoma with epidermal growth factor receptor mutations: Imaging features and histological subtypes. Eur Radiol. (2016) 26:3660–8. doi: 10.1007/s00330-015-4196-z
39. Berenguer R, Pastor-Juan M, Canales-Vazquez J, Castro-Garcia M, Villas MV, Mansilla LF, et al. Radiomics of CT features may be nonreproducible and redundant: influence of CT acquisition parameters. Radiology. (2018) 288:407–15. doi: 10.1148/radiol.2018172361
Keywords: EGFR, radiomics, nomogram, lung adenocarcinomas, CT
Citation: Zhao W, Wu Y, Xu Y, Sun Y, Gao P, Tan M, Ma W, Li C, Jin L, Hua Y, Liu J and Li M (2020) The Potential of Radiomics Nomogram in Non-invasively Prediction of Epidermal Growth Factor Receptor Mutation Status and Subtypes in Lung Adenocarcinoma. Front. Oncol. 9:1485. doi: 10.3389/fonc.2019.01485
Received: 08 October 2019; Accepted: 10 December 2019;
Published: 09 January 2020.
Edited by:
Umberto Malapelle, University of Naples Federico II, ItalyReviewed by:
Francesco Pepe, Department of Public Health, University of Naples Federico II, ItalyMarzia Del Re, University of Pisa, Italy
Copyright © 2020 Zhao, Wu, Xu, Sun, Gao, Tan, Ma, Li, Jin, Hua, Liu and Li. This is an open-access article distributed under the terms of the Creative Commons Attribution License (CC BY). The use, distribution or reproduction in other forums is permitted, provided the original author(s) and the copyright owner(s) are credited and that the original publication in this journal is cited, in accordance with accepted academic practice. No use, distribution or reproduction is permitted which does not comply with these terms.
*Correspondence: Ming Li, bWlubGk3N0AxNjMuY29t; Jun Liu, anVubGl1MTIzQGNzdS5lZHUuY24=