- Núcleo de Pesquisas em Produtos Naturais e Sintéticos, Faculdade de Ciências Farmacêuticas de Ribeirão Preto, Departamento de Ciências Biomoleculares, University of São Paulo, São Paulo, Brazil
The search for new Natural Products is one of the oldest interests for research in chemistry. The history of this discipline is very prolific, from its initial uses as medicine to advanced omics strategies to understand the mechanics of biological controls in living cells. The discoveries of morphine, penicillin, taxol and many other natural products revolutionized human health. During the last 40 years, excellent reviews published by Dr. David Newman and Dr. Gordon Crag have revealed that the pharmaceutical industry’s research into natural products has lost some of its steam. Nevertheless, new natural products for antimicrobials, antifungals, and anticancer drugs remained the main alternative. Furthermore, research institutes have seen great vigor, and several startup companies have emerged worldwide, seeking therapeutic alternatives based in natural products. Innovations on spectrometric hardware, allowing broader coverage of chemical classes and spatial mapping, and in software, enabling the interpretation of large volumes of data, afforded a renewed interest in natural products and are considered the most important enabling technology for the new companies. In this perspective article we give some historical context to exciting developments, on software and spatial resolution, that promise to pave the way for a bright future of Natural Products research.
Introduction
Natural products chemistry (NPC) is one of the oldest areas of investigation in chemistry, and the use of plant and animal preparations dates back to civilizations before the Christian time (Lopes and Vieira, 2017). The isolation of morphine at the beginning of the 19th century marked the inception of the rational isolation of natural products and its use for medicine (David et al., 2015). Since then, natural products with multiple uses, such as antimicrobials, antiparasitics, lipid control, immunosuppressants, and anticancer agents, have been isolated from various natural sources, including microbes, plants, and animals (Li and Vederas, 2009). Newman and Cragg (2020) reviewed drugs approved by United States’ Food and Drug Administration (FDA) and similar organizations from 01/1981 to 09/2019, and 41.9% (787) were derived from natural sources, showing the continued importance of natural products research for human health. Despite the historical success of natural products drug based development, by the beginning of the 21st century, the pharmaceutical industry started to reduce the investment in NPC research, mainly due to its complex chemistry, with properties that are not desired for drugs, which slows the testing process and leads to problems in the production phase (Li and Vederas, 2009). Instead, the big pharma companies invested in high-throughput screening (HTS) of synthetic libraries. Even with a low hit rate, the industry believed that any lead would be easy to produce and adapt (Li and Vederas, 2009). Another challenge for the industry was the projected revenue for a new drug. For example, the low treatment duration of antimicrobials, when compared to a hyertensive, make the development less attractive (David et al., 2015). The growth of NPC, especially in the 20th century, was never linear, and two explanations can be directly related. The first would be the economic one. For example, after the expansion of cost-effective organic synthesis, with decreased interest in NPC, it seemed that the search for natural models could have gone down in history. In recent years, a tide change showed a renewed interest in NPC. The recent Nobel Prize divided by William C. Campbell and Satoshi O̅mura put NPC in the spotlight. The review carried out by Pye and co-workers describing NPC potential in numbers showed, once more, how much NPC is still relevant and occupies a prominent place in science (Pye et al., 2017). It can be argued that the renewed interest in NPC was made possible by developing new methodologies and tools for structure elucidation. The main advances enabled analysis of natural extracts, often composed of complex mixtures. The desired compound can be in a low concentration, complicating isolation, and rendering common pharma industry test and production phases a challenging task. To explore the complex mixtures, chromatographic fractionation and HTS like testing, in parallel to mass spectrometry measurement, has proven to be an effective method to find new leads (Bugni et al., 2008).
In the development context described above, we can assume that mass spectrometry influenced the growth of startups in the area of natural products on at least two occasions, thus reducing the risks of the large pharmaceutical and veterinary industries, expanding the entry of new assets into the market. The first, around the 2000s, with the possibility of isolating ions at high resolution, enabling knowledge of fragmentation pathways. These innovations empowered the analysis of new derivatives, for example, ionophores. The ionophores were key to combine mass spectrometry with the selection of new strains of microorganisms (Gates, et al., 1999; Lopes et al., 2002; Cortes et al., 2003). In a second moment, the advance of mass spectrometry based metabolomics, supporting technology-based companies in the field of natural products (Guaratini, 2016). In this perspective article, some advances in strategies for natural products characterization are contextualized, highlighting mass spectrometry, hinting the major challenges for the next decade of NPC research.
Advances in natural products identification
In 1991, Professor Nicolas C. Thomas writing on the history of spectroscopy, cited the effects of developments in magnetic fields on NMR, the impact of McLafferty and colleagues’ results on mass spectrometry (MS), and the less impactful but important, advances observed in Ultraviolet and Infrared spectroscopy (Thomas 1991). Each advance allowed researchers to open new doors in the Universe of chemical characterization and a number of significant results were obtained on an unanticipated scale. In 2016, Professor James Hanson presented a historical review of the last 100 years of structural determination, from the classic chemical reaction experiments to define the identity of materials in the early 20th century to the most modern advances in spectroscopy and spectrometry. The review focused on the analysis of isolated substances. For Hanson, 2017, the advances in NMR magnetic fields. and the greater access to high-resolution mass spectrometers were the main achievements at the end of the last century (Hanson, 2017). In 1995, the concept of metabolomics studies emerged, but only in 2005 the first metabolomics tandem mass spectrometry database (METLIN) became available for this purpose (Smith et al., 2005). The following years led to a frantic search for metabolomics tools, seeking to understand natural products as candidates that may modulate ecological interactions, physiological functions, or pharmacological effects (Lopes and Vieira, 2017). In the dawn of metabolomics Mass Spectrometry was highlighted as a tool of choice to investigate biological systems (Demarque, et al., 2020). For a more general overview, an outline of some important advance landmarks in NPC is presented (Figure 1).
This greater adoption of MS, based mainly on the development and improvement of MS hardware, for example, with introduction of electrospray ionization (Fenn, et al., 1990) led to a leap in the understanding of the physiological function of natural products from different sources (Brunetti, et al., 2019), a greater coverage of biosynthetic pathways (Brady and Clardy., 2005) and a greater rationalization in the search for new biologically active compounds (Wishart. 2016). All these advances observed along the road that brought us here beg the question: Could spectrometric methods be present in ordinary analytical routines? Sure, advanced spectrometry practitioners will recognize that spectrometry techniques are already applied in airports, forensics, medicine, and other standard validation processes that require high sensitivity and accuracy. However, chemists also know that a few barriers are stopping them from using these advanced methods on daily quality control or inspection routines. The first main challenge is the cost, making the use prohibitive for most applications. The second is the complexity of data analysis, which makes the data interpretation of medical or environmental samples, for example, time-consuming and renders the approach not applicable for time-limited tasks.
For the cost challenge, new advances in hardware are most likely required. When analyzed over time, the cost of mass spectrometry analysis is steadily decreasing (Aksenov, et al., 2017). Nevertheless, when compared with sequencing, where a breakthrough on instrumentation, with massive parallel sequencing using nanotechnology, allowed an exponential cost drop, the decrease in MS cost is still modest (Aksenov et al., 2017). While it is not possible to anticipate a similar breakthrough in spectrometry hardware, the complementarity between new advances, when associated with software improvements, can allow the expansion of applications.
Sensitivity and speed are among the most desired characteristics of spectrometry techniques. These advantages make mass spectrometers and NMR the tools of choice to research on biomedicine, chemistry, and environmental areas, just to mention a few. For an expansion to research or, especially, for industry setup, where faster and easily interpretable data analysis results are required, new improvements are still needed. Traditionally, most spectrometry techniques rely on database matching of previously characterized signals. The initial databases were mainly commercially available and presented a slow expansion. As an example, the NIST mass spectral database, on its 2017 version had >210 k spectra, representing only 15 k different compounds. The NIST 2020 version (https://www.nist.gov/programs-projects/nist20-updates-nist-tandem-and-electron-ionization-spectral-libraries) doubled the compound coverage, including 31 k compounds, which is a significant expansion for the time span. However, as a proxy for the chemicals known in Nature, we have more than 100 million compounds on PubChem or SciFidened databases. If we think the limiting factor for the expansion of spectrometry application is reliable data interpretation, this pace of increase would be insufficient for a timely expansion.
Fortunately, we are experiencing fast developments in software that holds the promise to optimize the data analysis, possibly enabling the tipping point of the spectrometry application expansion to research and beyond. The new developments in data analysis can be experienced in our daily lives with virtual assistants, self-driving cars and internet of things. These new developments were enabled by advances in many fields, such as hardware development, with ever-increasing processing and storing power, or new algorithmic innovations, with the widespread application of machine learning techniques. However, the main driver of all the evolution we are experiencing is the availability of large volumes of data to train the algorithms.
The main reference in science is the development of AlphaFold (Jumper et al., 2021), by Google’s DeepMind company. The tool uses machine learning to predict 3D protein structures, outperforming all existing models. This achievement was only possible because of the availability of large experimentally determined 3D structures, being described over decades of hard work of scientists, funded mainly by public funding.
The same revolution is being experimented with in the mass spectrometry field. The creation of large public databases such as MassBank (Horai et al., 2010), MetaboLights (Steinbeck et al., 2012), GNPS (Wang et al., 2016), and Metabolomics Workbench (Sud et al., 2015) is enabling the development of tools that can quickly characterize large datasets acquired in complex samples. The first impact of public data is the use of traditional database search to promptly annotate previously described compounds in a new sample. Currently, there are estimates of spectra for approximately 60,000 compounds in the public domain (Stravs et al., 2022), for ESI-MS2 alone. This number is still far from covering the known chemical space, but it shows the power of data sharing compared to vendor libraries, as mentioned above. Another layer of use of public data is the metadata. Sample source and spectral similarity can provide a powerful combination to annotate a completely unknown spectrum. The efforts led by Dorrestein lab have developed a number of tools that enable access to public raw data and compare with a query spectrum (Wang et al., 2020) or dataset (Jarmusch et al., 2020; Gauglitz et al., 2022).
The second layer of public data contribution is the training of machine learning based methods. One group of methods is based on internal similarities contained in the data, and does not require human labeling of spectral identity, also known as unsupervised learning. Methods like MS2LDA (van der Hooft et al., 2017) and Spec2Vec (Huber et al., 2021) draw inspiration from text mining to detect recurring patterns of fragmentation in mass spectra and describe structural motifs from fragmentation patterns, for the first. For the second, similar recurring fragmentation patterns are also used to infer spectral similarities inside experimental sample(s), creating spectral networks that can speed structural elucidation, by leveraging connections to spectra that can be annotated by data in spectral databases.
Another layer of advances is enabled by supervised learning methods, which make use of publicly characterized data, by using the structural information associated with spectra. Given a spectrum, can we predict the structure? Not quite yet, but we are getting closer. The CASMI (Critical Assessment of Small Molecule Identification) context has been comparing computational structural elucidation methods for 10 years. Among the best-performing methods in the last contests is the SIRIUS (Böcker et al., 2017) (including CSI-FIngerID) tool. SIRIUS was first released in 2009, performing formula prediction from isotope patterns (Dührkop et al., 2019). The tool evolved over the last decade, in its fourth version, SIRIUS integrates isotopic pattern and fragmentation trees to predict structures (e.g. assign a structural annotation to a given spectrum) from large mass spectrometry fragmentation datasets.
The last, but certainly not the least, group of methods to cite are computational methods that do not rely on labeled or previously characterized structures and can potentially predict completely new 2D structures. As for unsupervised (Spec2vec) and supervised (components of SIRIUS), the deep learning algorithms have been applied in many spectrometry-related fields, for example, using Recurrent Neural Network (RNN) and Reinforcement Learning to predict new structures, without necessarily being present in the training set. Stravs et al. (2022) presented MSNovelist, combining fingerprint prediction performed by SIRIUS with a machine learning method, to perform de novo structural prediction, without the labeled data for training phase of the algorithm. The tool is an excellent addition for hypothesis generation, especially for new classes of compounds, poorly represented in spectral libraries. Soon, the combination of all these computational advances will exponentially increase the ability to annotate natural products and will take us one more step towards understanding the metabolome, which will be important to support even better resolved NPC studies from environment to single cell analysis.
Single cell analysis, the next step for NPC
Along with the advances obtained with metabolomics supported by LC-MS/MS techniques, the first images of natural products using the MALDI-MS technique allowed a much greater understanding of the function of these low molecular weight substances (Esquenazi, et al., 2009). Imaging of small molecules helped to leverage the interest in mass spectrometry as a tool for spatial metabolomics studies, such as, for example, in the study of tumor cell distribution in a tissue (Mittal et al., 2021), in the interface of chemical interaction in microbial co-cultures searching for new antibiotics, understanding chemical signaling in ecological relationships, among others (Buchberger et al., 2018). Imaging studies of natural products allow for the spatial mapping of the production and distribution of natural products in situ. It facilitates the description of chemical barriers for protection against UV radiation (Silva, et al., 2014), chelation of phenolics with metals to act as antimicrobials (Soares, et al., 2015), in addition, of course, to have given another strong boost to the discovery of new bioactive molecules for human use (Spraker, et al., 2020).
As highlighted, the generation of images from simple MALDI spectra (m/z versus intensity) helped to leverage the interest in mass spectrometry as a tool for metabolomics studies (Buchberger et al., 2018). These advances have changed the way we look at the distribution of chemicals in tissues. Let us now dig deeper with analytical techniques, adapted for targeted or non-targeted metabolomics studies (covering all chemical classes, such as lipids, polysaccharides, and secondary metabolites, among others) directly on a tissue, but with cellular resolution (Figure 2). The technological advancement, in terms of equipment, is already available, and recent publications allow us to dream with this new resolution to the chemistry of natural products. In a recent article, Tans and co-workers use spatial metabolomics where an image and MS/MS data are simultaneously generated, leading to the possibility of annotating the substances present in regions of 5 μm (Tans R. et al., 2021), something never thought of before. The addition of a third dimension provided by ionic mobility in imaging systems opens up enormous potential for the same studies in single-cell analysis. This application has been called “Spatialomics” since, in addition to annotating the compound in the 4D call (m/z, isotopic ratio, CCS, and fragmentation), it is also possible to show its location in a given tissue with a focus on the magnitude of 5 μm (Soltwisc et al., 2020). This possibility of understanding the function of a certain natural product in the cellular environment will surely open up new questions to be investigated and will give new strength to NPC.
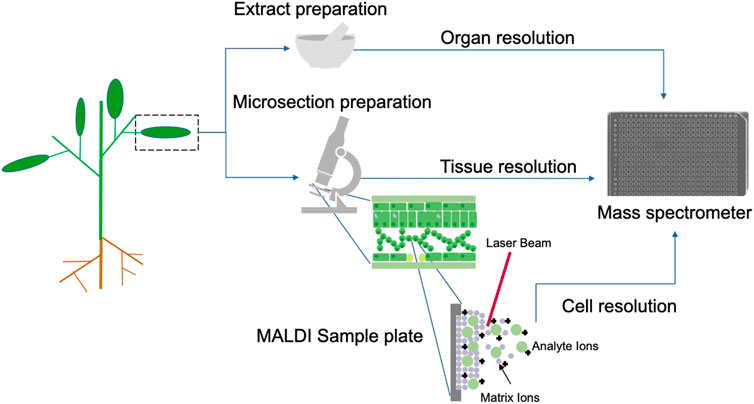
FIGURE 2. Different resolutions that a natural product can be detected. Advance in MALDI imaging allow analyte ionization at cell resolution.
Conclusion
Will this technological advance, associated with the new computational tools described above, be the necessary support for us to gain to a deeper understanding on the natural products’ function within cells? The road ahead in the next decade is full of wonders and excitement, so put on your lab coat and goggles and hop on for this new adventure to the unknown depths of the cell.
Data availability statement
The original contributions presented in the study are included in the article/Supplementary material, further inquiries can be directed to the corresponding authors.
Author contributions
All authors listed have made a substantial, direct, and intellectual contribution to the work and approved it for publication.
Funding
The authors researcher are supported by Conselho Nacional de Desenvolvimento Científico e Tecnológico (CNPq Project number 305297/2017-1) and Fundação de Pesquisa do Estado de São Paulo (FAPESP Project numbers: 2017/18922-2, 2019/05026-4 and 20/02207-5).
Conflict of interest
The authors declare that the research was conducted in the absence of any commercial or financial relationships that could be construed as a potential conflict of interest.
Publisher’s note
All claims expressed in this article are solely those of the authors and do not necessarily represent those of their affiliated organizations, or those of the publisher, the editors and the reviewers. Any product that may be evaluated in this article, or claim that may be made by its manufacturer, is not guaranteed or endorsed by the publisher.
References
Aksenov, A., da Silva, R., Knight, R., Lopes, N. P., and Dorrestein, P. (2017). Global chemical analysis of biology by mass spectrometry. Nat. Rev. Chem. 1, 0054. doi:10.1038/s41570-017-0054
Böcker, S., Kind, T., Torbašinović, H., Obrenović, S., Mehta, S. S., Tsugawa, H., et al. (2017). Searching molecular structure databases using tandem MS data: Are we there yet? Curr. Opin. Chem. Biol. 36, 1–6. doi:10.1016/j.cbpa.2016.12.010
Brady, S. F., and Clardy, J. (2005). Systematic investigation of the Escherichia coli metabolome for the biosynthetic origin of an isocyanide carbon atom. Angew. Chem. Int. Ed. 44, 7045–7048. doi:10.1002/anie.200501942
Brunetti, A. E., Lyra, M. L., Melo, W. G. P., Andrade, L. E., Palacios-Rodríguez, P., Prado, B. M., et al. (2019). Symbiotic skin bacteria as a source for sex-specific scents in frogs. Proc. Natl. Acad. Sci. 116, 2124–2129. doi:10.1073/pnas.1806834116
Buchberger, A. R., DeLaney, K., Johnson, J., and Li, L. (2018). Mass spectrometry imaging: A review of emerging advancements and future insights. Anal. Chem. 90 (1), 240–265. doi:10.1021/acs.analchem.7b04733
Bugni, T. S., Richards, B., Bhoite, L., Cimbora, D., Harper, M. K., and Ireland, C. M. (2008). Marine natural product libraries for high-throughput screening and rapid drug discovery. J. Nat. Prod. 71, 1095–1098. doi:10.1021/NP800184G
Cortes, J., Leadlay, P. F., Alastair, H., Mcarthur, I., and Staunton, J. (2003). “Polyketides and their synthesis,”. Patent AU765257B2 (Cambridge, UK: Biotica Technology Ltd Pfizer Inc).
David, B., Wolfender, J. L., and Dias, D. A. (2015). The pharmaceutical industry and natural products: Historical status and new trends. Phytochem. Rev. 14, 299–315. doi:10.1007/s11101-014-9367-z
Demarque, D. P., Dusi, R. G., de Sousa, F. D. M., Grossi, S. M., Silvério, M. R. S., Lopes, N. P., et al. (2020). Mass spectrometry-based metabolomics approach in the isolation of bioactive natural products. Sci. Rep. 10, 1051. doi:10.1038/s41598-020-58046-y
Dührkop, K., Fleischauer, M., Ludwig, M., Aksenov, A. A., Melnik, A. V., Meusel, M., et al. (2019). Sirius 4: A rapid tool for turning tandem mass spectra into metabolite structure information. Nat. Methods 16, 299–302. doi:10.1038/s41592-019-0344-8
Esquenazi, E., Yang, Y. L., Watrous, J., Gerwick, W. H., and Dorrestein, P. C. (2009). Imaging mass spectrometry of natural products. Nat. Prod. Rep. 26, 1521–1534. doi:10.1039/b915674g
Fenn, J. B., Mann, M., Meng, C. K., Wong, S. F., and Whitehouse, C. M. (1990). Electrospray ionization–principles and practice. Mass Spectrom. Rev. 9, 37–70. doi:10.1002/mas.1280090103
Gates, P. J., Kearney, G. C., Jones, R., Leadlay, P. F., and Staunton, J. (1999). Structural elucidation studies of erythromycins by electrospray tandem mass spectrometry. Rap. Comm. Mass Spectrom. 13, 242–246. doi:10.1002/(SICI)1097-0231(19990228)13:4<242:AID-RCM447>3.3.CO;2-2
Gauglitz, J. M., West, K. A., Bittremieux, W., Williams, C. L., Weldon, K. C., Panitchpakdi, M., et al. (2022). Enhancing untargeted metabolomics using metadata-based source annotation. Nat. Biotechnol. 25, 1774–1779. doi:10.1038/s41587-022-01368-1
Guaratini, T. (2016). Knowledge-intensive business services in Brazil: Entrepreneurship in a stimulating scenario. ACS Symp. Ser. 1219, 123–129. doi:10.1021/bk-2016-1219.ch014
Hanson, J. L. (2017). A hundred years in the elucidation of the structures of natural products. Sci. Prog. 100 (1), 63–79. Paper 1700221. doi:10.3184/003685017X14876775256129
Horai, H., Arita, M., Kanaya, S., Nihei, Y., Ikeda, T., Suwa, K., et al. (2010). MassBank: A public repository for sharing mass spectral data for life sciences. J. Mass Spectrom. 45, 703–714. doi:10.1002/jms.1777
Huber, F., Ridder, L., Verhoeven, S., Spaaks, J. H., Diblen, F., Rogers, S., et al. (2021). Spec2Vec: Improved mass spectral similarity scoring through learning of structural relationships. PLoS Comput. Biol. 17, e1008724. doi:10.1371/JOURNAL.PCBI.1008724
Jarmusch, A. K., Wang, M., Aceves, C. M., Advani, R. S., Aguirre, S., Aksenov, A. A., et al. (2020). ReDU: A framework to find and reanalyze public mass spectrometry data. Nat. Methods 179 (17), 901–904. doi:10.1038/s41592-020-0916-7
Jumper, J., Evans, R., Pritzel, A., Green, T., Figurnov, M., Ronneberger, O., et al. (2021). Highly accurate protein structure prediction with AlphaFold. Nat 2021, 583–589. doi:10.1038/s41586-021-03819-2
Li, J. W. H., and Vederas, J. C. (2009). Drug discovery and natural products: End of an era or an endless frontier? Science 325, 161–165. doi:10.1126/SCIENCE.1168243
Lopes, N. P., Stark, C. B. W., Gates, P. J., and Staunton, J. (2002). Fragmentation studies on monensin A by sequential electrospray mass spectrometry. Analyst 127, 503–506. doi:10.1039/b110412h
Lopes, N. P., and Vieira, P. C. (2017). Natural products chemistry: What’s the next step? ACS Omega 12, 8794–8795. doi:10.1021/acsomega.7b01671
Mittal, P., Condina, M. R., Klingler-Hoffmann, M., Kaur, G., Oehler, M. K., Sieber, O. M., et al. (2021). Cancer tissue classification using supervised machine learning applied to MALDI mass spectrometry imaging. Cancers 13, 5388. doi:10.3390/cancers13215388
Newman, D. J., and Cragg, G. M. (2020). Natural products as sources of new drugs over the nearly four decades from 01/1981 to 09/2019. J. Nat. Prod. 83, 770–803. doi:10.1021/acs.jnatprod.9b01285
Pye, C. R., Bertin, M. J., Lokey, R. S., Gerwick, W. H., and Linington, R. G. (2017). Retrospective analysis of natural products provides insights for future discovery trends. Proc. Natl. Acad. Sci. U. S. A. 114, 5601–5606. doi:10.1073/pnas.1614680114
Silva, D., Turatti, I., Gouveia, D., Ernst, M., Teixeira, S. P., and Lopes, N. P. (2014). Mass spectrometry of flavonoid vicenin-2, based sunlight barriers in lychnophora species. Sci. Rep. 4, 4309. doi:10.1038/srep04309
Smith, C. A., O'Maille, G., Want, E. J., Qin, C., Trauger, S. A., Brandon, T. R., et al. (2005). Metlin: A metabolite mass spectral database. Ther. Drug Monit. 27 (6), 747–751. doi:10.1097/01.ftd.0000179845.53213.39
Soares, M. S., da Silva, D. F., Forim, M. R., da Silva, M. F. D. F., Fernandes, J. B., Vieira, P. C., et al. (2015). Quantification and localization of hesperidin and rutin in Citrus sinensis grafted on C. limonia after Xylella fastidiosa infection by HPLC-UV and MALDI imaging mass spectrometry. Phytochem 115, 161–170. doi:10.1016/j.phytochem.2015.02.011
Soltwisch, J., Heijs, B., Koch, A., Vens-Cappell, S., Höhndorf, J., and Dreisewerd, K. (2020). MALDI-2 on a trapped ion mobility quadrupole time-of-flight instrument for rapid mass spectrometry imaging and ion mobility separation of complex lipid profiles. Anal. Chem. 92, 8697–8703. doi:10.1021/acs.analchem.0c01747
Spraker, J. E., Luu, G. T., and Sanchez, L. M. (2020). Imaging mass spectrometry for natural products discovery: A review of ionization methods. Nat. Prod. Rep. 37, 150–162. doi:10.1039/c9np00038k
Steinbeck, C., Conesa, P., Haug, K., Mahendraker, T., Williams, M., Maguire, E., et al. (2012). MetaboLights: Towards a new COSMOS of metabolomics data management. Metabolomics 8, 757–760. doi:10.1007/s11306-012-0462-0
Stravs, M. A., Dührkop, K., Böcker, S., and Zamboni, N. (2022). MSNovelist: De novo structure generation from mass spectra. Nat. Methods 2022, 865–870. doi:10.1038/s41592-022-01486-3
Sud, M., Fahy, E., Cotter, D., Azam, K., Vadivelu, I., Burant, C., et al. (2015). Metabolomics Workbench: An international repository for metabolomics data and metadata, metabolite standards, protocols, tutorials and training, and analysis tools. Nucleic Acids Res. 44, D463–D470. gkv1042. doi:10.1093/nar/gkv1042
Tans, R., Dey, S., Dey, N. S., Grant, C., O’Toole, P., Kaye, P. M., et al. (2021). Spatially resolved immunometabolism to understand infectious disease progression. Front. Microbiol. 12, 709728. doi:10.3389/fmicb.2021.709728
Thomas, N. C. (1991). The early history of spectroscopy. J. Chem. Educ. 68, 631–634. doi:10.1021/ed068p631
van der Hooft, J. J. J., Wandy, J., Young, F., Padmanabhan, S., Gerasimidis, K., Burgess, K. E. V., et al. (2017). Unsupervised discovery and comparison of structural families across multiple samples in untargeted metabolomics. Anal. Chem. acs.analchem.7b01391 89, 7569–7577. doi:10.1021/acs.analchem.7b01391
Wang, M., Carver, J. J., Phelan, V. V., Sanchez, L. M., Garg, N., Peng, Y., et al. (2016). Sharing and community curation of mass spectrometry data with global natural products social molecular networking. Nat. Biotechnol. 34, 828–837. doi:10.1038/nbt.3597
Wang, M., Jarmusch, A. K., Vargas, F., Aksenov, A. A., Gauglitz, J. M., Weldon, K., et al. (2020). Mass spectrometry searches using MASST. Nat. Biotechnol. 38, 23–26. doi:10.1038/s41587-019-0375-9
Keywords: natural product (NP), mass spectometry, single cell, metabolomic, computational chemistry
Citation: Lopes NP and Roberto da Silva R (2023) From structural determination of natural products in complex mixtures to single cell resolution: Perspectives on advances and challenges for mass spectrometry. Front. Nat. Prod. 2:1109557. doi: 10.3389/fntpr.2023.1109557
Received: 27 November 2022; Accepted: 20 January 2023;
Published: 02 February 2023.
Edited by:
Emerson Ferreira Queiroz, University of Geneva, SwitzerlandReviewed by:
María Marta Zanardi, Independent researcher, Rosario, ArgentinaCopyright © 2023 Lopes and Roberto da Silva. This is an open-access article distributed under the terms of the Creative Commons Attribution License (CC BY). The use, distribution or reproduction in other forums is permitted, provided the original author(s) and the copyright owner(s) are credited and that the original publication in this journal is cited, in accordance with accepted academic practice. No use, distribution or reproduction is permitted which does not comply with these terms.
*Correspondence: Norberto Peporine Lopes, bnBlbG9wZXNAZmNmcnAudXNwLmJy; Ricardo Roberto da Silva, cmlkYXNpbHZhQHVzcC5icg==