- Department of Neurobiology and Anatomy, Wake Forest School of Medicine, Winston-Salem, NC, USA
The prefrontal cortex is activated during working memory, as evidenced by fMRI results in human studies and neurophysiological recordings in animal models. Persistent activity during the delay period of working memory tasks, after the offset of stimuli that subjects are required to remember, has traditionally been thought of as the neural correlate of working memory. In the last few years several findings have cast doubt on the role of this activity. By some accounts, activity in other brain areas, such as the primary visual and posterior parietal cortex, is a better predictor of information maintained in visual working memory and working memory performance; dynamic patterns of activity may convey information without requiring persistent activity at all; and prefrontal neurons may be ill-suited to represent non-spatial information about the features and identity of remembered stimuli. Alternative interpretations about the role of the prefrontal cortex have thus been suggested, such as that it provides a top-down control of information represented in other brain areas, rather than maintaining a working memory trace itself. Here we review evidence for and against the role of prefrontal persistent activity, with a focus on visual neurophysiology. We show that persistent activity predicts behavioral parameters precisely in working memory tasks. We illustrate that prefrontal cortex represents features of stimuli other than their spatial location, and that this information is largely absent from early cortical areas during working memory. We examine memory models not dependent on persistent activity, and conclude that each of those models could mediate only a limited range of memory-dependent behaviors. We review activity decoded from brain areas other than the prefrontal cortex during working memory and demonstrate that these areas alone cannot mediate working memory maintenance, particularly in the presence of distractors. We finally discuss the discrepancy between BOLD activation and spiking activity findings, and point out that fMRI methods do not currently have the spatial resolution necessary to decode information within the prefrontal cortex, which is likely organized at the micrometer scale. Therefore, we make the case that prefrontal persistent activity is both necessary and sufficient for the maintenance of information in working memory.
Introduction
Working memory is the ability to maintain and manipulate information in mind, over a time span of seconds (Baddeley, 2012). The memory system storing information for a few seconds was termed “short-term memory” in the classical, three-store model of memory (Atkinson and Shiffrin, 1968). The modern definition of working memory emphasizes its dynamic nature of representing and manipulating information originating from the environment or retrieved from long-term memory, rather than being a passive conduit of information into the long-term memory store (Baddeley, 2003; Smith and Kosslyn, 2007). In recent years, some authors have reserved the term “working memory” to refer specifically to complex information that needs to be manipulated; the term “visual short term memory” has been used to denote memory of simple stimuli (e.g., colored squares) that needs to be maintained without any further transformation (Todd and Marois, 2004). Although important in its own right, working memory is a core component of a number of other cognitive functions, including language, problem solving, reasoning, and abstract thought (Baddeley, 1992). Its central role in cognitive function explains the intense research interest that spans several decades.
Studies of lesions in humans and non-human primates first implicated the cortical surface of the frontal lobe as the site of working memory function (Jacobsen, 1936; Milner, 1963). Lesions of the prefrontal cortex (PFC—Figure 1) rendered subjects unable to perform even simple tasks requiring working memory. A wide range of impairments in tasks requiring manipulation of information in memory has been confirmed in recent lesion studies (Rossi et al., 2007; Buckley et al., 2009). Subsequently, neurophysiological experiments identified neurons that not only respond to sensory stimuli, but remain active during a period after a stimulus was no longer present; this “persistent activity” therefore provided a neural correlate of working memory (Fuster and Alexander, 1971; Funahashi et al., 1989). Visuo-spatial working memory has been a particularly fruitful model since spatial location can be varied parametrically and the activity of neurons representing each location can be studied systematically. Persistent activity in the prefrontal cortex has been shown to explain many aspects of behavioral performance in visuo-spatial working memory tasks (Qi et al., 2015b).
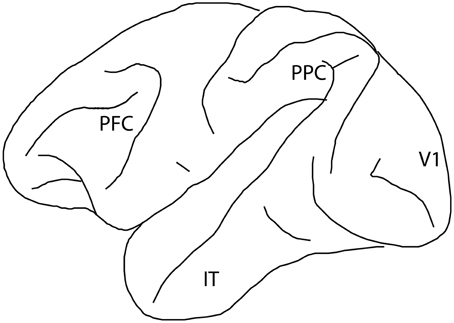
Figure 1. Diagram of the monkey brain, with four cortical regions implicated in visual working memory labeled: prefrontal cortex (PFC), posterior parietal cortex (PPC), primary visual cortex (V1), and inferior temporal cortex (IT).
The role of prefrontal cortex in working memory has been re-evaluated over the past few years (Sreenivasan et al., 2014a; D'Esposito and Postle, 2015) as several sources of experimental evidence have challenged the traditional views on prefrontal persistent activity. First, neurophysiological studies have demonstrated that persistent discharges are not limited to the prefrontal cortex, but are widespread in a network of cortical and subcortical areas, thus raising questions on the role of persistent firing in the prefrontal cortex (Constantinidis and Procyk, 2004; Pasternak and Greenlee, 2005). Secondly, phenomena such as repetition suppression illustrate that the activity of neurons may be modulated by prior stimuli in the absence of persistent activity (Grill-Spector et al., 2006). Third, human fMRI studies have been successful in decoding information held in memory from visual cortex (Harrison and Tong, 2009) and have identified correlates of working memory capacity in the posterior parietal cortex (Todd and Marois, 2004, 2005; Xu and Chun, 2006). Therefore, alternative models based on interpretation of BOLD signals (which do not directly measure spiking activity) ascribe control processes to PFC while reserving the representation of working memory for the sensory cortices (Curtis and D'esposito, 2003; D'Esposito and Postle, 2015).
In this review, we examine the role of prefrontal cortex in working memory. We take a position largely in favor of the classical model of working memory being represented in the persistent activity of prefrontal neurons based on evidence from neurophysiological experiments in non-human primates and critical evaluation of human imaging studies. We begin by examining the anatomical basis of working memory and the specializations of the prefrontal cortical circuit. We then review the range of phenomena accounted for by persistent activity in visuo-spatial working memory, illustrating the enduring appeal of the model. Activation during spatial working memory may be viewed as equivocal about the role of the prefrontal cortex because persistent activity might be explained by top-down control processes as well as by working memory itself. We therefore discuss the evidence of prefrontal persistent activity for other content types of working memory. We then review memory models not dependent on persistent activity and posit that these could only mediate a limited range of working memory tasks. We finally review activity decoded from brain areas other than the prefrontal cortex during working memory, concluding that the ultimate source of this activation is the prefrontal cortex, and these areas alone are not sufficient for mediating working memory maintenance.
Anatomical Organization of Working Memory Circuits
To understand why prefrontal cortex may represent robustly remembered information, it is instructive to review the anatomical basis of persistent activity. The primary source of sustained excitation is thought to be reverberating activity through layer II/III horizontal excitatory connections between prefrontal neurons with similar stimulus tuning (Constantinidis and Wang, 2004). PFC neurons receive horizontal connections from clusters of cells (Figure 2), arranged in stripe-like fashion, 0.2–0.8 mm wide (Goldman-Rakic, 1984; Levitt et al., 1993; Lund and Lewis, 1993; Kritzer and Goldman-Rakic, 1995; Pucak et al., 1996). Persistent firing between layer II/III neurons also depends on glutamate stimulating NMDA receptors (Wang et al., 2013). The relatively slow time constant of NMDA receptors allows the post-synaptic neuron to remain at a relatively depolarized state for a longer interval, compared to neurons containing AMPA receptors alone; without NMDA receptors, an unrealistically high level of firing rate would be required to sustain persistent activity (Wang, 2001). Additionally, sharper tuning for spatial location arises from GABAergic interneurons, which are essential in tuning the activity to represent specific spatial information (Rao et al., 1999, 2000; Constantinidis and Goldman-Rakic, 2002).
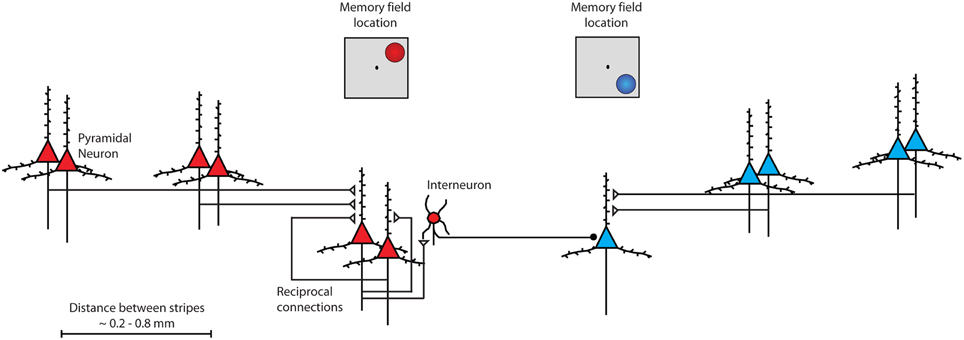
Figure 2. Schematic diagram of intrinsic connections between neurons within the prefrontal cortex. Neurons with similar tuning (memory field representing upper right location) are drawn in red color. Pyramidal neurons excite each other through reciprocal connections. Stripes of neurons with similar spatial tuning are repeated across the surface of the cortex. Interneurons inhibit other pyramidal neurons with different spatial tuning (memory field representing lower right location) drawn in blue color.
Several anatomical specializations endow the prefrontal cortex with unique properties in maintaining persistent activity. Prefrontal pyramidal neurons exhibit the most extensive dendritic trees and highest number of spines of any cortical neurons, some 23 times higher than the number of spines of layer III pyramidal cells in V1 (Elston, 2000, 2003). As a consequence, the spatial spread of functional interactions between neurons within the prefrontal cortex is more extensive than of neurons within the posterior parietal cortex (Katsuki et al., 2014). Additionally, dopaminergic innervation terminates predominantly in the frontal lobe and can improve the signal-to-noise ratio of persistent activity, mainly via enhancement of the NMDA conductance (Yang and Seamans, 1996; Durstewitz et al., 2000; Seamans et al., 2001; Chen et al., 2004). Specialized GABAergic types have also been implicated in stabilizing persistent activity in the face of distraction, and physiological signatures of these neurons have been specifically identified in the prefrontal cortex (Wang et al., 2004; Zhou et al., 2012). All of these specializations suggest that the prefrontal cortex is better suited to generate and sustain persistent activity than its afferent areas (Qi et al., 2015b).
Persistent Activity in Visuo-Spatial Working Memory
The most extensively used paradigm to study visuo-spatial working memory involves the oculomotor delayed response (ODR) task (Figure 3A), which presents subjects with a brief stimulus and, after a delay period, requires an eye movement to its remembered location (Funahashi et al., 1989; Rao et al., 1999; Constantinidis et al., 2001a). Another common task, the delayed alternation task, similarly requires a (hand or eye) movement to one of two locations, alternating in successive trials, therefore requiring memory for the location of the preceding choice (Kubota and Niki, 1971; Niki, 1974). Persistent activity selective for the spatial location of the remembered stimulus is apparent in a population of prefrontal neurons, comprising approximately a third of the total prefrontal neurons (Qi and Constantinidis, 2013). The location of the preceding stimulus in such tasks is sometimes confounded with the preparation for the motor response; however, more complex tasks reveal that the majority of prefrontal neurons represent the former rather than the latter. For example, when a task requires monkeys to make an eye movement toward a location other than the location of the visual stimulus, the majority of prefrontal neurons represent the location of the preceding stimulus rather than the location of the impeding saccade. This is the case in the delayed anti-saccade task (Funahashi et al., 1993b) and the rotational ODR task (Takeda and Funahashi, 2002).
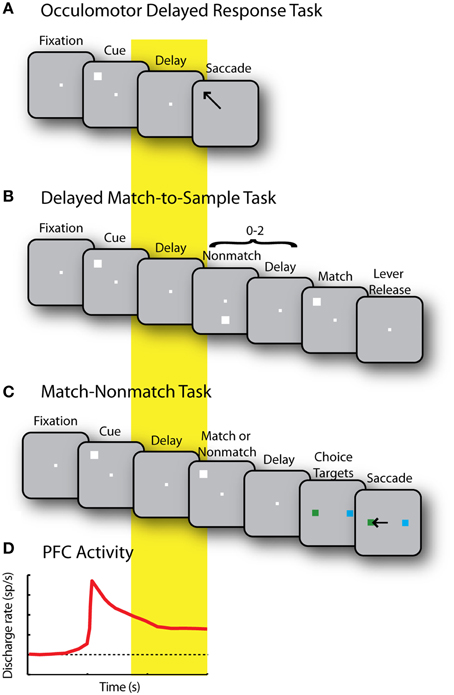
Figure 3. (A) Sequence of events in the Oculomotor Delayed Response (ODR) task. Successive frames represent the fixation period, stimulus presentation, delay period, and saccade toward the remembered stimulus location. (B) Delayed Match to Sample task. Monkeys first foveate the fixation point and pull a lever. They are then presented with a cue stimulus. This is followed by a random (0–2) number of non-match stimuli, separated by delay periods. When a match stimulus appears at the same location as the cue, the monkeys are required to release the lever. (C) Match/Non-match task. While monkeys fixate, two stimuli are presented in sequence, separated by delay periods. After another delay period, two choice targets are shown and the monkey has to saccade to the green target if the second stimulus matched the cue, and the blue stimulus, otherwise. (D) Schematic diagram of prefrontal activity elicited by the stimulus that is sustained during the delay period in each of the previous tasks.
A recent study revives the idea that persistent activity generated during ODR tasks represents motor preparation rather than memory for the stimulus (Markowitz et al., 2015). The study used two versions of the ODR task, one in which the stimulus appeared transiently (as in Figure 3A) and one in which it remained visible for the entire interval until the motor response. The conclusion that persistent activity represents motor preparation was predicated entirely on the assumption that memory storage is only mediated by neurons that exhibit persistent activity after the stimulus has been turned off, but do not continue to respond to the stimulus when it remains visible. Neurons exhibiting continuous activation by visual stimuli were considered “preparation” neurons, by default. This premise is tenuous. Neither direct evidence nor network models are available that would suggest that memory storage neurons are not activated continuously by a prolonged stimulus. In turn, this assumption leads to the conclusion that the activity of “storage units,” thus defined, has no influence on recall performance or other aspects of behavior in a memory task (Markowitz et al., 2015). This is a questionable conclusion, in our view.
Persistent activity tuned for the location of a stimulus appears in the prefrontal cortex even in tasks where the stimulus does not immediately allow planning of a movement. In the spatial delayed-match-to-sample task, subjects are required to release a lever or press a button when a stimulus appears at a previously cued location (Figure 3B); in the match/non-match task, the monkeys have to saccade to a green or blue response target depending on whether two stimuli presented in sequence appeared at the same location or not (Figure 3C). In such tasks, prefrontal neurons generate persistent activity following the presentation of the original stimulus that is tuned for its spatial location (Figure 3D), and not the preparation of a motor response, the direction of which is not known until later in the trial (Qi et al., 2010, 2011; Goodwin et al., 2012).
Persistent activity is not merely an epiphenomenon of spatial working memory, either. The most straightforward evidence in favor of this idea comes from analysis of error trials in the ODR task, which are characterized by lower levels of delay period activity (Funahashi et al., 1989; Zhou et al., 2013). In other words, trials in which persistent activity is diminished are more likely to result in errors. A near linear relationship between behavioral performance and persistent activity can be also revealed in tasks that modulate parametrically the discriminability of two remembered targets (Constantinidis et al., 2001b).
Computational models provide a detailed picture of the relationship between behavioral outcomes related to working memory performance and persistent activity (Figure 4). Persistent activity can be sustained in such models by virtue of re-entrant connections between neurons with similar tuning for stimulus properties, so that activation after afferent input is maintained in the system (Figure 4A). Drifts in neuronal activity across the network of prefrontal neurons (Figure 4B) have been shown to predict precisely the relationship between several aspects of firing rate and the endpoint of the saccade (the spatial location being recalled by the monkey) in the ODR task (Wimmer et al., 2014). For example, persistent activity recorded from trials in which monkeys make eye movements deviating clockwise vs. counterclockwise relative to the true location of the stimulus yields slightly different tuning curves, as would be expected if the location recalled was determined by the peak of activity at the end of the delay period (Figure 4C). Similarly, the variability of a neuron's delay period activity (estimated by the Fano factor of spike counts, i.e., the variance divided by the mean) is maximal for inaccurate saccades to locations at the flanks of the neuron's tuning curve but lower for locations in the peak or tail (Figure 4D). This counterintuitive finding is also explained if one appreciates that small deviations in saccadic endpoint correspond to the bump of activity shifting in one direction or another, and that activity of a single neuron changes most rapidly if the bump traverses the flank of its tuning curve rather than its peak or tail. Finally, spike-count correlations of two simultaneously recorded neurons are lowest and negative for inaccurate saccades when the cue appears between the peaks of their tuning curves (Figure 4E). This result is also consistent with the idea that working memory inaccuracies are caused by drifts of persistent activity in the delay period, and when the bump attractor randomly varies around a location between the peaks of two neurons, it inevitably causes an increase in firing rate for one neuron, but a decrease for the other. Importantly, these findings do not hold for neurons that do not exhibit persistent discharges, even though the latter are more numerous in the prefrontal cortex (Wimmer et al., 2014).
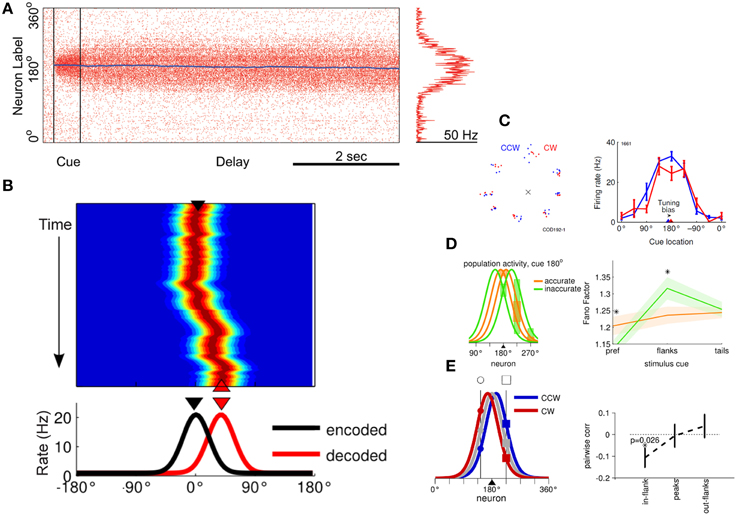
Figure 4. (A) Simulated, network activity in the ODR task, following presentation of a cue at the 180° location. Abscissa represents time during the trial; ordinate represents different neurons arranged based on their tuning. (B) Network activity illustrating drifts in the peak of activation during the delay period. Axes have been rotated relative to (A). Color represents firing rate. The black triangle represents the cue position at the beginning of the delay period (encoded population activity on the bottom graph). The red triangle represents the location decoded by the population activity at the end of the delay period. (C) Left, saccade endpoints in one behavioral session divided into trials that landed clockwise (red) or counterclockwise (blue) relative to the cue stimulus position. Right, delay-period responses of one neuron recorded during the same session. The triangles indicate the circular mean of the tuning curve obtained from trials that generated clockwise, or counterclockwise saccadic deviations. (D) Left, schematic representation of four different delay period population activity profiles to the same 180° cue. Red lines represent trials with saccadic endpoints closer to the target (accurate trials) and green lines represent trials farther from the target (inaccurate trials). Right, difference between discharge variability in inaccurate and accurate trials depending on the location of the cue. Variability is maximal for cue appearing at the flanks of the neuron's tuning curve, where small deviations cause large differences in firing rate. (E) Left, schematic representation of delay period activity of two neurons recorded simultaneously, whose tuning peaks lie at opposite sides of the activity bump. Right, trial-to-trial correlations are negative between these neurons as a bump in activity leads to an increase in firing rate of one neuron with a decrease in the other neuron. Panel (A) adapted with permission from Renart et al. (2003); panels (B–E) from Wimmer et al. (2014).
Persistent activity in the prefrontal cortex has also been shown to be subject to developmental changes, with lower levels of persistent activity present in older monkeys (Wang et al., 2011). This decline has been linked to alpha-adrenergic receptors. Drugs targeting these can ameliorate the effects of age-related cognitive deficits (Arnsten and Goldman-Rakic, 1985; Arnsten et al., 1988), as well as increase persistent discharges to levels seen in younger adults (Wang et al., 2011). An important concept to consider is that persistent activity is not the same as a generalized increase in neuronal excitability. For example, low doses of a nicotinic alpha-7 agonist enhance spatially tuned persistent activity but high doses produce non-specific excitation that erodes the representation of the remembered spatial location (Arnsten and Wang, 2016).
Persistent Activity in Non-Spatial Working Memory
Prefrontal neurons generate discharges that represent other types of information, in addition to spatial location. Ventrolateral prefrontal cortex receives input from regions of the ventral visual pathway, most importantly the inferior temporal cortex and superior temporal gyrus (Petrides and Pandya, 1988; Webster et al., 1994). Generally, smaller populations of prefrontal neurons are tuned for object attributes such as geometric shape, color, or complex features (e.g., specific faces), than spatial location; a regional specialization is also present, with spatial information more prevalent in the dorsolateral prefrontal cortex than the ventrolateral prefrontal cortex (Meyer et al., 2011). Nonetheless, robust, stimulus-selective persistent activity has been described in working memory tasks requiring subjects to remember the identity and features of stimuli. Examples include stimuli defined by simple, geometric shapes differing in color or luminance (Quintana et al., 1988; Hoshi et al., 1998; Constantinidis et al., 2001b; Sakagami et al., 2001; Averbeck et al., 2003; Inoue and Mikami, 2006; Genovesio et al., 2009), complex images, such as real objects and faces, or abstract pictures (Wilson et al., 1993; Miller et al., 1996; O Scalaidhe et al., 1997, 1999; Rao et al., 1997; Rainer et al., 1998; Rainer and Miller, 2000; Freedman et al., 2001; Roy et al., 2014) and the direction of motion of a random-dot stimulus that is always presented at the same location (Zaksas and Pasternak, 2006; Mendoza-Halliday et al., 2014).
In recent years, it has been recognized that persistent activity in the prefrontal cortex also represents information beyond the characteristics of stimuli. Activity may represent the abstract rules of the cognitive task subjects are required to perform (White and Wise, 1999; Wallis et al., 2001), categories (Freedman et al., 2001; Shima et al., 2007), and numerical quantities (Nieder et al., 2002). It may be also related to perceptual decisions (Kim and Shadlen, 1999; Barraclough et al., 2004), reward expectation (Leon and Shadlen, 1999), and sequences of events or actions (Averbeck et al., 2002; Inoue and Mikami, 2006; Sigala et al., 2008; Berdyyeva and Olson, 2010). Persistent activity of single neurons may represent more information than stimulus features and task variables simultaneously (Rigotti et al., 2013). For instance, persistent firing may represent different aspects of the task demands as they change over time, thus providing dynamic representations (Mante et al., 2013).
The realization that prefrontal activity is modulated by task factors to such extent has led to a re-evaluation of the nature of information represented in persistent activity (D'Esposito and Postle, 2015). Taken to the extreme, this idea would suggest that all stimulus-selective information that appears to be represented in the prefrontal cortex is in fact related to task rules or categorical judgments between alternatives rather than representing the memoranda themselves. In an attempt to pinpoint the nature of information represented in the prefrontal cortex, some experiments have relied on working memory for stimuli defined solely by elemental properties, such as direction of motion or color, and found the ability of prefrontal cortex to represent such features wanting. In an experiment requiring subjects to remember the overall direction of motion of an initial random-dot display and decide if the direction of a following display was the same or different, prefrontal neurons exhibited only transient representation of direction information in the delay period (Zaksas and Pasternak, 2006). Another experiment that required memory for the color of a stimulus revealed that very few prefrontal neurons exhibited pure color information, as opposed to information about its location (Lara and Wallis, 2014).
Ruling out prefrontal cortex as the cortical area mediating the representation of object information in working memory based on such negative findings appears premature. More recent experiments have succeeded in revealing robust persistent activity representing direction of motion throughout the delay period of a working memory task in the prefrontal cortex (and area MST) but not in area MT of the visual cortex, although MT was robustly activated during the presentation of these stimuli (Mendoza-Halliday et al., 2014). In the case of color, too, activation of only a small proportion of prefrontal neurons, in the order of 5–15% (Lara and Wallis, 2014) may be sufficient for the representation of stimulus information. It is also possible that color-selective neurons are concentrated in specific prefrontal “patches” (Lafer-Sousa and Conway, 2013) and persistent activity representing color information may be concentrated in such modules rather than be diffused across the entire prefrontal surface.
Persistent neuronal firing in prefrontal cortex has been observed even in the absence of performance of a task, or even learning of a task, while subjects view stimuli, passively. Prefrontal neurons have thus been shown to generate persistent discharges tuned for stimulus location and shape in monkeys never trained to perform a working memory (or other cognitive) task (Meyer et al., 2011; Meyers et al., 2012). The fact that prefrontal neurons generate persistent activity when not required to perform a working memory task is not incompatible with our intuition of working memory, either. We are able to recall stimuli we encounter even when we are not prompted to maintain them in memory ahead of time (Qi et al., 2015b). Consistent with this finding, recordings during passive fixation reveal persistent discharges selective for faces in the ventrolateral prefrontal cortex (O Scalaidhe et al., 1999). Prefrontal neurons also represent stimulus features even when they are irrelevant for the task at hand (Constantinidis et al., 2001b; Lauwereyns et al., 2001; Donahue and Lee, 2015). This evidence argues that persistent activity in the prefrontal cortex is sufficient to represent object-related information in working memory. In Section Alternative Working Memory Models, we will review the evidence that prefrontal cortex is also necessary for this role.
Alternative Working Memory Models
In recent years, the role of persistent activity has come into question by alternative models proposed to mediate working memory. By some accounts, information can be maintained in memory over a period of seconds through mechanisms other than persistent discharges. We will examine three categories of models here: non-spiking models dependent on synaptic mechanisms, rhythmic-spiking models conveying information based on the frequency and phase of discharges without necessarily an increase in overall activity, and dynamic-spiking models in which information is represented based on the pattern of neurons that are active without an elevation of mean firing rate across the population.
Non-spiking Models
Activity elicited after repeated presentation of the same stimulus is typically reduced, a phenomenon termed repetition suppression (Grill-Spector et al., 2006). As a result, the level of response to a particular stimulus in the context of a working memory task, such as the delayed match to sample task, can be informative about whether it was preceded by the same stimulus or not; match suppression may signal that the sample was the same as the match. This suppressed response to a matching stimulus is observed even though several seconds may intervene between the sample and match, and it does not require persistent activity (Miller et al., 1991, 1996). Match suppression (or enhancement, for some neurons) is observed for stimuli matching in shape, color, and form, in spatial location, or in direction of motion, in various cortical areas, including the prefrontal, posterior parietal, and inferior temporal cortex (Miller et al., 1991, 1996; Steinmetz et al., 1994; Zaksas and Pasternak, 2006; Woloszyn and Sheinberg, 2009). Furthermore, the extent of response difference to matching and non-matching stimuli has predictive power over behavioral performance, as it differs systematically in correct and error trials (Zaksas and Pasternak, 2006; Qi et al., 2012).
Computational models have been proposed that could account for such changes via mechanisms that do not depend on spike generation, but instead involve modification of synaptic strengths (Mongillo et al., 2008; Sugase-Miyamoto et al., 2008). Such mechanisms may be mediated by calcium availability at the presynaptic terminal, whose kinetics have a time constant in the scale of seconds (Mongillo et al., 2008). The duration and stability of working memory in such models may still be modulated by spiking activity.
Repetition suppression is a robust phenomenon observed across multiple cortical areas and the fact that the match/non-match effect differs in correct and error trials offers compelling evidence that memory performance has access to this activity. However, it is a phenomenon limited to recognition memory that may not even mediate representation of the identity of the remembered stimulus, and it cannot account for working memory performance in other tasks. It is hard to imagine an equivalent role of synaptic mechanisms for tasks such as the ODR, delayed alternation, N-back, or free recall tasks. Moreover, other computational models show that even though preference for a non-match over a match stimulus may be present in individual neurons with no persistent activity, the phenomenon may still be mediated by a network that depends on persistent activity (Engel and Wang, 2011). It is still an open question, therefore if synaptic mechanisms have a role in working memory in the absence of persistent activity.
Oscillatory Models
Rhythmic activity has long been implicated in hippocampal-dependent memory, and communication between the hippocampus and prefrontal cortex, in rodents (Buzsaki, 2010). In the human literature, the frequency of oscillations evident through MEG, EEG, and ECoG recordings has also been associated with distinct working memory processes (Roux and Uhlhaas, 2014). Recent neurophysiological studies in non-human primates have begun to address more specifically what role rhythmic firing may play in working memory (Siegel et al., 2009; Buschman et al., 2012; Liebe et al., 2012; Salazar et al., 2012; Brincat and Miller, 2015). The magnitude, frequency, and phase of oscillations within the prefrontal cortex and between the prefrontal cortex and other areas have been shown to be modulated depending on stimulus and task information (Buschman et al., 2012; Liebe et al., 2012). Therefore, information about the stimulus held in memory or task to be performed may be decoded based on these parameters. For example, oscillatory synchronization between LFP signals recorded from different sites within the prefrontal cortex has been shown to be modulated based on which of two task rules a monkey is performing (Buschman et al., 2012). The coherence in rhythmic synchronization between neurons in prefrontal and posterior parietal cortex has also been reported to be content dependent; in other words, prefrontal and parietal neurons synchronize their firing at specific frequencies, for different stimuli held in memory (Salazar et al., 2012). The phase of rhythmic activity could also differentiate information representing two sequentially presented stimuli (Siegel et al., 2009).
Oscillatory activity is not incompatible with persistent activity. For example, both robust persistent activity and gamma-band rhythmicity have been reported during the delay period of the ODR task (Pesaran et al., 2002), as well as the two-item memory task described above (Siegel et al., 2009). It is an open question whether oscillatory activity may dictate behavioral performance in working memory tasks independently of persistent activity.
Dynamic Information Models
Information may be represented dynamically in a neuronal population without having to be rhythmic. The precise pattern of activation of different neurons at each time point during a working memory task can be used to decode the identity of the stimulus, even though overall activity during the delay period is not significantly elevated above the baseline (Stokes et al., 2013). This result provides yet another alternative mechanism of working memory representation.
The existence of stimulus information that can be decoded by the dynamic pattern of activation in the prefrontal population (Stokes et al., 2013) presents challenges to the persistent activity model. We should consider however that the stimuli used in the Stokes et al. study are similar to those used in previous studies where persistent activity was observed (Miller et al., 1996; Rao et al., 1997; Rainer et al., 1998). It is possible therefore that a population of neurons did generate persistent activity but might have been too weak to detect when all neurons were averaged together. The demonstration of a condition where persistent activity is truly absent and information is encoded solely by the dynamic pattern of information in neurons whose activity is not modulated during working memory is an open question. Furthermore, dynamic firing models have yet to establish what aspects of information that can be decoded from the dynamic representation of stimulus information can predict behavioral variables, such as recall error rates, accuracy of recall, or reaction time, to the extent that models of persistent activity have been successful in doing (Wimmer et al., 2014).
Dynamic patterns of activation across the population of neurons are not mutually exclusive with persistent activity either. Dynamic activity informative about stimulus identity and task rules has been observed even when persistent activity is present in the population (Crowe et al., 2008; Meyers et al., 2012). Different populations of neurons may also be active at different time points of the ODR task representing stimulus attributes or response preparation (Markowitz et al., 2015). One possible resolution to the two seemingly incompatible mechanisms of information representation is found by analyzing the neuronal population activity during the ODR task. Principal Component Analysis reveals a dynamic, low-dimensional representation, where stimulus location evolves dynamically in time after the cue presentation, but different locations remain constrained in separable subspaces (Roy et al., 2013). Persistent firing specific for the location of a stimulus may thus sweep the population of neurons, in a specific pattern, during the time course of a trial.
Role of Other Areas in Working Memory
Persistent discharges are not an exclusive property of the prefrontal cortex. Neurons in premotor, parietal, cingulate, and temporal association areas generate robust persistent activity, as do subcortical structures including the basal ganglia and the mediodorsal nucleus of the thalamus (Constantinidis and Procyk, 2004; Pasternak and Greenlee, 2005). The proposed alternative mechanisms of memory maintenance reviewed before, and fMRI findings in humans have expanded the list of potential sites of memory into even more cortical areas, as early as the primary visual cortex (Harrison and Tong, 2009). We will next review the evidence of working memory representation in the posterior parietal and inferior temporal cortex (for spatial and object memory, respectively), and in visual cortical areas, including V1.
Posterior Parietal (PPC) and Inferior Temporal (IT) Cortex
The posterior parietal and inferior temporal cortex represent the two main cortical afferents of the prefrontal cortex, as they are strongly interconnected with the dorsolateral and ventrolateral prefrontal cortex, respectively (Constantinidis and Procyk, 2004). Posterior parietal and dorsolateral prefrontal cortex share many functional properties with respect to spatial working memory (Rawley and Constantinidis, 2009) and both regions are activated simultaneously in human imaging studies of working memory (Jonides et al., 1993; Courtney et al., 1997; Owen et al., 1998; Ungerleider et al., 1998; Marshuetz et al., 2000; Bunge et al., 2001; Stern et al., 2001). Neurons in posterior parietal cortex also generate persistent activity (Gnadt and Andersen, 1988), and this has been shown to represent the remembered locations of visual stimuli, independent of a planned motor response (Constantinidis and Steinmetz, 1996). Tested with the ODR task, virtually identical percentages of neurons exhibiting working memory responses were observed in posterior parietal and dorsolateral prefrontal areas (Chafee and Goldman-Rakic, 1998).
Responses of IT neurons related to object memory exhibit many intriguing parallels with spatial working memory in the posterior parietal cortex. IT cortex shares a number of physiological properties with ventrolateral prefrontal cortex and both exhibit memory-related activation. IT neurons discharge in a persistent fashion after the offset of visual stimuli and their activity encodes the features of the remembered stimulus (Fuster and Jervey, 1981, 1982; Miyashita and Chang, 1988; Miller et al., 1993; Nakamura and Kubota, 1995; Naya et al., 2001; Sigala and Logothetis, 2002).
This simultaneous activation of the areas that are interconnected with the prefrontal cortex during working memory has inspired views that the prefrontal cortex does not represent a memory trace for a particular item per se, but rather an abstract representation, allocation of cognitive resources, the focus of attention, or other top-down signals (Cowan, 1988; Miller and Cohen, 2001; Hazy et al., 2006; Postle, 2006; D'Esposito, 2007). In this framework, the contents of memory may be represented in PPC and IT, instead. Evidence against this idea comes from memory tasks that require maintenance in memory of an original item through sequential presentation of distracting stimuli, such as the delayed match to sample task. Both object and spatial versions of this task have been developed. In the context of the object delayed-match-to-sample task, persistent discharges of IT neurons are interrupted by non-matching, distractor stimuli presented after the sample (Miller et al., 1993). Conversely, responses in the ventral prefrontal cortex are able to represent the actively remembered sample's feature throughout the trial regardless of the distractor stimuli displayed (Miller et al., 1996). Equivalent findings have been obtained in the posterior parietal cortex for the spatial delayed-match-to-sample task (Katsuki and Constantinidis, 2012). Posterior parietal discharges represent the most recent stimulus location and are disrupted by distracting stimuli (Constantinidis and Steinmetz, 1996). Prefrontal neurons are able to represent the location of the original stimulus held in memory even after the appearance of distractors, in various tasks (di Pellegrino and Wise, 1993; Qi et al., 2010; Suzuki and Gottlieb, 2013).
Most recent studies have somewhat qualified these findings, for example demonstrating that differences between IT/PPC and prefrontal neurons in their ability to generate persistent activity that survives distractors are qualitative rather than quantitative (Woloszyn and Sheinberg, 2009; Qi et al., 2010), and that prefrontal neurons may respond better to distractors than actively remembered stimuli, in some tasks (Jacob and Nieder, 2014; Qi et al., 2015a). Nonetheless, in the context of the working memory tasks reviewed in the preceding paragraph, performance of the task is simply not possible based on the activation of the posterior parietal or inferior temporal cortex alone. The link of prefrontal activation with performance of working memory tasks that involve sequential presentation of distracting stimuli is confirmed by human imaging studies, as well: prefrontal activation is predictive of errors when activity representing an initial item is not maintained, whereas parietal cortex is indiscriminately activated by behaviorally relevant stimuli and distractors, alike (Sakai et al., 2002). Accumulating studies ascribing different roles in the activity of prefrontal and parietal cortex in working memory (Jacob and Nieder, 2014; Qi et al., 2015a), and functions such as attention and categorization (Swaminathan and Freedman, 2012; Crowe et al., 2013; Ibos et al., 2013), raise the alternative possibility that prefrontal and PPC/IT cortex are specialized for different aspects of working memory, as well as other cognitive functions (Katsuki and Constantinidis, 2012).
An instance of such differentiation may be the reported role of the posterior parietal cortex in determining the capacity of working memory (Todd and Marois, 2004, 2005). Activation of parietal cortex revealed by fMRI best predicts the number of simultaneous items maintained in working memory, relative to both earlier areas and the prefrontal cortex (Todd and Marois, 2004). The single-neuron basis of the phenomenon is not clear, however. Persistent discharges in the prefrontal and posterior parietal cortex reveal few differences between the two areas and no obvious neural correlate that is present only in the posterior parietal cortex and could determine capacity (Buschman et al., 2011).
The primacy of prefrontal cortex in working memory behavior is perhaps most vividly demonstrated in inactivation studies. Cooling experiments, which reversibly inactivate the underlying cortex by lowering its temperature, demonstrate much greater decreases in memory performance in the ODR task after prefrontal than posterior parietal cooling (Chafee and Goldman-Rakic, 2000), even when the areas inactivated have similar delay period activity (Chafee and Goldman-Rakic, 1998). The results of these studies parallel the effects of reversible inactivation of the frontal eye fields via muscimol injections, which similarly produce a significant impairment in memory-guided saccade performance (Sommer and Tehovnik, 1997; Dias and Segraves, 1999). In contrast, modest or no impairment was observed after muscimol inactivation of the posterior parietal cortex (Li et al., 1999; Chafee and Goldman-Rakic, 2000; Wilke et al., 2012), even though posterior parietal inactivation produces consistent deficits in tasks that require attention or selection between multiple stimuli (Wardak et al., 2002, 2004; Liu et al., 2010; Wilke et al., 2012). Small lesions to the dorsolateral prefrontal cortex also produce impairment in working memory performance for remembered stimuli in the contralateral space, an effect termed a “mnemonic scotoma” (Funahashi et al., 1993a; Funahashi, 2015). Equivalent results from localized lesions of the posterior parietal cortex are not available.
Visual Cortex
In recent years, human imaging studies have been successful in decoding information held in memory from the visual cortex, including the primary (Harrison and Tong, 2009; Albers et al., 2013; Xing et al., 2013) and extrastriate visual cortex (Ester et al., 2013; Sreenivasan et al., 2014b), suggesting that these areas maintain the contents of working memory (Tong and Pratte, 2012). This extraction of information has been possible with Multi-Variate Pattern Analysis (MVPA), examining the simultaneous pattern of activation of multiple voxels to different task conditions; the overall levels of activity in visual cortex may not rise above baseline during working memory (Offen et al., 2009). Imaging studies have gone as far as to determine that the size of the primary visual cortex alone is the best predictor of working memory ability (Bergmann et al., 2016). Importantly, MVPA could not decode information from the prefrontal cortex, or could not fully account for behavioral performance in the task (Harrison and Tong, 2009; Sreenivasan et al., 2014b).
This negative finding of information failing to be decoded from the prefrontal cortex during working memory, despite the known activation of prefrontal neuron in similar tasks, is telling about the interpretative limitations of these results. A tacit assumption when comparing the results of MVPA analysis across different cortical areas is that the structure of the voxel (typically in the order of 3 × 3 × 3 mm) is equivalent in the primary visual and prefrontal cortex. This is definitely not the case. Unlike the precise topography of visual space in the primary visual cortex, no retinotopic map (or other overarching organizational principle) has been revealed in the prefrontal cortex (Constantinidis and Procyk, 2004). Sampling the prefrontal cortex with chronic arrays of micro-electrodes spaced at 0.4 mm of each other reveal that the same cortical location is represented multiple times across the surface, and with no obvious map of space (Leavitt et al., 2013; Kiani et al., 2015). Simultaneously recorded neurons with movable electrodes spaced as close as to 0.2 mm of each other reveal only a slight bias toward similar spatial preference among neighboring prefrontal neurons (Constantinidis et al., 2001a). Precise stimulus location information is therefore represented in an extremely fine spatial scale, with the entire visual hemifield possibly represented in prefrontal modules no large than 0.5 × 0.5 mm in surface (Constantinidis et al., 2001a). Voxels averaging cortical volumes an order of magnitude larger are thus likely to obliterate stimulus information and will predictably fail to decode the information held in working memory, even if this is robustly represented in the activity of prefrontal neurons.
A recent fMRI study has in fact been successful in retrieving features of remembered stimuli, the orientation of a grating, from the prefrontal cortex during working memory (Ester et al., 2015). Such information may be represented more coarsely across the surface of the prefrontal cortex, making it possible to decode from fMRI activation patterns. In any case, these results argue directly against models of working memory that postulate solely a top-down control role for the prefrontal cortex, and place feature storage networks in the visual cortex (Ester et al., 2015).
MVPA methods still yield undeniable positive findings of fMRI imaging in the visual cortex and it is important to consider the neural basis of this activity that yields information about the contents of working memory. Early visual areas do not generate persistent activity. A recent study comparing activity in three cortical areas in the same animals, required to remember the direction of motion of a random-dot display, found virtually no persistent discharges in visual area MT, but robust activation in parietal area MST, in addition to prefrontal persistent activation (Mendoza-Halliday et al., 2014). This suggests an abrupt generation of feature-selective persistent activity in areas beyond the visual cortex. On the other hand, a small percentage of V1 neurons exhibit suppressed levels of discharges during working memory, below background levels (Super et al., 2001). It is unclear, however, whether V1 activity can be predictive of behavior in working memory task as this modulation was present for both correct and incorrect trials (Super et al., 2001). Changes in levels of activity in V1 during working memory are likely due to top-down projections from higher associative cortices, since V1 activation appears first in superficial layers (Roelfsema, 2015). A key aspect of this phenomenon is that background levels of activity in V1 are relatively “quiet,” thus making it possible to capture the subtle backwash from higher cortical areas, while the higher cortical areas themselves may be too noisy to detect these small signals. fMRI activation may additionally be detecting pre-synaptic activation of V1 neurons from higher cortical areas (Logothetis and Wandell, 2004), which makes V1 activity even less likely to be the ultimate storage of working memory contents and determinant of working memory performance.
Conclusions and Unresolved Questions
The role of prefrontal persistent activity in working memory has been the focus of renewed attention in the past few years. This interest has been spurred by the realization that other brain areas are also active during working memory maintenance, that persistent activity may be shaped by the demands of the task rather than merely be representing information, and that dynamic patterns of activity can represent information in working memory. These results have inspired alternative models of working memory maintenance in the brain.
In this review, we make the case that persistent activity in the prefrontal cortex is both necessary and sufficient to account for information held in memory, across a variety of tasks and experimental conditions. Prefrontal persistent activity is also present in working memory tasks that do not rely on spatial stimuli and can encode attributes of stimuli (such as direction of motion and shape) or task variables and rules. Computational models based on persistent activity can account for levels of performance and patterns of errors depending on neuronal discharges to a greater extent than any alternative models.
Phenomena like repetition suppression are likely to be generated by synaptic rather than spiking mechanisms and they appear to correlate with behavior. However, they can only account for a limited set of behaviors and memory functions. Similarly, rhythmic or otherwise dynamic patterns of activity across the population of prefrontal neurons may convey information about stimulus properties. Such patterns of activation are not incompatible with persistent activity, either. It is upon future research to determine whether a causal relationship exists between such mechanisms and working memory performance.
The prefrontal cortex is not the only area that represents working memory information. Posterior parietal and inferior temporal areas have been long known to be active during working memory, though they appear insufficient to sustain information, for at least some tasks. It remains an open question on whether these areas are specialized for different aspects of working memory performance, or if their activity supports the maintenance of working memory in a distributed network that requires the prefrontal cortex. Information decoded from the primary visual cortex but not in the prefrontal cortex in fMRI studies cannot rule out a prefrontal involvement in working memory due to interpretational limitations that have to do with the topography of stimulus representation in these areas. It remains unclear whether neuronal activity in primary visual cortex plays any role in determining working memory behavior. Future work should aim to resolve these issues.
Author Contributions
MR and CC conceptually developed and wrote this review.
Conflict of Interest Statement
The authors declare that the research was conducted in the absence of any commercial or financial relationships that could be construed as a potential conflict of interest.
Acknowledgments
Research reported in this paper was supported by the National Eye Institute of the National Institutes of Health under award numbers R01 EY017077 and R01 EY016773 to CC; NIMH award F31 MH104012 to MR; and by the Tab Williams Family Endowment and Harry O'Parker Neurosciences Fund at the Wake Forest School of Medicine.
References
Albers, A. M., Kok, P., Toni, I., Dijkerman, H. C., and De Lange, F. P. (2013). Shared representations for working memory and mental imagery in early visual cortex. Curr. Biol. 23, 1427–1431. doi: 10.1016/j.cub.2013.05.065
Arnsten, A. F., Cai, J. X., and Goldman-Rakic, P. S. (1988). The alpha-2 adrenergic agonist guanfacine improves memory in aged monkeys without sedative or hypotensive side effects: evidence for alpha-2 receptor subtypes. J. Neurosci. 8, 4287–4298.
Arnsten, A. F., and Goldman-Rakic, P. S. (1985). Alpha 2-adrenergic mechanisms in prefrontal cortex associated with cognitive decline in aged nonhuman primates. Science 230, 1273–1276. doi: 10.1126/science.2999977
Arnsten, A. F., and Wang, M. (2016). Targeting prefrontal cortical systems for drug development: potential therapies for cognitive disorders. Annu. Rev. Pharmacol. Toxicol. [Epub ahead of print].
Atkinson, R. C., and Shiffrin, R. M. (1968). “Human memory: a proposed system and its control processes,” in The Psychology of Learning and Motivation, eds K. W. Spence and J. T. Spence (London: Academic Press), 89–195.
Averbeck, B. B., Chafee, M. V., Crowe, D. A., and Georgopoulos, A. P. (2002). Parallel processing of serial movements in prefrontal cortex. Proc. Natl. Acad. Sci. U.S.A. 99, 13172–13177. doi: 10.1073/pnas.162485599
Averbeck, B. B., Chafee, M. V., Crowe, D. A., and Georgopoulos, A. P. (2003). Neural activity in prefrontal cortex during copying geometrical shapesI. Single cells encode shape, sequence, and metric parameters. Exp. Brain Res. 150, 127–141.
Baddeley, A. (2003). Working memory: looking back and looking forward. Nat. Rev. Neurosci. 4, 829–839. doi: 10.1038/nrn1201
Baddeley, A. (2012). Working memory: theories, models, and controversies. Annu. Rev. Psychol. 63, 1–29. doi: 10.1146/annurev-psych-120710-100422
Barraclough, D. J., Conroy, M. L., and Lee, D. (2004). Prefrontal cortex and decision making in a mixed-strategy game. Nat. Neurosci. 7, 404–410. doi: 10.1038/nn1209
Berdyyeva, T. K., and Olson, C. R. (2010). Rank signals in four areas of macaque frontal cortex during selection of actions and objects in serial order. J. Neurophysiol. 104, 141–159. doi: 10.1152/jn.00639.2009
Bergmann, J., Genc, E., Kohler, A., Singer, W., and Pearson, J. (2016). Neural anatomy of primary visual cortex limits visual working memory. Cereb. Cortex 26, 43–50. doi: 10.1093/cercor/bhu168
Brincat, S. L., and Miller, E. K. (2015). Frequency-specific hippocampal-prefrontal interactions during associative learning. Nat. Neurosci. 18, 576–581. doi: 10.1038/nn.3954
Buckley, M. J., Mansouri, F. A., Hoda, H., Mahboubi, M., Browning, P. G., Kwok, S. C., et al. (2009). Dissociable components of rule-guided behavior depend on distinct medial and prefrontal regions. Science 325, 52–58. doi: 10.1126/science.1172377
Bunge, S. A., Ochsner, K. N., Desmond, J. E., Glover, G. H., and Gabrieli, J. D. (2001). Prefrontal regions involved in keeping information in and out of mind. Brain 124, 2074–2086. doi: 10.1093/brain/124.10.2074
Buschman, T. J., Denovellis, E. L., Diogo, C., Bullock, D., and Miller, E. K. (2012). Synchronous oscillatory neural ensembles for rules in the prefrontal cortex. Neuron 76, 838–846. doi: 10.1016/j.neuron.2012.09.029
Buschman, T. J., Siegel, M., Roy, J. E., and Miller, E. K. (2011). Neural substrates of cognitive capacity limitations. Proc. Natl. Acad. Sci. U.S.A. 108, 11252–11255. doi: 10.1073/pnas.1104666108
Buzsáki, G. (2010). Neural syntax: cell assemblies, synapsembles, and readers. Neuron 68, 362–385. doi: 10.1016/j.neuron.2010.09.023
Chafee, M. V., and Goldman-Rakic, P. S. (1998). Matching patterns of activity in primate prefrontal area 8a and parietal area 7ip neurons during a spatial working memory task. J. Neurophysiol. 79, 2919–2940.
Chafee, M. V., and Goldman-Rakic, P. S. (2000). Inactivation of parietal and prefrontal cortex reveals interdependence of neural activity during memory-guided saccades. J. Neurophysiol. 83, 1550–1566.
Chen, G., Greengard, P., and Yan, Z. (2004). Potentiation of NMDA receptor currents by dopamine D1 receptors in prefrontal cortex. Proc. Natl. Acad. Sci. U.S.A. 101, 2596–2600. doi: 10.1073/pnas.0308618100
Constantinidis, C., Franowicz, M. N., and Goldman-Rakic, P. S. (2001a). Coding specificity in cortical microcircuits: a multiple electrode analysis of primate prefrontal cortex. J. Neurosci. 21, 3646–3655.
Constantinidis, C., Franowicz, M. N., and Goldman-Rakic, P. S. (2001b). The sensory nature of mnemonic representation in the primate prefrontal cortex. Nat. Neurosci. 4, 311–316. doi: 10.1038/85179
Constantinidis, C., and Goldman-Rakic, P. S. (2002). Correlated discharges among putative pyramidal neurons and interneurons in the primate prefrontal cortex. J. Neurophysiol. 88, 3487–3497. doi: 10.1152/jn.00188.2002
Constantinidis, C., and Procyk, E. (2004). The primate working memory networks. Cogn. Affect. Behav. Neurosci. 4, 444–465. doi: 10.3758/CABN.4.4.444
Constantinidis, C., and Steinmetz, M. A. (1996). Neuronal activity in posterior parietal area 7a during the delay periods of a spatial memory task. J. Neurophysiol. 76, 1352–1355.
Constantinidis, C., and Wang, X. J. (2004). A neural circuit basis for spatial working memory. Neuroscientist 10, 553–565. doi: 10.1177/1073858404268742
Courtney, S. M., Ungerleider, L. G., Keil, K., and Haxby, J. V. (1997). Transient and sustained activity in a distributed neural system for human working memory. Nature 386, 608–611. doi: 10.1038/386608a0
Cowan, N. (1988). Evolving conceptions of memory storage, selective attention, and their mutual constraints within the human information processing system. Psychol. Bull. 104, 163–191. doi: 10.1037/0033-2909.104.2.163
Crowe, D. A., Averbeck, B. B., and Chafee, M. V. (2008). Neural ensemble decoding reveals a correlate of viewer- to object-centered spatial transformation in monkey parietal cortex. J. Neurosci. 28, 5218–5228. doi: 10.1523/JNEUROSCI.5105-07.2008
Crowe, D. A., Goodwin, S. J., Blackman, R. K., Sakellaridi, S., Sponheim, S. R., Macdonald, A. W. III, et al. (2013). Prefrontal neurons transmit signals to parietal neurons that reflect executive control of cognition. Nat. Neurosci. 16, 1484–1491. doi: 10.1038/nn.3509
Curtis, C. E., and D'esposito, M. (2003). Persistent activity in the prefrontal cortex during working memory. Trends Cogn. Sci. (Regul. Ed). 7, 415–423. doi: 10.1016/S1364-6613(03)00197-9
D'Esposito, M. (2007). From cognitive to neural models of working memory. Philos. Trans. R. Soc. Lond. B Biol. Sci. 362, 761–772. doi: 10.1098/rstb.2007.2086
D'Esposito, M., and Postle, B. R. (2015). The cognitive neuroscience of working memory. Annu. Rev. Psychol. 66, 115–142. doi: 10.1146/annurev-psych-010814-015031
Dias, E. C., and Segraves, M. A. (1999). Muscimol-induced inactivation of monkey frontal eye field: effects on visually and memory-guided saccades. J. Neurophysiol. 81, 2191–2214.
di Pellegrino, G., and Wise, S. P. (1993). Effects of attention on visuomotor activity in the premotor and prefrontal cortex of a primate. Somatosens. Mot. Res. 10, 245–262. doi: 10.3109/08990229309028835
Donahue, C. H., and Lee, D. (2015). Dynamic routing of task-relevant signals for decision making in dorsolateral prefrontal cortex. Nat. Neurosci. 18, 295–301. doi: 10.1038/nn.3918
Durstewitz, D., Seamans, J. K., and Sejnowski, T. J. (2000). Dopamine-mediated stabilization of delay-period activity in a network model of prefrontal cortex. J. Neurophysiol. 83, 1733–1750.
Elston, G. N. (2000). Pyramidal cells of the frontal lobe: all the more spinous to think with. J. Neurosci. 20, RC95.
Elston, G. N. (2003). The pyramidal neuron in occipital, temporal and prefrontal cortex of the owl monkey (Aotus trivirgatus): regional specialization in cell structure. Eur. J. Neurosci. 17, 1313–1318. doi: 10.1046/j.1460-9568.2003.02552.x
Engel, T. A., and Wang, X. J. (2011). Same or different? A neural circuit mechanism of similarity-based pattern match decision making. J. Neurosci. 31, 6982–6996. doi: 10.1523/JNEUROSCI.6150-10.2011
Ester, E. F., Anderson, D. E., Serences, J. T., and Awh, E. (2013). A neural measure of precision in visual working memory. J. Cogn. Neurosci. 25, 754–761. doi: 10.1162/jocn_a_00357
Ester, E. F., Sprague, T. C., and Serences, J. T. (2015). Parietal and frontal cortex encode stimulus-specific mnemonic representations during visual working memory. Neuron 87, 893–905. doi: 10.1016/j.neuron.2015.07.013
Freedman, D. J., Riesenhuber, M., Poggio, T., and Miller, E. K. (2001). Categorical representation of visual stimuli in the primate prefrontal cortex. Science 291, 312–316. doi: 10.1126/science.291.5502.312
Funahashi, S. (2015). Functions of delay-period activity in the prefrontal cortex and mnemonic scotomas revisited. Front. Syst. Neurosci. 9:2. doi: 10.3389/fnsys.2015.00002
Funahashi, S., Bruce, C. J., and Goldman-Rakic, P. S. (1989). Mnemonic coding of visual space in the monkey's dorsolateral prefrontal cortex. J. Neurophysiol. 61, 331–349.
Funahashi, S., Bruce, C. J., and Goldman-Rakic, P. S. (1993a). Dorsolateral prefrontal lesions and oculomotor delayed-response performance: evidence for mnemonic “scotomas.” J. Neurosci. 13, 1479–1497.
Funahashi, S., Chafee, M. V., and Goldman-Rakic, P. S. (1993b). Prefrontal neuronal activity in rhesus monkeys performing a delayed anti-saccade task. Nature 365, 753–756. doi: 10.1038/365753a0
Fuster, J. M., and Alexander, G. E. (1971). Neuron activity related to short-term memory. Science 173, 652–654. doi: 10.1126/science.173.3997.652
Fuster, J. M., and Jervey, J. P. (1981). Inferotemporal neurons distinguish and retain behaviorally relevant features of visual stimuli. Science 212, 952–955. doi: 10.1126/science.7233192
Fuster, J. M., and Jervey, J. P. (1982). Neuronal firing in the inferotemporal cortex of the monkey in a visual memory task. J. Neurosci. 2, 361–375.
Genovesio, A., Tsujimoto, S., and Wise, S. P. (2009). Feature- and order-based timing representations in the frontal cortex. Neuron 63, 254–266. doi: 10.1016/j.neuron.2009.06.018
Gnadt, J. W., and Andersen, R. A. (1988). Memory related motor planning activity in posterior parietal cortex of macaque. Exp. Brain Res. 70, 216–220.
Goldman-Rakic, P. S. (1984). Modular organization of prefrontal cortex. Trends Neurosci. 7, 419–424. doi: 10.1016/S0166-2236(84)80146-0
Goodwin, S. J., Blackman, R. K., Sakellaridi, S., and Chafee, M. V. (2012). Executive control over cognition: stronger and earlier rule-based modulation of spatial category signals in prefrontal cortex relative to parietal cortex. J. Neurosci. 32, 3499–3515. doi: 10.1523/JNEUROSCI.3585-11.2012
Grill-Spector, K., Henson, R., and Martin, A. (2006). Repetition and the brain: neural models of stimulus-specific effects. Trends Cogn. Sci. (Regul. Ed). 10, 14–23. doi: 10.1016/j.tics.2005.11.006
Harrison, S. A., and Tong, F. (2009). Decoding reveals the contents of visual working memory in early visual areas. Nature 458, 632–635. doi: 10.1038/nature07832
Hazy, T. E., Frank, M. J., and O'reilly, R. C. (2006). Banishing the homunculus: making working memory work. Neuroscience 139, 105–118. doi: 10.1016/j.neuroscience.2005.04.067
Hoshi, E., Shima, K., and Tanji, J. (1998). Task-dependent selectivity of movement-related neuronal activity in the primate prefrontal cortex. J. Neurophysiol. 80, 3392–3397.
Ibos, G., Duhamel, J. R., and Ben Hamed, S. (2013). A functional hierarchy within the parietofrontal network in stimulus selection and attention control. J. Neurosci. 33, 8359–8369. doi: 10.1523/JNEUROSCI.4058-12.2013
Inoue, M., and Mikami, A. (2006). Prefrontal activity during serial probe reproduction task: encoding, mnemonic, and retrieval processes. J. Neurophysiol. 95, 1008–1041. doi: 10.1152/jn.00552.2005
Jacob, S. N., and Nieder, A. (2014). Complementary roles for primate frontal and parietal cortex in guarding working memory from distractor stimuli. Neuron 83, 226–237. doi: 10.1016/j.neuron.2014.05.009
Jonides, J., Smith, E. E., Koeppe, R. A., Awh, E., Minoshima, S., and Mintun, M. A. (1993). Spatial working memory in humans as revealed by PET. Nature 363, 623–625. doi: 10.1038/363623a0
Katsuki, F., and Constantinidis, C. (2012). Unique and shared roles of the posterior parietal and dorsolateral prefrontal cortex in cognitive functions. Front. Integr. Neurosci. 6:17. doi: 10.3389/fnint.2012.00017
Katsuki, F., Qi, X. L., Meyer, T., Kostelic, P. M., Salinas, E., and Constantinidis, C. (2014). Differences in intrinsic functional organization between dorsolateral prefrontal and posterior parietal cortex. Cereb. Cortex 24, 2334–2349. doi: 10.1093/cercor/bht087
Kiani, R., Cueva, C. J., Reppas, J. B., Peixoto, D., Ryu, S. I., and Newsome, W. T. (2015). Natural grouping of neural responses reveals spatially segregated clusters in prearcuate cortex. Neuron 85, 1359–1373. doi: 10.1016/j.neuron.2015.02.014
Kim, J. N., and Shadlen, M. N. (1999). Neural correlates of a decision in the dorsolateral prefrontal cortex of the macaque. Nat. Neurosci. 2, 176–185. doi: 10.1038/5739
Kritzer, M. F., and Goldman-Rakic, P. S. (1995). Intrinsic circuit organization of the major layers and sublayers of the dorsolateral prefrontal cortex in the rhesus monkey. J. Comp. Neurol. 359, 131–143. doi: 10.1002/cne.903590109
Kubota, K., and Niki, H. (1971). Prefrontal cortical unit activity and delayed alternation performance in monkeys. J. Neurophysiol. 34, 337–347.
Lafer-Sousa, R., and Conway, B. R. (2013). Parallel, multi-stage processing of colors, faces and shapes in macaque inferior temporal cortex. Nat. Neurosci. 16, 1870–1878. doi: 10.1038/nn.3555
Lara, A. H., and Wallis, J. D. (2014). Executive control processes underlying multi-item working memory. Nat. Neurosci. 17, 876–883. doi: 10.1038/nn.3702
Lauwereyns, J., Sakagami, M., Tsutsui, K., Kobayashi, S., Koizumi, M., and Hikosaka, O. (2001). Responses to task-irrelevant visual features by primate prefrontal neurons. J. Neurophysiol. 86, 2001–2010.
Leavitt, M. L., Pieper, F., Sachs, A., Joober, R., and Martinez-Trujillo, J. C. (2013). Structure of spike count correlations reveals functional interactions between neurons in dorsolateral prefrontal cortex area 8a of behaving primates. PLoS ONE 8:e61503. doi: 10.1371/journal.pone.0061503
Leon, M. I., and Shadlen, M. N. (1999). Effect of expected reward magnitude on the response of neurons in the dorsolateral prefrontal cortex of the macaque. Neuron 24, 415–425. doi: 10.1016/S0896-6273(00)80854-5
Levitt, J. B., Lewis, D. A., Yoshioka, T., and Lund, J. S. (1993). Topography of pyramidal neuron intrinsic connections in macaque monkey prefrontal cortex (areas 9 and 46). J. Comp. Neurol. 338, 360–376. doi: 10.1002/cne.903380304
Li, C. S., Mazzoni, P., and Andersen, R. A. (1999). Effect of reversible inactivation of macaque lateral intraparietal area on visual and memory saccades. J. Neurophysiol. 81, 1827–1838.
Liebe, S., Hoerzer, G. M., Logothetis, N. K., and Rainer, G. (2012). Theta coupling between V4 and prefrontal cortex predicts visual short-term memory performance. Nat. Neurosci. 15, 456–462, S451–S452. doi: 10.1038/nn.3038
Liu, Y., Yttri, E. A., and Snyder, L. H. (2010). Intention and attention: different functional roles for LIPd and LIPv. Nat. Neurosci. 13, 495–500. doi: 10.1038/nn.2496
Logothetis, N. K., and Wandell, B. A. (2004). Interpreting the BOLD signal. Annu. Rev. Physiol. 66, 735–769. doi: 10.1146/annurev.physiol.66.082602.092845
Lund, J. S., and Lewis, D. A. (1993). Local circuit neurons of developing and mature macaque prefrontal cortex: Golgi and immunocytochemical characteristics. J. Comp. Neurol. 328, 282–312. doi: 10.1002/cne.903280209
Mante, V., Sussillo, D., Shenoy, K. V., and Newsome, W. T. (2013). Context-dependent computation by recurrent dynamics in prefrontal cortex. Nature 503, 78–84. doi: 10.1038/nature12742
Markowitz, D. A., Curtis, C. E., and Pesaran, B. (2015). Multiple component networks support working memory in prefrontal cortex. Proc. Natl. Acad. Sci. U.S.A. 112, 11084–11089. doi: 10.1073/pnas.1504172112
Marshuetz, C., Smith, E. E., Jonides, J., Degutis, J., and Chenevert, T. L. (2000). Order information in working memory: fMRI evidence for parietal and prefrontal mechanisms. J. Cogn. Neurosci. 12, 130–144. doi: 10.1162/08989290051137459
Mendoza-Halliday, D., Torres, S., and Martinez-Trujillo, J. C. (2014). Sharp emergence of feature-selective sustained activity along the dorsal visual pathway. Nat. Neurosci. 17, 1255–1262. doi: 10.1038/nn.3785
Meyer, T., Qi, X. L., Stanford, T. R., and Constantinidis, C. (2011). Stimulus selectivity in dorsal and ventral prefrontal cortex after training in working memory tasks. J. Neurosci. 31, 6266–6276. doi: 10.1523/JNEUROSCI.6798-10.2011
Meyers, E. M., Qi, X. L., and Constantinidis, C. (2012). Incorporation of new information into prefrontal cortical activity after learning working memory tasks. Proc. Natl. Acad. Sci. U.S.A. 109, 4651–4656. doi: 10.1073/pnas.1201022109
Miller, E. K., and Cohen, J. D. (2001). An integrative theory of prefrontal cortex function. Annu. Rev. Neurosci. 24, 167–202. doi: 10.1146/annurev.neuro.24.1.167
Miller, E. K., Erickson, C. A., and Desimone, R. (1996). Neural mechanisms of visual working memory in prefrontal cortex of the macaque. J. Neurosci. 16, 5154–5167.
Miller, E. K., Li, L., and Desimone, R. (1991). A neural mechanism for working and recognition memory in inferior temporal cortex. Science 254, 1377–1379. doi: 10.1126/science.1962197
Miller, E. K., Li, L., and Desimone, R. (1993). Activity of neurons in anterior inferior temporal cortex during a short-term memory task. J. Neurosci. 13, 1460–1478.
Milner, B. (1963). Effects of different brain lesions on card sorting. Arch. Neurol. 9, 100–110. doi: 10.1001/archneur.1963.00460070100010
Miyashita, Y., and Chang, H. S. (1988). Neuronal correlate of pictorial short-term memory in the primate temporal cortex. Nature 331, 68–70. doi: 10.1038/331068a0
Mongillo, G., Barak, O., and Tsodyks, M. (2008). Synaptic theory of working memory. Science 319, 1543–1546. doi: 10.1126/science.1150769
Nakamura, K., and Kubota, K. (1995). Mnemonic firing of neurons in the monkey temporal pole during a visual recognition memory task. J. Neurophysiol. 74, 162–178.
Naya, Y., Yoshida, M., and Miyashita, Y. (2001). Backward spreading of memory-retrieval signal in the primate temporal cortex. Science 291, 661–664. doi: 10.1126/science.291.5504.661
Nieder, A., Freedman, D. J., and Miller, E. K. (2002). Representation of the quantity of visual items in the primate prefrontal cortex. Science 297, 1708–1711. doi: 10.1126/science.1072493
Niki, H. (1974). Prefrontal unit activity during delayed alternation in the monkey. I. Relation to direction of response. Brain Res. 68, 185–196. doi: 10.1016/0006-8993(74)90388-6
Offen, S., Schluppeck, D., and Heeger, D. J. (2009). The role of early visual cortex in visual short-term memory and visual attention. Vision Res. 49, 1352–1362. doi: 10.1016/j.visres.2007.12.022
O Scalaidhe, S. P., Wilson, F. A., and Goldman-Rakic, P.S. (1999). Face-selective neurons during passive viewing and working memory performance of rhesus monkeys: evidence for intrinsic specialization of neuronal coding. Cereb. Cortex 9, 459–475. doi: 10.1093/cercor/9.5.459
O Scalaidhe, S., Wilson, F. A., and Goldman-Rakic, P.S. (1997). Areal segregation of face-processing neurons in prefrontal cortex. Science 278, 1135–1138. doi: 10.1126/science.278.5340.1135
Owen, A. M., Stern, C. E., Look, R. B., Tracey, I., Rosen, B. R., and Petrides, M. (1998). Functional organization of spatial and nonspatial working memory processing within the human lateral frontal cortex. Proc. Natl. Acad. Sci. U.S.A. 95, 7721–7726. doi: 10.1073/pnas.95.13.7721
Pasternak, T., and Greenlee, M. W. (2005). Working memory in primate sensory systems. Nat. Rev. Neurosci. 6, 97–107. doi: 10.1038/nrn1603
Pesaran, B., Pezaris, J. S., Sahani, M., Mitra, P. P., and Andersen, R. A. (2002). Temporal structure in neuronal activity during working memory in macaque parietal cortex. Nat. Neurosci. 5, 805–811. doi: 10.1038/nn890
Petrides, M., and Pandya, D. N. (1988). Association fiber pathways to the frontal cortex from the superior temporal region in the rhesus monkey. J. Comp. Neurol. 273, 52–66. doi: 10.1002/cne.902730106
Postle, B. R. (2006). Working memory as an emergent property of the mind and brain. Neuroscience 139, 23–38. doi: 10.1016/j.neuroscience.2005.06.005
Pucak, M. L., Levitt, J. B., Lund, J. S., and Lewis, D. A. (1996). Patterns of intrinsic and associational circuitry in monkey prefrontal cortex. J. Comp. Neurol. 376, 614–630. doi: 10.1002/(SICI)1096-9861(19961223)376:4<614::AID-CNE9>3.0.CO;2-4
Qi, X. L., and Constantinidis, C. (2013). Neural changes after training to perform cognitive tasks. Behav. Brain Res. 241, 235–243. doi: 10.1016/j.bbr.2012.12.017
Qi, X. L., Elworthy, A. C., Lambert, B. C., and Constantinidis, C. (2015a). Representation of remembered stimuli and task information in the monkey dorsolateral prefrontal and posterior parietal cortex. J. Neurophysiol. 113, 44–57. doi: 10.1152/jn.00413.2014
Qi, X. L., Katsuki, F., Meyer, T., Rawley, J. B., Zhou, X., Douglas, K. L., et al. (2010). Comparison of neural activity related to working memory in primate dorsolateral prefrontal and posterior parietal cortex. Front. Syst. Neurosci. 4:12. doi: 10.3389/fnsys.2010.00012
Qi, X. L., Meyer, T., Stanford, T. R., and Constantinidis, C. (2011). Changes in prefrontal neuronal activity after learning to perform a spatial working memory task. Cereb. Cortex 21, 2722–2732. doi: 10.1093/cercor/bhr058
Qi, X. L., Meyer, T., Stanford, T. R., and Constantinidis, C. (2012). Neural correlates of a decision variable before learning to perform a Match/Nonmatch task. J. Neurosci. 32, 6161–6169. doi: 10.1523/JNEUROSCI.6365-11.2012
Qi, X. L., Zhou, X., and Constantinidis, C. (2015b). “Neurophysiological mechanisms of working memory: cortical specialization & plasticity,” in Attention and Performance XXV, eds. P. Jolicoeur, C. Lefebre and J. C. Martinez-Trujillo (London: Academic Press), 171–186. doi: 10.1016/b978-0-12-801371-7.00014-4
Quintana, J., Yajeya, J., and Fuster, J. M. (1988). Prefrontal representation of stimulus attributes during delay tasks. I. Unit activity in cross-temporal integration of sensory and sensory-motor information. Brain Res. 474, 211–221. doi: 10.1016/0006-8993(88)90436-2
Rainer, G., Asaad, W. F., and Miller, E. K. (1998). Selective representation of relevant information by neurons in the primate prefrontal cortex. Nature 393, 577–579. doi: 10.1038/31235
Rainer, G., and Miller, E. K. (2000). Effects of visual experience on the representation of objects in the prefrontal cortex. Neuron 27, 179–189. doi: 10.1016/S0896-6273(00)00019-2
Rao, S. C., Rainer, G., and Miller, E. K. (1997). Integration of what and where in the primate prefrontal cortex. Science 276, 821–824. doi: 10.1126/science.276.5313.821
Rao, S. G., Williams, G. V., and Goldman-Rakic, P. S. (1999). Isodirectional tuning of adjacent interneurons and pyramidal cells during working memory: evidence for microcolumnar organization in PFC. J. Neurophysiol. 81, 1903–1916.
Rao, S. G., Williams, G. V., and Goldman-Rakic, P. S. (2000). Destruction and creation of spatial tuning by disinhibition: GABA(A) blockade of prefrontal cortical neurons engaged by working memory. J. Neurosci. 20, 485–494.
Rawley, J. B., and Constantinidis, C. (2009). Neural correlates of learning and working memory in the primate posterior parietal cortex. Neurobiol. Learn. Mem. 91, 129–138. doi: 10.1016/j.nlm.2008.12.006
Renart, A., Song, P., and Wang, X-J. (2003). Robust spatial working memory through homeostatic synaptic scaling in heterogeneous cortical networks. Neuron 38, 473–485. doi: 10.1016/S0896-6273(03)00255-1
Rigotti, M., Barak, O., Warden, M. R., Wang, X. J., Daw, N. D., Miller, E. K., et al. (2013). The importance of mixed selectivity in complex cognitive tasks. Nature 497, 585–590. doi: 10.1038/nature12160
Roelfsema, P. R. (2015). The role of the different layers of primary visual cortex in working memory. J. Vis. 15:1406. doi: 10.1167/15.12.1406
Rossi, A. F., Bichot, N. P., Desimone, R., and Ungerleider, L. G. (2007). Top down attentional deficits in macaques with lesions of lateral prefrontal cortex. J. Neurosci. 27, 11306–11314. doi: 10.1523/JNEUROSCI.2939-07.2007
Roux, F., and Uhlhaas, P. J. (2014). Working memory and neural oscillations: alpha–gamma versus theta–gamma codes for distinct WM information? Trends Cogn. Sci. (Regul. Ed). 18, 16–25. doi: 10.1016/j.tics.2013.10.010
Roy, J. E., Buschman, T. J., and Miller, E. K. (2014). PFC neurons reflect categorical decisions about ambiguous stimuli. J. Cogn. Neurosci. 26, 1283–1291. doi: 10.1162/jocn_a_00568
Roy, N., Murray, J. D., Romo, R., Constantinidis, C., and Wang, X. J. (2013). Dynamics and temporal stability of neural representations in the prefrontal cortex during encoding and maintenance of working memory. Soc. Neurosci. Abstr. 388, 304.
Sakagami, M., Tsutsui, K., Lauwereyns, J., Koizumi, M., Kobayashi, S., and Hikosaka, O. (2001). A code for behavioral inhibition on the basis of color, but not motion, in ventrolateral prefrontal cortex of macaque monkey. J. Neurosci. 21, 4801–4808.
Sakai, K., Rowe, J. B., and Passingham, R. E. (2002). Active maintenance in prefrontal area 46 creates distractor-resistant memory. Nat. Neurosci. 5, 479–484. doi: 10.1038/nn846
Salazar, R. F., Dotson, N. M., Bressler, S. L., and Gray, C. M. (2012). Content-specific fronto-parietal synchronization during visual working memory. Science 338, 1097–1100. doi: 10.1126/science.1224000
Seamans, J. K., Durstewitz, D., Christie, B. R., Stevens, C. F., and Sejnowski, T. J. (2001). Dopamine D1/D5 receptor modulation of excitatory synaptic inputs to layer V prefrontal cortex neurons. Proc. Natl. Acad. Sci. U.S.A. 98, 301–306. doi: 10.1073/pnas.98.1.301
Shima, K., Isoda, M., Mushiake, H., and Tanji, J. (2007). Categorization of behavioural sequences in the prefrontal cortex. Nature 445, 315–318. doi: 10.1038/nature05470
Siegel, M., Warden, M. R., and Miller, E. K. (2009). Phase-dependent neuronal coding of objects in short-term memory. Proc. Natl. Acad. Sci. U.S.A. 106, 21341–21346. doi: 10.1073/pnas.0908193106
Sigala, N., Kusunoki, M., Nimmo-Smith, I., Gaffan, D., and Duncan, J. (2008). Hierarchical coding for sequential task events in the monkey prefrontal cortex. Proc. Natl. Acad. Sci. U.S.A. 105, 11969–11974. doi: 10.1073/pnas.0802569105
Sigala, N., and Logothetis, N. K. (2002). Visual categorization shapes feature selectivity in the primate temporal cortex. Nature 415, 318–320. doi: 10.1038/415318a
Smith, E. E., and Kosslyn, S. M. (2007). Cognitive Psychology: Mind and Brain. Upper Saddle River, NJ: Pearson Education.
Sommer, M. A., and Tehovnik, E. J. (1997). Reversible inactivation of macaque frontal eye field. Exp. Brain Res. 116, 229–249. doi: 10.1007/PL00005752
Sreenivasan, K. K., Curtis, C. E., and D'Esposito, M. (2014a). Revisiting the role of persistent neural activity during working memory. Trends Cogn. Sci. (Regul. Ed). 18, 82–89. doi: 10.1016/j.tics.2013.12.001
Sreenivasan, K. K., Vytlacil, J., and D'Esposito, M. (2014b). Distributed and dynamic storage of working memory stimulus information in extrastriate cortex. J. Cogn. Neurosci. 26, 1141–1153. doi: 10.1162/jocn_a_00556
Steinmetz, M. A., Connor, C. E., Constantinidis, C., and Mclaughlin, J. R. (1994). Covert attention suppresses neuronal responses in area 7a of the posterior parietal cortex. J. Neurophysiol. 72, 1020–1023.
Stern, C. E., Sherman, S. J., Kirchhoff, B. A., and Hasselmo, M. E. (2001). Medial temporal and prefrontal contributions to working memory tasks with novel and familiar stimuli. Hippocampus 11, 337–346. doi: 10.1002/hipo.1048
Stokes, M. G., Kusunoki, M., Sigala, N., Nili, H., Gaffan, D., and Duncan, J. (2013). Dynamic coding for cognitive control in prefrontal cortex. Neuron 78, 364–375. doi: 10.1016/j.neuron.2013.01.039
Sugase-Miyamoto, Y., Liu, Z., Wiener, M. C., Optican, L. M., and Richmond, B. J. (2008). Short-term memory trace in rapidly adapting synapses of inferior temporal cortex. PLoS Comput. Biol. 4:e1000073. doi: 10.1371/journal.pcbi.1000073
Supèr, H., Spekreijse, H., and Lamme, V. A. (2001). A neural correlate of working memory in the monkey primary visual cortex. Science 293, 120–124. doi: 10.1126/science.1060496
Suzuki, M., and Gottlieb, J. (2013). Distinct neural mechanisms of distractor suppression in the frontal and parietal lobe. Nat. Neurosci. 16, 98–104. doi: 10.1038/nn.3282
Swaminathan, S. K., and Freedman, D. J. (2012). Preferential encoding of visual categories in parietal cortex compared with prefrontal cortex. Nat. Neurosci. 15, 315–320. doi: 10.1038/nn.3016
Takeda, K., and Funahashi, S. (2002). Prefrontal task-related activity representing visual cue location or saccade direction in spatial working memory tasks. J. Neurophysiol. 87, 567–588.
Todd, J. J., and Marois, R. (2004). Capacity limit of visual short-term memory in human posterior parietal cortex. Nature 428, 751–754. doi: 10.1038/nature02466
Todd, J. J., and Marois, R. (2005). Posterior parietal cortex activity predicts individual differences in visual short-term memory capacity. Cogn. Affect. Behav. Neurosci. 5, 144–155. doi: 10.3758/CABN.5.2.144
Tong, F., and Pratte, M. S. (2012). Decoding patterns of human brain activity. Annu. Rev. Psychol. 63, 483–509. doi: 10.1146/annurev-psych-120710-100412
Ungerleider, L. G., Courtney, S. M., and Haxby, J. V. (1998). A neural system for human visual working memory. Proc. Natl. Acad. Sci. U.S.A. 95, 883–890. doi: 10.1073/pnas.95.3.883
Wallis, J. D., Anderson, K. C., and Miller, E. K. (2001). Single neurons in prefrontal cortex encode abstract rules. Nature 411, 953–956. doi: 10.1038/35082081
Wang, M., Gamo, N. J., Yang, Y., Jin, L. E., Wang, X.-J., Laubach, M., et al. (2011). Neuronal basis of age-related working memory decline. Nature 476, 210–213. doi: 10.1038/nature10243
Wang, M., Yang, Y., Wang, C. J., Gamo, N. J., Jin, L. E., Mazer, J. A., et al. (2013). NMDA receptors subserve persistent neuronal firing during working memory in dorsolateral prefrontal cortex. Neuron 77, 736–749. doi: 10.1016/j.neuron.2012.12.032
Wang, X. J. (2001). Synaptic reverberation underlying mnemonic persistent activity. Trends Neurosci. 24, 455–463. doi: 10.1016/S0166-2236(00)01868-3
Wang, X. J., Tegnér, J., Constantinidis, C., and Goldman-Rakic, P. S. (2004). Division of labor among distinct subtypes of inhibitory neurons in a cortical microcircuit of working memory. Proc. Natl. Acad. Sci. U.S.A. 101, 1368–1373. doi: 10.1073/pnas.0305337101
Wardak, C., Olivier, E., and Duhamel, J. R. (2002). Saccadic target selection deficits after lateral intraparietal area inactivation in monkeys. J. Neurosci. 22, 9877–9884.
Wardak, C., Olivier, E., and Duhamel, J. R. (2004). A deficit in covert attention after parietal cortex inactivation in the monkey. Neuron 42, 501–508. doi: 10.1016/S0896-6273(04)00185-0
Webster, M. J., Bachevalier, J., and Ungerleider, L. G. (1994). Connections of inferior temporal areas TEO and TE with parietal and frontal cortex in macaque monkeys. Cereb. Cortex 4, 470–483. doi: 10.1093/cercor/4.5.470
White, I. M., and Wise, S. P. (1999). Rule-dependent neuronal activity in the prefrontal cortex. Exp. Brain Res. 126, 315–335. doi: 10.1007/s002210050740
Wilke, M., Kagan, I., and Andersen, R. A. (2012). Functional imaging reveals rapid reorganization of cortical activity after parietal inactivation in monkeys. Proc. Natl. Acad. Sci. U.S.A. 109, 8274–8279. doi: 10.1073/pnas.1204789109
Wilson, F. A., O Scalaidhe, S. P., and Goldman-Rakic, P. S. (1993). Dissociation of object and spatial processing domains in primate prefrontal cortex. Science 260, 1955–1958. doi: 10.1126/science.8316836
Wimmer, K., Nykamp, D. Q., Constantinidis, C., and Compte, A. (2014). Bump attractor dynamics in prefrontal cortex explains behavioral precision in spatial working memory. Nat. Neurosci. 17, 431–439. doi: 10.1038/nn.3645
Woloszyn, L., and Sheinberg, D. L. (2009). Neural dynamics in inferior temporal cortex during a visual working memory task. J. Neurosci. 29, 5494–5507. doi: 10.1523/JNEUROSCI.5785-08.2009
Xing, Y., Ledgeway, T., Mcgraw, P. V., and Schluppeck, D. (2013). Decoding working memory of stimulus contrast in early visual cortex. J. Neurosci. 33, 10301–10311. doi: 10.1523/JNEUROSCI.3754-12.2013
Xu, Y., and Chun, M. M. (2006). Dissociable neural mechanisms supporting visual short-term memory for objects. Nature 440, 91–95. doi: 10.1038/nature04262
Yang, C. R., and Seamans, J. K. (1996). Dopamine D1 receptor actions in layers V-VI rat prefrontal cortex neurons in vitro: modulation of dendritic-somatic signal integration. J. Neurosci. 16, 1922–1935.
Zaksas, D., and Pasternak, T. (2006). Directional signals in the prefrontal cortex and in area MT during a working memory for visual motion task. J. Neurosci. 26, 11726–11742. doi: 10.1523/JNEUROSCI.3420-06.2006
Zhou, X., Katsuki, F., Qi, X. L., and Constantinidis, C. (2012). Neurons with inverted tuning during the delay periods of working memory tasks in the dorsal prefrontal and posterior parietal cortex. J. Neurophysiol. 108, 31–38. doi: 10.1152/jn.01151.2011
Keywords: prefrontal cortex, monkey, neurophysiology, fMRI, neuron
Citation: Riley MR and Constantinidis C (2016) Role of Prefrontal Persistent Activity in Working Memory. Front. Syst. Neurosci. 9:181. doi: 10.3389/fnsys.2015.00181
Received: 12 September 2015; Accepted: 07 December 2015;
Published: 05 January 2016.
Edited by:
Natasha Sigala, University of Sussex, UKReviewed by:
Amy F. T. Arnsten, Yale University School of Medicine, USAJulio Martinez-Trujillo, University of Western Ontario, Canada
Copyright © 2016 Riley and Constantinidis. This is an open-access article distributed under the terms of the Creative Commons Attribution License (CC BY). The use, distribution or reproduction in other forums is permitted, provided the original author(s) or licensor are credited and that the original publication in this journal is cited, in accordance with accepted academic practice. No use, distribution or reproduction is permitted which does not comply with these terms.
*Correspondence: Christos Constantinidis, cconstan@wakehealth.edu