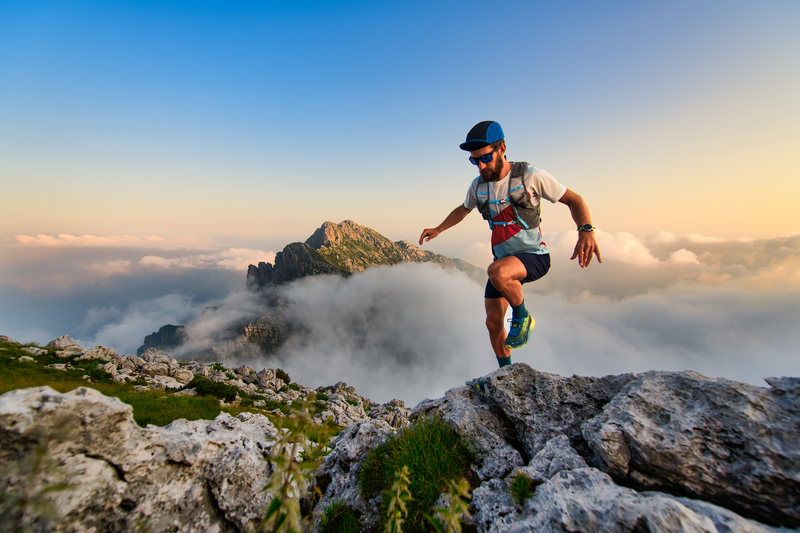
94% of researchers rate our articles as excellent or good
Learn more about the work of our research integrity team to safeguard the quality of each article we publish.
Find out more
PERSPECTIVE article
Front. Oncol. , 12 February 2018
Sec. Cancer Molecular Targets and Therapeutics
Volume 8 - 2018 | https://doi.org/10.3389/fonc.2018.00023
This article is part of the Research Topic Harnessing the Power of Patient Derived Models of Cancer View all 6 articles
Despite substantial effort and resources dedicated to drug discovery and development, new anticancer agents often fail in clinical trials. Among many reasons, the lack of reliable predictive preclinical cancer models is a fundamental one. For decades, immortalized cancer cell cultures have been used to lay the groundwork for cancer biology and the quest for therapeutic responses. However, cell lines do not usually recapitulate cancer heterogeneity or reveal therapeutic resistance cues. With the rapidly evolving exploration of cancer “omics,” the scientific community is increasingly investigating whether the employment of short-term patient-derived tumor cell cultures (two- and three-dimensional) and/or patient-derived xenograft models might provide a more representative delineation of the cancer core and its therapeutic response. Patient-derived cancer models allow the integration of genomic with drug sensitivity data on a personalized basis and currently represent the ultimate approach for preclinical drug development and biomarker discovery. The proper use of these patient-derived cancer models might soon influence clinical outcomes and allow the implementation of tailored personalized therapy. When assessing drug efficacy for the treatment of glioblastoma multiforme (GBM), currently, the most reliable models are generated through direct injection of patient-derived cells or more frequently the isolation of glioblastoma cells endowed with stem-like features and orthotopically injecting these cells into the cerebrum of immunodeficient mice. Herein, we present the key strengths, weaknesses, and potential applications of cell- and animal-based models of GBM, highlighting our experience with the glioblastoma stem-like patient cell-derived xenograft model and its utility in drug discovery.
Despite considerable effort that has been dedicated to drug development in oncology, more than 90% of novel therapeutics fail in human trials (1). Undoubtedly, one of the reasons for this high attrition rate is the lack of reliable preclinical models (2). Fast-paced advances in scientific research, technology discovery, and optimization have revealed that cancer is a much more complex disease than previously thought (3). Indeed, the disease is characterized by intense inter- and intra-tumor heterogeneity at the genetic, epigenetic, metabolic, and phenotypic levels. Models are, by definition, in one way or the other imprecise. They are simplified versions of intricate systems and, as such, prone to approximation and partiality. Concordantly, most of the current preclinical cancer models are often unable to recapitulate tumor complexity associated with heterogeneity or predict patient response to treatment (2).
Cell-based assays represent an important pillar of the drug discovery process. Although widely used for testing the therapeutic efficacy of anticancer drugs (4), the clinical value of immortalized cancer cell lines, with some notable exceptions (e.g. U251 GBM cells) (5), as a truly representative tumor model has been continuously questioned (6). Unmanipulated patient-derived tumor cell cultures surely present a more reliable depiction of the tumor features than established cell lines. The 3D culture systems are preferred over the 2D cultures because they mimic tumor conditions in vivo (7). Animal models are better representatives of the complex tumor repertoire because they endow a multicellular microenvironment in which patient tumor cells reside, although they are more difficult to establish.
The scientific community has been adapting to the use of patient-derived xenograft (PDX) models, obtained by engrafting patient tumor fragments, or tumor-derived cells into immunocompromised mice. Databases of annotated PDX models, such as the EurOPDX Consortium (8) and the NCI patient-derived models repository (9) have become publicly available. PDXs could maintain the principal histological and genetic characteristics of their originating tumor across early passages (10). Thus, PDXs could be used to improve and direct drug developments toward more personalized approaches. This concept has encouraged the approach of “co-clinical trials,” where PDX models, derived from patients enrolled in a clinical trial, could be used as “avatars” to identify the best therapeutic approach to be administered to patients in real time (11). Still, the use of PDXs in personalized medicine and targeted therapy is challenging, mainly because of the long time required to obtain the preclinical data needed for clinical decision making (10). Nevertheless, the clinical impact of PDX models is not restricted to their use in precision medicine. They can be utilized to gain a better understanding of cancer biology, identify predictive biomarkers for therapy outcome, and could also represent a reliable translational platform for the investigation of the correlation between treatments and genotypes (12) and for identifying novel anticancer therapeutics (13–15). In the next section, we present a concise overview of the currently available preclinical models for glioblastoma multiforme (GBM) and describe our findings on the use of GBM PDX models, which will be invaluable for drug discovery and personalized therapy.
It is estimated that over 23,000 patients with primary brain cancers were diagnosed in 2017, and over 16,000 brain cancer-related deaths occur annually in the USA (16). GBM, a grade IV astrocytoma, is the most prevalent and lethal primary brain tumor. GBM is classified into four molecular subtypes, depending on the status of PDGFRA, IDH1, EGFR, and NF1 (17). However, all four molecular subtypes were detected in the same patient’s GBM (18), reflecting intense intra-tumor heterogeneity. Only EGFR amplification, IDH1/IDH2 mutations and methylguanine-DNA-methyltransferase (MGMT) hyper-methylation are utilized clinically. GBM patients are typically treated by surgical resection followed initially by radiation therapy plus temozolomide and then with maintenance therapy comprising temozolomide. Despite this therapeutic regimen, 95% of patients suffer from GBM relapse (19). Recurrent GBM patients are treated with the monoclonal antibody Bevacizumab (Avastin), an anti-angiogenic agent targeting VEGF (20). However, the overall survival remains very low, <10% at 5 years, with a median overall survival of merely 14.8 months (21). Because of these dismal survival rates, there is an urgent need for effective therapeutic strategies to prevent or delay GBM recurrence. Thus, it is imperative to have access to reliable preclinical models that can faithfully predict patients’ responses to specific agents.
For the last five decades, investigation of GBM pathogenesis and resistance mechanisms has been carried out using standard cell lines, such as U87, U251, and T98G, among a handful of others, accounting for over 5,000 citations. Yet, these cell models have several limiting imperfections, restricting their reliability in drug development, such as genome and transcriptome adaptation to rapid growth on plastic, as well as alterations and clonal selection caused by culture conditions over many years (6). When injected into rodent brains, these cell lines, except for U251 (5), generally fail to generate tumors with morphological features typical of GBM, such as infiltration into the surrounding brain parenchyma and necrosis. Additionally, a recent genetic analysis of U87 cells (most used GBM cell line) revealed that the DNA profile of the current cell line differs from that of the original cell line established in 1968 (22). For these reasons, the reliability of these cell models is constantly questioned.
There is now a wide consensus among the scientific community about the hypothesis that a dynamic subpopulation of cells within each tumor is responsible for progression and recurrence (23). These cells, endowed with stem-like features, are called cancer stem cells (CSCs) or GBM-initiating cells (GICs). GICs possess unique dynamic features, such as tumor-initiating ability (24), sustained self-renewal and/or intensified clonogenic ability. Abundant evidence corroborates the notion that high grade tumors, such as GBM, are driven by such cells (25, 26), that are therapy-resistant (27, 28) and cause GBM relapse (29). GICs can be isolated from primary tumors based on the expression of specific surface markers, such as CD15 (also called SSEA-1) (30), CD24 (31), and CD133 (32). However, ongoing debates are still challenging the specificity of CD133 as a single marker (33, 34). GICs can also be isolated from tumors using functional assays and grown as neurospheres, using conditions similar to those employed for normal neural stem cell culture (35). The sphere assay (36) is one of such functional assays used to establish GICs expressing stemness factors such as CD133, BMI1, NESTIN, SOX2, SOX9, OLIG2, and ZEB1 (37, 38). When basic fibroblast growth factor and epidermal growth factor (EGF) in sphere culture are replaced with differentiation media, cells undergo differentiation, with loss of stemness factors, and acquisition of mature cell markers (39, 40).
We successfully generated GIC neurospheres from surgical specimens of consented glioma patients (40) (Figure 1A) through Rutgers Cancer Institute of New Jersey Biospecimen Repository Service using protocols approved by the Institutional Review Board of Rutgers University. By exposing patient-derived dissociated cells to serum-free media supplemented with EGF, FGF, and B27, we generated GIC neurosphere lines from GBM patient samples. Consistent with other studies, these neurospheres are characterized by heterogeneous clonogenic ability (Figure 1B), an aggressive tumor-formation ability and resistance to both radiation and targeted therapy (41). As expected, they possess stem-like properties and can differentiate upon exposure to differentiating media (Figure 1C). This long-term culture method allows the selection of the stem-like population using their functional characteristics (ability to grow in suspension) and responsiveness to niche- (or media)-derived clues (by maintaining stem-like and/or multilineage differentiation abilities) (Figure 1C), rather than relying on sorting cells for the expression of surface markers, whose expression would dynamically change based on the environment and association with stemness features (42). Moreover, in contrast to most standard cell lines, GIC neurospheres could generate tumors when labeled and injected orthotopically into the mouse brain cerebrum (Figures 1D,E), using a protocol approved by the Institutional Animal Care and Use Committee of Rutgers University. These tumor grafts retain the morphological and molecular features of the GBM tumor from which they originated (43). Additionally, the GIC neurosphere model has a recognized clinical relevance and could be used as an independent predictor of clinical outcome in GBM (36). For these reasons, culture of GICs as GBM neurospheres is considered a more representative and reliable cell model compared to standard cell lines. The quest for these models has been exponential, and to satisfy this need, a biobank of GICs, the human glioblastoma cell culture resource, was recently created and made public (44).
Figure 1. Analysis of neurospheres derived from primary glioblastoma surgical tissues. (A) Model of patient-derived sphere assay. Neurospheres are kept in serum-free growth factor condition. To examine their differentiation potential, they are cultured in polyornithine-coated plates. (B) Neurosphere clonogenic potential of four different patient-derived glioblastoma multiforme (GBM) cells. (C) Immunofluorescence for NESTIN, which is used as a marker of stem cells, being expressed in central nervous system (CNS) stem cells but not in mature CNS cells, and TUBULIN and glial fibrillary acidic protein (GFAP; differentiation markers). TUBULIN-beta-III is a neuron-specific early marker of signal commitment in primitive neurepithelium. GFAP is used as a marker of CNS mature astrocytes and ependymal cells. DAPI is used to label the nuclei, while phalloidin is used to mark the cell architecture within the spheres. (D) GBM spheres transduced with lentiviruses expressing either luciferase (Luc) and EGFP or DsRed. (E) The two types of transduced spheres were used to generate patient-derived orthotopic xenografts (PDOXs) when injected into NSG mouse brains with 2 × 105 labeled cells and monitored with the IVIS system. Luc was used to longitudinally track tumor growth in vivo. Images were from PDOX mice imaged at 8-weeks postinjection. Please note that the image showing Luc and DsRed is zoomed in to demonstrate the brain regions. Color scale was generated with the IVIS software and represents pixel intensities in luminescent or fluorescent images. Color scale units are photons/cm2/second. Scale bars are 200 µM in (D).
Although cell-based models are of paramount importance for the investigation of GBM pathogenesis, at present, they have limited utility in the context of drug discovery. We cannot fully assess the efficacy of therapeutic compounds without testing them in physiologically relevant systems, such as laboratory animals. In vivo models are required especially for diseases affecting the central nervous system, including GBM. In fact, many promising drug candidates for GBM fail during the clinical phases of development due to the blood–brain barrier, limiting or preventing drug permeation to the brain (45). Mice, and to a lesser extent rats, have been the selected species for biomedical research and drug development for gliomas.
There are three main in vivo models for primary brain tumors: (1) chemically induced, (2) genetically engineered (GEM), and (3) xenograft (46). Chemically induced models are now considered outdated, since they generate tumors that differ from human gliomas, both at the morphological and molecular levels. On the other hand, GEM and xenograft models have been highly valuable to study the mechanistic determinants of GBM formation and progression and investigate potential therapeutics in the brain tumor field. GEM models are generated based on specific genetic alterations observed in human tumors. In parallel to the increasing understanding of the genetic, epigenetic, and metabolic perturbations involved in GBM and the intensifying advances in genome editing technologies, GEM models have been growing increasingly more complex. Mouse models characterized by conditional and tissue/cell-specific expression of multiple genes simultaneously or developed by altering key signaling pathways involved in GBM (such as Egfr, Nf1, Ras, Pten) are recently used, improving the genetic and phenotypic heterogeneity of mouse gliomas, that are typical of human GBM. In vivo, GEM models were proven beneficial to study GICs (47–50), particularly in the clinically relevant immune-competent setting (51).
Lineage-tracing studies provided genetic evidence for CSC-driven clonal growth in several cancers including GBM (52–54). In hGFAP-Cre;Nf1±;p53fl/fl;Ptenfl/+ glioma GEM model (100% penetrance in 20–40 weeks), temozolomide could diminish the proliferating GBM cells, while repopulation of GBM was driven by residual quiescent GICs, recruited (to expand and/or divide) after temozolomide was discontinued (53). We reported that Bmi1+ cells are highly enriched for GICs (40). Because of their nature, GEM models are an excellent tool to evaluate the role of single genes (or combination of few genes) in GBM onset and progression and could function as ideal models to assess the selectivity of a drug. We are currently using GEM models (47, 49, 53) in lineage tracing studies, by labeling Bmi1+ cells (GICs), to study Bmi1 contribution to GICs, cell cycling parameters and small molecule-mediated Bmi1 ablation. GBM resistance to alkylating agents could be driven by deregulation of mismatch and base excision DNA repair pathways (29), which could result from elevated poly-ADP-ribose polymerase (PARP) activation within GICs (55), without altering the proliferative hierarchy of GBM (56), providing a therapeutic opportunity. Resistance to therapy has also been shown to be driven by factors within the microenvironment (57). Moreover, single cell GBM RNA sequencing revealed that GBM phenotypes are influenced by immune cell contributions (18).
The xenograft model is generated by engrafting human cells into immunocompromised animals. The origin of human cells implanted and site of implantation are important considerations, because these conditions determine the formation of different tumors in terms of phenotypical and genetic similarity to human GBM. Since the orthotopic injection site provides the murine stromal support and an environment most similar to the site of the human tumor, it is generally preferred over the heterotopic sites (58). Preclinical studies in the past few decades have been dominated by models created by injecting cell lines subcutaneously into mice. These cells generate xenografts that are mostly dissimilar from the original tumor, both phenotypically and genetically. For instance, genetic analyses of commonly used GBM cell lines revealed how these cells possess DNA profiles divergent from those of human GBM (59). Not surprisingly, standard cell line-derived xenografts, although highly proliferative, lack key features of human GBM such as necrosis and infiltrative nature (5). Thus, due to the clonal selection and genetic drift consequences of adaptation to monolayer culture, cell line-derived xenografts do not satisfy the criteria for a reliable preclinical model. A more representative model of human GBM is the patient-derived orthotopic xenograft models (PDOX), in which dissociated tumor cells or tumor tissue fragments are implanted into mouse brains. As shown recently (60, 61), it is assumed that since these cells or fragments are not cultured in vitro, the flaws associated with culture conditions do not technically apply. Another strategy was used to maintain GBM PDXs by serial subcutaneous passaging in nude mice then PDX cells were used generate a panel of GBM PDOXs that, like clinical samples, maintained MGMT promoter methylation (62) and could be used to evaluate temozolomide sensitizing combination therapy (63). Thus, GBM PDOXs largely retain the morphological characteristics of the tumor from which they are derived, such as infiltrative growth patterns, microvascular abnormalities, and necrosis. Moreover, PDOXs also maintain the histological, genetic, and epigenetic features of the parental tumor (60). On the other hand, it has been most recently revealed that selection pressures could lead to distinct tumor evolution between primary human tumors and their corresponding clones propagated in mouse PDXs, including those of GBM (64). Hence, PDOXs, within early passages and/or with an established genomic parity with their corresponding primary GBM, represent a more superior model compared to GEMs in the sense that PDOXs more faithfully resemble human GBM.
Despite the advantages of GBM PDOXs, they are far from perfect. First, the tumor-establishment rate of this model is highly variable, ranging from a reported 80–90% success rate (60) to a complete failure in generating GBM PDOXs (65), especially when small tumor fragments are the source material (65). Second, the initial engraftment has a long latency (2–11 months) (66), and could potentially fail to progress, thus limiting their use for the “co-clinical trials” approach. Third, there is high variability between PDOXs in terms of clonal composition of the tumors. This could represent an advantage, in the sense that different PDOXs reflect the inter-tumoral heterogeneity observed in GBM patients. However, such heterogeneity does not favor experimental standardization, thus challenging the use of PDOX models in drug development.
Other alternatives to the “classic” PDOX models have been proposed, realized by adding an in vitro step. One such model is represented by injection of spheres (66), the second is by injection of organoids (67), usually derived from resections of GBMs from patients, and both spheres and/or organoids could also be generated upon initial propagation of PDOXs in mice. The biopsy tissue is minced and exposed to matrix-coated culture vessels in serum-free media. Injection of GBM spheres yields a tumor take rate close to 100%, thus significantly improving on the take rate of PDOX models (66). Although these models require validation of genomic, epigenomic, metabolic and expression parity with the original GBM (because tumor specimens are exposed to culture), in our opinion, when generated and paired with defined clonal tumors and/or single cells reflecting the original tumor diversity, they provide a way to refine and further exploit these models. Indeed, these alternative approaches offer the advantages of the use of the 3D spheres and organoids in drug screening and selecting personalized therapy, while still supporting the development of reliable PDOXs.
In our experience, the time needed for the initial engraftment of the GIC neurospheres generated in our lab (40) ranged between 2 and 6 weeks. To longitudinally track tumor growth in vivo without sacrificing the engrafted animals, we transduced GIC neurospheres with lentiviruses encoding Luciferase and either EGFP or DsRed before slow-release injection with an infusion pump, thus allowing us to precisely control the site of injection at the mouse brain cerebrum and the growth rate through bioluminescence and/or fluorescence in vivo monitoring (Figures 1D,E and 2). Just two months after injection of as low as 104 GICs, most animals showed neurological signs associated with tumor burden. PDOXs recapitulated key GBM morphological features (Figure 2B), including necrosis and overall expression of the stem cell proteins BMI1, NESTIN, and SOX9 and the proliferation marker Ki67 (Figure 2C). Moreover, PDOXs demonstrated a high degree of hyperplastic blood vessels, a hallmark of GBM (68) representing the original GBP patient tissues (Figure 2D). Additionally, GBM cells expressing the GIC CD15 (30) were present in clusters of GBM niches in proximity of blood vessels, and cells expressing the hypoxia protein carbonic anhydrase IX were in close proximity to cells expressing CD15, within both the original GBM patient tissue and the corresponding PDOXs (Figure 2D). Our studies demonstrate that PDOXs generated from patient-derived GIC neurospheres could make a reliable model for developing targeted and personalized therapies for GBM patients.
Figure 2. Histologic and immunophenotypic parity between original glioblastoma tissue and patient-derived orthotopic xenografts (PDOXs). (A) Strategy to generate spheres and PDOX from primary glioblastoma tissue. The diagram displays the process of microinjecting sphere cells into the cerebrum region of the mouse brain. The location of burr drilling hole (red circle) in NSG mice skull is demonstrated for microinjections using stereotactic infusion pump resulting in effective (90% take) generation of orthotopic glioblastoma multiforme (GBM) PDOXs. (B) Histological H&E analysis of original patient-derived glioblastoma tissue (patient (Pt) #46) and four different PDOX lines generated from the same patient-derived spheres. Note that the cell density is different in these sections as it depends on the number of cells engrafted into the PDOXs. The lower panels are 1,000× higher magnification of the outlined areas in the top panels. (C) Representative sections for comparison of the expression of stem cell proteins (BMI1, NESTIN, and SOX9) and the proliferation marker Ki67 in the original patient GBM tissue and the corresponding PDOX. Red arrows indicate positive cells. (D) Representative sections for comparison of the clustered expression of the GBM stem cell marker (CD15) in vascular niches and with the hypoxia protein carbonic anhydrase IX (CA9) near blood vessels expressing CD31, both in the original patient GBM tissue and the corresponding PDOX. Black and/or red arrows indicate positive cells. Scale bars are 500 µM in the upper panel of (B) and 20 µM in the lower or magnified panel of (B–D).
Nevertheless, even this model is not flawless. First, although proliferating spheres can be easily obtained within 1–2 weeks of culture, the success rate for their generation is highly variable, ranging from 10 to 100%, and like PDXs, depending on the laboratory expertise and, most likely, on the nature of the initial human specimen (69). Second, experimental standardization remains challenging, given the variability among xenografts generated from different patients. Third, like other models involving manipulation of the original tumor before injection into the animal, there might be a selection of a specific subpopulation (in this case of the one endowed with stem-like features) that could result in an under-representation of the genetic and functional cellular heterogeneity found in the patient’s tumor. However, we believe that selection of GICs could be a more accurate way to generate an animal model aimed at testing therapeutics to target therapy resistant cells and/or prevent GBM recurrence. The first-line of treatment for GBM patients is the surgical removal of the tumor mass. Since many studies confirmed that GICs are responsible for tumor recurrence (26, 29) and GICs regulate GBM molecular heterogeneity (70), the use of PDOX and/or PDX clinical trials (12), to develop strategies to target GICs may be more effective in overcoming therapy resistance caused by intratumoral heterogeneity (71). The PDOX models generated with genomically defined GICs could be a more ideal model to develop and test such therapeutic strategies tailored for each patient and/or pointed toward prevention or delay of recurrence.
Here, we presented a concise overview of the available preclinical models of GBM and explained their benefits and pitfalls. Although all described models have their unique utility, we can assert with confidence that some are better than others, especially in the context of testing therapeutics aimed at preventing GBM recurrence. Within this scope, the model of election is represented by PDOX models, which allow, to a certain extent, the recreation of the genetic, histologic, and morphologic profiles of human GBM. Particularly, our experimental work and that of other labs showed that, if preventing or delaying recurrence is the goal, GIC neurosphere-derived models are excellent. However, although informative, this model can still be largely improved by addressing some of its major limitations. For instance, we should consider the cellular plasticity associated with GICs (72), and evaluate how heterogeneity and plasticity influence generation of neurospheres or organoids in vitro (73). Moreover, solutions to address the experimental standardization of PDOX would be desirable, especially for testing antineoplastic agents. Mouse PDOX could be preceded with rapid screening assays such as the zebrafish PDX assay using fewer cells and multiple clones from the same tumor (74) to screen for response to single and/or combination therapy tailored against patient’s specific perturbations, as we (14, 75, 76) and others (77) have recently shown, could shorten the time required to obtain the preclinical data needed for clinical decision making in precision medicine. Additionally, GBM PDOX and PDX models of other cancers suffer from the same limitations in terms of under-representation of the human cellular stromal and immunologic contribution to therapy outcome. The use of “humanized” mice (expressing components of the human immune system) and the co-injection of human stromal/immune cells and human cytokines, together with patient-derived cancer cells might solve this later limitation (78).
To maximize the utility of patient-derived GBM models, both the scientific investigation and the drug discovery process should consider the characteristics of the different models and utilize the most appropriate one for the specific scientific question/investigational goal to be addressed. The use of multiple models is likely to be required to comprehensively assess treatment efficacy. If we do not fall into the temptation of generalizing the findings obtained from one model to the whole field of cancer investigations, GBM preclinical models can be very informative, even at their present state.
Glioblastoma studies were performed using surgical specimens of consented glioma patients collected through Rutgers Cancer Institute of New Jersey Biospecimen Repository Service using protocols approved by the Institutional Review Board of Rutgers University. Animal studies were conducted using a protocol approved by the Institutional Animal Care and Use Committee of Rutgers University.
MP: designed research, performed research, analyzed data, and wrote the manuscript. MB: designed research, performed research, analyzed data, and edited the manuscript. SP: designed research, analyzed data, and edited the manuscript. HS: designed research, performed research, analyzed data, wrote the manuscript, and supervised the study.
The authors declare that the research was conducted in the absence of any commercial or financial relationships that could be construed as a potential conflict of interest.
The reviewer SL and the handling editor declared their shared affiliation.
We thank Rutgers Cancer Institute of New Jersey Biorepository and Histology Services, including Julia Arnsdorff, Kelly Walton, Lucyyann Franciosa, Lei Cong, and Shafiq Bhat.
This project is supported by National Cancer Institute (F31CA220839 to MP, R01CA190578 to SP, and P30CA072720 to SL) and New Jersey Health Foundation award (Research grant PC-72-16 to HS).
1. Hutchinson L, Kirk R. High drug attrition rates – where are we going wrong? Nat Rev Clin Oncology (2011) 8(4):189–90. doi:10.1038/nrclinonc.2011.34
2. Kamb A. What’s wrong with our cancer models? Nat Rev Drug Discov (2005) 4(2):161–5. doi:10.1038/nrd1635
3. Hanahan D, Weinberg RA. Hallmarks of cancer: the next generation. Cell (2011) 144(5):646–74. doi:10.1016/j.cell.2011.02.013
4. Sharma SV, Haber DA, Settleman J. Cell line-based platforms to evaluate the therapeutic efficacy of candidate anticancer agents. Nat Rev Cancer (2010) 10(4):241–53. doi:10.1038/nrc2820
5. Radaelli E, Ceruti R, Patton V, Russo M, Degrassi A, Croci V, et al. Immunohistopathological and neuroimaging characterization of murine orthotopic xenograft models of glioblastoma multiforme recapitulating the most salient features of human disease. Histol Histopathol (2009) 24(7):879–91. doi:10.14670/HH-24.879
6. Gillet JP, Varma S, Gottesman MM. The clinical relevance of cancer cell lines. J Natl Cancer Inst (2013) 105(7):452–8. doi:10.1093/jnci/djt007
7. Zanoni M, Piccinini F, Arienti C, Zamagni A, Santi S, Polico R, et al. 3D tumor spheroid models for in vitro therapeutic screening: a systematic approach to enhance the biological relevance of data obtained. Sci Rep (2016) 6:19103. doi:10.1038/srep19103
8. EurOPDX Consortium. Available from: http://europdx.eu/pdx-collection.html
9. NCI Patient-Derived Models Repository. Available from: https://pdmr.cancer.gov/
10. Tentler JJ, Tan AC, Weekes CD, Jimeno A, Leong S, Pitts TM, et al. Patient-derived tumour xenografts as models for oncology drug development. Nat Rev Clin Oncol (2012) 9(6):338–50. doi:10.1038/nrclinonc.2012.61
11. Malaney P, Nicosia SV, Dave V. One mouse, one patient paradigm: new avatars of personalized cancer therapy. Cancer Lett (2014) 344(1):1–12. doi:10.1016/j.canlet.2013.10.010
12. Gao H, Korn JM, Ferretti S, Monahan JE, Wang Y, Singh M, et al. High-throughput screening using patient-derived tumor xenografts to predict clinical trial drug response. Nat Med (2015) 21(11):1318–25. doi:10.1038/nm.3954
13. Hidalgo M, Amant F, Biankin AV, Budinska E, Byrne AT, Caldas C, et al. Patient-derived xenograft models: an emerging platform for translational cancer research. Cancer Discov (2014) 4(9):998–1013. doi:10.1158/2159-8290.CD-14-0001
14. Bansal N, Bartucci M, Yusuff S, Davis S, Flaherty K, Huselid E, et al. BMI-1 targeting interferes with patient-derived tumor-initiating cell survival and tumor growth in prostate cancer. Clin Cancer Res (2016) 22(24):6176–91. doi:10.1158/1078-0432.CCR-15-3107
15. Morgan KM, Riedlinger GM, Rosenfeld J, Ganesan S, Pine SR. Patient-derived xenograft models of non-small cell lung cancer and their potential utility in personalized medicine. Front Oncol (2017) 7:2. doi:10.3389/fonc.2017.00002
16. Siegel RL, Miller KD, Jemal A. Cancer statistics, 2017. CA Cancer J Clin (2017) 67(1):7–30. doi:10.3322/caac.21387
17. Verhaak RG, Hoadley KA, Purdom E, Wang V, Qi Y, Wilkerson MD, et al. Integrated genomic analysis identifies clinically relevant subtypes of glioblastoma characterized by abnormalities in PDGFRA, IDH1, EGFR, and NF1. Cancer Cell (2010) 17(1):98–110. doi:10.1016/j.ccr.2009.12.020
18. Patel AP, Tirosh I, Trombetta JJ, Shalek AK, Gillespie SM, Wakimoto H, et al. Single-cell RNA-seq highlights intratumoral heterogeneity in primary glioblastoma. Science (2014) 344(6190):1396–401. doi:10.1126/science.1254257
19. Stupp R, Hegi ME, Mason WP, van den Bent MJ, Taphoorn MJ, Janzer RC, et al. Effects of radiotherapy with concomitant and adjuvant temozolomide versus radiotherapy alone on survival in glioblastoma in a randomised phase III study: 5-year analysis of the EORTC-NCIC trial. Lancet Oncol (2009) 10(5):459–66. doi:10.1016/S1470-2045(09)70025-7
20. Yu Z, Zhao G, Zhang Z, Li Y, Chen Y, Wang N, et al. Efficacy and safety of bevacizumab for the treatment of glioblastoma. Exp Ther Med (2016) 11(2):371–80. doi:10.3892/etm.2015.2947
21. Siegel RL, Miller KD, Jemal A. Cancer statistics, 2016. CA Cancer J Clin (2016) 66(1):7–30. doi:10.3322/caac.21332
22. Allen M, Bjerke M, Edlund H, Nelander S, Westermark B. Origin of the U87MG glioma cell line: good news and bad news. Sci Transl Med (2016) 8(354):354re3. doi:10.1126/scitranslmed.aaf6853
23. Kreso A, Dick JE. Evolution of the cancer stem cell model. Cell Stem Cell (2014) 14(3):275–91. doi:10.1016/j.stem.2014.02.006
24. Singh SK, Hawkins C, Clarke ID, Squire JA, Bayani J, Hide T, et al. Identification of human brain tumour initiating cells. Nature (2004) 432(7015):396–401. doi:10.1038/nature03128
25. Lathia JD, Mack SC, Mulkearns-Hubert EE, Valentim CL, Rich JN. Cancer stem cells in glioblastoma. Genes Dev (2015) 29(12):1203–17. doi:10.1101/gad.261982.115
26. Cho DY, Lin SZ, Yang WK, Lee HC, Hsu DM, Lin HL, et al. Targeting cancer stem cells for treatment of glioblastoma multiforme. Cell Transplant (2013) 22(4):731–9. doi:10.3727/096368912X655136
27. Apostoli AJ, Ailles L. Clonal evolution and tumor-initiating cells: new dimensions in cancer patient treatment. Crit Rev Clin Lab Sci (2016) 53(1):40–51. doi:10.3109/10408363.2015.1083944
28. Dingli D, Michor F. Successful therapy must eradicate cancer stem cells. Stem Cells (2006) 24(12):2603–10. doi:10.1634/stemcells.2006-0136
29. Sarkaria JN, Kitange GJ, James CD, Plummer R, Calvert H, Weller M, et al. Mechanisms of chemoresistance to alkylating agents in malignant glioma. Clin Cancer Res (2008) 14(10):2900–8. doi:10.1158/1078-0432.CCR-07-1719
30. Son MJ, Woolard K, Nam DH, Lee J, Fine HA. SSEA-1 is an enrichment marker for tumor-initiating cells in human glioblastoma. Cell Stem Cell (2009) 4(5):440–52. doi:10.1016/j.stem.2009.03.003
31. Senner V, Sturm A, Baur I, Schrell UH, Distel L, Paulus W. CD24 promotes invasion of glioma cells in vivo. J Neuropathol Exp Neurol (1999) 58(8):795–802. doi:10.1097/00005072-199908000-00002
32. Shu Q, Wong KK, Su JM, Adesina AM, Yu LT, Tsang YT, et al. Direct orthotopic transplantation of fresh surgical specimen preserves CD133+ tumor cells in clinically relevant mouse models of medulloblastoma and glioma. Stem Cells (2008) 26(6):1414–24. doi:10.1634/stemcells.2007-1009
33. Clement V, Dutoit V, Marino D, Dietrich PY, Radovanovic I. Limits of CD133 as a marker of glioma self-renewing cells. Int J Cancer (2009) 125(1):244–8. doi:10.1002/ijc.24352
34. Wang J, Sakariassen PO, Tsinkalovsky O, Immervoll H, Boe SO, Svendsen A, et al. CD133 negative glioma cells form tumors in nude rats and give rise to CD133 positive cells. Int J Cancer (2008) 122(4):761–8. doi:10.1002/ijc.23130
35. Azari H, Millette S, Ansari S, Rahman M, Deleyrolle LP, Reynolds BA. Isolation and expansion of human glioblastoma multiforme tumor cells using the neurosphere assay. J Vis Exp (2011) 56:e3633. doi:10.3791/3633
36. Laks DR, Masterman-Smith M, Visnyei K, Angenieux B, Orozco NM, Foran I, et al. Neurosphere formation is an independent predictor of clinical outcome in malignant glioma. Stem Cells (2009) 27(4):980–7. doi:10.1002/stem.15
37. Singh DK, Kollipara RK, Vemireddy V, Yang XL, Sun Y, Regmi N, et al. Oncogenes activate an autonomous transcriptional regulatory circuit that drives glioblastoma. Cell Rep (2017) 18(4):961–76. doi:10.1016/j.celrep.2016.12.064
38. Garros-Regulez L, Aldaz P, Arrizabalaga O, Moncho-Amor V, Carrasco-Garcia E, Manterola L, et al. mTOR inhibition decreases SOX2-SOX9 mediated glioma stem cell activity and temozolomide resistance. Expert Opin Ther Targets (2016) 20(4):393–405. doi:10.1517/14728222.2016.1151002
39. Lee J, Kotliarova S, Kotliarov Y, Li A, Su Q, Donin NM, et al. Tumor stem cells derived from glioblastomas cultured in bFGF and EGF more closely mirror the phenotype and genotype of primary tumors than do serum-cultured cell lines. Cancer Cell (2006) 9(5):391–403. doi:10.1016/j.ccr.2006.03.030
40. Mehta M, Khan A, Danish S, Haffty BG, Sabaawy HE. Radiosensitization of primary human glioblastoma stem-like cells with low-dose AKT inhibition. Mol Cancer Ther (2015) 14(5):1171–80. doi:10.1158/1535-7163.MCT-14-0708
41. Teng J, Carla da Hora C, Kantar RS, Nakano I, Wakimoto H, Batchelor TT, et al. Dissecting inherent intratumor heterogeneity in patient-derived glioblastoma culture models. Neuro Oncol (2017) 19(6):820–32. doi:10.1093/neuonc/now253
42. Facchino S, Abdouh M, Bernier G. Brain cancer stem cells: current status on glioblastoma multiforme. Cancers (Basel) (2011) 3(2):1777–97. doi:10.3390/cancers3021777
43. Galli R, Binda E, Orfanelli U, Cipelletti B, Gritti A, De Vitis S, et al. Isolation and characterization of tumorigenic, stem-like neural precursors from human glioblastoma. Cancer Res (2004) 64(19):7011–21. doi:10.1158/0008-5472.CAN-04-1364
44. Xie Y, Bergstrom T, Jiang Y, Johansson P, Marinescu VD, Lindberg N, et al. The human glioblastoma cell culture resource: validated cell models representing all molecular subtypes. EBioMedicine (2015) 2(10):1351–63. doi:10.1016/j.ebiom.2015.08.026
45. Pardridge WM. The blood-brain barrier: bottleneck in brain drug development. NeuroRx (2005) 2(1):3–14. doi:10.1602/neurorx.2.1.3
46. Huszthy PC, Daphu I, Niclou SP, Stieber D, Nigro JM, Sakariassen PO, et al. In vivo models of primary brain tumors: pitfalls and perspectives. Neuro Oncol (2012) 14(8):979–93. doi:10.1093/neuonc/nos135
47. Zheng H, Ying H, Yan H, Kimmelman AC, Hiller DJ, Chen AJ, et al. p53 and Pten control neural and glioma stem/progenitor cell renewal and differentiation. Nature (2008) 455(7216):1129–33. doi:10.1038/nature07443
48. Zhu Y, Guignard F, Zhao D, Liu L, Burns DK, Mason RP, et al. Early inactivation of p53 tumor suppressor gene cooperating with NF1 loss induces malignant astrocytoma. Cancer Cell (2005) 8(2):119–30. doi:10.1016/j.ccr.2005.07.004
49. Kwon CH, Zhao D, Chen J, Alcantara S, Li Y, Burns DK, et al. Pten haploinsufficiency accelerates formation of high-grade astrocytomas. Cancer Res (2008) 68(9):3286–94. doi:10.1158/0008-5472.CAN-07-6867
50. Alcantara Llaguno S, Chen J, Kwon CH, Jackson EL, Li Y, Burns DK, et al. Malignant astrocytomas originate from neural stem/progenitor cells in a somatic tumor suppressor mouse model. Cancer Cell (2009) 15(1):45–56. doi:10.1016/j.ccr.2008.12.006
51. Lin F, de Gooijer MC, Hanekamp D, Chandrasekaran G, Buil LC, Thota N, et al. PI3K-mTOR pathway inhibition exhibits efficacy against high-grade glioma in clinically relevant mouse models. Clin Cancer Res (2017) 23(5):1286–98. doi:10.1158/1078-0432.CCR-16-1276
52. Driessens G, Beck B, Caauwe A, Simons BD, Blanpain C. Defining the mode of tumour growth by clonal analysis. Nature (2012) 488(7412):527–30. doi:10.1038/nature11344
53. Chen J, Li Y, Yu TS, McKay RM, Burns DK, Kernie SG, et al. A restricted cell population propagates glioblastoma growth after chemotherapy. Nature (2012) 488(7412):522–6. doi:10.1038/nature11287
54. Schepers AG, Snippert HJ, Stange DE, van den Born M, van Es JH, van de Wetering M, et al. Lineage tracing reveals Lgr5+ stem cell activity in mouse intestinal adenomas. Science (2012) 337(6095):730–5. doi:10.1126/science.1224676
55. Venere M, Hamerlik P, Wu Q, Rasmussen RD, Song LA, Vasanji A, et al. Therapeutic targeting of constitutive PARP activation compromises stem cell phenotype and survival of glioblastoma-initiating cells. Cell Death Differ (2014) 21(2):258–69. doi:10.1038/cdd.2013.136
56. Lan X, Jorg DJ, Cavalli FMG, Richards LM, Nguyen LV, Vanner RJ, et al. Fate mapping of human glioblastoma reveals an invariant stem cell hierarchy. Nature (2017) 549(7671):227–32. doi:10.1038/nature23666
57. Quail DF, Bowman RL, Akkari L, Quick ML, Schuhmacher AJ, Huse JT, et al. The tumor microenvironment underlies acquired resistance to CSF-1R inhibition in gliomas. Science (2016) 352(6288):aad3018. doi:10.1126/science.aad3018
58. Kim MP, Evans DB, Wang H, Abbruzzese JL, Fleming JB, Gallick GE. Generation of orthotopic and heterotopic human pancreatic cancer xenografts in immunodeficient mice. Nat Protoc (2009) 4(11):1670–80. doi:10.1038/nprot.2009.171
59. Clark MJ, Homer N, O’Connor BD, Chen Z, Eskin A, Lee H, et al. U87MG decoded: the genomic sequence of a cytogenetically aberrant human cancer cell line. PLoS Genet (2010) 6(1):e1000832. doi:10.1371/journal.pgen.1000832
60. Joo KM, Kim J, Jin J, Kim M, Seol HJ, Muradov J, et al. Patient-specific orthotopic glioblastoma xenograft models recapitulate the histopathology and biology of human glioblastomas in situ. Cell Rep (2013) 3(1):260–73. doi:10.1016/j.celrep.2012.12.013
61. Oh YT, Cho HJ, Kim J, Lee JH, Rho K, Seo YJ, et al. Translational validation of personalized treatment strategy based on genetic characteristics of glioblastoma. PLoS One (2014) 9(8):e103327. doi:10.1371/journal.pone.0103327
62. Kitange GJ, Carlson BL, Mladek AC, Decker PA, Schroeder MA, Wu W, et al. Evaluation of MGMT promoter methylation status and correlation with temozolomide response in orthotopic glioblastoma xenograft model. J Neurooncol (2009) 92(1):23–31. doi:10.1007/s11060-008-9737-8
63. Gupta SK, Kizilbash SH, Carlson BL, Mladek AC, Boakye-Agyeman F, Bakken KK, et al. Delineation of MGMT hypermethylation as a biomarker for veliparib-mediated temozolomide-sensitizing therapy of glioblastoma. J Natl Cancer Inst (2016) 108(5). doi:10.1093/jnci/djv369
64. Ben-David U, Ha G, Tseng YY, Greenwald NF, Oh C, Shih J, et al. Patient-derived xenografts undergo mouse-specific tumor evolution. Nat Genet (2017) 49(11):1567–75. doi:10.1038/ng.3967
65. Kim KM, Shim JK, Chang JH, Lee JH, Kim SH, Choi J, et al. Failure of a patient-derived xenograft for brain tumor model prepared by implantation of tissue fragments. Cancer Cell Int (2016) 16:43. doi:10.1186/s12935-016-0319-0
66. Wang J, Miletic H, Sakariassen PO, Huszthy PC, Jacobsen H, Brekka N, et al. A reproducible brain tumour model established from human glioblastoma biopsies. BMC Cancer (2009) 9:465. doi:10.1186/1471-2407-9-465
67. Hubert CG, Rivera M, Spangler LC, Wu Q, Mack SC, Prager BC, et al. A three-dimensional organoid culture system derived from human glioblastomas recapitulates the hypoxic gradients and cancer stem cell heterogeneity of tumors found in vivo. Cancer Res (2016) 76(8):2465–77. doi:10.1158/0008-5472.CAN-15-2402
68. Jain RK, di Tomaso E, Duda DG, Loeffler JS, Sorensen AG, Batchelor TT. Angiogenesis in brain tumours. Nat Rev Neurosci (2007) 8(8):610–22. doi:10.1038/nrn2175
69. Wan F, Zhang S, Xie R, Gao B, Campos B, Herold-Mende C, et al. The utility and limitations of neurosphere assay, CD133 immunophenotyping and side population assay in glioma stem cell research. Brain Pathol (2010) 20(5):877–89. doi:10.1111/j.1750-3639.2010.00379.x
70. Garner JM, Ellison DW, Finkelstein D, Ganguly D, Du Z, Sims M, et al. Molecular heterogeneity in a patient-derived glioblastoma xenoline is regulated by different cancer stem cell populations. PLoS One (2015) 10(5):e0125838. doi:10.1371/journal.pone.0125838
71. Jin X, Kim LJY, Wu Q, Wallace LC, Prager BC, Sanvoranart T, et al. Targeting glioma stem cells through combined BMI1 and EZH2 inhibition. Nat Med (2017) 23(11):1352–61. doi:10.1038/nm.4415
72. Friedmann-Morvinski D. Glioblastoma heterogeneity and cancer cell plasticity. Crit Rev Oncog (2014) 19(5):327–36. doi:10.1615/CritRevOncog.2014011777
73. Iwadate Y. Epithelial-mesenchymal transition in glioblastoma progression. Oncol Lett (2016) 11(3):1615–20. doi:10.3892/ol.2016.4113
74. Sabaawy HE. Genetic heterogeneity and clonal evolution of tumor cells and their impact on precision cancer medicine. J Leuk (2013) 1(4):1000124. doi:10.4172/2329-6917.1000124
75. Bansal N, Davis S, Tereshchenko I, Budak-Alpdogan T, Zhong H, Stein MN, et al. Enrichment of human prostate cancer cells with tumor initiating properties in mouse and zebrafish xenografts by differential adhesion. Prostate (2014) 74(2):187–200. doi:10.1002/pros.22740
76. Bartucci M, Hussein MS, Huselid E, Flaherty K, Patrizii M, Laddha SV, et al. Synthesis and characterization of novel BMI1 inhibitors targeting cellular self-renewal in Hepatocellular Carcinoma. Target Oncol (2017) 12(4):449–62. doi:10.1007/s11523-017-0501-x
77. Fior R, Povoa V, Mendes RV, Carvalho T, Gomes A, Figueiredo N, et al. Single-cell functional and chemosensitive profiling of combinatorial colorectal therapy in zebrafish xenografts. Proc Natl Acad Sci U S A (2017) 114(39):E8234–43. doi:10.1073/pnas.1618389114
Keywords: glioblastoma, patient-derived xenografts, mouse models, personalized therapy, precision medicine
Citation: Patrizii M, Bartucci M, Pine SR and Sabaawy HE (2018) Utility of Glioblastoma Patient-Derived Orthotopic Xenografts in Drug Discovery and Personalized Therapy. Front. Oncol. 8:23. doi: 10.3389/fonc.2018.00023
Received: 28 August 2017; Accepted: 22 January 2018;
Published: 12 February 2018
Edited by:
Zhi Sheng, Virginia Tech, United StatesReviewed by:
Shiv K. Gupta, Mayo Clinic Minnesota, United StatesCopyright: © 2018 Patrizii, Bartucci, Pine and Sabaawy. This is an open-access article distributed under the terms of the Creative Commons Attribution License (CC BY). The use, distribution or reproduction in other forums is permitted, provided the original author(s) and the copyright owner are credited and that the original publication in this journal is cited, in accordance with accepted academic practice. No use, distribution or reproduction is permitted which does not comply with these terms.
*Correspondence: Hatem E. Sabaawy, c2FiYWF3aGVAcnV0Z2Vycy5lZHU=
Disclaimer: All claims expressed in this article are solely those of the authors and do not necessarily represent those of their affiliated organizations, or those of the publisher, the editors and the reviewers. Any product that may be evaluated in this article or claim that may be made by its manufacturer is not guaranteed or endorsed by the publisher.
Research integrity at Frontiers
Learn more about the work of our research integrity team to safeguard the quality of each article we publish.