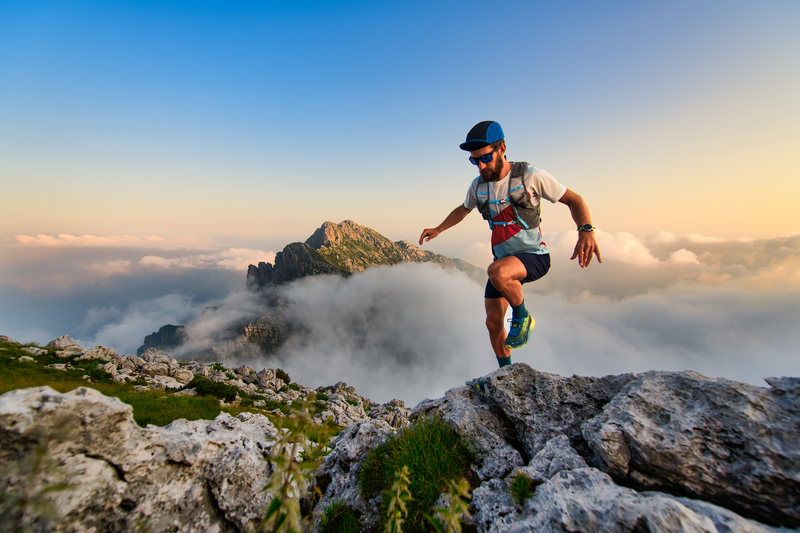
95% of researchers rate our articles as excellent or good
Learn more about the work of our research integrity team to safeguard the quality of each article we publish.
Find out more
ORIGINAL RESEARCH article
Front. Oncol. , 17 December 2012
Sec. Pediatric Oncology
Volume 2 - 2012 | https://doi.org/10.3389/fonc.2012.00194
Technological advances now allow us to rapidly produce CARs and other antibody-derived therapeutics targeting cell surface receptors. To maximize the potential of these new technologies, relevant extracellular targets must be identified. The Pediatric Oncology Branch of the NCI curates a freely accessible database of gene expression data for both pediatric cancers and normal tissues, through which we have defined discrete sets of over-expressed transcripts in 12 pediatric cancer subtypes as compared to normal tissues. We coupled gene expression profiles to current annotation databases (i.e., Affymetrix, Gene Ontology, Entrez Gene), in order to categorize transcripts by their sub-cellular location. In this manner we generated a list of potential immune targets expressed on the cell surface, ranked by their difference from normal tissue. Global differences from normal between each of the pediatric tumor types studied varied, indicating that some malignancies expressed transcript sets that were more highly diverged from normal tissues than others. The validity of our approach is seen by our findings for pre-B cell ALL, where targets currently in clinical trials were top-ranked hits (CD19, CD22). For some cancers, reagents already in development could potentially be applied to a new disease class, as exemplified by CD30 expression on sarcomas. Moreover, several potential new targets shared among several pediatric solid tumors are herein identified, such as MCAM (MUC18), metadherin (MTDH), and glypican-2 (GPC2). These targets have been identified at the mRNA level and are yet to be validated at the protein level. The safety of targeting these antigens has yet to be demonstrated and therefore the identified transcripts should be considered preliminary candidates for new CAR and therapeutic antibody targets. Prospective candidate targets will be evaluated by proteomic analysis including Westerns and immunohistochemistry of normal and tumor tissues.
“Tumor-associated antigens” are multi-faceted and can be defined as any entity that the immune system can avail itself of to protect the host from disease. Virus-encoded tumor antigens are currently being targeted by preventive vaccines, such as for papilloma viruses, or by adoptive immunotherapy, as in EBV-associated post-transplant lymphoma. Self-antigens presented on major histocompatibility antigens (MHC) molecules are also key to clearing disease by donor-derived cells in the context of bone-marrow or hematopoietic stem cell transplantation (HSCT; Miller et al., 2010). However, with the advent of antibody-based therapies, cell-surface antigens on tumor cells can be targeted without first requiring processing and presentation by the MHC. Thus, a tumor antigen may be a unique molecule expressed by a tumor that is not encoded by the healthy genome, a non-mutated developmental antigen now re-expressed on a tumor cell, or a self-antigen than can be safely targeted without loss of host integrity. One example of recent interest is the re-expression of the developmentally regulated ALK protein on neuroblastoma (Mosse et al., 2008). The work we present here indicates that there are other such targets that remain to be discovered.
Our goal was to develop a method for identifying tumor antigen candidates that could be targeted by antibody or CAR-based therapies by leveraging publically available microarray gene expression databases of pediatric cancer. Previously we explored a series of established xenograft cell lines from pediatric cancers in support of the Pediatric Oncology Pre-clinical Protein-Tissue Array Project (POPP-TAP), a project jointly supported by the Children’s Oncology Group and the NCI (Whiteford et al., 2007). In analyzing these xenograft models we also began to assemble comparator normal tissue databases1 (“Pediatric Xenograft & Tumor Gene Expression Database”). This earlier study was carried out using a gene expression array from Research Genetics (Huntsville, AL, USA). These studies were expanded to include normal tissue analysis on the commonly available Affymetrix platform (“Pediatric Tumor Affymetrix Database” at see text footnote 1), allowing for easier comparison to analyses from other groups (Chen et al., 2008). Averaging gene expression levels from individual tumor samples according to diagnostic category, and comparing this expression level transcript by transcript to average normal tissue expression levels, allowed a statistical measure of the difference of that transcripts expression from its expression in normal tissue. Ranking the identified transcripts, and then filtering them for plasma membrane expression is a first step in the high-throughput identification of all available targets on the surface of pediatric cancers. We propose that this approach will be especially valuable in solid tumors, as a paucity of well-described targets remains a challenge to the field. The data we present here should allow for the rapid assessment of these target antigens for their suitability as candidates for immunotherapy.
The Pediatric Tumor Affymetrix Database is freely accessible and can be found on-line at the NCI Pediatric Oncology Branch Oncogenomics Section web-site2. The gene chip used was U133 P2 (Affymetrix, Santa Clara, CA, USA).
The design of this program was broken down into stages. First, annotation data from several public databases were collated and used to identify cell surface proteins. This included Gene Ontology3, Affymetrix chip data references, the Human Protein Reference Database4, and the primary literature. Second, a database was constructed using this data, along with our gene expression data, to store the data in a usable format for analysis in MySQL, using a set of Python scripts to automate core database functions. Using this database, an auxiliary table was built, combining gene expression levels over tissue sample categories, corresponding specifically to samples from indicated pediatric cancers, using a two-sampled t-test (implemented using the SciPy Python package for scientific and statistical functions) with all samples in each category tested against a series of normal tissue samples (expression profiles of lung, liver, kidney, heart, adrenal, cerebrum, cerebellum, uterus, testes, stomach, spleen, bladder, skeletal muscle, prostate, and ovary, previously described in; Whiteford et al., 2007) in order to generate a T-statistic and p value, scoring each gene’s expression level in each cancer type vs. normal expression levels. Genes in this auxiliary table were then sorted in order of descending differential expression.
We restricted our current analysis to the 12 pediatric tumor types that had more than five samples available in the Pediatric Tumor Affymetrix Database: Pre-B Acute Lymphocytic Leukemia (Pre_B_ALL), Embryonal Rhabdomyosarcoma (ERMS), Alveolar Rhabdomyosarcoma (ARMS), Soft-Tissue Sarcoma (STS) that is not classified as Rhabdomyosarcoma (Non-RMS_STS or simply STS), Desmoplastic Small Round Cell Tumor (DSRCT), Ewing’s Sarcoma (EWS), Alveolar Soft Part Sarcoma (ASPS), Glioblastoma (GBM), Osteosarcoma (OS), Neuroblastoma-MYCN-amplified (NBL_MA, MYCNA-NBL), Neuroblastoma non-MYCN-amplified (NBL), and Hepatoblastoma (HBL). Some well-known tumors, like Wilm’s tumor, could not yet be included; nevertheless, these 12 types represent the majority of all pediatric solid tumors, and also includes the most common hematologic malignancy of children.
We present here Pre_B_ALL as an example to demonstrate how data mining searches were organized. A standard t-test was used to compare the average gene expression signal from tumor vs. the set of normal tissues analyzed in the database. The normal tissue data was used as an aggregate average expression score per each query. The algorithm was also set to report out a p value, while filtering for surface membrane expression to define the targets of interest. We initially calculated t-test values > 10, and ordered the output to select for the highest T values. This process was repeated in a similar manner for each disease category. Table 1 shows the number of hits for each disease type in the database returned when this arbitrary T threshold of >10 was selected. A wide range of hits was returned, with some diseases like ARMS having 62 hits score above 10, while DSRCT had 0. This does not mean DSRCT has no significant hits, as a T-statistic of 10 is a very high-value. Rather it illustrates that on a global level each malignancy has developed its own phenotypic “distance” from the normal cell surface landscape.
A major challenge for our approach is that annotation of membrane-associated protein expression has not (or perhaps currently cannot) been validated for accuracy. On-line programs such as the highly sophisticated TMHMM package5 can predict transmembrane structure, but cannot assign sub-cellular localization. The most extensive and accurate protein database groups (for example the Human Protein Reference Database/Pandey Lab6) are hand-annotating proteins and tracing them to the original literature in order to define sub-cellular localization. Therefore, we also had to utilize this approach and individually examine each membrane protein hit yielded by our algorithm by searching the available primary literature, primarily using Gene hosted by NCBI7, to determine if the “membrane” tag associated with a transcript’s annotation truly denotes the extracellular plasma membrane. If an antigen is not expressed at the surface, that antigen will not be useful for immune targeting as we have described. We thus excluded proteins restricted to the mitochondria, nuclear membrane, Golgi, endoplasmic reticulum, sorting vesicles, and other intracellular membrane-bound bodies. Membrane proteins expressed both on the surface and another sub-cellular compartment were included. Table 2 lists the top 25 extracellular membrane proteins for each disease type after individual annotation/inspection.
To compare global differences in the immune landscape for the 25 antigens most different from normal for each tumor type, we plotted the T value range of those 25 hits for each tumor type, Figure 1. When comparing the expression of a particular transcript in a tumor type versus normal tissue, we used a T-statistic and report the associated p value for that particular transcript (both with respect to difference from normal tissue). In looking at the top 25 hits for each tumor type, the lowest set of T values (that is membrane proteins that were least distinct from normal), were DSRCT and NBL. T values ranged from 9.3 to 6.9 for DSRCT and from 12.6 to 5.8 for NBL. The highest T values (tissues scoring the most different from normal) were seen for ASPS, Pre-B ALL, STS, and ARMS, which scored from 25.5 to 12.5, 19.8 to 11.0, 15.0 to 9.8, and 27.7 to 10.0, respectively. When p values were evaluated an essentially inverse pattern was seen; that is, high scoring T values had smaller (more significant) p values (not shown). These values demonstrate very good separation from normal and represent a set of targets that are important to further evaluate in each of these tumor types. As to the true immunogenicity of these tumor types, further studies are required to determine whether these differences can be accounted for by different strategies of immune escape or immune editing (Schreiber et al., 2011).
Figure 1. T-Statistic overview of the top 25 tumor-specific transcripts. For each disease category (listed on the x-axis), the range of T-statistic values for the 25 membrane proteins most different from normal tissue, along with their average and quartiles, is represented as a box and whisker plot.
To better understand the antigens we have identified, we ordered the transcripts according to functional groups (Table 3). The first group consists of known CD antigens or immune marker proteins. The possession of a CD designation ensures that an antibody has been created to that transcript. The only known CD antigen we did not list in this section was CD222 (glucagon-like peptide 1 R), which was placed in the growth factor receptor category. In the CD antigen group, 12 out of 47 (appx. 25%) are expressed in pre-B ALL. This is representative of the tissue of origin, as CD antigens are often of immune cell origin. Nevertheless, many CD antigens are also expressed on other pediatric tumors.
Antigens in the “Cell Adhesion, Cell Junction” group include well-known adhesion family receptor members, like cadherins, cell-to-cell junction associated proteins, and other scavenger or immunoglobulin-related proteins. One would expect that the proteins in this family also would readily have antibodies created to them, and are likely to receive CD designations in the future. This is also likely to be true for the “Growth Factor Receptor/Development Receptor” grouping. These hits also provide ready targets such as the FGFR2, FGFR4, known oncogenes like MET, and the activin and ephrin receptors, that are likely to be linked to the oncogenic activation of the cancer tissues in which they are expressed. The final groups of cell surface proteins that are likely to be targetable are the “Migration/Metastasis/Motility” and the “Enzymatic Function” group. In general these proteins exhibit their activity outside of the cell and mediate interaction of the cell with its surrounding matrix.
Comprising about half the proteins on the list are membrane-expressed proteins to which it cannot be assumed an antibody or CAR exists or that could be readily generated. These groups are the Neurotransmitter Receptors, Ion Channels, Solute Carrier Family, Tetraspanin, and G-protein groups. Although exceptions certainly exist, these membrane proteins interact with small molecules or ions and transport them across the cell membrane, or they co-ordinate other proteins within the membrane. In general they do not evidence a large extracellular component.
Another way to ascribe relative value to the “hits” we have identified is to determine how broadly they are expressed across the different pediatric cancer types. Figure 2 demonstrates that 12 proteins are expressed in at least three distinct disease categories, and that another 12 transcripts are expressed in more than three disease types. From this view, the highest value targets are MCAM and GPC2, which are expressed in the Top 25 for eight different cancers. Similarly, small molecule transporters like SLC19A1 and neurotransmitter receptors like purogenic G-protein coupled (P2RY11) are prevalent across histologies. Whether the limited size of their extracellular domains will affect the ability to target these antigens with antibody derivatives or CARs is unknown, but their prevalence across disease types makes this an interesting question.
Figure 2. Frequency of hits across disease category. Transcripts appearing in the pediatric Top 25 were often shared between disease types. All of the transcripts expressed in more than three (A), or at least three (B), cancer types are listed. The x-axis lists the individual gene, and the stacked boxes represent presence of that transcript in the Top 25 for that disease type.
The ability of our algorithm to identify high-value targets is demonstrated by our results for Pre-B ALL, where a number of cell surface molecules currently being targeted in the clinic were identified as hits, Table 2. One of the most important immunotherapeutic trials to date features CAR-modified T-cells specific for CD19, which is the top hit for B_ALL (Kochenderfer et al., 2010; Porter et al., 2011). Another Top 25 hit, CD22, is the target of an antibody-toxin conjugate experimental protocol that continues to show promise for children with ALL (Mussai et al., 2010). Another target, CD79 is also the subject of interest in therapy for B cell malignancy, as antibody-drug conjugates are being developed and tested (Polson et al., 2007). While our bioinformatic approach is validated by these results with ALL, the value of our approach is in the identification of antigens of interest for pediatric solid tumors for which substantial numbers of candidate antigens have not been previously identified.
The two most broadly expressed targets we identified for pediatric solid tumors are melanoma cell adhesion molecule (MCAM, CD146, MUC18), and glypican-2 (GPC2). MCAM and GPC2 are expressed in eight of the 12 tumor types analyzed, Figure 2. The other most commonly expressed adhesion molecule, metadherin (MTDH), is expressed in four of 12 tumor types. MCAM is involved in the pathogenesis of melanoma, breast carcinoma, and other cancers (Wu et al., 2011; Zeng et al., 2012). Both antibody-based and vaccine approaches targeting MCAM/MUC18 have been proposed (Melnikova and Bar-Eli, 2006; Leslie et al., 2007). GPC2 (also know as cerebroglycan) is normally expressed in the developing brain (Stipp et al., 1994). Molecules in the same family, Glypican-3 and Glypican-5, have been described in melanoma, neuroblastoma, and rhabdomyosarcoma (Saikali and Sinnett, 2000; Nakatsura et al., 2004a,b; Williamson et al., 2007). Our report here of the specific expression of Glypican-2 on pediatric tumors should now focus attention on this glypican as well. Metadherin (MTDH) is expressed on hepatocellular carcinoma, may play a role in epithelial-to-mesenchymal transition (Zhu et al., 2011), and plays a role in the metastatic spread of breast cancer to the lung (Brown and Ruoslahti, 2004). These three adhesion receptors are therefore top candidates to explore further as targets in pediatric tumors given their overexpression and the ability to create antibodies against cell surface adhesion receptors.
The largest family of cell surface proteins is the G-proteins (GPCR, approximately 800 members), followed by the solute carrier family (SLC, approximately 380 members; Almen et al., 2009; Hoglund et al., 2011). One of our top hits, SLC19A1 (expressed in ALL, HBL, MYCNA-NBL, GBM, ERMS, and ARMS), is a folate transporter. Unlike the GPI-linked α folate receptor (FOLR1), which is currently the target of antibody-based trials, SLC19A1 (FOLT) is a multi-pass membrane protein that looks very much like an ion channel (Konner et al., 2010). Each of the SLC proteins are regulated in expression, responsive to cellular stress or nutritional requirement, and could serve as suitable immune targets, but little is known about targeting them. The better known GPCR, ion channels, and neurotransmitter receptors we have described have in some cases had antibodies produced against them. In general, producing antibodies, and therefore scFv and CARs, against multi-membrane pass proteins with limited extracellular sequence is more difficult than for proteins with large extracellular domains.
The solid pediatric malignancies we analyzed also preferentially overexpress a number of receptors associated with the activin and ephrin receptor systems. Hits in the activin family include ACVR2A (ERMS, ARMS), ACVR2B (HBL); and in the ephrin family, EPHB2 (DRCT, HBL), EPHB3 (DRCT), EPHB4 (ERMS, DRCT), and EFNB1 (EWS). Activins are growth factors in the TGF-β family that bind dual chain receptors composed of ligand-binding and signaling subunits. The ACVR2 chains have the ability to signal as constitutive kinases and are thought to regulate muscle growth through binding of myostatin (Lee et al., 2005). The ACVR2A/2B expression patterns and their biological activity make them very high-value hits for pediatric oncology. Ephrins function in neuronal development and are divided in to A and B types, as are the receptors that bind to them. The ligand, ephrin-B1, is a transmembrane protein and the receptors we list as Top 25 hits, all in the receptor tyrosine kinase family, are also of the B type. Aberrant expression of these family members has been described in colon carcinoma (Herath et al., 2012). EPHB4 plays a role in vascular development and may participate in tumor metastatic spread (Heroult et al., 2010). Antibodies to EphB4 have been described to inhibit tumor growth (Krasnoperov et al., 2010). Both activin and ephrin receptors are promising for further investigation as cell surface targets in pediatric solid tumors.
Fibroblast growth factor receptors (FGFRs) are of great interest in pediatric oncology, and our bioinformatic hit for FGFR4 in ARMS confirms our previous genomic studies. There are 4 FGFRs that mediate cellular activation by the more than 20 known fibroblast growth factors (Olsen et al., 2003). Alterations or mutation of the FGFRs have been described in a number of cancer types, and they have been proposed as targets for both kinase inhibitor chemotherapy as well as immunotherapy (Wesche et al., 2011). Our group had previously identified FGFR4 as a target in rhabdomyosarcoma using gene expression array analysis and we have demonstrated FGFR4 activity in disease (Taylor et al., 2009). The closely related FGFR2 was also identified as a hit in STS and GBM. FGFR2 has been associated with a number of cancers making both of these FGFRs exciting findings for pediatric oncology (Bai et al., 2010).
The most intriguing hit for Non-Rhabdomyosarcoma STS is CD30 (TNFRSF8). CD30 is the current target of a number of immunotherapy trials, and our report suggests that these reagents should be evaluated for use in STS (Kasamon and Ambinder, 2008). In DSRCT we find expression of two very exciting hits, poliovirus receptor (PVR)/CD115 and PVR-related 2 (PVRL2)/CD112. Both these adhesion molecules have been described as NK-ligands and should serve as suitable immune targets (Pende et al., 2005). An equally exciting target on DSRCT is PDCD1 (PD1/CD279). The antigen is well-described as an anti-immune effector and efforts to block or target PD1 in cancer are actively underway (Flies et al., 2011).
The immune markers expressed EWS represent a unique group of targets. XG, is part of the Xg blood group antigen, the only other member of which is CD99, a well-known over-expressed protein in EWS, and expression of these antigens is closely linked (Johnson, 2011). Although transcripts are known to be found in non-erythroid tissues not much else is known for this target (Fouchet et al., 2000). Meynet et al. (2010), have also recently described XG as a marker for EWS and demonstrate that its expression is associated with poor outcome. Three cytokine receptors are expressed on EWS: IL17RE, IL27RA, and IL1RAP. A recent mutational study demonstrated that IL27RA has transforming potential, although its expression on non-lymphoid malignancies has not previously been described (Lambert et al., 2011). IL1RAP, Interleukin-1 receptor accessory protein, can exist as a membrane or soluble form and is essential for IL-1 receptor activity. Expression of IL1RAP has been described in endometriosis and various leukemias, notably in leukemia stem cells, making IL1RAP a high-value target for EWS (Jaras et al., 2010; Guay et al., 2011).
Glioblastoma expresses the immune markers in CD73/NT5E (5′ nucleotidase, ecto), and CKLF. CD73 is present on cancer exosomes and has been shown to blunt immune responses (Clayton et al., 2011). CD73 is also a hit for HBL. CKLF, chemokine-like factor 1, binds CCR4 and can be either secreted or membrane-bound and contains a MARVEL transmembrane domain (Chowdhury et al., 2008). MARVELD2 is a transmembrane protein associated with tight junction that serves as a hit for HBL. The wide distribution of these targets requires more developmental work before considering using them as immune targets. GALR2, galanin receptor 2, is a growth factor receptor that also is an interesting GBM target. GALR2 has been proposed as a therapeutic target in head and neck carcinoma and is an active area of study (Kanazawa et al., 2010). GAL2R targeting should be explored in GBM as well.
Osteosarcoma expresses the intriguing hit UL16 binding protein 2 (ULBP2), which is a ligand for the NK cell activation marker NKGD2. To date, ULBP2 has been thought of as a means to alert to immune system due to p53 activation on target cells, and not as a mean of cancer immune escape (Textor et al., 2011). The matrix metalloproteinase MMP14 is known to participate in tumor metastasis and is the subject of intense research activity, making this a high-value target in OS and ASPS (Zarrabi et al., 2011). VASN, vasorin, is a transforming growth factor beta (TGF-β) trap, its biology is regulated by ADAM17, and it appears to play a role in epithelial to mesenchymal transition in other cancer types (Malapeira et al., 2011). Both of these two OS surface targets may play a role in disease progression.
In the analysis of MYCN-Amplified Neuroblastoma (NBL-MYCNA) our algorithm identified one of the most interesting current targets, CD276 (B7-H3). CD276 is the target of a number of immune directed therapies in NBL (Castriconi et al., 2004). The other targets identified for NML-MYCNA and for NB are all of great interest, but CD276 is likely to be a focus of future immune based strategies.
Hepatoblastoma overexpresses ASGR1 asialoglycoprotein receptor 1, and ASGR2. ASGR2 serves as a receptor for a series of glycoproteins including those on the surface of hepatitis virus (Yang et al., 2010). The only report of association with cancer is the reported increase in the rate of cell division of colorectal carcinoma cell lines when grown on ASGR1 coated surfaces, which makes both ASGRs intriguing hits (Fang et al., 2009). Another potential target for HBL is MR1, MHC class I-related, which is also expressed in ASPS. MR1 is an invariant class I MHC molecule known to interact with a subset of T lymphocytes with invariant or restricted TCRs (Gozalbo-Lopez et al., 2009). The expression of MR1 in cancer has not been reported, making this a novel finding. CD301 (CLEC10A, C-type lectin domain family 10, member A) has not been reported in cancer either, but like any adhesion receptor it has the potential to mediate metastasis. Another adhesion receptor hit is CD353, SLAMF8 [signal lymphocyte activation molecule (SLAM), family member 8]. SLAM proteins are in the CD2 family of lymphocyte activation proteins and may also contribute to the activation of cancer cells (Furukawa et al., 2010).
ASPS is a very distinct entity as evidenced by the unique set of antigens we report as top 25 hits, many of which are not shared with other tumors. EPOR, the erythropoietin receptor, was the highest-ranking hit for ASPS. The ETV6-RUNX1 fusion in ALL activates transcription of EPOR and it is likely to contribute a growth signal to leukemia, making this a potential target of interest (Torrano et al., 2011). Two CD antigens that were strong hits for ASPS are also growth factor receptors. Colony-stimulating factor 1/CD115 (CSF1R) modulates a number of myeloid differentiation steps and inhibitors have been designed for a number of disease states (Hume and Macdonald, 2012). CD222/ insulin-like growth factor-2 (IGF2R) has long been recognized as a cancer-expressed protein (Martin-Kleiner and Gall Troselj, 2010). Each of these receptors should be explored as targets in ASPS. Also intriguing is the expression of HLA-G. HLA-G is a class I MHC paralog, normally expressed on placental cells, and its expression has been described on malignant cells, perhaps shielding them from immunosurveillance (Yan, 2011). CD204/macrophage scavenger receptor 1 (MSR1) is another immune molecule expressed on ASPS. Expression of CD204 on tumor stromal macrophages has been associated with aggressiveness in lung cancer (Ohtaki et al., 2010). Thus ASPS provides a number of CD or CD-like antigens that can be targeted by means of immunotherapy.
How a cell surface antigen expressed on a tumor becomes a locus of tumor-protective immune activation, and how this response spares healthy tissue has yet to be fully defined. An antigen may be “revealed” by inhibiting immune checkpoints, or newly recognized as an antigenic target by vaccination (such as with PMSA; May et al., 2011). The key for both approaches is that a response has been induced that now sufficiently differentiates between cancer and normal host tissue. What “sufficient differentiation” is, remains a term in need of clarification. For example, some self-antigens, such as CD20, can readily be targeted, while others, such as HER2, are highly dependent on how they are targeted. The mature B cells compartment is apparently dispensable, as use of anti-CD20 antibody in lymphoma therapy has demonstrated. Once the B cell compartment is no longer targeted by the therapy, the B cell population is then replenished. HER2 (Neu, CD340, ErbB-2) has been effectively targeted in thousands of women with breast cancer using anti-HER2 antibody. However, administration of a T-cell population expressing a HER2-specific CAR resulted in treatment related mortality. In this special instance a large number of anti-HER-2-CAR engineered T-cells presumably bound to the low levels of HER2 present in the lung, and a massive cytokine storm ensued leading to death (Morgan et al., 2010). This event clearly shows that “how” a tumor antigen is targeted by the immune system may be as important as the expression level of that antigen on various tissues. It also illustrates that we have yet to create a universal definition of whether or not it is safe to target a tumor antigen expressed at very low levels on normal tissue. Targeting B cell malignancies has worked so well because the mature B cell compartment that expresses CD20 and CD19 is expendable (Biagi et al., 2007). A database that would begin to define what an “expendable” normal tissue is would be ideal. However, we have yet to even clearly formulate this question with regard to a specific bioinformatic search algorithm. For pediatric cancers, the issue is even more concerning, as certain growth factor receptors may be present on normal tissue that are crucial to development, and would not be part of the “normal” gene expression signature, if it was defined by specimens from adult tissues. Before proceeding with targeting any of the antigens we have identified in this report, we must both confirm expression on tumor and more importantly, confirm as best as we can the lack of expression on “non-expendable” tissues. In this report we identified the major pediatric tumor-associated antigens that are expressed on the plasma membrane, as defined by standard gene chip analysis, statistical analysis, and filtering of annotated attributes. The strength of gene expression profiling is its utility in differential analysis (comparisons of transcript expression in different samples or comparison of tumor versus normal tissue). One potential drawback is that gene chip technology may be skewed, in some instances, toward identification of 3′ transcripts, and thus tumor-specific splice variations or deletions may be missed. Nevertheless studies, including our own, have shown a significant correlation between mRNA and protein levels (Chen et al., 2010). The Affymetrix plus2 array used here attempts to capture multiple independent measurements for each transcript and therefore measures expression of the major transcript as well as other splice variants. However a better more precise but not yet perfect measurement of the expression levels of each transcript is afforded by next generation technologies, e.g., whole RNA sequencing (RNAseq). We are pursuing these measurements, but until complete RNASeq is available for the major pediatric tumor types, along with normal tissue, our approach remains current. Validation with antibody or ligand-based staining for receptors in tumor normal tissues will be needed to definitively credential each candidate transcript.
Ultimately, we plan to continue to refine our definition of which membrane proteins can be safely targeted by CAR or antibody-based therapies, as new analysis techniques emerge. We propose that given the breadth of the antigen list presented here, that a large-scale effort be made to systematically evaluate cell surface targets on pediatric tumors for their potential for immunotherapy. Specifically, antibodies or scFv binding fragments for these antigens need to be screened in a large-scale manner for reactivity to normal tissues. The small size of the overall market in pediatric oncology for these reagents makes it unlikely that industry will engage in this approach on its own. However, given the wealth of genomic and proteomic data being generated, academia can take advantage of these findings and translate them into reagents that can be tested in pre-clinical settings. The analysis framework we present here may also inspire a new look at common adult malignancies, and encourage the development of a new generation of broad-based approaches for identifying immunotherapy targets.
The authors declare that the research was conducted in the absence of any commercial or financial relationships that could be construed as a potential conflict of interest.
This research was supported by the Intramural Research Program of the NIH, CCR, NCI.
Almen, M. S., Nordstrom, K. J., Fredriksson, R., and Schioth, H. B. (2009). Mapping the human membrane proteome: a majority of the human membrane proteins can be classified according to function and evolutionary origin. BMC Biol. 7:50. doi:10.1186/1741-7007-7-50
Bai, A., Meetze, K., Vo, N. Y., Kollipara, S., Mazsa, E. K., Winston, W. M., et al. (2010). GP369, an FGFR2-IIIb-specific antibody, exhibits potent antitumor activity against human cancers driven by activated FGFR2 signaling. Cancer Res. 70, 7630–7639.
Biagi, E., Marin, V., Giordano Attianese, G. M., Dander, E., D’Amico, G., and Biondi, A. (2007). Chimeric T-cell receptors: new challenges for targeted immunotherapy in hematologic malignancies. Haematologica 92, 381–388.
Brown, D. M., and Ruoslahti, E. (2004). Metadherin, a cell surface protein in breast tumors that mediates lung metastasis. Cancer Cell 5, 365–374.
Castriconi, R., Dondero, A., Augugliaro, R., Cantoni, C., Carnemolla, B., Sementa, A. R., et al. (2004). Identification of 4Ig-B7-H3 as a neuroblastoma-associated molecule that exerts a protective role from an NK cell-mediated lysis. Proc. Natl. Acad. Sci. U.S.A. 101, 12640–12645.
Chen, Q. R., Song, Y. K., Wei, J. S., Bilke, S., Asgharzadeh, S., Seeger, R. C., et al. (2008). An integrated cross-platform prognosis study on neuroblastoma patients. Genomics 92, 195–203.
Chen, Q. R., Song, Y. K., Yu, L. R., Wei, J. S., Chung, J. Y., Hewitt, S. M., et al. (2010). Global genomic and proteomic analysis identifies biological pathways related to high-risk neuroblastoma. J. Proteome Res. 9, 373–382.
Chowdhury, M. H., Nagai, A., Terashima, M., Sheikh, A., Murakawa, Y., Kobayashi, S., et al. (2008). Chemokine-like factor expression in the idiopathic inflammatory myopathies. Acta Neurol. Scand. 118, 106–114.
Clayton, A., Al-Taei, S., Webber, J., Mason, M. D., and Tabi, Z. (2011). Cancer exosomes express CD39 and CD73, which suppress T cells through adenosine production. J. Immunol. 187, 676–683.
Fang, J., Izawa, R., Gomez-Santos, L., Ueno, S., Sawaguchi, T., Usami, K., et al. (2009). Potentiation of proliferation of some but not all human colon carcinoma cell lines by immobilized hepatic asialoglycoprotein receptor 1. Oncol. Res. 17, 437–445.
Flies, D. B., Sandler, B. J., Sznol, M., and Chen, L. (2011). Blockade of the B7-H1/PD-1 pathway for cancer immunotherapy. Yale J. Biol. Med. 84, 409–421.
Fouchet, C., Gane, P., Huet, M., Fellous, M., Rouger, P., Banting, G., et al. (2000). A study of the coregulation and tissue specificity of XG and MIC2 gene expression in eukaryotic cells. Blood 95, 1819–1826.
Furukawa, H., Tohma, S., Kitazawa, H., Komori, H., Nose, M., and Ono, M. (2010). Role of SLAM-associated protein in the pathogenesis of autoimmune diseases and immunological disorders. Arch. Immunol. Ther. Exp. (Warsz.) 58, 37–44.
Gozalbo-Lopez, B., Gomez del Moral, M., Campos-Martin, Y., Setien, F., Martin, P., Bellas, C., et al. (2009). The MHC-related protein 1 (MR1) is expressed by a subpopulation of CD38+, IgA+ cells in the human intestinal mucosa. Histol. Histopathol. 24, 1439–1449.
Guay, S., Michaud, N., Bourcier, N., Leboeuf, M., Lemyre, M., Mailloux, J., et al. (2011). Distinct expression of the soluble and the membrane-bound forms of interleukin-1 receptor accessory protein in the endometrium of women with endometriosis. Fertil. Steril. 95, 1284–1290.
Herath, N. I., Spanevello, M. D., Doecke, J. D., Smith, F. M., Pouponnot, C., and Boyd, A. W. (2012). Complex expression patterns of Eph receptor tyrosine kinases and their ephrin ligands in colorectal carcinogenesis. Eur. J. Cancer 48, 753–762.
Heroult, M., Schaffner, F., Pfaff, D., Prahst, C., Kirmse, R., Kutschera, S., et al. (2010). EphB4 promotes site-specific metastatic tumor cell dissemination by interacting with endothelial cell-expressed ephrinB2. Mol. Cancer Res. 8, 1297–1309.
Hoglund, P. J., Nordstrom, K. J., Schioth, H. B., and Fredriksson, R. (2011). The solute carrier families have a remarkably long evolutionary history with the majority of the human families present before divergence of Bilaterian species. Mol. Biol. Evol. 28, 1531–1541.
Hume, D. A., and Macdonald, K. P. (2012). Therapeutic applications of macrophage colony-stimulating factor (CSF-1) and antagonists of CSF-1 receptor (CSF-1R) signaling. Blood 119, 1810–1820.
Jaras, M., Johnels, P., Hansen, N., Agerstam, H., Tsapogas, P., Rissler, M., et al. (2010). Isolation and killing of candidate chronic myeloid leukemia stem cells by antibody targeting of IL-1 receptor accessory protein. Proc. Natl. Acad. Sci. U.S.A. 107, 16280–16285.
Kanazawa, T., Misawa, K., and Carey, T. E. (2010). Galanin receptor subtypes 1 and 2 as therapeutic targets in head and neck squamous cell carcinoma. Expert Opin. Ther. Targets 14, 289–302.
Kasamon, Y. L., and Ambinder, R. F. (2008). Immunotherapies for Hodgkin’s lymphoma. Crit. Rev. Oncol. Hematol. 66, 135–144.
Kochenderfer, J. N., Wilson, W. H., Janik, J. E., Dudley, M. E., Stetler-Stevenson, M., Feldman, S. A., et al. (2010). Eradication of B-lineage cells and regression of lymphoma in a patient treated with autologous T cells genetically engineered to recognize CD19. Blood 116, 4099–4102.
Konner, J. A., Bell-McGuinn, K. M., Sabbatini, P., Hensley, M. L., Tew, W. P., Pandit-Taskar, N., et al. (2010). Farletuzumab, a humanized monoclonal antibody against folate receptor alpha, in epithelial ovarian cancer: a phase I study. Clin. Cancer Res. 16, 5288–5295.
Krasnoperov, V., Kumar, S. R., Ley, E., Li, X., Scehnet, J., Liu, R., et al. (2010). Novel EphB4 monoclonal antibodies modulate angiogenesis and inhibit tumor growth. Am. J. Pathol. 176, 2029–2038.
Lambert, Q. T., Pradhan, A., Roll, J. D., and Reuther, G. W. (2011). Mutations in the transmembrane and juxtamembrane domains enhance IL27R transforming activity. Biochem. J. 438, 155–164.
Lee, S. J., Reed, L. A., Davies, M. V., Girgenrath, S., Goad, M. E., Tomkinson, K. N., et al. (2005). Regulation of muscle growth by multiple ligands signaling through activin type II receptors. Proc. Natl. Acad. Sci. U.S.A. 102, 18117–18122.
Leslie, M. C., Zhao, Y. J., Lachman, L. B., Hwu, P., Wu, G. J., and Bar-Eli, M. (2007). Immunization against MUC18/MCAM, a novel antigen that drives melanoma invasion and metastasis. Gene Ther. 14, 316–323.
Malapeira, J., Esselens, C., Bech-Serra, J. J., Canals, F., and Arribas, J. (2011). ADAM17 (TACE) regulates TGFbeta signaling through the cleavage of vasorin. Oncogene 30, 1912–1922.
Martin-Kleiner, I., and Gall Troselj, K. (2010). Mannose-6-phosphate/insulin-like growth factor 2 receptor (M6P/IGF2R) in carcinogenesis. Cancer Lett. 289, 11–22.
May, K. F. Jr., Gulley, J. L., Drake, C. G., Dranoff, G., and Kantoff, P. W. (2011). Prostate cancer immunotherapy. Clin. Cancer Res. 17, 5233–5238.
Melnikova, V. O., and Bar-Eli, M. (2006). Bioimmunotherapy for melanoma using fully human antibodies targeting MCAM/MUC18 and IL-8. Pigment Cell Res. 19, 395–405.
Meynet, O., Scotlandi, K., Pradelli, E., Manara, M. C., Colombo, M. P., Schmid-Antomarchi, H., et al. (2010). Xg expression in Ewing’s sarcoma is of prognostic value and contributes to tumor invasiveness. Cancer Res. 70, 3730–3738.
Miller, J. S., Warren, E. H., van den Brink, M. R., Ritz, J., Shlomchik, W. D., Murphy, W. J., et al. (2010). NCI first international workshop on the biology, prevention, and treatment of relapse after allogeneic hematopoietic stem cell transplantation: report from the committee on the biology underlying recurrence of malignant disease following allogeneic HSCT: graft-versus-tumor/leukemia reaction. Biol. Blood Marrow Transplant. 16, 565–586.
Morgan, R. A., Yang, J. C., Kitano, M., Dudley, M. E., Laurencot, C. M., and Rosenberg, S. A. (2010). Case report of a serious adverse event following the administration of T cells transduced with a chimeric antigen receptor recognizing ERBB2. Mol. Ther. 18, 843–851.
Mosse, Y. P., Laudenslager, M., Longo, L., Cole, K. A., Wood, A., Attiyeh, E. F., et al. (2008). Identification of ALK as a major familial neuroblastoma predisposition gene. Nature 455, 930–935.
Mussai, F., Campana, D., Bhojwani, D., Stetler-Stevenson, M., Steinberg, S. M., Wayne, A. S., et al. (2010). Cytotoxicity of the anti-CD22 immunotoxin HA22 (CAT-8015) against paediatric acute lymphoblastic leukaemia. Br. J. Haematol. 150, 352–358.
Nakatsura, T., Kageshita, T., Ito, S., Wakamatsu, K., Monji, M., Ikuta, Y., et al. (2004a). Identification of glypican-3 as a novel tumor marker for melanoma. Clin. Cancer Res. 10, 6612–6621.
Nakatsura, T., Komori, H., Kubo, T., Yoshitake, Y., Senju, S., Katagiri, T., et al. (2004b). Mouse homologue of a novel human oncofetal antigen, glypican-3, evokes T-cell-mediated tumor rejection without autoimmune reactions in mice. Clin. Cancer Res. 10, 8630–8640.
Ohtaki, Y., Ishii, G., Nagai, K., Ashimine, S., Kuwata, T., Hishida, T., et al. (2010). Stromal macrophage expressing CD204 is associated with tumor aggressiveness in lung adenocarcinoma. J. Thorac. Oncol. 5, 1507–1515.
Olsen, S. K., Garbi, M., Zampieri, N., Eliseenkova, A. V., Ornitz, D. M., Goldfarb, M., et al. (2003). Fibroblast growth factor (FGF) homologous factors share structural but not functional homology with FGFs. J. Biol. Chem. 278, 34226–34236.
Pende, D., Bottino, C., Castriconi, R., Cantoni, C., Marcenaro, S., Rivera, P., et al. (2005). PVR (CD155) and Nectin-2 (CD112) as ligands of the human DNAM-1 (CD226) activating receptor: involvement in tumor cell lysis. Mol. Immunol. 42, 463–469.
Polson, A. G., Yu, S. F., Elkins, K., Zheng, B., Clark, S., Ingle, G. S., et al. (2007). Antibody-drug conjugates targeted to CD79 for the treatment of non-Hodgkin lymphoma. Blood 110, 616–623.
Porter, D. L., Levine, B. L., Kalos, M., Bagg, A., and June, C. H. (2011). Chimeric antigen receptor-modified T cells in chronic lymphoid leukemia. N. Engl. J. Med. 365, 725–733.
Saikali, Z., and Sinnett, D. (2000). Expression of glypican 3 (GPC3) in embryonal tumors. Int. J. Cancer 89, 418–422.
Schreiber, R. D., Old, L. J., and Smyth, M. J. (2011). Cancer immunoediting: integrating immunity’s roles in cancer suppression and promotion. Science 331, 1565–1570.
Stipp, C. S., Litwack, E. D., and Lander, A. D. (1994). Cerebroglycan: an integral membrane heparan sulfate proteoglycan that is unique to the developing nervous system and expressed specifically during neuronal differentiation. J. Cell Biol. 124, 149–160.
Taylor, J. G., Cheuk, A. T., Tsang, P. S., Chung, J. Y., Song, Y. K., Desai, K., et al. (2009). Identification of FGFR4-activating mutations in human rhabdomyosarcomas that promote metastasis in xenotransplanted models. J. Clin. Invest. 119, 3395–3407.
Textor, S., Fiegler, N., Arnold, A., Porgador, A., Hofmann, T. G., and Cerwenka, A. (2011). Human NK cells are alerted to induction of p53 in cancer cells by upregulation of the NKG2D ligands ULBP1 and ULBP2. Cancer Res. 71, 5998–6009.
Torrano, V., Procter, J., Cardus, P., Greaves, M., and Ford, A. M. (2011). ETV6-RUNX1 promotes survival of early B lineage progenitor cells via a dysregulated erythropoietin receptor. Blood 118, 4910–4918.
Wesche, J., Haglund, K., and Haugsten, E. M. (2011). Fibroblast growth factors and their receptors in cancer. Biochem. J. 437, 199–213.
Whiteford, C. C., Bilke, S., Greer, B. T., Chen, Q., Braunschweig, T. A., Cenacchi, N., et al. (2007). Credentialing preclinical pediatric xenograft models using gene expression and tissue microarray analysis. Cancer Res. 67, 32–40.
Williamson, D., Selfe, J., Gordon, T., Lu, Y. J., Pritchard-Jones, K., Murai, K., et al. (2007). Role for amplification and expression of glypican-5 in rhabdomyosarcoma. Cancer Res. 67, 57–65.
Wu, G. J., Wu, M. W., Wang, C., and Liu, Y. (2011). Enforced expression of METCAM/MUC18 increases tumorigenesis of human prostate cancer LNCaP cells in nude mice. J. Urol. 185, 1504–1512.
Yan, W. H. (2011). Human leukocyte antigen-G in cancer: are they clinically relevant? Cancer Lett. 311, 123–130.
Yang, J., Wang, F., Tian, L., Su, J., Zhu, X., Lin, L., et al. (2010). Fibronectin and asialoglyprotein receptor mediate hepatitis B surface antigen binding to the cell surface. Arch. Virol. 155, 881–888.
Zarrabi, K., Dufour, A., Li, J., Kuscu, C., Pulkoski-Gross, A., Zhi, J., et al. (2011). Inhibition of matrix metalloproteinase 14 (MMP-14)-mediated cancer cell migration. J. Biol. Chem. 286, 33167–33177.
Zeng, G., Cai, S., Liu, Y., and Wu, G. J. (2012). METCAM/MUC18 augments migration, invasion, and tumorigenicity of human breast cancer SK-BR-3 cells. Gene 492, 229–238.
Keywords: cancer antigens, rhabdomyosarcoma, Ewing’s sarcoma, osteosarcoma, hepatoblastoma, glioblastoma, neuroblastoma, sarcoma
Citation: Orentas RJ, Yang JJ, Wen X, Wei JS, Mackall CL and Khan J (2012) Identification of cell surface proteins as potential immunotherapy targets in 12 pediatric cancers. Front. Oncol. 2:194. doi: 10.3389/fonc.2012.00194
Received: 28 September 2012; Accepted: 30 November 2012;
Published online: 17 December 2012.
Edited by:
Peter Bader, University Hospital for Childhood and Adolescence Medicine, GermanyReviewed by:
Beat W. Schäfer, University Children’s Hospital, SwitzerlandCopyright: © 2012 Orentas, Yang, Wen, Wei, Mackall and Khan. This is an open-access article distributed under the terms of the Creative Commons Attribution License, which permits use, distribution and reproduction in other forums, provided the original authors and source are credited and subject to any copyright notices concerning any third-party graphics etc.
*Correspondence: Rimas J. Orentas, Pediatric Oncology Branch, National Institutes of Health, 10 Center Drive, 1W3840, Bethesda, MD 20892, USA. e-mail:cmltYXMub3JlbnRhc0BuaWguZ292
Disclaimer: All claims expressed in this article are solely those of the authors and do not necessarily represent those of their affiliated organizations, or those of the publisher, the editors and the reviewers. Any product that may be evaluated in this article or claim that may be made by its manufacturer is not guaranteed or endorsed by the publisher.
Research integrity at Frontiers
Learn more about the work of our research integrity team to safeguard the quality of each article we publish.