- 1Department of Radiation Oncology, Dana-Farber Cancer Institute, Brigham and Women’s Hospital, Harvard Medical School, Boston MA, USA
- 2Department of Radiology, Dana-Farber Cancer Institute, Harvard Medical School, Boston MA, USA
Purpose: Although change in standardized uptake value (SUV) measures and PET-based textural features during treatment have shown promise in tumor response prediction, it is unclear which quantitative measure is the most predictive. We compared the relationship between PET-based features and pathologic response and overall survival with the SUV measures in esophageal cancer.
Methods: Fifty-four esophageal cancer patients received PET/CT scans before and after chemoradiotherapy. Of these, 45 patients underwent surgery and were classified into complete, partial, and non-responders to the preoperative chemoradiation. SUVmax and SUVmean, two cooccurrence matrix (Entropy and Homogeneity), two run-length matrix (RLM) (high-gray-run emphasis and Short-run high-gray-run emphasis), and two size-zone matrix (high-gray-zone emphasis and short-zone high-gray emphasis) textures were computed. The relationship between the relative difference of each measure at different treatment time points and the pathologic response and overall survival was assessed using the area under the receiver-operating-characteristic curve (AUC) and Kaplan–Meier statistics, respectively.
Results: All Textures, except Homogeneity, were better related to pathologic response than SUVmax and SUVmean. Entropy was found to significantly distinguish non-responders from the complete (AUC = 0.79, p = 1.7 × 10−4) and partial (AUC = 0.71, p = 0.01) responders. Non-responders can also be significantly differentiated from partial and complete responders by the change in the run-length and size-zone matrix textures (AUC = 0.71–0.76, p ≤ 0.02). Homogeneity, SUVmax, and SUVmean failed to differentiate between any of the responders (AUC = 0.50–0.57, p ≥ 0.46). However, none of the measures were found to significantly distinguish between complete and partial responders with AUC <0.60 (p = 0.37). Median Entropy and RLM textures significantly discriminated patients with good and poor survival (log-rank p < 0.02), while all other textures and survival were poorly related (log-rank p > 0.25).
Conclusion: For the patients studied, temporal changes in Entropy and all RLM were better correlated with pathological response and survival than the SUV measures. The hypothesis that these metrics can be used as clinical predictors of better patient outcomes will be tested in a larger patient dataset in the future.
Introduction
Esophageal cancer is among the most aggressive gastrointestinal cancers with a high mortality rate (1, 2). Neoadjuvant chemotherapy and, or, radiotherapy are commonly used in combination with surgery to provide systemic and local control of the disease (3–5). Pathologic examinations of the surgical specimen have shown that preoperative chemoradiation alone can eradicate viable tumor cells in 10–29% of the patients (3, 6–9). Early prediction of the pathologic response allows physicians to identify which patients may or may not benefit from chemoradiotherapy, subsequently selecting an effective therapy for individual patients, while avoiding complications associated with ineffective or unnecessary treatment.
Non-invasive position emission tomography (PET) imaging with 18F-fluorodoxyglucose ([18F]FDG) is increasingly utilized for imaging of glucose metabolism for esophageal cancer diagnosis, staging, and monitoring disease progression (10–13). Due to its quantitative nature, standardized uptake value (SUV) measures, such as maximum and mean SUV, have been used to quantify tumor characteristics (14, 15). Furthermore, the reduction of SUVmax and SUVmean between the longitudinal PET images has been shown to be significant predictors of tumor response to preoperative therapy and patients’ overall survival (16–23). However, SUVmax and SUVmean fail to capture the heterogeneity in intratumoral [18F]FDG uptake resulting from the spatial variations in biological and genetic properties (24–26). Intratumoral heterogeneity is often found in cancer patients and has been shown to correlate with poor prognosis and treatment resistance (27–29). Accurate quantification of tumor heterogeneity may lead to more accurate prediction of treatment response (30, 31).
Textural features extracted from PET images through complex mathematical models of the spatial relationship between multiple voxels and their neighborhood have been proposed to describe the tumor heterogeneity (25, 26, 32, 33). For example, gray level cooccurrence matrix (GLCM) textures, such as Homogeneity and Entropy, measure the local relationship between two voxels (voxel pair) (34, 35). Tan et al. observed that [18F]FDG uptake became more homogeneous in the tumors that responded to preoperative chemoradiotherapy (22, 36). They used local GLCM textures to describe the evolution of the [18F]FDG uptake during therapy and found that the textures outperformed SUV measure in predicting the pathologic response (22).
Regional textures, such as those derived from the run-length matrix (RLM) and size-zone matrix (SZM), assess the spatial relationship of voxels beyond two neighboring voxels (37, 38). In a study by Yang et al., 20 patients with cervical cancer were classified into metabolic complete responders, partial responders, and new disease according to the PET images acquired 3 months after the concurrent chemoradiotherapy (39). They observed that the early changes (2–4 weeks) in the RLM and SZM textures during the therapy were more sensitive than SUV measures in detecting the metabolic tumor responders (39). Their results therefore suggested that the RLM and SZM textures may be a more significant prognostic indicator than the SUV measures.
Although changes in SUV measures and PET-based textural features during treatment have shown promise in tumor response prediction, it is unclear which quantitative measure is the most predictive. In this study, we evaluated the relationship between the change in PET-based texture features and overall survival and pathologic response to preoperative chemoradiotherapy in esophageal cancer patients and compared to the same correlations of standard SUV measures. We attempted to generate a hypothesis regarding which texture features, if any, should be explored as predictors of pathologic response and patient outcome. Two sensitivity studies were also conducted to investigate the impact of change in PET resampling scheme and tumor volume on the association between textures and pathologic response.
Materials and Methods
Patient Characteristics and Imaging
This retrospective study was conducted under a Dana-Farber/Harvard Cancer Center institutional review board (IRB) approved protocol. All images and clinical data were analyzed anonymously and retrospectively. This study included 54 patients (10 females and 44 males, median age = 65 years) with esophageal cancer (one tumor/patient) received [18F]FDG–PET/CT scans, on average, 9 ± 1 weeks before and 5 ± 5 weeks after the chemoradiotherapy between August 2009 and April 2013. There were fifty patients with adenocarcinoma and four patients with squamous cell carcinoma. Table 1 shows the clinical tumor stage assessed before the initiation of treatment according to the TNM staging criteria established by the American Joint Committee on Cancer (seventh edition).
Patients were injected with 10.3–22.4 mCi of [18F]FDG and scanned about 65 min after injection either on a GE scanner (GE Healthcare, Waukesha, WI, USA) or a Siemens Biograph PET/CT scanner (Siemens AG, Erlangen, Germany) based on the availability of the scanners. The acquisition time was 3–5 min/bed position for a whole-body scan from the base of skull to the proximal femora. The acquired PET data were reconstructed using 3D iterative VUE Point reconstruction (2 iterations/35 subsets for GE-DST) and order subset expectation maximization (2 iterations/28 subsets for GE-DSTE or DLS, 2 iterations/21 subsets for GE-DRX, and 4 iterations/8 subsets for Siemens Biograph). Attenuation correction of PET images was performed using the CT images. The types of crystals and the PET spatial resolution for each PET resolution are summarized in Table 2.
Chemoradiotherapy
Total radiation dose of 45–50.4 Gy were delivered to the patients over 5 weeks with five fractions per week (1.8 Gy/fractions and 1 fractions/day). The concurrent chemotherapy received by 18 patients included cisplatin combined with 5-fluorouracil (5-FU), irinotecan, or paclitaxel. Thirty-six patients received a chemotherapy regimen consisting of carboplatin and paclitaxel.
Surgery and Pathologic Response Classification
Of 54 patients, 45 (83%) underwent surgery after the concurrent chemoradiotherapy. Patients with low tolerance of surgery due to toxicity from chemoradiation and other medical problems were excluded from surgery. Surgery was performed, on average, 7 ± 2 weeks after preoperative chemoradiotherapy. All surgical specimens were examined and staged (ypTNM) by the pathologists (Table 1). Patients were further classified into complete responders, partial responders, and non-responders to preoperative chemoradiation. A complete responder was defined as having no microscopic evidence of viable tumor cells. A partial response was defined as the downstaging of pretreatment TNM staging. Patients who had no change or increased in tumor stage were defined as non-responders. Eight, twenty-two, and fifteen patients were identified as complete, partial, and non-responders, respectively.
Textural Features
A large number of textural features computed from complex mathematical models of the spatial relationship among multiple image voxels can be extracted from medical images (25, 26, 32, 35, 40, 41). However, if we were to assess the ability of numerous textures in predicting pathologic response, then at least some textures would be shown to be predictive merely based on random chance alone (42).
Therefore, only six textures, including GLCM-derived Homogeneity and Entropy (34, 35) were assessed. RLM-derived high-gray-run emphasis and Short-run high-gray-run emphasis (37) and SZM-derived high-gray-zone emphasis and short-zone high-gray emphasis were included for the analysis (38). These six PET-based textures were chosen due to their potential clinical value in prognosis and treatment response assessment (22, 30, 31, 39).
PET Textural Features Computation
We extracted the textural features from both the PET images acquired before (pretreatment) and after (posttreatment) chemoradiotherapy. Fifty-four tumor volumes were manually delineated by an experienced radiation oncologist using both the PET and CT images. For each patient, the CT counterparts of the pre- and posttreatment PET/CT images were deformably registered (43). The transformation resulting from the deformable registration was then applied to propagate the manually defined tumor volume on the pretreatment PET onto the posttreatment PET. The propagated tumor volumes were used to define the tumor region on the posttreatment PET. The deformable registration-based contour propagation has been shown to expedite the tumor contouring and texture quantification processes while not compromising the predictive ability of the textures (44).
Prior to texture computation, all PET images [PET()] were cropped to the tumor regions and processed using the following equation,
where minPET and maxPET are the maximum and minimum intensities of PET within the tumor region. The intensity range of the postprocessed image was resampled (or converted) into 256 (28) discrete values.
We calculated the metabolic tumor volumes (MTV) as thresholded PET images with SUV over 40% of the maximum SUV within the tumor regions (45, 46). Within the MTV, the textural features were then computed using the MATLAB-based (The Mathworks Inc., Natick, MA, USA) Chang-Gung Image Texture Analysis Toolbox (47, 48). The maximum and mean SUV were also computed from the pre- and posttreatment PET images.
Temporal Change in Quantitative Measures
The relative difference (ΔTexture) of each texture at different treatment time points was used to quantify the change in tumor texture values before and after chemoradiotherapy. The relative difference (ΔTexture) was defined as follows:
where Texturepre and Texturepost are the textural features extracted from the PET images acquired before and after the chemoradiotherapy, respectively. We also defined ΔSUV to determine the change in the SUV measures, including SUVmax and SUVmean, before and after chemoradiation.
Quantification of the ΔTexture and Pathologic Response Relation
Univariate analysis was performed with R (version 3.2) using the survcomp and pROC packages from Bioconductor (49, 50). We assessed the relationship between the quantitative measures and pathologic response by evaluating the performance of the measures to differentiate patients into different response classifications, including (1) complete from non-responders, (2) partial from non-responders, and (3) complete from partial responders. The performance was quantified using the area under the receiver operating characteristic curve (AUC). AUC is interpreted as the probability of correctly classifying the patients into different response categories (i.e., complete, partial, and non-responders). AUC ranges from 0 to 1 with the value 1 indicates perfect classification.
Survival Analysis
Two analyses were performed to assess the relationship between the quantitative measures and patients’ overall survival. In the first analysis, the median value of ΔTextures or ΔSUV was used to stratify all 54 patients into two risk groups. Kaplan–Meier curves with log-rank statistics were then performed to compare the outcomes between these two groups. Unlike Kaplan–Meier analysis, concordance index (c-index) does not rely on a single cutoff value (i.e., median ΔTexture or ΔSUV). In the second analysis, we computed the c-index. Given two randomly drawn samples (patients), c-index determines the probability that an event (death) will happen to the sample with higher risk value (e.g., ΔTexture) (51, 52).
In our analysis, c-index and AUC <0.50 indicate that the ΔTexture (or ΔSUV) performs worse than random guessing (52–54). Noether test was used to determine if AUC or c-index was significantly greater than 0.50 with p < 0.05 for each quantitative measure (55, 56).
Sensitivity Studies
We conducted two sensitivity studies to investigate the impact of change in PET resampling scheme and MTV on the performance of ΔTexture in differentiating pathologic response. In the first sensitivity study, PET images were also resampled to 32 (25), 64 (26), and 128 (27) discrete values using Eq. (1). In the second study, PET-based textures were determined within the MTV as thresholded PET images with SUV over 30% (MTV30%), 50% (MTV50%), and 60% (MTV60%) the SUVmax. The default parameters for the PET-based textures computation were 256 resampled discrete values and 40% SUVmax (MTV40%) threshold value.
Results
The Relationship between Pathologic Response and ΔTexture (ΔSUV)
The boxplots in Figure 1 visually highlight the performance of four example measures in differentiating non-responders from complete and partial responders. The temporal changes in texture (ΔTexture) generally were observed to be better related to pathologic response than ΔSUV (Figure 2). ΔEntropy was found to significantly distinguish non-responders from the complete (AUC = 0.79, p = 1.7 × 10−4) and partial (AUC = 0.71, p = 0.01) responders. Non-responders can also be significantly differentiated from partial and complete responders by the change in the run length and SZM textures (AUC = 0.71–0.76, p = 7.7 × 10−4–0.02) (Figure 2). ΔHomogeneity, ΔSUVmax, and ΔSUVmean failed to separate any of the responders (AUC = 0.50–0.57, p > 0.46). However, none of the measures were found to significantly distinguish between complete and partial responders with AUC = 0.51–0.59 (p > 0.37).
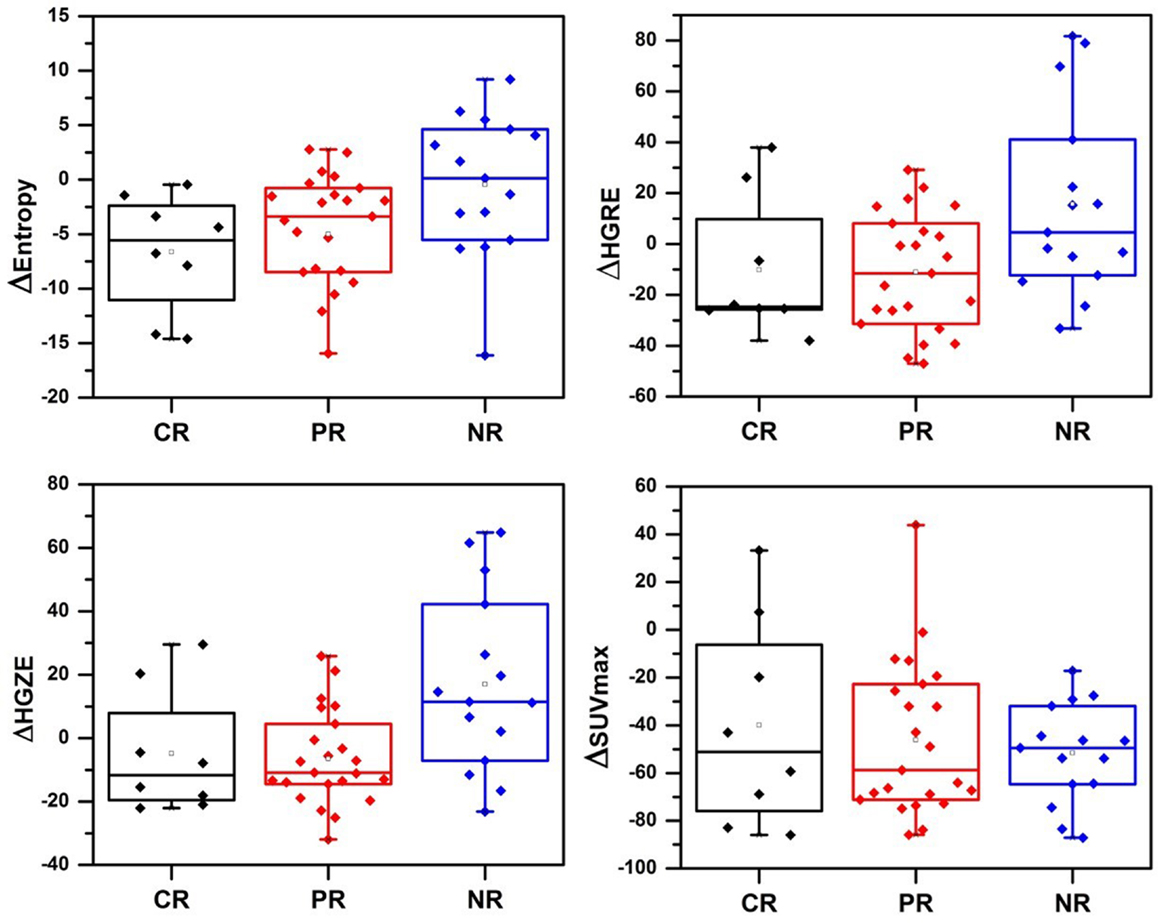
Figure 1. Change in textures and SUVmax before and after chemoradiation. CR, complete responders; PR, partial responders; NR, non-responder; HGRE, high-gray-run emphasis; HGZE, high-gray zone-run emphasis.
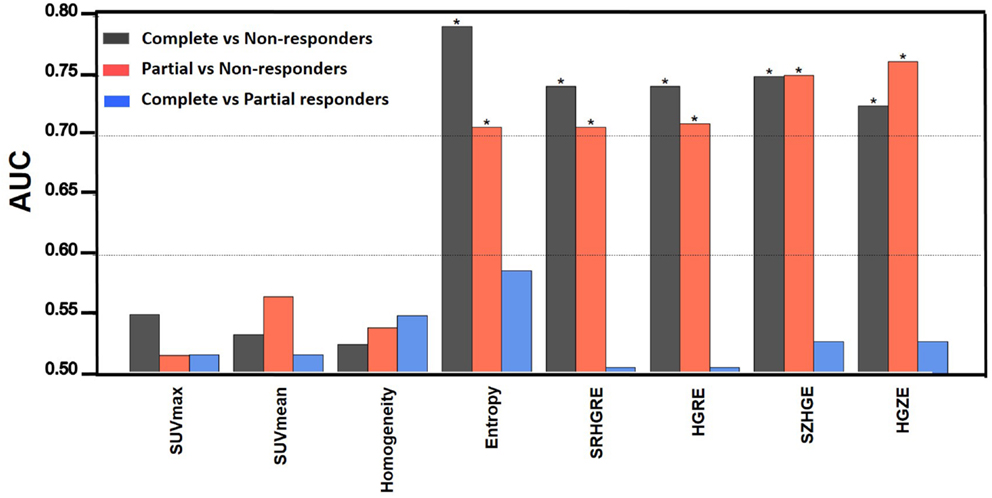
Figure 2. The area under the receiver operating curve (AUC). * indicates p < 0.05. HGRE, high-gray-run emphasis; HGZE, high-gray zone-run emphasis; SRHGRE, Short-run high-gray-run emphasis; SZHGE, short-zone high-gray-run emphasis.
Sensitivity Studies
While the relationship between ΔTextures and pathologic response generally became stronger with the increase in the number of discrete values, ΔRLM-derived textures and ΔHigh-ray-zone emphasis significantly distinguished non-responders from partial and complete responders for all discrete values (AUC = 0.70–0.77, p < 0.02) (Figure 3). Although ΔShort-zone high-gray emphasis significantly differentiated between complete and non-responders (AUC = 0.69–0.75, p < 0.05) for over 128 discrete values, the differentiation was poor for the texture computed with PET images resampled to <128 values with AUC~0.55 (p > 0.55). ΔEntropy computed with 32–256 discrete values increased its performance and significance between AUC = 0.55–0.79 and 0.59–0.71 for complete/non-responders and partial/non-responders differentiations respectively as observed in Figure 3.
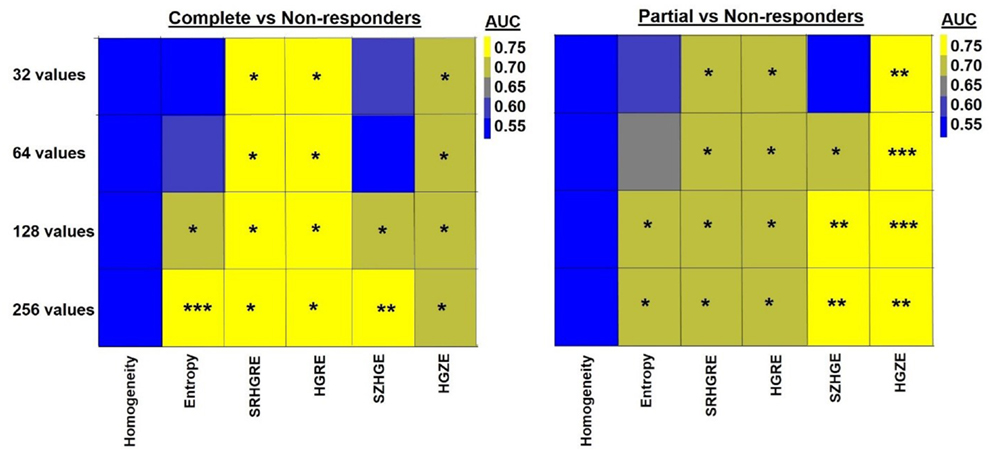
Figure 3. Heatmap shows the quantification (AUC) of the relationship between ΔTexture and pathologic response computed with 32, 64, 128, and 256 discrete values. * indicates 0.005 < p < 0.05, ** indicates 0.0005 < p < 0.005, *** indicates p < 0.0005. HGRE, high-gray-run emphasis; HGZE, high-gray zone-run emphasis; SRHGRE, Short-run high-gray-run emphasis; SZHGE, short-zone high-gray-run emphasis.
The MTV30%, MTV40%, MTV50%, and MTV60% on pretreatment PET images had median value of 28, 19, 12, and 7 cm3, respectively. The median posttreatment MTV30%, MTV40%, MTV50%, and MTV60% was 26, 14, 8, and 4 cm3, respectively.
While the relationship between the ΔTextures and pathologic response became stronger with decrease in threshold values, significant differentiation between complete and non-responders was found with ΔEntropy for all threshold values with AUC = 0.73–0.80, p < 0.05 (Figure 4). Figure 4 also shows that the ΔRLM textures computed with threshold values of 30–50% SUVmax both led to significant differentiation of non-responders from complete and partial responders with AUC = 0.71–0.81 (p < 0.02). ΔTextures computed within MTV60% were least related to the pathologic response. Response differentiation based on the 60% SUVmax volume calculated ΔRLM were moderate with only with AUC ~0.60 (p > 0.30) (Figure 4).
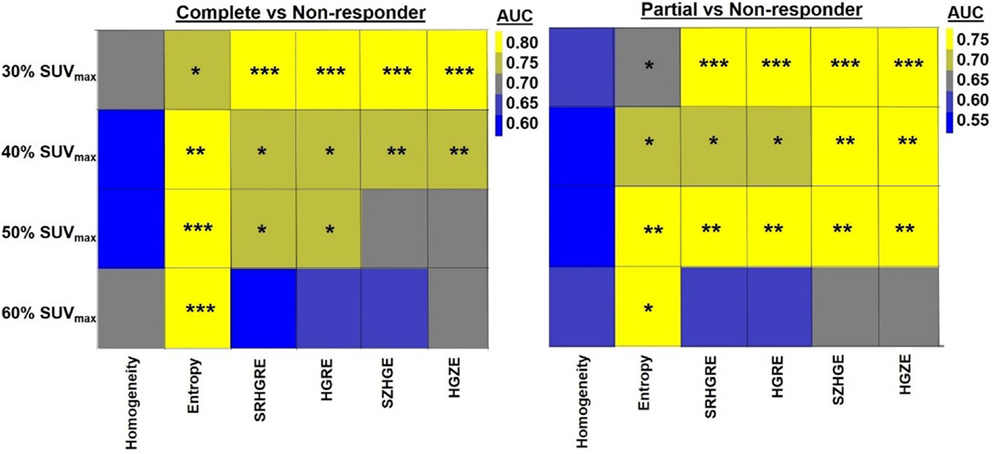
Figure 4. Heatmap shows the quantification (AUC) of the relationship between ΔTexture and pathologic response with the metabolic tumor volumes (MTV) of >30, 40, 50, and 60% SUVmax. * indicates 0.005 < p < 0.05, ** indicates 0.0005 < p < 0.005, *** indicates p < 0.0005. HGRE, high-gray-run emphasis; HGZE, high-gray zone-run emphasis; SRHGRE, Short-run high-gray-run emphasis; SZHGE, short-zone high-gray-run emphasis.
None of the threshold value, discrete value, and texture combination significantly differentiated complete from partial responders with AUC = 0.50–0.65 (p > 0.15). Regardless of the resampling schemes and threshold values, among all the textures, Homogeneity had the worst performance in identifying non-responders from complete (AUC = 0.51–0.66) and partial responders (AUC = 0.50–0.59) with p > 0.20.
Survival Analysis
The overall survival was defined as the time from initiation of treatment to patient’s death or censoring time. The median follow up of all 54 patients was 24.7 months. The median survival was 25.5 months. Kaplan–Meier curves shown in Figure 5. Figure 5 demonstrated that median ΔEntropy, ΔHigh-gray-run emphasis, ΔShort-run high-gray-run emphasis, and ΔHigh-gray-zone emphasis significantly discriminated patients with poor and good survival (log-rank test p < 0.02). Median values of Short-zone high-gray emphasis, ΔHomogeneity, ΔSUVmax, and ΔSUVmean failed to stratify patients into different survival groups (log-rank test p = 0.25–0.68).
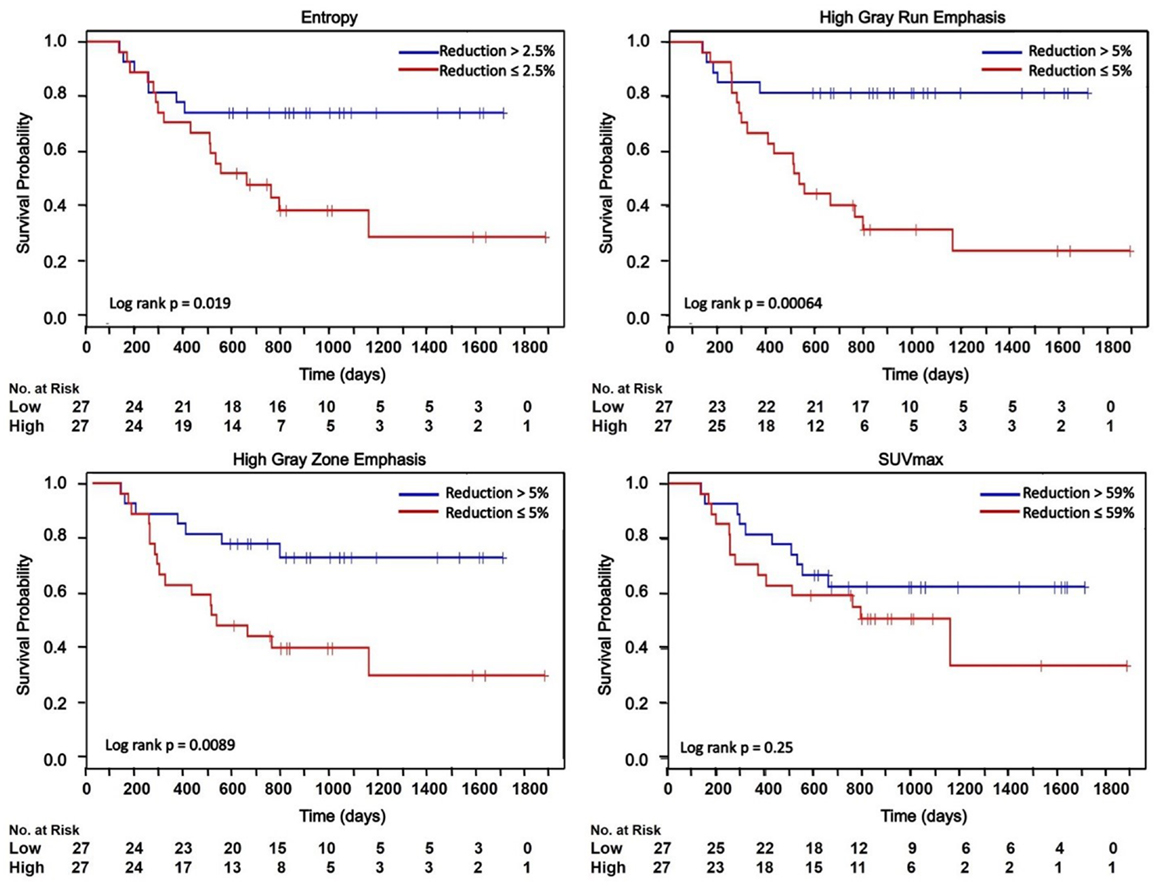
Figure 5. Kaplan–Meier curves dichotomized based on the median of ΔTextures and ΔSUVmax. HGRE, high-gray-run emphasis; HGZE, high-gray zone-run emphasis; SRHGRE, Short-run high-gray-run emphasis; SZHGE, short-zone high-gray-run emphasis. + indicates censored data.
However, c-index indicated that the performance of ΔHigh-gray-run emphasis, ΔShort-run high-gray-run emphasis, and ΔHigh-gray-zone emphasis were moderately related to the patients’ overall survival (c-index = 0.61–0.62, p = 0.06–0.08). All other measures performed poorly related to the overall survival (c-index = 0.52–0.58, p > 0.22).
Discussion
Although changes in SUV measures and PET-based textural features during treatment have shown promise in tumor response prediction, it is unclear which quantitative measure is the most predictive. In this study, we attempted to generate a hypothesis regarding which texture features, if any, should be explored as predictors of pathologic response and patient outcome.
Temporal changes in textural features are significantly related to the pathologic response to preoperative chemoradiotherapy, whereas SUV measures are not. Weber et al. observed the change in tumor [18F]FDG-PET uptake 2 weeks after neoadjuvant chemotherapy in 40 esophageal cancer patients. They found that the reduction of tumor SUVmax by 35% can best predict pathologic response with over 90% sensitivity and specificity (21). Song et al. found that the decrease in average tumor metabolic activity (SUVmean) significantly correlated with the pathologic response in 32 esophageal cancer patients undergoing neoadjuvant chemoradiotherapy (57). However, many studies, including ours, fail to confirm the association between the SUV measures and pathologic response (58–61). The conflicting findings may suggest that the SUV measures are inadequate for tumor characterization as they cannot fully describe the heterogeneity of intratumoral [18F]FDG distribution (25, 26). Studies therefore have proposed to use imaging features extracted from PET images to describe the [18F]FDG uptake heterogeneity (25, 26). Accurate description of the heterogeneous [18F]FDG distribution is important for assessing the underlying spatial variation in tumor biological and genetic properties (24), which may provide valuable information to improve treatment outcome prediction (22, 39). Our study confirms this hypothesis and finds that the changes in local GLCM-Entropy and regional (run length and SZM) textures (AUC >0.70) between longitudinal PET images outperformed the SUV measures (AUC ~0.55) in differentiating non-responders from complete and partial responders.
Computation of textural features requires a resampling scheme with at least 128 discrete values and MTV threshold value no more than 40% SUVmax. Orlhac et al. computed 31 PET-based textures using resampling schemes with 8 to 128 discrete values in 188 lesions from metastatic colorectal, lung, and breast cancer patients (62). They showed that the textures, especially Entropy and Short-zone high-gray emphasis, computed with <32 values are unreliable. They thus concluded that the textures should be computed with at least 32 discrete values. We also observed that the relationship between ΔTextures and pathologic response became stronger with the number of discrete values in the resampling schemes (Figure 3). Particularly, ΔEntropy and ΔShort-zone high-gray emphasis were found to be least robust to the resampling schemes (Figure 3). ΔEntropy and Short-zone high-gray emphasis computed with 32 and 64 discrete values performed poorly in separating complete and non-responders (AUC <0.60, p > 0.37), while the performance improved when 128 and 256 discrete values were used (AUC >0.70, p < 0.05) (Figure 3). ΔRLM textures and ΔHigh-gray-zone emphasis are robust to resampling scheme with AUC >0.70 for all discrete values.
Furthermore, we found that the relationship between the pathologic response and ΔTextures, except Entropy, became weaker with the increase in metabolic volume threshold values. Hatt et al. computed two local and two regional textures on 555 PET images consisting of breast, cervical, lung, esophageal, and head-and-neck tumors (63). They found that the PET-based textures computed for tumor size <10 cm3 do not provide important prognostic information. Our results are consistent with the findings of Hatt et al. We observed in Figure 4 that the ΔTextures computed with tumor volumes <10 cm3 based on 50–60% SUVmax thresholds were generally less related to the pathologic response than volumes >10 cm3 computed with thresholds of 30–40% SUVmax.
Temporal changes in tumor [18F]FDG distribution after chemoradiotherapy assessed by ΔRLM textures were moderately related to the patients’ overall survival. In the survival analysis, we dichotomized the Kaplan–Meier curves according to the median reduction in the texture values. Patients with reduction in texture greater than the median values were found to have significant survival benefit (Figure 5). For example, log-rank test showed that median ΔHigh-gray-run emphasis can significantly discriminate patients with good and poor survivals with p-value <10−3. However, the results of the Kaplan–Meier curves include a dichotomization based on a post hoc cutoff value. Concordance index is a more conservative measure that assesses the relationship between ΔTextures and survival without relying on a particular cutoff value (54). Among all textures, the relationship between the survival and ΔRLM textures was found to be the strongest with c-index = 0.62 comparing to the c-index <0.55 for ΔSUVmax and ΔSUVmean. In this study, the textures were extracted from PET images acquired before and after chemoradiotherapy, but prior to the surgery. Incorporating the survival benefit of surgery may lead to improvement of c-index. In future studies, we will build a multivariate predictive model of survival by incorporating the effect of surgery and combined textural features on a larger dataset.
Texture quantification has been shown to be sensitive to the acquisition modes and reconstruction parameters of PET images (64). In this study, we found that the temporal change in textures, such as Run length and Size zone textures, can significantly differentiate pathologic non-responders from responders with AUC >0.70 (p < 0.01) (Figure 2), despite the PET images were acquired from five different PET/CT scanners and reconstructed using different reconstruction parameters. We showed using Kruskal–Wallis test that the differences in ΔSUVmax and ΔSUVmean between different PET/CT scanners were not significant (p = 0.651 and p = 0.287 for ΔSUVmax and ΔSUVmean, respectively) (results not shown). The SUV measures were observed to decrease the most in images acquired by the GE discovery RX scanner. In particular, the average ΔSUVmax was found to be −53.5 ± 20.4, −55.4 ± 33.0, −64.0 ± 24.5, −47.9 (only one patient), and −57.1 ± 33.3 for the GE Discovery ST, STE, RX, and LS, and Siemens Biograph PET/CT scanners, respectively. The average ΔSUVmean was found to be −69.7 ± 21.3, −79.0 ± 17.2, −85.1 ± 8.2, −67.58 (only one patient), and −73.8 ± 29.7 for the GE Discovery ST, STE, RX, and LS, and Siemens Biograph PET/CT scanners, respectively. Our results suggest that some textures may be robust to PET reconstruction parameters in identifying pathologic responders. However, this hypothesis needs to be further investigated.
Conclusion
The temporal changes in all textures, except Homogeneity, were better correlated to pathologic response and overall survival than the SUVmax and SUVmean. Computation of the PET-based textural features requires a resampling scheme with at least 128 discrete values and MTV threshold value no more than 40% SUVmax. The hypothesis that the temporal changes in PET-based textures can be used as clinical predictors of better patient outcomes will be tested in a larger patient dataset in the future.
Author Contributions
SY, NS, HM, HA, and RB conceived and designed the study. SY and TC performed the data analysis. NS and HM contributed data. HA and TC provide expert guidance and contributed analysis tools. SY and RB wrote the paper. TC, NS, HM, and HA reviewed the manuscript.
Conflict of Interest Statement
The authors declare that the research was conducted in the absence of any commercial or financial relationships that could be construed as a potential conflict of interest.
Funding
This work is in part supported by the Kaye scholar grant from the Brigham and Women’s Hospital. The authors would like to acknowledge the support from the National Institutes of Health (NIH-USA U01CA190234; NIH-USA U24CA194354).
References
1. Lordick F, Hölscher A, Haustermans K, Wittekind C. Multimodal treatment of esophageal cancer. Langenbecks Arch Surg (2013) 398:177–87. doi:10.1007/s00423-012-1001-1
2. Siegel R, Ma J, Zou Z, Jemal A. Cancer statistics, 2014. CA Cancer J Clin (2014) 64:9–29. doi:10.3322/caac.21208
3. Walsh TN, Noonan N, Hollywood D, Kelly A, Keeling N, Hennessy TPJ. A comparison of multimodal therapy and surgery for esophageal adenocarcinoma. N Engl J Med (1996) 335:462–7. doi:10.1056/NEJM199608153350702
4. Burmeister BH, Smithers BM, Gebski V, Fitzgerald L, Simes RJ, Devitt P, et al. Surgery alone versus chemoradiotherapy followed by surgery for resectable cancer of the oesophagus: a randomised controlled phase III trial. Lancet Oncol (2005) 6:659–68. doi:10.1016/S1470-2045(05)70288-6
5. Tepper J, Krasna MJ, Niedzwiecki D, Hollis D, Reed CE, Goldberg R, et al. Phase III trial of trimodality therapy with cisplatin, fluorouracil, radiotherapy, and surgery compared with surgery alone for esophageal cancer: CALGB 9781. J Clin Oncol (2008) 26:1086–92. doi:10.1200/JCO.2007.12.9593
6. Berger AC, Farma J, Scott WJ, Freedman G, Weiner L, Cheng JD, et al. Complete response to neoadjuvant chemoradiotherapy in esophageal carcinoma is associated with significantly improved survival. J Clin Oncol (2005) 23:4330–7. doi:10.1200/JCO.2005.05.017
7. Bosset JF, Gignoux M, Triboulet JP, Tiret E, Mantion G, Elias D, et al. Chemoradiotherapy followed by surgery compared with surgery alone in squamous-cell cancer of the esophagus. N Engl J Med (1997) 337:161–7. doi:10.1056/NEJM199707173370304
8. Urba SG, Orringer MB, Turrisi A, Iannettoni M, Forastiere A, Strawderman M. Randomized trial of preoperative chemoradiation versus surgery alone in patients with locoregional esophageal carcinoma. J Clin Oncol (2001) 19:305–13.
9. van Hagen P, Hulshof MC, van Lanschot JJ, Steyerberg EW, van Berge Henegouwen MI, Wijnhoven BP, et al. Preoperative chemoradiotherapy for esophageal or junctional cancer. N Engl J Med (2012) 366:2074–84. doi:10.1056/NEJMoa1112088
10. Schollaert P, Crott R, Bertrand C, D’Hondt L, Borght T, Krug B. A systematic review of the predictive value of 18FDG-PET in esophageal and esophagogastric junction cancer after neoadjuvant chemoradiation on the survival outcome stratification. J Gastrointest Surg (2014) 18:894–905. doi:10.1007/s11605-014-2488-2
11. Flamen P, Lerut A, Van Cutsem E, De Wever W, Peeters M, Stroobants S, et al. Utility of positron emission tomography for the staging of patients with potentially operable esophageal carcinoma. J Clin Oncol (2000) 18:3202–10.
12. Bruzzi JF, Munden RF, Truong MT, Marom EM, Sabloff BS, Gladish GW, et al. PET/CT of esophageal cancer: its role in clinical management. Radiographics (2007) 27:1635–52. doi:10.1148/rg.276065742
13. Weber WA. Use of PET for monitoring cancer therapy and for predicting outcome. J Nucl Med (2005) 46:983–95.
14. Wahl RL, Jacene H, Kasamon Y, Lodge MA. From RECIST to PERCIST: evolving considerations for PET response criteria in solid tumors. J Nucl Med (2009) 50:122S–50S. doi:10.2967/jnumed.108.057307
15. Vanderhoek M, Perlman SB, Jeraj R. Impact of different standardized uptake value measures on PET-based quantification of treatment response. J Nucl Med (2013) 54:1188–94. doi:10.2967/jnumed.112.113332
16. Abdelsalam M, Bazarbashi S, Abouzied M, Amin T, Soudy H, Rahal M, et al. Whole body 18F-FDG PET predicts progression free and overall survival in squamous cell carcinoma of the esophagus: results of a prospective trial. Hematol Oncol Stem Cell Ther (2010) 3:179–84. doi:10.5144/1658-3876.2010.179
17. Levine EA, Farmer MR, Clark P, Mishra G, Ho C, Geisinger KR, et al. Predictive value of 18-fluoro-deoxy-glucose-positron emission tomography ((18)F-FDG-PET) in the identification of responders to chemoradiation therapy for the treatment of locally advanced esophageal cancer. Ann Surg (2006) 243:472–8. doi:10.1097/01.sla.0000208430.07050.61
18. Flamen P, Van Cutsem E, Lerut A, Cambier JP, Haustermans K, Bormans G, et al. Positron emission tomography for assessment of the response to induction radiochemotherapy in locally advanced oesophageal cancer. Ann Oncol (2002) 13:361–8. doi:10.1093/annonc/mdf081
19. Port JL, Lee PC, Korst RJ, Liss Y, Meherally D, Christos P, et al. Positron emission tomographic scanning predicts survival after induction chemotherapy for esophageal carcinoma. Ann Thorac Surg (2007) 84:393–400. doi:10.1016/j.athoracsur.2007.03.094
20. Brücher BL, Weber W, Bauer M, Fink U, Avril N, Stein HJ, et al. Neoadjuvant therapy of esophageal squamous cell carcinoma: response evaluation by positron emission tomography. Ann Surg (2001) 233:300–9. doi:10.1097/00000658-200103000-00002
21. Weber WA, Ott K, Becker K, Dittler HJ, Helmberger H, Avril NE, et al. Prediction of response to preoperative chemotherapy in adenocarcinomas of the esophagogastric junction by metabolic imaging. J Clin Oncol (2001) 19:3058–65.
22. Tan S, Kligerman S, Chen W, Lu M, Kim G, Feigenberg S, et al. Spatial-temporal [18F]FDG-PET features for predicting pathologic response of esophageal cancer to neoadjuvant chemoradiation therapy. Int J Radiat Oncol Biol Phys (2013) 85:1375–82. doi:10.1016/j.ijrobp.2012.10.017
23. Sun W, Xu J, Hu W, Zhang Z, Shen W. The role of sequential 18F-FDG PET/CT in predicting tumour response after preoperative chemoradiation for rectal cancer. Colorectal Dis (2013) 15:e231–8. doi:10.1111/codi.12165
24. Henriksson E, Kjellen E, Wahlberg P, Ohlsson T, Wennerberg J, Brun E. 2-deoxy-2-[18F]fluoro-D-glucose uptake and correlation to intratumoral heterogeneity. Anticancer Res (2007) 27:2155–9.
25. Cook GR, Siddique M, Taylor B, Yip C, Chicklore S, Goh V. Radiomics in PET: principles and applications. Clin Transl Imaging (2014) 2:269–76. doi:10.1007/s40336-014-0064-0
26. Chicklore S, Goh V, Siddique M, Roy A, Marsden P, Cook GR. Quantifying tumour heterogeneity in 18F-FDG PET/CT imaging by texture analysis. Eur J Nucl Med Mol Imaging (2013) 40:133–40. doi:10.1007/s00259-012-2247-0
28. Burrell RA, McGranahan N, Bartek J, Swanton C. The causes and consequences of genetic heterogeneity in cancer evolution. Nature (2013) 501:338–45. doi:10.1038/nature12625
29. Marusyk A, Almendro V, Polyak K. Intra-tumour heterogeneity: a looking glass for cancer? Nat Rev Cancer (2012) 12:323–34. doi:10.1038/nrc3261
30. Hatt M, Tixier F, Cheze Le Rest C, Pradier O, Visvikis D. Robustness of intratumour 18F-FDG PET uptake heterogeneity quantification for therapy response prediction in oesophageal carcinoma. Eur J Nucl Med Mol Imaging (2013) 40:1662–71. doi:10.1007/s00259-013-2486-8
31. Tixier F, Le Rest CC, Hatt M, Albarghach N, Pradier O, Metges JP, et al. Intratumor heterogeneity characterized by textural features on baseline 18F-FDG PET images predicts response to concomitant radiochemotherapy in esophageal cancer. J Nucl Med (2011) 52:369–78. doi:10.2967/jnumed.110.082404
32. El Naqa I, Grigsby PW, Apte A, Kidd E, Donnelly E, Khullar D, et al. Exploring feature-based approaches in PET images for predicting cancer treatment outcomes. Pattern Recognit (2009) 42:1162–71. doi:10.1016/j.patcog.2008.08.011
33. Lambin P, Rios-Velazquez E, Leijenaar R, Carvalho S, van Stiphout RG, Granton P, et al. Radiomics: extracting more information from medical images using advanced feature analysis. Eur J Cancer (2012) 48:441–6. doi:10.1016/j.ejca.2011.11.036
34. Haralick RM, Shanmugam K, Dinstein IH. Textural features for image classification. IEEE Trans Syst Man Cybern (1973) SMC-3:610–21. doi:10.1109/TSMC.1973.4309314
35. Rahim M, Kim S, So H, Kim HJ, Cheon GJ, Lee ES, et al. Recent trends in PET image interpretations using volumetric and texture-based quantification methods in nuclear oncology. Nucl Med Mol Imaging (2014) 48:1–15. doi:10.1007/s13139-013-0260-2
36. Zhang H, Tan S, Chen W, Kligerman S, Kim G, D’Souza WD, et al. Modeling pathologic response of esophageal cancer to chemoradiation therapy using spatial-temporal 18F-FDG PET features, clinical parameters, and demographics. Int J Radiat Oncol Biol Phys (2014) 88:195–203. doi:10.1016/j.ijrobp.2013.09.037
37. Galloway MM. Texture analysis using gray level run lengths. Comput Graphics Image Process (1975) 4:172–9. doi:10.1016/S0146-664X(75)80008-6
38. Thibault G, Fertil B, Navarro C, Pereira S, Cau P, Levy N, et al. Shape and texture indexes application to cell nuclei classification. Intern J Pattern Recognit Artif Intell (2013) 27:1357002. doi:10.1142/S0218001413570024
39. Yang F, Thomas M, Dehdashti F, Grigsby P. Temporal analysis of intratumoral metabolic heterogeneity characterized by textural features in cervical cancer. Eur J Nucl Med Mol Imaging (2013) 40:716–27. doi:10.1007/s00259-012-2332-4
40. Aerts HJWL, Velazquez ER, Leijenaar RTH, Parmar C, Grossmann P, Carvalho S, et al. Decoding tumour phenotype by noninvasive imaging using a quantitative radiomics approach. Nat Commun (2014) 5:4006. doi:10.1038/ncomms5006
41. Leijenaar RTH, Carvalho S, Velazquez ER, van Elmpt WJ, Parmar C, Hoekstra OS, et al. Stability of FDG-PET radiomics features: an integrated analysis of test-retest and inter-observer variability. Acta Oncol (2013) 52:1391–7. doi:10.3109/0284186X.2013.812798
43. Yang D, Li H, Low DA, Deasy JO, Naqa IE. A fast inverse consistent deformable image registration method based on symmetric optical flow computation. Phys Med Biol (2008) 53:6143. doi:10.1088/0031-9155/53/21/017
44. Yip SSF, Coroller TP, Sanford NN, Huynh E, Mamon H, Aerts HJWL, et al. Use of registration-based contour propagation in texture analysis for esophageal cancer pathologic response prediction. Phys Med Biol (2016) 61:906–22. doi:10.1088/0031-9155/61/2/906
45. Aristophanous M, Yong Y, Yap JT, Killoran JH, Allen AM, Berbeco RI, et al. Evaluating FDG uptake changes between pre and post therapy respiratory gated PET scans. Radiother Oncol (2012) 102:377–82. doi:10.1016/j.radonc.2011.12.015
46. Yip S, McCall K, Aristophanous M, Chen AB, Aerts HJWL, Berbeco R. Comparison of texture features derived from static and respiratory-gated PET images in non-small cell lung cancer. PLoS One (2014) 9:e115510. doi:10.1371/journal.pone.0115510
47. Cheng N-M, Dean Fang Y-H, Tung-Chieh Chang J, Huang CG, Tsan DL, Ng SH, et al. Textural features of pretreatment 18F-FDG PET/CT images: prognostic significance in patients with advanced T-stage oropharyngeal squamous cell carcinoma. J Nucl Med (2013) 54:1703–9. doi:10.2967/jnumed.112.119289
48. Fang Y-HD, Lin C-Y, Shih M-J, Wang HM, Ho TY, Liao CT, et al. Development and evaluation of an open-source software package CGITA; for quantifying tumor heterogeneity with molecular images. Biomed Res Int (2014) 2014:9. doi:10.1155/2014/248505
49. Gentleman RC, Carey VJ, Bates DM, Bolstad B, Dettling M, Dudoit S, et al. Bioconductor: open software development for computational biology and bioinformatics. Genome Biol (2004) 5:R80–80. doi:10.1186/gb-2004-5-10-r80
50. Schröder MS, Culhane AC, Quackenbush J, Haibe-Kains B. survcomp: an R/Bioconductor package for performance assessment and comparison of survival models. Bioinformatics (2011) 27:3206–8. doi:10.1093/bioinformatics/btr511
51. Pencina MJ, D’Agostino RB. Overall C as a measure of discrimination in survival analysis: model specific population value and confidence interval estimation. Stat Med (2004) 23:2109–23. doi:10.1002/sim.1802
52. Harrell FE. Regression Modeling Strategies: With Applications to Linear Models, Logistic Regression, and Survival Analysis. New York: Springer (2001).
53. Hanley JA, McNeil BJ. A method of comparing the areas under receiver operating characteristic curves derived from the same cases. Radiology (1983) 148:839–43. doi:10.1148/radiology.148.3.6878708
54. Harrell FE, Lee KL, Mark DB. Multivariable prognostic models: issues in developing models, evaluating assumptions and adequacy, and measuring and reducing errors. Stat Med (1996) 15:361–87. doi:10.1002/(SICI)1097-0258(19960229)15:4<361::AID-SIM168>3.0.CO;2-4
55. Bamber D. The area above the ordinal dominance graph and the area below the receiver operating characteristic graph. J Math Psychol (1975) 12:387–415. doi:10.1016/0022-2496(75)90001-2
57. Song SY, Kim JH, Ryu JS, Lee GH, Kim SB, Park SI, et al. FDG-PET in the prediction of pathologic response after neoadjuvant chemoradiotherapy in locally advanced, resectable esophageal cancer. Int J Radiat Oncol Biol Phys (2005) 63:1053–9. doi:10.1016/j.ijrobp.2005.03.033
58. Brink I, Hentschel M, Bley TA, Walch A, Mix M, Kleimaier M, et al. Effects of neoadjuvant radio-chemotherapy on 18F-FDG-PET in esophageal carcinoma. Eur J Surg Oncol (2004) 30:544–50. doi:10.1016/j.ejso.2004.03.007
59. Malik V, Lucey JA, Duffy GJ, Wilson L, McNamara L, Keogan M, et al. Early repeated 18F-FDG PET scans during neoadjuvant chemoradiation fail to predict histopathologic response or survival benefit in adenocarcinoma of the esophagus. J Nucl Med (2010) 51:1863–9. doi:10.2967/jnumed.110.079566
60. Gillies RS, Middleton MR, Blesing C, Patel K, Warner N, Marshall RE, et al. Metabolic response at repeat PET/CT predicts pathological response to neoadjuvant chemotherapy in oesophageal cancer. Eur Radiol (2012) 22:2035–43. doi:10.1007/s00330-012-2459-5
61. Smithers BM, Couper GC, Thomas JM, Wong D, Gotley DC, Martin I, et al. Positron emission tomography and pathological evidence of response to neoadjuvant therapy in adenocarcinoma of the esophagus. Dis Esophagus (2008) 21:151–8. doi:10.1111/j.1442-2050.2007.00732.x
62. Orlhac F, Soussan M, Maisonobe J-A, Garcia CA, Vanderlinden B, Buvat I. Tumor texture analysis in 18F-FDG PET: relationships between texture parameters, histogram indices, standardized uptake values, metabolic volumes, and total lesion glycolysis. J Nucl Med (2014) 55:414–22. doi:10.2967/jnumed.113.129858
63. Hatt M, Majdoub M, Vallières M, Tixier F, Le Rest CC, Groheux D, et al. 18F-FDG PET uptake characterization through texture analysis: investigating the complementary nature of heterogeneity and functional tumor volume in a multi-cancer site patient cohort. J Nucl Med (2015) 56:38–44. doi:10.2967/jnumed.114.144055
Keywords: pathologic response, PET imaging, texture analysis, esophageal cancer, neoadjuvant therapy
Citation: Yip SSF, Coroller TP, Sanford NN, Mamon H, Aerts HJWL and Berbeco RI (2016) Relationship between the Temporal Changes in Positron-Emission-Tomography-Imaging-Based Textural Features and Pathologic Response and Survival in Esophageal Cancer Patients. Front. Oncol. 6:72. doi: 10.3389/fonc.2016.00072
Received: 10 December 2015; Accepted: 14 March 2016;
Published: 29 March 2016
Edited by:
Issam El Naqa, McGill University, CanadaReviewed by:
Andre Konski, University of Pennsylvania, USASunyoung Jang, Princeton Radiation Oncology, USA
Copyright: © 2016 Yip, Coroller, Sanford, Mamon, Aerts and Berbeco. This is an open-access article distributed under the terms of the Creative Commons Attribution License (CC BY). The use, distribution or reproduction in other forums is permitted, provided the original author(s) or licensor are credited and that the original publication in this journal is cited, in accordance with accepted academic practice. No use, distribution or reproduction is permitted which does not comply with these terms.
*Correspondence: Stephen S. F. Yip, c3lpcCYjeDAwMDQwO2xyb2MuaGFydmFyZC5lZHU=