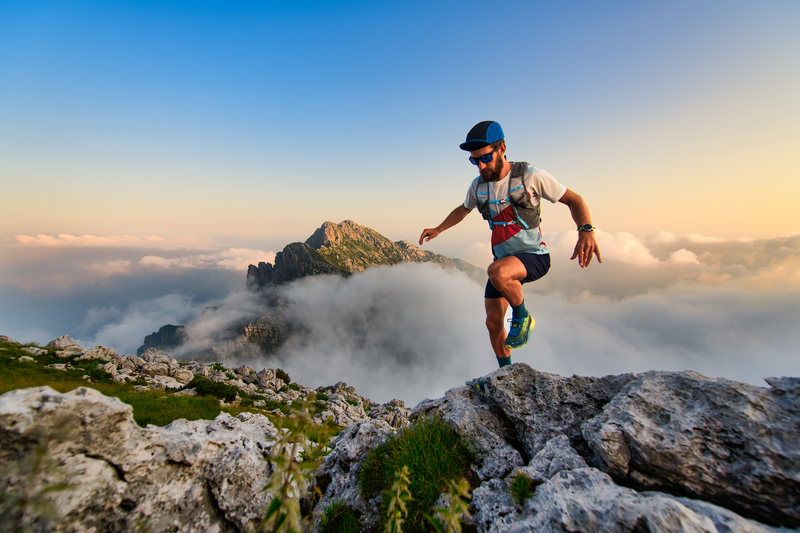
94% of researchers rate our articles as excellent or good
Learn more about the work of our research integrity team to safeguard the quality of each article we publish.
Find out more
EDITORIAL article
Front. Syst. Neurosci. , 19 December 2022
Volume 16 - 2022 | https://doi.org/10.3389/fnsys.2022.1002095
This article is part of the Research Topic Closed-Loop Iterations Between Neuroscience and Artificial Intelligence View all 7 articles
Editorial on the Research Topic
Closed-loop iterations between neuroscience and artificial intelligence
“There is no scientific study more vital to man than the study of his own brain. Our entire view of the universe depends on it.” No one will doubt the importance of neuroscience addressed by Francis Crick. Neuroscience, studying the structure and function of the nervous system, is the central focus of the 21st century. The brain is the only known example of truly general intelligence, which is of great enlightening significance to the study of artificial intelligence (AI) (Potter, 2007; Hassabis et al., 2017). Many AI researchers continue to look to neuroscience as a source of inspiration and insight (Ullman, 2019). Also, AI is rapidly becoming a valuable tool for neuroscience, helping to analyze various neural data and understand how the brain computes (Landhuis, 2020; Richards et al., 2022). Thus, the two synergistic fields have been keeping deep communication and exchange (Savage, 2019; Ito et al., 2022).
“If the human brain were so simple that we could understand it, we would be so simple that we couldn't.” This interesting quote from Emerson M. Pugh reflects our current position in trying to understand the human brain. Indeed, it is unclear how our brain works. We require multi-disciplinary inputs and efforts to unravel its intrinsic complexity. The mutual reinforcement between neuroscience and AI should be a closed loop for linking mind and machine. Computational neuroscience (Wang et al., 2020) could bridge these two fast-developing fields through adequate models representing and simulating the brain's unique architecture and functions as shown in Figure 1. The biophysics and algorithms obtained in neuroscience are crucial to implement brain-inspired intelligence. In turn, the AI system based on artificial neural network (ANN) inspired by the nervous system is being applied back to parse and interpret the brain. The full closed-loop may be organized as iterations and thus benefit both neuroscience and AI.
Figure 1. Schematic diagram of closed-loop iteration between neuroscience and artificial intelligence.
This Research Topic aims to provide a state-of-the-art review of computational neuroscience, including the development of AI inspired by neuroscience, the application of AI in brain research, and the closed-loop iterations between neuroscience and AI. So far, this Research Topic has collected 6 articles (5 original researches and 1 review) that represent the collaboration between AI and neuroscience.
There are 2 papers hitting on the topic of AI inspired by neuroscience. Zhao and Zeng proposed a brain-inspired synaptic pruning (BSP) algorithm, which can effectively modulate neural network architecture by pruning redundant synapses while retaining valid synapses during the learning process (Zhao and Zeng). Experimental results demonstrated that the BSP algorithm can significantly compress the network, showing its strengths and effectiveness in different sizes and the number of training samples on the network. Similarly, Liang and Zeng constructed a brain-inspired impulse neural network with the mechanism of human creativity, which can compose melodies with different styles of composers or genres (Liang and Zeng). It has been the first attempt to create musical melodies using biologically plausible ANN with the spike-timing-dependent plasticity learning rule. These two studies show that AI inspired by brain structures and functions is promising and moving toward human-like intelligence.
There are 4 papers focusing on the application of AI in neuroscience. (1) A detailed neocortical network model (also ANN) was presented to compare the capacities for signal processing between the human and rodent (Zhang et al.). The computational modeling suggests that the unique membrane properties in human neocortical neurons might improved the signal transmission accuracy. (2) Using electroencephalography (EEG) as input data, a machine learning method called random forest was used to detect schizophrenia (Vázquez et al.). The computational algorithm not only produces a diagnostic recommendation, but also helps the clinicians to locate key areas and/or connections in the brain associated with schizophrenia. (3) An ANN was pre-trained and exploited as a classifier achieving 74% accuracy on the EEG data of newly recruited subjects (Kuc et al.). This may greatly facilitate the development of brain-computer interfaces (BCI) by reducing the reliance of complex decoding algorithms. (4) The last review article comprehensively summarized the applications of reinforcement learning in BCI (Girdler et al.). Various reinforcement learning-based neural decoders have demonstrated impressive results and can be seamlessly integrated into more versatile and realistic BCIs. The aforementioned papers emphasize that the AI approaches are key techniques to make more discoveries and understand brain, even profit neural diseases and robotics.
Although there is no article presenting a complete closed-loop iteration in current Research Topic, this will potentially be a great work in the future. More recently, a single cortical neuron has been well approximated as a 5–8 layer deep ANN (Beniaguev et al., 2021). In addition, a general ANN has been optimized by introducing the principles of synaptic plasticity named group responsibility for adjusting the propagation of error signals (Dellaferrera et al., 2022). AI and improved microscopy have made it feasible to parse the connectome of the nervous system at ever-higher resolution (Landhuis, 2020). Just as Lichtman says, “Machines are learning to be smarter by studying the wiring of machines that are fundamentally smarter-biological machines.” No doubt, the closed-loop iterations between neuroscience and AI is a useful research paradigm for closing the gap between human intelligence and machine intelligence (Roy et al., 2019).
All authors listed have made a substantial, direct, and intellectual contribution to the work and approved it for publication.
This work was supported by the National Natural Science Foundation of China (Grant No. 61890951) and the Director Fund of WNLO. AZ thanks Center of Excellence “Center of Photonics” funded by the Ministry of Science and Higher Education of the Russian Federation, Contract No. 075-15-2022-293.
We would like to thank Mr. Rong Xiao and Ms. Ziqi Zhang for their help in preparing illustration and text.
The authors declare that the research was conducted in the absence of any commercial or financial relationships that could be construed as a potential conflict of interest.
All claims expressed in this article are solely those of the authors and do not necessarily represent those of their affiliated organizations, or those of the publisher, the editors and the reviewers. Any product that may be evaluated in this article, or claim that may be made by its manufacturer, is not guaranteed or endorsed by the publisher.
Beniaguev, D., Segev, I., and London, M. (2021). Single cortical neurons as deep artificial neural networks. Neuron 109, 2727–2739. doi: 10.1016/j.neuron.2021.07.002
Dellaferrera, G., Wozniak, S., Indiveri, G., Pantazi, A., and Eleftheriou, E. (2022). Introducing principles of synaptic integration in the optimization of deep neural networks. Nat. Commun. 13, 1885. doi: 10.1038/s41467-022-29491-2
Hassabis, D., Kumaran, D., Summerfield, C., and Botvinick, M. (2017). Neuroscience-inspired Artificial Intelligence. Neuron 95, 245–258. doi: 10.1016/j.neuron.2017.06.011
Ito, T., Yang, G. R., Laurent, P., Schultz, D. H., and Cole, M. W. (2022). Constructing neural network models from brain data reveals representational transformations linked to adaptive behavior. Nat. Commun. 13, 673. doi: 10.1038/s41467-022-28323-7
Landhuis, E. (2020). Probing fine-scale connections in the brain–Artificial intelligence and improved microscopy make it feasible to map the nervous system at ever-higher resolution. Nature 586, 631–633. doi: 10.1038/d41586-020-02947-5
Potter, S. M. (2007). What can AI get from neuroscience? in 50 Years of Artificial Intelligence, eds. M. Lungarella, F. Iida J. Bongard, R. Pfeifer (Berlin, Heidelberg: Springer) 174–185. doi: 10.1007/978-3-540-77296-5_17
Richards, B., Tsao, D., and Zador, A. (2022). The application of artificial intelligence to biology and neuroscience. Cell 185, 2640–2643. doi: 10.1016/j.cell.2022.06.047
Roy, K., Jaiswal, A., and Panda, P. (2019). Towards spike-based machine intelligence with neuromorphic computing. Nature 575, 607–617. doi: 10.1038/s41586-019-1677-2
Savage, N. (2019). Marriage of mind and machine. Nature 571, S15–S17. doi: 10.1038/d41586-019-02212-4
Ullman, S. (2019). Using neuroscience to develop artificial intelligence. Science 363, 692–693. doi: 10.1126/science.aau6595
Keywords: neuroscience, artificial intelligence, brain-inspired intelligence, computational model, neural network
Citation: Li J, Zaikin A, Zhang X and Chen S (2022) Editorial: Closed-loop iterations between neuroscience and artificial intelligence. Front. Syst. Neurosci. 16:1002095. doi: 10.3389/fnsys.2022.1002095
Received: 24 July 2022; Accepted: 29 November 2022;
Published: 19 December 2022.
Edited and reviewed by: Anna P. Malykhina, University of Colorado Anschutz Medical Campus, United States
Copyright © 2022 Li, Zaikin, Zhang and Chen. This is an open-access article distributed under the terms of the Creative Commons Attribution License (CC BY). The use, distribution or reproduction in other forums is permitted, provided the original author(s) and the copyright owner(s) are credited and that the original publication in this journal is cited, in accordance with accepted academic practice. No use, distribution or reproduction is permitted which does not comply with these terms.
*Correspondence: Shangbin Chen, c2JjaGVuQG1haWwuaHVzdC5lZHUuY24=
Disclaimer: All claims expressed in this article are solely those of the authors and do not necessarily represent those of their affiliated organizations, or those of the publisher, the editors and the reviewers. Any product that may be evaluated in this article or claim that may be made by its manufacturer is not guaranteed or endorsed by the publisher.
Research integrity at Frontiers
Learn more about the work of our research integrity team to safeguard the quality of each article we publish.