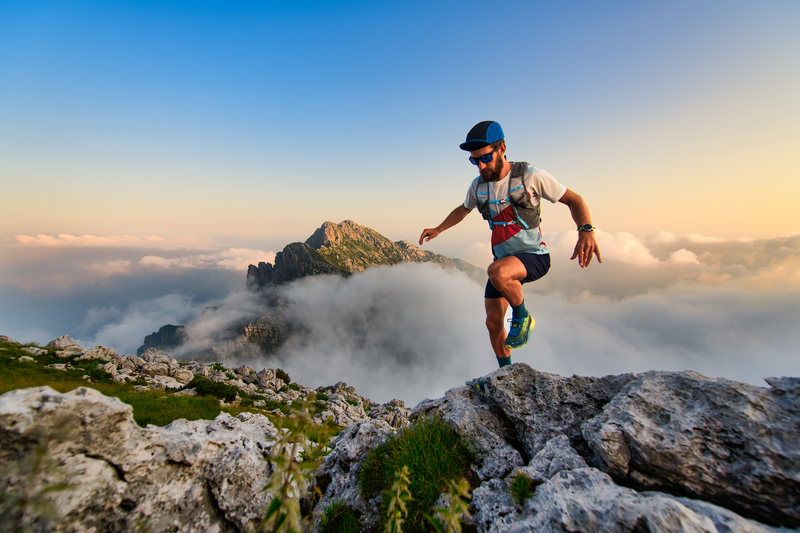
95% of researchers rate our articles as excellent or good
Learn more about the work of our research integrity team to safeguard the quality of each article we publish.
Find out more
EDITORIAL article
Front. Nucl. Eng. , 10 July 2024
Sec. Nuclear Reactor Design
Volume 3 - 2024 | https://doi.org/10.3389/fnuen.2024.1448953
This article is part of the Research Topic Artificial Intelligence in Advanced Nuclear Reactor Design View all 6 articles
Editorial on the Research Topic
Artificial intelligence in advanced nuclear reactor design
With the evolution of artificial intelligence (AI) technology, AI has become sophisticated and capable of solving complex problems in various fields, including manufacturing, finance, and education. In a similar vein, the rapid growth of AI has offered new considerations for the design of advanced nuclear reactors. Different to the traditional light water reactors, the advanced reactors have many innovative structures and accident conditions to consider. These reactors have necessitated cutting-edge technological approaches for their design and optimization. The integration of AI in this field is a testament to its transformative potential. It offers tools for the optimization of reactor design, autonomous control, and the development of advanced nuclear power software. To research the application of AI in advanced nuclear reactor design, the team of editors has curated a Research Topic in the journal Frontiers in Nuclear Engineering titled “Artificial Intelligence in Advanced Nuclear Reactor Design”. Five articles have been collected, and they cover different yet essential aspects of AI in advanced nuclear reactor design.
Lou et al. optimized the conceptual design of a lead-based modular nuclear power reactor core loaded with uranium alloy fuel, focusing on the selection of an optimal rod-to-diameter ratio and effective density to align with the core’s power level and lifetime of the core.
Ejigu et al. have provided a comprehensive review of the interplay between AI technologies and big data, elucidating their integration with nuclear power plants and the ensuing opportunities and challenges for the nuclear industry.
Wei et al. have used computational fluid dynamics (CFD) to investigate the three-dimensional flow field under normal operating conditions in the reactor pressure vessel (RPV) in the Hualong One nuclear power plants (NPPs). With a particular focus on the flowrate distribution at the core inlet, the numerical framework is validated against the integral hydraulic experiment in a 1:4-scaled RPV of CNP1000, the prototype of the Hualong One reactor. The simulation results of the normalized flowrate at the core inlet agree reasonably well with the measured data. Based on the experimental data, several methods of calibrating the CFD turbulence model coefficients have been suggested by introducing the concepts of data assimilation and machine learning.
Li et al. have introduced a predictive method for loss of coolant accidents (LOCAs) in the HPR1000 reactor, integrating multi-headed self-attention mechanisms with quantile regression to enhance prediction accuracy and uncertainty assessment.
Lastly, Li and Chen et al. have applied a novel deep learning model, zLSTM, evolved from Long-short Term Memory (LSTM) networks, to predict LOCAs with enhanced long-term processing capabilities, thereby illustrating the profound impact of deep learning on nuclear safety analysis.
After 1 year of revisions and updates, this Research Topic “Artificial Intelligence in Advanced Nuclear Reactor Design” finally selected these five articles, which have now been published in the journal Frontiers in Nuclear Engineering. The Research Topic underscores the growing intersection of AI and advanced nuclear reactor design, paving the way for safer, more efficient data-driven nuclear power solutions. More high-quality Research Topics and articles are welcomed to this open platform in the future. All the editors are open to further information and communications.
JD: Writing–original draft, Writing–review and editing, Resources. SC: Investigation, Writing–review and editing. YL: Investigation, Writing–review and editing. ZQ: Investigation, Writing–review and editing, JX: Investigation, Writing–review and editing.
The author(s) declare that no financial support was received for the research, authorship, and/or publication of this article.
The authors declare that the research was conducted in the absence of any commercial or financial relationships that could be construed as a potential conflict of interest.
All claims expressed in this article are solely those of the authors and do not necessarily represent those of their affiliated organizations, or those of the publisher, the editors and the reviewers. Any product that may be evaluated in this article, or claim that may be made by its manufacturer, is not guaranteed or endorsed by the publisher.
Keywords: artificial intelligence (AI), advanced nuclear reactor design, computational fluid dynamics (CFD), machine learning, reactor optimization, data assimilation, long-short term memory (LSTM)
Citation: Deng J, Xiao J, Cheng S, Liu Y and Qiu Z (2024) Editorial: Artificial intelligence in advanced nuclear reactor design. Front. Nucl. Eng. 3:1448953. doi: 10.3389/fnuen.2024.1448953
Received: 14 June 2024; Accepted: 24 June 2024;
Published: 10 July 2024.
Edited and reviewed by:
Wenxi Tian, Xi'an Jiaotong University, ChinaCopyright © 2024 Deng, Xiao, Cheng, Liu and Qiu. This is an open-access article distributed under the terms of the Creative Commons Attribution License (CC BY). The use, distribution or reproduction in other forums is permitted, provided the original author(s) and the copyright owner(s) are credited and that the original publication in this journal is cited, in accordance with accepted academic practice. No use, distribution or reproduction is permitted which does not comply with these terms.
*Correspondence: Jian Deng, ZGVuZ2ppYW5fZm5lQDE2My5jb20=
Disclaimer: All claims expressed in this article are solely those of the authors and do not necessarily represent those of their affiliated organizations, or those of the publisher, the editors and the reviewers. Any product that may be evaluated in this article or claim that may be made by its manufacturer is not guaranteed or endorsed by the publisher.
Research integrity at Frontiers
Learn more about the work of our research integrity team to safeguard the quality of each article we publish.