- 1Department of Psychiatry, School of Medicine, University of California, San Diego, San Diego, CA, United States
- 2Department of Basic Medical Sciences, College of Veterinary Medicine, Purdue University, West Lafayette, IN, United States
- 3Weldon School of Biomedical Engineering, Purdue University, West Lafayette, IN, United States
- 4Purdue Institute for Inflammation, Immunology, and Infectious Disease, West Lafayette, IN, United States
A large focus of modern neuroscience has revolved around preselected brain regions of interest based on prior studies. While there are reasons to focus on brain regions implicated in prior work, the result has been a biased assessment of brain function. Thus, many brain regions that may prove crucial in a wide range of neurobiological problems, including neurodegenerative diseases and neuropsychiatric disorders, have been neglected. Advances in neuroimaging and computational neuroscience have made it possible to make unbiased assessments of whole-brain function and identify previously overlooked regions of the brain. This review will discuss the tools that have been developed to advance neuroscience and network-based computational approaches used to further analyze the interconnectivity of the brain. Furthermore, it will survey examples of neural network approaches that assess connectivity in clinical (i.e., human) and preclinical (i.e., animal model) studies and discuss how preclinical studies of neurodegenerative diseases and neuropsychiatric disorders can greatly benefit from the unbiased nature of whole-brain imaging and network neuroscience.
Introduction
Historically, neuroscience has focused on specific regions of the brain such as the hippocampus for learning and memory (Scoville and Milner, 1957; Milner et al., 1998; Bird and Burgess, 2008), the hypothalamus for basal survival functions and motivated behavior (Swanson, 2000; Sternson, 2013), and the cerebellum for sensorimotor control (Buckner, 2013). These regions have distinct morphology and are large in comparison to the rest of the brain, making them simple targets for early exploration in neuroanatomy, staining, and electrophysiology techniques. This approach has left many regions understudied. This is highlighted in Figure 1, where during a search of 197 brain
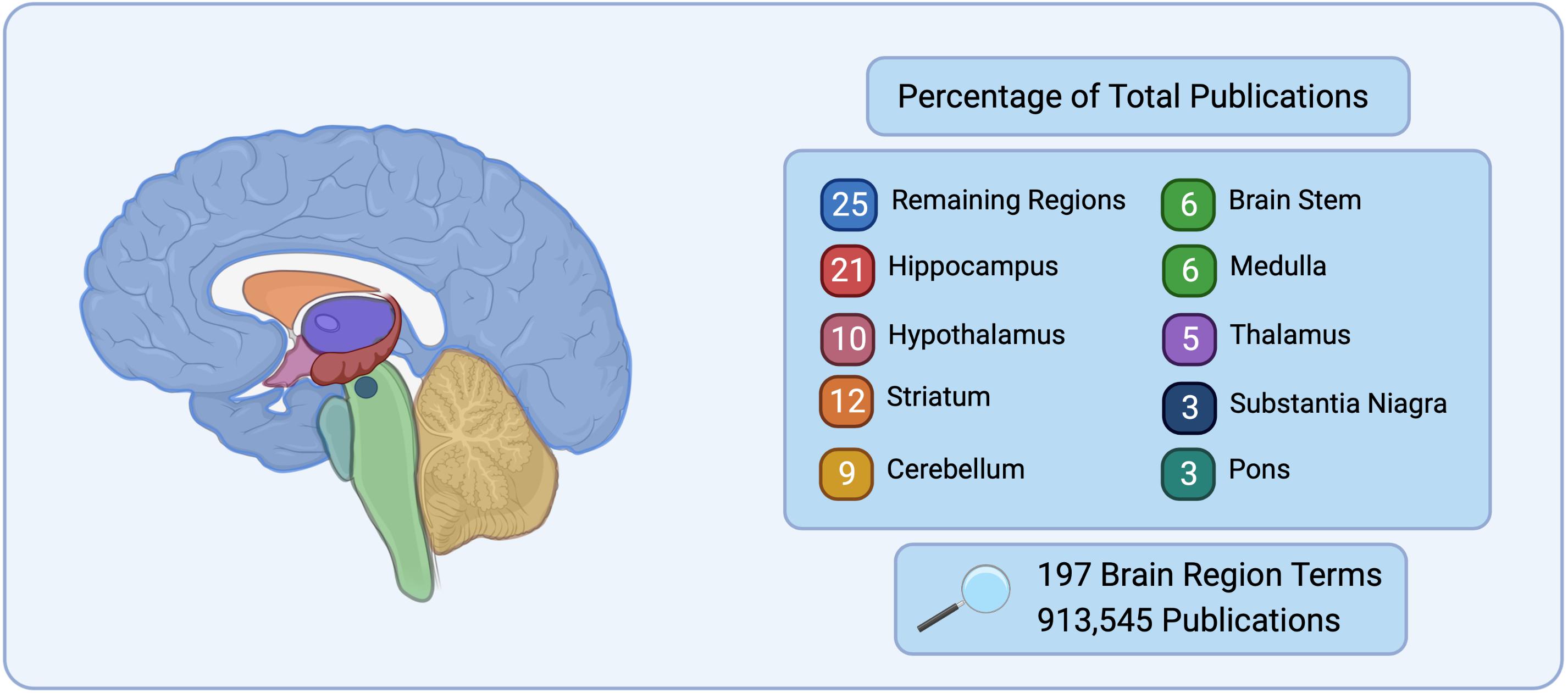
Figure 1. Number of publications listed on PubMed for 197 brain region terms searched for based on the Allen Mouse Brain Atlas (Allen Institute for Brain Science, 2004) nomenclature. The top nine brain regions represent 75% of the total publications, while the remaining 188 regions searched only represent 25% of total publications.
regions, 75% of the publications in PubMed were found to correspond to only 9 brain regions, while the remaining 188 brain regions belonged to 25% of the search results. It is the natural progression of good science to continue the examination of brain regions or circuits that have prior evidence to suggest their importance to a given disease, however this neglects a vast array of brain regions and circuits that may also be critical.
Until recent years there was a lack of the technology and computing power necessary to assess the whole brain in a truly unbiased manner, as well as to interrogate the interconnectivity and functionality of the entire neural network. Probing the brain as an integrated organ allows for novel methods to map, record, and analyze interactions between and within regions. The vastness and complexity of large data sets from the brain have required new ways to reduce dimensionality without significant data loss or bias (Hinton and Salakhutdinov, 2006; Cunningham and Yu, 2014; Mwangi et al., 2014; Beyeler et al., 2019; Crimi et al., 2019). Recent advances in computational tools have enabled the processing of dense information clouds following modulation of circuits, as well as the ability to create comprehensive connectivity maps to make network analysis possible (Thompson et al., 2007; Hawrylycz et al., 2012; Wohnoutka et al., 2014; Bakken et al., 2016; Bassett and Sporns, 2017).
Preclinical (i.e., animal model) whole-brain imaging approaches are now capable of providing immense data sets of neural activity with improved resolution (either timescale or brain structure resolution) than previously available. Additionally, advances in computational analysis of brain-wide function offer unique ways to assess brain activity from these data sets, during critical neural states, in an unbiased manner. Together, these approaches can help identify previously overlooked brain regions that may be critical for given disease states and help to contextualize the contribution of heavily studied brain regions to overall brain function. This review will cover the use of preclinical whole brain imaging and neural network tools to assess neural activity of the brain.
Circuit Manipulation and Whole Brain Imaging Methods in Preclinical Neuroscience
The ability to alter the excitatory or inhibitory properties of a neuron is critical to the study of the brain. Multiple circuit and imaging approaches can be leveraged to measure and manipulate brain activity. An early example of these tools is electrodes, which are a robust way to electrically stimulate or inhibit cells. Deep-brain stimulation using implantable electrodes has been highly effective in the treatment of epilepsy (Boon et al., 2009; Zangiabadi et al., 2019) but this technique requires invasive surgery and implantation. Clinically, transcranial magnetic stimulation (TMS) and focused ultrasound (FUS) are alternative ways to stimulate the brain without the need for invasive implantation (Lynn et al., 1942; Fry et al., 1955; Fishman and Frenkel, 2017). Further discussion of the methods and the progression of emergent technologies to manipulate the brain will be discussed in the following section.
Preclinical methods to influence neural signaling include electrophysiology, pharmacology, and more recently, optogenetics and chemogenetics for more specific neural stimulation. Optogenetics, leverages light sensitive ion channels to alter the activity of brain regions with a high degree of specificity. Light-gated cation channels such as excitatory channelrhodopsin-2 (ChR2) and inhibitory channels (i.e., halorhodopsin and archaerhopsin) can easily be integrated into neural tissue using viral vectors to confer temporal and spatial specificity (Boyden et al., 2005; Deisseroth et al., 2006; Zhang et al., 2006; Gradinaru et al., 2008; Miesenbock, 2009; Chow et al., 2010; Abe et al., 2012). Optogenetics has been employed to map neural circuitry, identify behaviors associated with poorly understood brain regions, and develop animal models to better understand of the contributions of a specific brain region or cell type in behavioral and emotional states (Bernstein and Boyden, 2011; Tye et al., 2011, 2013; Kim et al., 2013; Ye et al., 2016).
Furthermore, the combination of optogenetics and fMRI (ofMRI) has enabled preclinical investigation of the functional connectivity between neural circuits with spatial and temporal specificity (Lee et al., 2010; Abe et al., 2012; Lee, 2012; Lin et al., 2016; Lein et al., 2018). The use of ofMRI enables millisecond-timescale modulation of activity in the intact brain and has elucidated novel global and local fMRI signals driven by populations of optogenetically defined neurons. Development of this paradigm has improved the understanding of widespread brain responses to specific local activation (Weitz and Lee, 2013).
Chemogenetics, like optogenetics, has been used to identify distinct neural circuits associated with behavioral and emotional states (Strader et al., 1991; Bishop et al., 1998; Chen et al., 2005; Sternson and Roth, 2014; Zhu et al., 2014; Vetere et al., 2017). A benefit of chemogenetics is that the receptors can be genetically encoded and do not require the implantation of a light delivery device; however, the timescale is different from the millisecond activation of optogenetics, ranging 1–6 h for CNO DREADDS, and 5–60 min for KORD-activated DREADDS (Alexander et al., 2009; Guettier et al., 2009; Sternson and Roth, 2014; Roth, 2016; Urban et al., 2016; Whissell et al., 2016). Chemogenetics approaches can reduce surgical manipulation, leaving the brain tissue intact for later analysis, and simplify the associated behavioral assays. Additionally, these receptors can have a diverse range of cellular functions and signaling processes ranging from engineered kinases (Strader et al., 1991; Bishop et al., 1998; Chen et al., 2005), and G-protein coupled receptors (GPCRs) (Redfern et al., 1999; Armbruster et al., 2007; Alexander et al., 2009; Vardy et al., 2015) to ligand-gated ion channels (Lerchner et al., 2007; Arenkiel et al., 2008; Magnus et al., 2011) and the most commonly implemented DREADDs (Armbruster et al., 2007).
While optogenetics and chemogenetics focus on altering the excitability of the brain, visualizing the innate activation of neurons is just as crucial. Previously, the innate electrical activity of the brain was monitored by single electrodes or multielectrode arrays (Nicolelis and Ribeiro, 2002); however, this technique is limited by spatial specificity and is difficult to scale at the cellular level (Lewis et al., 2015). Ex vivo approaches, such as measurement of brain-wide protein from the immediate early gene c-fos, accomplish a snapshot of activity at a particular brain state (Ragan et al., 2012; Osten and Margrie, 2013) with intricate pipelines to employ serial sectioning and realignment (Mesina et al., 2016). However, this approach is limited to a generalized timescale with no way to repeatedly sample the same brain. The invention of two-photon calcium imaging allowed for some of the first in vivo visualizations of the activity of distinct neurons in brain tissue (Tsien, 1988). This real-time analysis revealed activity at the cellular and subcellular level. Calcium imaging can also be employed in in vitro studies in brain slices and in vivo preparations using two-photon microscopy (Denk et al., 1990; Mao et al., 2001; Oh et al., 2005; Benninger and Piston, 2013) or in combination with multielectrode recordings in freely moving animals to interrogate and reconstruct functional connectivity in real-time (Ozbay et al., 2018; Bonifazi and Massobrio, 2019).
Resting-state fMRI (R-fMRI) is another useful technique in comparing functional similarities across species. Xu et al. explored R-fMRI data from macaques and humans combined with a computational approach called joint embedding. They were able to assign common brain architecture features between human and macaque brains. Xu et al. (2020) further developed a Functional Connectivity Homology Index (FCHI) to quantify the cross-species similarities, pushing the limits of network analysis both within a species and between species. Additional methods to elucidate common features within fMRI data such as independent component analysis (ICA) can be employed as an exploratory method to reveal network patterning even when the stimuli are complex or are not time-locked to a specific event (Calhoun et al., 2001; Mckeown et al., 2003; Beckmann et al., 2005; Calhoun and Adali, 2012). This type of exploratory approach is data-driven, eliminating bias of a specific brain region or treatment/event (Mckeown et al., 1998). A complementary tool to ICA is Sparse Dictionary Learning (SDL) which is capable of evaluating functional networks with significant spatial overlap. However, ICA performs better in networks without spatial overlap (Zhang et al., 2019). Tools such as these are useful in bridging the cross-species gap that often emerges comparing preclinical models and human.
Another emerging technique in preclinical network science is 4D functional ultrasound (4DFUS) imagining of whole-brain for preclinical applications (Rabut et al., 2019). This approach developed by Rabut et al. implements multiplate wave transmissions on matrix arrays at thousands of frames per second to allow for volumetric recordings of blood volume changes in the brain with high resolution in both space and time. Ultrafast imaging relies on coherent compounding of backscattered echoes. The use of Hadamard coefficients can increase resolution without compromising the frame rate (Tiran et al., 2015). 4DFUS complements electrophysiological and optical methods because while those approaches provide similar specificity in terms of resolution, they lack the ability to expand monitoring to a larger-scale network.
Functional readouts provide insight to activity in the awake, behaving brain, but lack cellular resolution. Recent preclinical developments in tissue-clearing methods allow for three-dimensional imaging of the intact brain. There are many approaches for clearing tissues which include CLARITY (cleared lipid-extracted acryl-hybridized rigid immunostaining) (Chung et al., 2013), DISCO (Three-dimensional imaging of solvent-cleared organs) (Erturk et al., 2011, 2012), iDISCO (immunolabeling-enabled DISCO) (Renier et al., 2014), SHIELD (stabilization under harsh conditions via intra molecular epoxide linkages to prevent degradation) (Park et al., 2018), FocusClear (Fu and Tang, 2010), SeeDB (see Deep Brain) (Ke et al., 2013), FRUIT (Fructose Urea in α-Thioglycerol) (Hou et al., 2015), and CUBIC (clear, unobstructed brain imaging cocktails and computational analysis) (Susaki et al., 2015; Susaki and Ueda, 2016; Murakami et al., 2018; Matsumoto et al., 2019). The CLARITY approach consists of a hydrogel-based method which uses covalent linkage to an acryl-based hydrogel for complete lipid removal with limited structural damage and protein loss (Chung et al., 2013). The DISCO approach uses tetrahydrofuran, a dehydrating and delipidating agent, instead of an alcohol (Erturk et al., 2011, 2012). Further iterating on this method, Renier et al. (2014, 2016) developed iDISCO and iDISCO+, which allows for whole-mount immunolabeling of whole cleared organs and wide-scale mapping of brain activity by analysis of immediate early genes. As an alternative to the use of hydrophobic solvent clearing techniques, tissues can be impregnated with high-osmotic aqueous solutions for a hydrophilic approach with beneficial refractive indices (Tainaka et al., 2018). These methods include SeeDB (Ke et al., 2013), FRUIT (Hou et al., 2015), FocusClear (Fu and Tang, 2010), and CUBIC (Susaki and Ueda, 2016; Tainaka et al., 2018). For an extensive comparison of clearing techniques and microscopy applications (see Richardson and Lichtman, 2015; Silvestri et al., 2016; Vigouroux et al., 2017; Muntifering et al., 2018).
From circuit manipulation to novel clearing techniques the development of tools to enable the observation and alteration of an intact brain with a spatial and temporal resolution has encouraged the exploration of brain regions that were previously impossible to assess. However, the data that is produced by these techniques is immense and difficult to directly interpret. Advanced computational network-based approaches, especially with regard to brain clearing and neural activity, such as ClearMap (Renier et al., 2016) are necessary for taking full advantage of data from cleared brains.
Computational Analysis of Neural Networks
The combination of preclinical whole-brain imaging approaches with advances in computational analysis of brain-wide function serves as a unique way to assess brain activity during critical neural states in an unbiased manner. Methods of unbiased assessment including fMRI, calcium imaging, and immediate early gene imaging, may provide a pivotal way to identify brain regions that have been overlooked previously due to technical difficulty or lack of interest that are critical for a given behavior or disease state. These types of imaging data can be studied in greater detail using network-based approaches.
In neuroscience, network-based approaches offer unique ways to assess neural activity at a brain-wide scale that may be critical for identifying aspects of disease, such as, such as improvement of treatment methods and identification of biomarkers (Lydon-Staley and Bassett, 2018; Zhang et al., 2020). Network neuroscience can be used to assess the structural or functional connectivity of the brain. Structural networks measure the physical connectivity of brain regions (i.e., do two brain regions have physical connections for direct communication) by identifying fiber tracts and axonal connections, whereas functional networks examine the correlative connectivity of neural activity between regions (i.e., are two brain regions usually activated simultaneously in a given state, suggesting direct or indirect communication) in an unbiased manner (Bullmore and Sporns, 2009; Vertes et al., 2012; Bassett and Sporns, 2017).
Networks measuring functional connectivity, using graph theory, can be employed to identify specific features of neural networks in more detail. Graph theory can be applied to neural network data across multiple levels (e.g., whole brain, regions, circuits, neurons). This approach models the pairwise relations between nodes, through connected edges (Sporns, 2018). When modeling the brain, the nodes can be individual neurons or specific anatomical brain regions, allowing for the scale that is often lost using traditional recording techniques. Edges are defined as the functional connectivity between neurons or brain regions, as measured by correlation of neural activity.
Networks can be divided into modules (i.e., groups brain regions) of nodes (i.e., brain regions) that may share specific neural functions (Meunier et al., 2010). For instance, the nucleus accumbens and ventral tegmental area are both involved in reward processes and could be grouped together in a reward module as such for a given brain state. In human data, many neural networks show small-worldness and modular organization (Bassett and Bullmore, 2006; Sporns and Betzel, 2016). The “world” of a network is termed as “small” if the average number of connections between nodes (geodesic distance) is small relative to the total number of nodes within the network (Achard et al., 2006; Humphries and Gurney, 2008; Muldoon et al., 2016; Bassett and Bullmore, 2017). This means that most nodes are not connected to each other but are connected indirectly through the overall network through only a few connections. In contrast a highly modular network contains a large number of interconnected nodes and few intra-connected nodes (Stam, 2004; Ahn et al., 2010; Sporns and Betzel, 2016). A graphical representation of small-world networks and highly modular networks can be found in Figure 2A. To simplify these complex datasets, machine learning can be used. Machine and deep learning are among the novel computational methods employed to reduce network complexity. Although an in-depth discussion of these techniques is outside the scope of this review, we recommend Vu et al. (2018); Glaser et al. (2019), and Valliani et al. (2019) for further review of the topic.
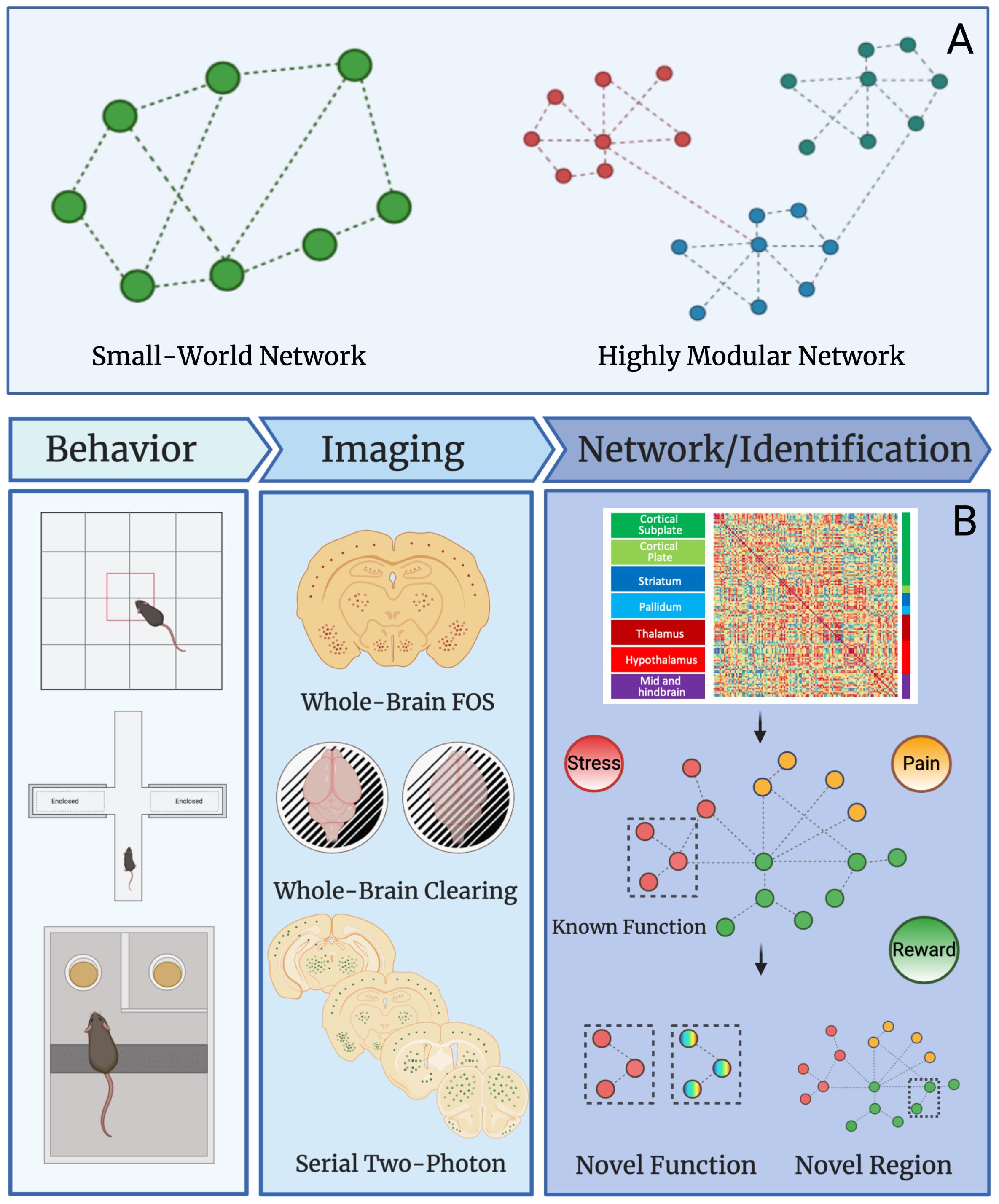
Figure 2. Network properties and uses in preclinical studies. (A) Graphical example of small-world and highly modular networks. (B) Workflow for preclinical network analysis using Fos as a marker for neural activity. Animals first undergo a behavioral, pharmacological, or alternative manipulation to induce neural activity. Brains are collected and then processed to identify Fos protein expression using one of several immunostaining and imaging strategies. The main strategies are: (1) traditional immunohistochemistry and microscopy on Fos stained sliced brain tissue, (2) whole brain immunostaining/clearing of Fos and light-sheet microscopy, and (3) serial two-photon imaging of fluorescent brain slices. Once data is collected from any given imaging strategy, functional connectivity networks can be delineated by calculating Pearson correlations of Fos activity from one brain region to another brain region across animals in a given treatment group. This is done for all brain regions expressing Fos to create a functional connectivity matrix. The functional connectivity matrix can be used to create a modular network that contains brain regions grouped based on function (e.g., stress, pain, or reward). Network analysis can then reveal novel functions for brain regions with other known roles and additionally, the function of overlooked and understudied brain regions can be identified. This workflow will be useful for identifying novel brain regions that contribute to neuropsychiatric diseases in the future.
Graph theory can be used illuminate other intrinsic qualities of the network, such as overall network efficiency (how easily information is exchanged between nodes) and node centrality (the most influential component of a node). In this context, the efficiency of a given network is characterized by the average of the shortest path lengths between any set of nodes (Sporns et al., 2004; Bassett and Bullmore, 2006). Node centrality is the output of the relative importance of a node for a given network. Centrality looks at several measurements such as degree, efficiency (Achard and Bullmore, 2007; Joyce et al., 2010), closeness, and betweenness of each node (Wang et al., 2010). Nodes with a high number of connections (lower path length) and that are central to the network (most important) are considered hubs. Groupings of important hubs with many connects are grouped together in something called a “rich club” to identify high-importance nodes (Guimera et al., 2005; Sporns et al., 2007; Vertes et al., 2014).
A network hub is a node with high intramodule connectivity (a provincial hub), high intermodule connectivity (a connector hub), or both high intra- and inter- module connectivity (a dual hub) (Guimera and Nunes Amaral, 2005; Guimera et al., 2005; Joyce et al., 2010; Stevens et al., 2012; Pedersen et al., 2020). In the context of the brain, hub brain regions represent the highest level of connectivity and are thought to be critical to the function of the neural network (Sporns et al., 2007; Rubinov and Sporns, 2010; Wheeler et al., 2013; Vetere et al., 2017; Kimbrough et al., 2020; Pedersen et al., 2020). Importantly, hubs identified to be crucial in neural networks have been demonstrated to be conserved across species and scales (Arnatkeviciute et al., 2019), both validating the use of the model and suggesting the importance of assessment of networks in disease states across species. To determine activity, immediate early genes have been leveraged, of which Fos is most widely recognized as a marker of activity. The nuclear staining of Fos also makes it an easy marker for imaging and computational analysis.
A key feature of imaging approaches paired with network analysis, whether in clinical or preclinical studies is the unbiased nature of the brain-wide activity being assessed, which can uncover important connectivity and function of the brain without relying on a priori selection of a specific circuit or region to study. Clinical and preclinical studies focused on neuropsychiatric disorders and other brain diseases can greatly benefit from taking advantage of network neuroscience to determine important connections between brain regions and potential hubs associated with a given brain state. Identification of critical hub brain regions within a given disease state help to delineate novel signaling pathways and find potential brain regions and/or therapeutic treatments that were previously overlooked.
Applications of Network Neuroscience in the Treatment and Study of Disease
The primary application of network neuroscience over the last 10 years has been in human studies. One of the main ways to study functional connectivity of the human brain has been through R-fMRI, which can assess differences in default mode network (DMN) function among groups (Gonen et al., 2020). R-fMRI is not without issues, however, as there is a need to standardize the methods among research groups (Dinis Fernandes et al., 2020) and to determine the importance of time-varying functional connectivity measured at rest (Lurie et al., 2020). Using fMRI and DTI scans Akiki et al. (2018) described DMN abnormalities in PTSD patients through a novel network restricted topology approach. They combined fMRI, DTI, and graph theory to systematically examine DMN connectivity and its relationship with PTSD symptom severity. DMN abnormalities were observed in patients with severe PTSD and computational analysis revealed decreased overall interconnections within this group. Other neuropsychiatric disorders such as depression (Jacob et al., 2020), schizophrenia (Whitfield-Gabrieli et al., 2018) and anxiety (Qiao et al., 2017) have been studied similarly. Clinical approaches using machine and deep learning are applied to data collection in prior studies to identify novel markers and disease states. Jo et al. compiled functional scans of brains from Alzheimer’s disease patients and were able to develop a model to predict Alzheimer’s disease progression with a high level of confidence (Jo et al., 2019). Machine learning has also been applied to comorbid psychiatric disease that are difficult to tease apart such as anxiety and depression. This analysis clarifies distinct behavioral measures that contribute to the prediction and crucial mechanisms in one condition vs. the other (Richter et al., 2020).
Network neuroscience has been recently been applied to study preclinical animal models of disease. In preclinical research brain-wide neural networks can be assessed by pairing brain imaging techniques such as fMRI, traditional immunohistochemistry, single-cell whole-brain imaging, and two-photon imaging with network analysis. Using fMRI for preclinical network analysis, Gass et al. interrogated the difference in neural network reorganization between stress-resilient rats and stress-sensitive rats (Gass et al., 2016). This study identified alterations in the role of hubs in a default-mode-like-network sensitive vs. resilient rats that uncovered novel internodal shifts that would have been undetectable using traditional methods.
Calcium imaging is appropriate for the preclinical exploration of the brain; however, it is not applicable to clinical studies in humans. To overcome this issue, the most commonly used clinical neuroimaging techniques are MRI, fMRI, and DTI, which are all relatively fast and non-invasive (Kwong et al., 1992; Basser et al., 1994; Glover, 2011). Used in combination with traditional MRI, DTI extends the imaging capability of the whole brain in vivo. These approaches are beginning to bridge the gap between clinical and preclinical studies, enabling comparison of functional brain activity in human subjects as well as preclinical animal models (Denic et al., 2011; Glover, 2011).
Although preclinical calcium and fMRI imaging provide near instant time resolution, the resolution of individual brain regions is greatly reduced. Further, animals often need to be head fixed or anesthetized in order to record activity data, which greatly limits the ability to assess activity during complex behavioral tasks. Thus, there is value in taking advantage of postmortem immediate early gene protein signaling measurements of neural activity (e.g., tissue clearing techniques and two-photon imaging) to examine brain-wide neural activity.
In addition to the foundational studies focused on whole-brain imaging of Fos activity (Osten and Margrie, 2013; Renier et al., 2014, 2016), others have begun to combine Fos measures with functional connectivity and network analysis to assess activity in the brain. Traditional quantification of brain regions by staining for Fos after fear conditioning was used to establish a neural network associated with fear memory (Wheeler et al., 2013). Further assessment of the fear memory network using functional chemogenetic silencing of different network nodes in vivo aided in the identification of a novel causal role of the reuniens and laterodorsal thalamic nucleus in behavior for key hub brain regions predicted by network models (Vetere et al., 2017). Additionally, graph theory was applied to predict the influence of the hippocampus in driving transitions between non-dependent and dependent states leveraging a control theoretic approach (Brynildsen et al., 2020). Figure 2B represents the workflow from behavior through novel function and region detection. These studies provided a blueprint for using Fos immunostaining in network models that could be extended to the whole brain with advances in brain-wide imaging. There are some caveats to the use of Fos as a marker of activity. Not all immediate early genes are expressed similarly by all neurons (Gallo et al., 2018). Other immediate early genes such as Egr1 and Arc have been demonstrated to have distinct patterns on expression from Fos, and exhibit different timescales from the initiation of activity (Milbrandt, 1987). The present review has highlighted how network-based approaches in preclinical models of disease can examine brain-wide neural activity in an unbiased manner, which will help identify critical brain regions that may have been overlooked previously.
Recently, the combination of iDISCO+, light-sheet microscopy, functional connectivity, and graph theory has been used to reveal the neural network involved in alcohol abstinence. A massive increase in functional coactivation among brain regions and reduced modular structuring of the brain was found in the alcohol abstinence network (Kimbrough et al., 2020). Further, the study by Kimbrough et al. identified critical hub brain regions, validating regions known to play a role in alcohol withdrawal (e.g., the central amygdala; Gilpin et al., 2015) and identified some regions such as the parasubthalamic nucleus, tuberal nucleus, cortical amygdala, and intercalated amygdala that has been overlooked in alcohol studies and need more detailed research (Kimbrough et al., 2020). Combining single-cell whole-brain imaging approaches of immediate early gene immunostaining (e.g., iDISCO/light-sheet microscopy, traditional immunohistochemistry, or serial two-photon imaging) with network analysis can be used to identify novel brain regions of interest for various disease states and neuropsychiatric disorders that were previously overlooked, and also alternative functions for known regions that may contribute to disease progression (Figure 2).
As network neuroscience approaches develop, the way to analyze imaging data of disease states from the clinic has expanded. Neural networks provide unveil previously hidden contributors to disease states (Lydon-Staley and Bassett, 2018; Zhang et al., 2020). Neural network analysis of humans for various neuropsychiatric disorders such as depression, anxiety, post-traumatic stress disorder (PTSD), and Alzheimer’s disease have uncovered contributions of the brain to the disorders (Bashyal, 2005; Jo et al., 2019).
Discussion
Past research in neuroscience has been heavily skewed to focus on a handful of major groups of brain regions and circuits (Figure 1). The use of single-cell whole-brain imaging combined with network analysis in preclinical models is a valuable tool moving forward in neuroscience research, especially when taking advantage of the synergy with other recently developed preclinical technologies. For example, pharmacological, chemogenetic, and optogenetics approaches can be combined with single-cell whole-brain imaging to examine how the modification of specific cell types or brain regions impact the connectivity and granularity of each hub within the whole brain. This approach provides a platform for testing brain regions identified by network analysis as potential hubs for a causal role in behavioral output. Additionally, the combination of single-cell whole-brain imaging and chemogenetics or optogenetics allows for modifying regions known to play a role in a given behavior to explore the interconnectedness of brain regions associated with the specific circuit but that is at a tertiary, quaternary, or further connection away from the region of interest. Functional read-outs such as calcium imaging can be used to elucidate neuron specificity at the cellular and local network levels within a given brain region in combination with the unbiased evaluation of brain-wide networks within the same animals.
There are some caveats to preclinical whole-brain imaging/network analysis approaches. First, depending on the method there will be limitations on the resolution of timescale (in the case of immediate early gene imaging) or brain region specificity (in the case of fMRI and calcium imaging). The issue of resolution suggests that there are benefits to both approaches and they can both prove informative in different aspects of the study of disease. In animal models, fMRI also restricts the type of behavior or brain state that can be examined due to the methods for imaging, whereas using immediate early genes requires postmortem tissue and does not allow for assessment of future behavior/brain activity. Another weakness with preclinical studies is the ability to translate the information from animal models to relevance in human disease states. However, development of methods for comparison of functional brain activity in human subjects with preclinical animal models has helped to alleviate these issues (Denic et al., 2011; Glover, 2011). Furthermore, important hubs identified using neural network methods are maintained across species and scales (Arnatkeviciute et al., 2019). Whole brain imaging and network analysis in preclinical animal models will only improve as time goes on and the issues are minimized.
Despite the limitations discussed above, network-based approaches in preclinical studies have the potential to make significant contributions to our understanding of how the brain functions during behavior and as a result of neuropsychiatric disease. In combination with other modern neuroscience techniques, whole-brain imaging and network analysis may vastly enhance our systems-based understanding of the brain in a way that was not previously available. Importantly, examining network function across the whole brain provides an unbiased examination of brain activity that will help to identify brain regions that are critical for function but have been previously overlooked.
Author Contributions
SS and AK conceptualized the topic and wrote the manuscript. LS, YC, EW, and BA provided background research for the topic. SS designed the figures. SS, YC, EW, LS, OG, and AK edited the manuscript. All authors contributed to the article and approved the submitted version.
Funding
This work was supported by the National Institutes of Health Grants DA051972-0, DA050239, AA026081, AA006420, DA043799, DA044451, AA022977, AA026281, DA047113, and AA027301 and the Tobacco Related Disease Research Program Grant 27IR-0047. The content is solely the responsibility of the authors and does not necessarily represent the official views of the National Institutes of Health.
Conflict of Interest
The authors declare that the research was conducted in the absence of any commercial or financial relationships that could be construed as a potential conflict of interest.
Acknowledgments
The figures were created at Biorender.com. We thank the Preclinical Addiction Research Consortium.
References
Abe, Y., Sekino, M., Terazono, Y., Ohsaki, H., Fukazawa, Y., Sakai, S., et al. (2012). Opto-fMRI analysis for exploring the neuronal connectivity of the hippocampal formation in rats. Neurosci. Res. 74, 248–255. doi: 10.1016/j.neures.2012.08.007
Achard, S., and Bullmore, E. (2007). Efficiency and cost of economical brain functional networks. PLoS Comput. Biol. 3:e17. doi: 10.1371/journal.pcbi.0030017
Achard, S., Salvador, R., Whitcher, B., Suckling, J., and Bullmore, E. (2006). A resilient, low-frequency, small-world human brain functional network with highly connected association cortical hubs. J. Neurosci. 26, 63–72. doi: 10.1523/jneurosci.3874-05.2006
Ahn, Y. Y., Bagrow, J. P., and Lehmann, S. (2010). Link communities reveal multiscale complexity in networks. Nature 466, 761–764. doi: 10.1038/nature09182
Akiki, T. J., Averill, C. L., Wrocklage, K. M., Scott, J. C., Averill, L. A., Schweinsburg, B., et al. (2018). Default mode network abnormalities in posttraumatic stress disorder: a novel network-restricted topology approach. Neuroimage 176, 489–498. doi: 10.1016/j.neuroimage.2018.05.005
Alexander, G. M., Rogan, S. C., Abbas, A. I., Armbruster, B. N., Pei, Y., Allen, J. A., et al. (2009). Remote control of neuronal activity in transgenic mice expressing evolved G protein-coupled receptors. Neuron 63, 27–39. doi: 10.1016/j.neuron.2009.06.014
Allen Institute for Brain Science (2004). Allen Mouse Brain Atlas [Online]. Washington, DC: Allen Institute for Brain Science.
Arenkiel, B. R., Klein, M. E., Davison, I. G., Katz, L. C., and Ehlers, M. D. (2008). Genetic control of neuronal activity in mice conditionally expressing TRPV1. Nat. Methods 5, 299–302. doi: 10.1038/nmeth.1190
Armbruster, B. N., Li, X., Pausch, M. H., Herlitze, S., and Roth, B. L. (2007). Evolving the lock to fit the key to create a family of G protein-coupled receptors potently activated by an inert ligand. Proc. Natl. Acad. Sci. U.S.A. 104, 5163–5168. doi: 10.1073/pnas.0700293104
Arnatkeviciute, A., Fulcher, B. D., and Fornito, A. (2019). Uncovering the transcriptional correlates of hub connectivity in neural networks. Front. Neural Circuits 13:47. doi: 10.3389/fncir.2019.00047
Bakken, T. E., Miller, J. A., Ding, S. L., Sunkin, S. M., Smith, K. A., Ng, L., et al. (2016). A comprehensive transcriptional map of primate brain development. Nature 535, 367–375.
Bashyal, S. (2005). “Classification of psychiatric disorders using artificial neural network,” in Advances in Neural Networks – ISNN 2005, eds J. Wang, X.-F. Liao, and Z. Yi (Berlin: Springer), 796–800. doi: 10.1007/11427469_126
Basser, P. J., Mattiello, J., and Lebihan, D. (1994). MR diffusion tensor spectroscopy and imaging. Biophys. J. 66, 259–267. doi: 10.1016/s0006-3495(94)80775-1
Bassett, D. S., and Bullmore, E. T. (2017). Small-world brain networks revisited. Neuroscientist 23, 499–516. doi: 10.1177/1073858416667720
Beckmann, C. F., Deluca, M., Devlin, J. T., and Smith, S. M. (2005). Investigations into resting-state connectivity using independent component analysis. Philos. Trans. R. Soc. B Biol. Sci. 360, 1001–1013. doi: 10.1098/rstb.2005.1634
Benninger, R. K., and Piston, D. W. (2013). Two-photon excitation microscopy for the study of living cells and tissues. Curr. Protoc. Cell Biol. 4, 4.11.1–4.11.24.
Bernstein, J. G., and Boyden, E. S. (2011). Optogenetic tools for analyzing the neural circuits of behavior. Trends Cogn. Sci. 15, 592–600. doi: 10.1016/j.tics.2011.10.003
Beyeler, M., Rounds, E. L., Carlson, K. D., Dutt, N., and Krichmar, J. L. (2019). Neural correlates of sparse coding and dimensionality reduction. PLoS Comput. Biol. 15:e1006908. doi: 10.1371/journal.pcbi.1006908
Bird, C. M., and Burgess, N. (2008). The hippocampus and memory: insights from spatial processing. Nat. Rev. Neurosci. 9, 182–194. doi: 10.1038/nrn2335
Bishop, A. C., Shah, K., Liu, Y., Witucki, L., Kung, C., and Shokat, K. M. (1998). Design of allele-specific inhibitors to probe protein kinase signaling. Curr. Biol. 8, 257–266. doi: 10.1016/s0960-9822(98)70198-8
Bonifazi, P., and Massobrio, P. (2019). Reconstruction of functional connectivity from multielectrode recordings and calcium imaging. Adv. Neurobiol. 22, 207–231. doi: 10.1007/978-3-030-11135-9_9
Boon, P., Raedt, R., De Herdt, V., Wyckhuys, T., and Vonck, K. (2009). Electrical stimulation for the treatment of epilepsy. Neurotherapeutics 6, 218–227.
Boyden, E. S., Zhang, F., Bamberg, E., Nagel, G., and Deisseroth, K. (2005). Millisecond-timescale, genetically targeted optical control of neural activity. Nat. Neurosci. 8, 1263–1268. doi: 10.1038/nn1525
Brynildsen, J. K., Mace, K. D., Cornblath, E. J., Weidler, C., Pasqualetti, F., Bassett, D. S., et al. (2020). Gene coexpression patterns predict opiate-induced brain-state transitions. Proc. Natl. Acad. Sci. U.S.A. 117, 19556–19565. doi: 10.1073/pnas.2003601117
Buckner, R. L. (2013). The cerebellum and cognitive function: 25 years of insight from anatomy and neuroimaging. Neuron 80, 807–815. doi: 10.1016/j.neuron.2013.10.044
Bullmore, E., and Sporns, O. (2009). Complex brain networks: graph theoretical analysis of structural and functional systems. Nat. Rev. Neurosci. 10, 186–198. doi: 10.1038/nrn2575
Calhoun, V. D., and Adali, T. (2012). Multisubject independent component analysis of fMRI: a decade of intrinsic networks, default mode, and neurodiagnostic discovery. IEEE Rev. Biomed. Eng. 5, 60–73. doi: 10.1109/rbme.2012.2211076
Calhoun, V. D., Adali, T., Pearlson, G. D., and Pekar, J. J. (2001). A method for making group inferences from functional MRI data using independent component analysis. Hum. Brain Mapp. 14, 140–151. doi: 10.1002/hbm.1048
Chen, X., Ye, H., Kuruvilla, R., Ramanan, N., Scangos, K. W., Zhang, C., et al. (2005). A chemical-genetic approach to studying neurotrophin signaling. Neuron 46, 13–21. doi: 10.1016/j.neuron.2005.03.009
Chow, B. Y., Han, X., Dobry, A. S., Qian, X., Chuong, A. S., Li, M., et al. (2010). High-performance genetically targetable optical neural silencing by light-driven proton pumps. Nature 463, 98–102. doi: 10.1038/nature08652
Chung, K., Wallace, J., Kim, S. Y., Kalyanasundaram, S., Andalman, A. S., Davidson, T. J., et al. (2013). Structural and molecular interrogation of intact biological systems. Nature 497, 332–337.
Crimi, A., Giancardo, L., Sambataro, F., Gozzi, A., Murino, V., and Sona, D. (2019). MultiLink analysis: brain network comparison via sparse connectivity analysis. Sci. Rep. 9:65.
Cunningham, J. P., and Yu, B. M. (2014). Dimensionality reduction for large-scale neural recordings. Nat. Neurosci. 17, 1500–1509. doi: 10.1038/nn.3776
Deisseroth, K., Feng, G., Majewska, A. K., Miesenbock, G., Ting, A., and Schnitzer, M. J. (2006). Next-generation optical technologies for illuminating genetically targeted brain circuits. J. Neurosci. 26, 10380–10386. doi: 10.1523/jneurosci.3863-06.2006
Denic, A., Macura, S. I., Mishra, P., Gamez, J. D., Rodriguez, M., and Pirko, I. (2011). MRI in rodent models of brain disorders. Neurotherapeutics 8, 3–18. doi: 10.1007/s13311-010-0002-4
Denk, W., Strickler, J. H., and Webb, W. W. (1990). Two-photon laser scanning fluorescence microscopy. Science 248, 73–76. doi: 10.1126/science.2321027
Dinis Fernandes, C., Varsou, O., Stringer, M., Macleod, M. J., and Schwarzbauer, C. (2020). Scanning conditions in functional connectivity magnetic resonance imaging: how to standardise resting-state for optimal data acquisition and visualisation? Adv. Exp. Med. Biol. 1235, 35–52. doi: 10.1007/978-3-030-37639-0_3
Erturk, A., Becker, K., Jahrling, N., Mauch, C. P., Hojer, C. D., Egen, J. G., et al. (2012). Three-dimensional imaging of solvent-cleared organs using 3disco. Nat. Protoc. 7, 1983–1995. doi: 10.1038/nprot.2012.119
Erturk, A., Mauch, C. P., Hellal, F., Forstner, F., Keck, T., Becker, K., et al. (2011). Three-dimensional imaging of the unsectioned adult spinal cord to assess axon regeneration and glial responses after injury. Nat. Med. 18, 166–171. doi: 10.1038/nm.2600
Fishman, P. S., and Frenkel, V. (2017). Focused ultrasound: an emerging therapeutic modality for neurologic disease. Neurotherapeutics 14, 393–404. doi: 10.1007/s13311-017-0515-1
Fry, W. J., Barnard, J. W., Fry, E. J., Krumins, R. F., and Brennan, J. F. (1955). Ultrasonic lesions in the mammalian central nervous system. Science 122, 517–518. doi: 10.1126/science.122.3168.517
Fu, Y. Y., and Tang, S. C. (2010). Optical clearing facilitates integrated 3D visualization of mouse ileal microstructure and vascular network with high definition. Microvasc. Res. 80, 512–521. doi: 10.1016/j.mvr.2010.06.003
Gallo, F. T., Katche, C., Morici, J. F., Medina, J. H., and Weisstaub, N. V. (2018). Immediate early genes, memory and psychiatric disorders: focus on c-Fos, Egr1 and Arc. Front. Behav. Neurosci. 12:79. doi: 10.3389/fnbeh.2018.00079
Gass, N., Becker, R., Schwarz, A. J., Weber-Fahr, W., Clemm Von Hohenberg, C., Vollmayr, B., et al. (2016). Brain network reorganization differs in response to stress in rats genetically predisposed to depression and stress-resilient rats. Transl. Psychiatry 6:e970. doi: 10.1038/tp.2016.233
Gilpin, N. W., Herman, M. A., and Roberto, M. (2015). The central amygdala as an integrative hub for anxiety and alcohol use disorders. Biol. Psychiatry 77, 859–869. doi: 10.1016/j.biopsych.2014.09.008
Glaser, J. I., Benjamin, A. S., Farhoodi, R., and Kording, K. P. (2019). The roles of supervised machine learning in systems neuroscience. Prog. Neurobiol. 175, 126–137. doi: 10.1016/j.pneurobio.2019.01.008
Glover, G. H. (2011). Overview of functional magnetic resonance imaging. Neurosurg. Clin. N. Am. 22, 133–139.
Gonen, O. M., Kwan, P., O’brien, T. J., Lui, E., and Desmond, P. M. (2020). Resting-state functional MRI of the default mode network in epilepsy. Epilepsy Behav. 111:107308. doi: 10.1016/j.yebeh.2020.107308
Gradinaru, V., Thompson, K. R., and Deisseroth, K. (2008). eNpHR: a Natronomonas halorhodopsin enhanced for optogenetic applications. Brain Cell Biol. 36, 129–139. doi: 10.1007/s11068-008-9027-6
Guettier, J. M., Gautam, D., Scarselli, M., Ruiz De Azua, I., Li, J. H., Rosemond, E., et al. (2009). A chemical-genetic approach to study G protein regulation of beta cell function in vivo. Proc. Natl. Acad. Sci. U.S.A. 106, 19197–19202. doi: 10.1073/pnas.0906593106
Guimera, R., Mossa, S., Turtschi, A., and Amaral, L. A. (2005). The worldwide air transportation network: anomalous centrality, community structure, and cities’ global roles. Proc. Natl. Acad. Sci. U.S.A. 102, 7794–7799. doi: 10.1073/pnas.0407994102
Guimera, R., and Nunes Amaral, L. A. (2005). Functional cartography of complex metabolic networks. Nature 433, 895–900. doi: 10.1038/nature03288
Hawrylycz, M. J., Lein, E. S., Guillozet-Bongaarts, A. L., Shen, E. H., Ng, L., Miller, J. A., et al. (2012). An anatomically comprehensive atlas of the adult human brain transcriptome. Nature 489, 391–399.
Hinton, G. E., and Salakhutdinov, R. R. (2006). Reducing the dimensionality of data with neural networks. Science 313:504. doi: 10.1126/science.1127647
Hou, B., Zhang, D., Zhao, S., Wei, M., Yang, Z., Wang, S., et al. (2015). Scalable and DiI-compatible optical clearance of the mammalian brain. Front. Neuroanat. 9:19. doi: 10.3389/fnana.2015.00019
Humphries, M. D., and Gurney, K. (2008). Network ‘small-world-ness’: a quantitative method for determining canonical network equivalence. PLoS One 3:e0002051. doi: 10.1371/journal.pone.0002051
Jacob, Y., Morris, L. S., Huang, K. H., Schneider, M., Rutter, S., Verma, G., et al. (2020). Neural correlates of rumination in major depressive disorder: a brain network analysis. Neuroimage Clin. 25:102142. doi: 10.1016/j.nicl.2019.102142
Jo, T., Nho, K., and Saykin, A. J. (2019). Deep learning in Alzheimer’s disease: diagnostic classification and prognostic prediction using neuroimaging data. Front. Aging Neurosci. 11:220. doi: 10.3389/fnagi.2019.00220
Joyce, K. E., Laurienti, P. J., Burdette, J. H., and Hayasaka, S. (2010). A new measure of centrality for brain networks. PLoS One 5:e12200. doi: 10.1371/journal.pone.0012200
Ke, M. T., Fujimoto, S., and Imai, T. (2013). Seedb: a simple and morphology-preserving optical clearing agent for neuronal circuit reconstruction. Nat. Neurosci. 16, 1154–1161. doi: 10.1038/nn.3447
Kim, S. Y., Adhikari, A., Lee, S. Y., Marshel, J. H., Kim, C. K., Mallory, C. S., et al. (2013). Diverging neural pathways assemble a behavioural state from separable features in anxiety. Nature 496, 219–223. doi: 10.1038/nature12018
Kimbrough, A., Lurie, D. J., Collazo, A., Kreifeldt, M., Sidhu, H., Macedo, G. C., et al. (2020). Brain-wide functional architecture remodeling by alcohol dependence and abstinence. Proc. Natl. Acad. Sci. U.S.A. 117, 2149–2159. doi: 10.1073/pnas.1909915117
Kwong, K. K., Belliveau, J. W., Chesler, D. A., Goldberg, I. E., Weisskoff, R. M., Poncelet, B. P., et al. (1992). Dynamic magnetic resonance imaging of human brain activity during primary sensory stimulation. Proc. Natl. Acad. Sci. U.S.A. 89, 5675–5679. doi: 10.1073/pnas.89.12.5675
Lee, J. H. (2012). Informing brain connectivity with optogenetic functional magnetic resonance imaging. Neuroimage 62, 2244–2249. doi: 10.1016/j.neuroimage.2012.01.116
Lee, J. H., Durand, R., Gradinaru, V., Zhang, F., Goshen, I., Kim, D. S., et al. (2010). Global and local fmri signals driven by neurons defined optogenetically by type and wiring. Nature 465, 788–792. doi: 10.1038/nature09108
Lein, E. S., Hawrylycz, M. J., Ao, N., Ayres, M., Bensinger, A., Bernard, A., et al. (2018). Optogenetic auditory fMRI reveals the effects of visual cortical inputs on auditory midbrain response. Sci. Rep. 8:8736.
Lerchner, W., Xiao, C., Nashmi, R., Slimko, E. M., Van Trigt, L., Lester, H. A., et al. (2007). Reversible silencing of neuronal excitability in behaving mice by a genetically targeted, ivermectin-gated Cl- channel. Neuron 54, 35–49. doi: 10.1016/j.neuron.2007.02.030
Lewis, C. M., Bosman, C. A., and Fries, P. (2015). Recording of brain activity across spatial scales. Curr. Opin. Neurobiol. 32, 68–77. doi: 10.1016/j.conb.2014.12.007
Lin, P., Fang, Z., Liu, J., and Lee, J. H. (2016). Optogenetic functional MRI. J. Vis. Exp. 2016:53346.
Lurie, D. J., Kessler, D., Bassett, D. S., Betzel, R. F., Breakspear, M., Kheilholz, S., et al. (2020). Questions and controversies in the study of time-varying functional connectivity in resting fMRI. Netw. Neurosci. 4, 30–69. doi: 10.1162/netn_a_00116
Lydon-Staley, D. M., and Bassett, D. S. (2018). Network neuroscience: a framework for developing biomarkers in psychiatry. Curr. Top. Behav. Neurosci. 40, 79–109. doi: 10.1007/7854_2018_41
Lynn, J. G., Zwemer, R. L., Chick, A. J., and Miller, A. E. (1942). A new method for the generation and use of focused ultrasound in experimental biology. J. Gen. Physiol. 26, 179–193. doi: 10.1085/jgp.26.2.179
Magnus, C. J., Lee, P. H., Atasoy, D., Su, H. H., Looger, L. L., and Sternson, S. M. (2011). Chemical and genetic engineering of selective ion channel-ligand interactions. Science 333, 1292–1296. doi: 10.1126/science.1206606
Mao, B. Q., Hamzei-Sichani, F., Aronov, D., Froemke, R. C., and Yuste, R. (2001). Dynamics of spontaneous activity in neocortical slices. Neuron 32, 883–898. doi: 10.1016/s0896-6273(01)00518-9
Matsumoto, K., Mitani, T. T., Horiguchi, S. A., Kaneshiro, J., Murakami, T. C., Mano, T., et al. (2019). Advanced Cubic tissue clearing for whole-organ cell profiling. Nat. Protoc. 14, 3506–3537. doi: 10.1038/s41596-019-0240-9
Mckeown, M. J., Hansen, L. K., and Sejnowsk, T. J. (2003). Independent component analysis of functional MRI: what is signal and what is noise? Curr. Opin. Neurobiol. 13, 620–629. doi: 10.1016/j.conb.2003.09.012
Mckeown, M. J., Makeig, S., Brown, G. G., Jung, T. P., Kindermann, S. S., Bell, A. J., et al. (1998). Analysis of fMRI data by blind separation into independent spatial components. Hum. Brain Mapp. 6, 160–188. doi: 10.1002/(sici)1097-0193(1998)6:3<160::aid-hbm5>3.0.co;2-1
Mesina, L., Wilber, A. A., Clark, B. J., Dube, S., Demecha, A. J., Stark, C. E., et al. (2016). A methodological pipeline for serial-section imaging and tissue realignment for whole-brain functional and connectivity assessment. J. Neurosci. Methods 266, 151–160. doi: 10.1016/j.jneumeth.2016.03.021
Meunier, D., Lambiotte, R., and Bullmore, E. T. (2010). Modular and hierarchically modular organization of brain networks. Front. Neurosci. 4:200. doi: 10.3389/fnins.2010.00200
Miesenbock, G. (2009). The optogenetic catechism. Science 326, 395–399. doi: 10.1126/science.1174520
Milbrandt, J. (1987). A nerve growth factor-induced gene encodes a possible transcriptional regulatory factor. Science 238:797. doi: 10.1126/science.3672127
Milner, B., Squire, L. R., and Kandel, E. R. (1998). Cognitive neuroscience and the study of memory. Neuron 20, 445–468.
Muldoon, S. F., Bridgeford, E. W., and Bassett, D. S. (2016). Small-world propensity and weighted brain networks. Sci. Rep. 6:22057.
Muntifering, M., Castranova, D., Gibson, G. A., Meyer, E., Kofron, M., and Watson, A. M. (2018). Clearing for deep tissue imaging. Curr. Protoc. Cytom. 86:e38. doi: 10.1002/cpcy.38
Murakami, T. C., Mano, T., Saikawa, S., Horiguchi, S. A., Shigeta, D., Baba, K., et al. (2018). A three-dimensional single-cell-resolution whole-brain atlas using Cubic-X expansion microscopy and tissue clearing. Nat. Neurosci. 21, 625–637. doi: 10.1038/s41593-018-0109-1
Mwangi, B., Tian, T. S., and Soares, J. C. (2014). A review of feature reduction techniques in neuroimaging. Neuroinformatics 12, 229–244. doi: 10.1007/s12021-013-9204-3
Nicolelis, M. A., and Ribeiro, S. (2002). Multielectrode recordings: the next steps. Curr. Opin. Neurobiol. 12, 602–606. doi: 10.1016/s0959-4388(02)00374-4
Oh, S. W., Harris, J. A., Ng, L., Winslow, B., Cain, N., Mihalas, S., et al. (2005). Functional imaging with cellular resolution reveals precise micro-architecture in visual cortex. Nature 433, 597–603. doi: 10.1038/nature03274
Osten, P., and Margrie, T. W. (2013). Mapping brain circuitry with a light microscope. Nat. Methods 10, 515–523. doi: 10.1038/nmeth.2477
Ozbay, B. N., Futia, G. L., Ma, M., Bright, V. M., Gopinath, J. T., Hughes, E. G., et al. (2018). Three dimensional two-photon brain imaging in freely moving mice using a miniature fiber coupled microscope with active axial-scanning. Sci. Rep. 8:8108.
Park, Y. G., Sohn, C. H., Chen, R., Mccue, M., Yun, D. H., Drummond, G. T., et al. (2018). Protection of tissue physicochemical properties using polyfunctional crosslinkers. Nat. Biotechnol. [Epub ahead of print].
Pedersen, M., Omidvarnia, A., Shine, J. M., Jackson, G. D., and Zalesky, A. (2020). Reducing the influence of intramodular connectivity in participation coefficient. Netw. Neurosci. 4, 416–431. doi: 10.1162/netn_a_00127
Qiao, J., Li, A., Cao, C., Wang, Z., Sun, J., and Xu, G. (2017). Aberrant functional network connectivity as a biomarker of generalized anxiety disorder. Front. Hum. Neurosci. 11:626. doi: 10.3389/fnhum.2017.00626
Rabut, C., Correia, M., Finel, V., Pezet, S., Pernot, M., Deffieux, T., et al. (2019). 4D functional ultrasound imaging of whole-brain activity in rodents. Nat. Methods 16, 994–997. doi: 10.1038/s41592-019-0572-y
Ragan, T., Kadiri, L. R., Venkataraju, K. U., Bahlmann, K., Sutin, J., Taranda, J., et al. (2012). Serial two-photon tomography for automated ex vivo mouse brain imaging. Nat. Methods 9, 255–258. doi: 10.1038/nmeth.1854
Redfern, C. H., Coward, P., Degtyarev, M. Y., Lee, E. K., Kwa, A. T., Hennighausen, L., et al. (1999). Conditional expression and signaling of a specifically designed Gi-coupled receptor in transgenic mice. Nat. Biotechnol. 17, 165–169. doi: 10.1038/6165
Renier, N., Adams, E. L., Kirst, C., Wu, Z., Azevedo, R., Kohl, J., et al. (2016). Mapping of brain activity by automated volume analysis of immediate early genes. Cell 165, 1789–1802. doi: 10.1016/j.cell.2016.05.007
Renier, N., Wu, Z., Simon, D. J., Yang, J., Ariel, P., and Tessier-Lavigne, M. (2014). iDISCO: a simple, rapid method to immunolabel large tissue samples for volume imaging. Cell 159, 896–910. doi: 10.1016/j.cell.2014.10.010
Richardson, D. S., and Lichtman, J. W. (2015). Clarifying tissue clearing. Cell 162, 246–257. doi: 10.1016/j.cell.2015.06.067
Richter, T., Fishbain, B., Markus, A., Richter-Levin, G., and Okon-Singer, H. (2020). Using machine learning-based analysis for behavioral differentiation between anxiety and depression. Sci. Rep. 10:16381.
Roth, B. L. (2016). Dreadds for neuroscientists. Neuron 89, 683–694. doi: 10.1016/j.neuron.2016.01.040
Rubinov, M., and Sporns, O. (2010). Complex network measures of brain connectivity: uses and interpretations. Neuroimage 52, 1059–1069. doi: 10.1016/j.neuroimage.2009.10.003
Scoville, W. B., and Milner, B. (1957). Loss of recent memory after bilateral hippocampal lesions. J. Neurol. Neurosurg. Psychiatry 20, 11–21. doi: 10.1136/jnnp.20.1.11
Silvestri, L., Costantini, I., Sacconi, L., and Pavone, F. S. (2016). Clearing of fixed tissue: a review from a microscopist’s perspective. J. Biomed. Opt. 21:081205. doi: 10.1117/1.jbo.21.8.081205
Sporns, O. (2018). Graph theory methods: applications in brain networks. Dialog. Clin. Neurosci. 20, 111–121. doi: 10.31887/dcns.2018.20.2/osporns
Sporns, O., and Betzel, R. F. (2016). Modular brain networks. Annu. Rev. Psychol. 67, 613–640. doi: 10.1146/annurev-psych-122414-033634
Sporns, O., Chialvo, D. R., Kaiser, M., and Hilgetag, C. C. (2004). Organization, development and function of complex brain networks. Trends Cogn. Sci. 8, 418–425. doi: 10.1016/j.tics.2004.07.008
Sporns, O., Honey, C. J., and Kotter, R. (2007). Identification and classification of hubs in brain networks. PLoS One 2:e1049. doi: 10.1371/journal.pone.0001049
Stam, C. J. (2004). Functional connectivity patterns of human magnetoencephalographic recordings: a ‘small-world’ network? Neurosci. Lett. 355, 25–28. doi: 10.1016/j.neulet.2003.10.063
Sternson, S. M. (2013). Hypothalamic survival circuits: blueprints for purposive behaviors. Neuron 77, 810–824. doi: 10.1016/j.neuron.2013.02.018
Sternson, S. M., and Roth, B. L. (2014). Chemogenetic tools to interrogate brain functions. Annu. Rev. Neurosci. 37, 387–407. doi: 10.1146/annurev-neuro-071013-014048
Stevens, A. A., Tappon, S. C., Garg, A., and Fair, D. A. (2012). Functional brain network modularity captures inter- and intra-individual variation in working memory capacity. PLoS One 7:e30468. doi: 10.1371/journal.pone.0030468
Strader, C. D., Gaffney, T., Sugg, E. E., Candelore, M. R., Keys, R., Patchett, A. A., et al. (1991). Allele-specific activation of genetically engineered receptors. J. Biol. Chem. 266, 5–8. doi: 10.1016/s0021-9258(18)52392-9
Susaki, E. A., Tainaka, K., Perrin, D., Yukinaga, H., Kuno, A., and Ueda, H. R. (2015). Advanced CUBIC protocols for whole-brain and whole-body clearing and imaging. Nat. Protoc. 10, 1709–1727. doi: 10.1038/nprot.2015.085
Susaki, E. A., and Ueda, H. R. (2016). Whole-body and whole-organ clearing and imaging techniques with single-cell resolution: toward organism-level systems biology in mammals. Cell Chem. Biol. 23, 137–157. doi: 10.1016/j.chembiol.2015.11.009
Swanson, L. W. (2000). Cerebral hemisphere regulation of motivated behavior. Brain Res. 886, 113–164. doi: 10.1016/s0006-8993(00)02905-x
Tainaka, K., Murakami, T. C., Susaki, E. A., Shimizu, C., Saito, R., Takahashi, K., et al. (2018). Chemical landscape for tissue clearing based on hydrophilic reagents. Cell. Rep. 24, 2196.e–2210.e.
Thompson, C. L., Varnam, L. R., Visel, A., Whitlock, R. M., Wohnoutka, P. E., Wolkey, C. K., et al. (2007). Genome-wide atlas of gene expression in the adult mouse brain. Nature 445, 168–176.
Tiran, E., Deffieux, T., Correia, M., Maresca, D., Osmanski, B. F., Sieu, L. A., et al. (2015). Multiplane wave imaging increases signal-to-noise ratio in ultrafast ultrasound imaging. Phys. Med. Biol. 60, 8549–8566. doi: 10.1088/0031-9155/60/21/8549
Tsien, R. Y. (1988). Fluorescence measurement and photochemical manipulation of cytosolic free calcium. Trends Neurosci. 11, 419–424. doi: 10.1016/0166-2236(88)90192-0
Tye, K. M., Mirzabekov, J. J., Warden, M. R., Ferenczi, E. A., Tsai, H. C., Finkelstein, J., et al. (2013). Dopamine neurons modulate neural encoding and expression of depression-related behaviour. Nature 493, 537–541. doi: 10.1038/nature11740
Tye, K. M., Prakash, R., Kim, S. Y., Fenno, L. E., Grosenick, L., Zarabi, H., et al. (2011). Amygdala circuitry mediating reversible and bidirectional control of anxiety. Nature 471, 358–362. doi: 10.1038/nature09820
Urban, D. J., Zhu, H., Marcinkiewcz, C. A., Michaelides, M., Oshibuchi, H., Rhea, D., et al. (2016). Elucidation of the behavioral program and neuronal network encoded by dorsal raphe serotonergic neurons. Neuropsychopharmacology 41, 1404–1415. doi: 10.1038/npp.2015.293
Valliani, A. A., Ranti, D., and Oermann, E. K. (2019). Deep learning and neurology: a systematic review. Neurol. Ther. 8, 351–365. doi: 10.1007/s40120-019-00153-8
Vardy, E., Robinson, J. E., Li, C., Olsen, R. H. J., Diberto, J. F., Giguere, P. M., et al. (2015). A new dreadd facilitates the multiplexed chemogenetic interrogation of behavior. Neuron 86, 936–946. doi: 10.1016/j.neuron.2015.03.065
Vertes, P. E., Alexander-Bloch, A., and Bullmore, E. T. (2014). Generative models of rich clubs in Hebbian neuronal networks and large-scale human brain networks. Philos. Trans. R. Soc. Lond. B Biol. Sci. 369:20130531. doi: 10.1098/rstb.2013.0531
Vertes, P. E., Alexander-Bloch, A. F., Gogtay, N., Giedd, J. N., Rapoport, J. L., and Bullmore, E. T. (2012). Simple models of human brain functional networks. Proc. Natl. Acad. Sci. U.S.A. 109, 5868–5873.
Vetere, G., Kenney, J. W., Tran, L. M., Xia, F., Steadman, P. E., Parkinson, J., et al. (2017). Chemogenetic interrogation of a brain-wide fear memory network in mice. Neuron 94:e4.
Vigouroux, R. J., Belle, M., and Chedotal, A. (2017). Neuroscience in the third dimension: shedding new light on the brain with tissue clearing. Mol. Brain 10:33.
Vu, M. T., Adali, T., Ba, D., Buzsaki, G., Carlson, D., Heller, K., et al. (2018). A shared vision for machine learning in neuroscience. J. Neurosci. 38, 1601–1607. doi: 10.1523/jneurosci.0508-17.2018
Wang, J., Zuo, X., and He, Y. (2010). Graph-based network analysis of resting-state functional MRI. Front. Syst. Neurosci. 4:16. doi: 10.3389/fnsys.2010.00016
Weitz, A. J., and Lee, J. H. (2013). Progress with optogenetic functional MRI and its translational implications. Future Neurol. 8, 691–700. doi: 10.2217/fnl.13.42
Wheeler, A. L., Teixeira, C. M., Wang, A. H., Xiong, X., Kovacevic, N., Lerch, J. P., et al. (2013). Identification of a functional connectome for long-term fear memory in mice. PLoS Comput. Biol. 9:e1002853. doi: 10.1371/journal.pcbi.1002853
Whissell, P. D., Tohyama, S., and Martin, L. J. (2016). The use of DREADDs to deconstruct behavior. Front. Genet. 7:70. doi: 10.3389/fgene.2016.00070
Whitfield-Gabrieli, S., Fischer, A. S., Henricks, A. M., Khokhar, J. Y., Roth, R. M., Brunette, M. F., et al. (2018). Understanding marijuana’s effects on functional connectivity of the default mode network in patients with schizophrenia and co-occurring cannabis use disorder: a pilot investigation. Schizophr. Res. 194, 70–77. doi: 10.1016/j.schres.2017.07.029
Wohnoutka, P., Gerfen, C. R., Koch, C., Bernard, A., Dang, C., Jones, A. R., et al. (2014). A mesoscale connectome of the mouse brain. Nature 508, 207–214.
Xu, T., Nenning, K. H., Schwartz, E., Hong, S. J., Vogelstein, J. T., Goulas, A., et al. (2020). Cross-species functional alignment reveals evolutionary hierarchy within the connectome. Neuroimage 223:117346. doi: 10.1016/j.neuroimage.2020.117346
Ye, L., Allen, W. E., Thompson, K. R., Tian, Q., Hsueh, B., Ramakrishnan, C., et al. (2016). Wiring and molecular features of prefrontal ensembles representing distinct experiences. Cell 165, 1776–1788. doi: 10.1016/j.cell.2016.05.010
Zangiabadi, N., Ladino, L. D., Sina, F., Orozco-Hernandez, J. P., Carter, A., and Tellez-Zenteno, J. F. (2019). Deep brain stimulation and drug-resistant epilepsy: a review of the literature. Front. Neurol. 10:601. doi: 10.3389/fneur.2019.00601
Zhang, F., Wang, L. P., Boyden, E. S., and Deisseroth, K. (2006). Channelrhodopsin-2 and optical control of excitable cells. Nat. Methods 3, 785–792. doi: 10.1038/nmeth936
Zhang, W., Lv, J., Li, X., Zhu, D., Jiang, X., Zhang, S., et al. (2019). Experimental comparisons of sparse dictionary learning and independent component analysis for brain network inference from fMRI data. IEEE Trans. Biomed. Eng. 66, 289–299. doi: 10.1109/tbme.2018.2831186
Zhang, X., Braun, U., Tost, H., and Bassett, D. S. (2020). Data-driven approaches to neuroimaging analysis to enhance psychiatric diagnosis and therapy. Biol. Psychiatry Cogn. Neurosci. Neuroimaging 5, 780–790. doi: 10.1016/j.bpsc.2019.12.015
Keywords: iDISCO, fMRI, immunohistochemistry, modularity, graph theory, network neuroscience, iDISCO+
Citation: Simpson S, Chen Y, Wellmeyer E, Smith LC, Aragon Montes B, George O and Kimbrough A (2021) The Hidden Brain: Uncovering Previously Overlooked Brain Regions by Employing Novel Preclinical Unbiased Network Approaches. Front. Syst. Neurosci. 15:595507. doi: 10.3389/fnsys.2021.595507
Received: 16 August 2020; Accepted: 26 March 2021;
Published: 21 April 2021.
Edited by:
Regina A. Mangieri, University of Texas at Austin, United StatesReviewed by:
Adrienne Grzenda, University of California, Los Angeles, United StatesRachel J. Smith, Texas A&M University, United States
Copyright © 2021 Simpson, Chen, Wellmeyer, Smith, Aragon Montes, George and Kimbrough. This is an open-access article distributed under the terms of the Creative Commons Attribution License (CC BY). The use, distribution or reproduction in other forums is permitted, provided the original author(s) and the copyright owner(s) are credited and that the original publication in this journal is cited, in accordance with accepted academic practice. No use, distribution or reproduction is permitted which does not comply with these terms.
*Correspondence: Adam Kimbrough, a2ltYnJvdWFAcHVyZHVlLmVkdQ==