- 1 Cortical Networks and Cognitive Functions, Max Planck Institute for Human Cognitive and Brain Sciences, Leipzig, Germany
- 2 Cortical Networks Group, Max Planck Institute for Neurological Research, Cologne, Germany
This review focuses on the role of long-range connectivity as one element of brain structure that is of key importance for the functional–anatomical organization of the cortex. In this context, we discuss the putative guiding principles for mapping brain function and structure onto the cortical surface. Such mappings reveal a high degree of functional–anatomical segregation. Given that brain regions frequently maintain characteristic connectivity profiles and the functional repertoire of a cortical area is closely related to its anatomical connections, long-range connectivity may be used to define segregated cortical areas. This methodology is called connectivity-based parcellation. Within this framework, we investigate different techniques to estimate connectivity profiles with emphasis given to non-invasive methods based on diffusion magnetic resonance imaging (dMRI) and diffusion tractography. Cortical parcellation is then defined based on similarity between diffusion tractograms, and different clustering approaches are discussed. We conclude that the use of non-invasively acquired connectivity estimates to characterize the functional–anatomical organization of the brain is a valid, relevant, and necessary endeavor. Current and future developments in dMRI technology, tractography algorithms, and models of the similarity structure hold great potential for a substantial improvement and enrichment of the results of the technique.
Mapping of Structure and Function onto the Cortex
The human brain, in regard to both its function and structure, has been subject to intensive investigation for more than a century. Classical neuroanatomy and electrophysiology as well as modern brain imaging methods have gathered a wealth of information bearing potential cues for understanding the complex interaction of the brain with its environment. In this line, it is particularly interesting how structure, or anatomy, of the brain relates to its function and hence to its astonishing performance. Here, we will specifically address the question, how structural details, such as obtained by modern imaging methods, can be described and organized in a functionally meaningful way.
The interaction of the brain with its environment is characterized by a very large number of functional features at different hierarchical levels. For example, visual input can be described by low-level functional features, such as position in the visual field, color, and intensity, but also by higher-level functional features like orientation, motion direction and even object type. Neurons, but also populations of neurons, are tuned to certain loci (areas or parts of an area) in this functional feature space. In the perceptual domain, these areas are also referred to as receptive fields. For example, there are cells responding to a particular section of the visual field and others that specifically respond to faces. This tuning in the functional feature space must be paralleled in the structural feature space of the neural tissue. In other words, neurons with different functions can be assumed to also differ in terms of structure. Relevant structural traits include neuronal properties, such as types and distribution of neurotransmitters and receptors, number and distribution of synapses, morphology of dendritic and axonal arbors, but also the connectivity between neurons, both intrinsically (local circuits) and extrinsically (inter-regional pathways).
In sum, a high-dimensional functional feature space maps onto a high-dimensional structural feature space. Both spaces then have to map onto the two-dimensional cortical sheet (see Figure 1). Moreover, a vast number of studies have shown that neither functional tuning nor structural properties of neurons are distributed over the cortex at random (Toga et al., 2006; Op De Beeck et al., 2008). Instead, structure and function are arranged in distinct patterns. It has been a major issue in neuroscience to document these patterns, thereby charting the brain. Moreover, it seems worthwhile to investigate the origin and the meaning of the observed brain maps.
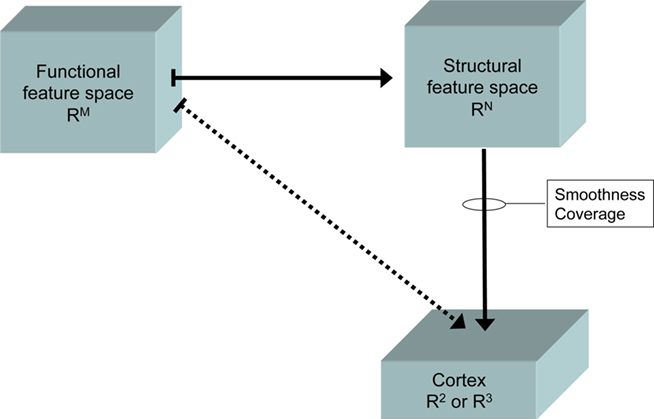
Figure 1. Schematic drawing of the mappings between functional features space (e. g., stimulus features, movement parameters, cognitive operations, etc.), structural feature space (cell morphology, connectivity, neurotransmitter type, etc.), and cerebral cortex, modeled as two-dimensional sheet (R2) or three-dimensional volume (R3).
One prevailing property of this mapping seems to arise from the maximum similarity between topological neighbors (Durbin and Mitchison, 1990; Tononi et al., 1992), which we will refer to as feature map smoothness. This applies to both functional and structural features. For example, it is a classical finding in neuroanatomy that cortical units with similar myeloarchitectural, cytoarchitectural, and receptorarchitectural profiles tend to cluster together and form compact areas (Kaas, 1989; Friston, 1994; Tononi et al., 1994; Miller, 1996; Aertsen and Preißl, 1999; Stephan et al., 2000). Another example is provided by the numerous topographic maps in the sensory and motor cortices, where neighboring cortical sites represent similar functional properties, such as adjacent sound frequencies in the tonotopic maps of auditory cortex (e.g., Kaas and Hackett, 2000; Petkov et al., 2006), neighboring positions in a visual field represented in the retinotopic map (e.g., Silver and Kastner, 2009; Smith and Häusser, 2010), or related body parts in the somatotopic maps (e.g., Graziano, 2006; Meier et al., 2008). The second important principle of this high-to-low dimensional mapping is its coverage (Barone et al., 2000), meaning that the entire relevant feature space must be represented adequately. This implies that some features are represented in a much more fine-grained way (e.g., somatotopic maps of the fingers) than others.
There are a number of possible ontogenetic and phylogenetic foundations to these mapping principles (see, e.g., Goodhill, 2007; Graziano and Aflalo, 2007a,b). First, they might be epiphenomenal consequences of the fact that during development, axonal growth is guided by chemical gradients, thereby ensuring a certain topographic continuity in connectivity (Gierer and Müller, 1995; Tuch et al., 2005). Second, similar connectivity leads to a correlated input between neighboring units, thereby increasing mutual connectivity and functional similarity by Hebbian learning (Graziano and Aflalo, 2007a). Such an upstream continuation of existing maps is, for instance, corroborated by the finding that the size of the lateral geniculate nucleus (LGN) causally influences the size of V1 (see Ragsdale and Grove, 2001). Third, functional similarity between neighboring units minimizes the required wiring and may contribute to the high efficiency for information transfer (Bullmore and Sporns, 2009; Sporns, 2010), thereby forming a phylogenetic driving force toward smooth feature maps (Tononi et al., 1992; Zucker and Regehr, 2002; Chklovskii and Koulakov, 2004).
It has been shown in a number of computer simulations that self-organization maximizing feature map smoothness leads to anatomically plausible cortical compartmentalization. The most widely applied approach is the Kohonen model (Braitenberg, 1962; Durbin and Mitchison, 1990; Kohonen, 2006), also referred to as self-organized map (SOM) approach. By using this model, biologically plausible maps of the visual cortex have been achieved (Saarinen and Kohonen, 1985; Obermayer et al., 1990; Barone et al., 2000). In the motor domain, Graziano and Aflalo (2007a) demonstrated that mapping of the motor repertoire onto the cortical sheet could be explained by the same principle. For a more comprehensive review of different modeling approaches to cortical map formation, see Goodhill (2007).
The necessity to map high-dimensional feature spaces onto the two-dimensional cortical sheet, together with the principles of feature map smoothness and coverage, results in a variety of possible cortical compartmentalizations based on different structural and functional criteria that are not necessarily congruent, but quite often complementary. In the context of functional brain imaging, both in basic research and for clinical application, such maps are of great importance (Op De Beeck et al., 2008). For example, they are needed for model construction, for the prediction and interpretation of functional activity, and as prior knowledge in the otherwise non-unique reconstruction of the generators of M/EEG signals. In the past, mainly local structural and functional criteria have been used to define cortical organization, while aspects of connectivity and information exchange between distant areas have been largely neglected. In the following, we argue for extrinsic (i.e., long-range) connectivity patterns being among the most relevant structural features with respect to brain function. Such connectivity patterns exist and can be measured in the structural as well as in the functional domain. In the functional domain, they have been established on the basis of correlative relationships between distant brain activities, detected by, for example, functional magnetic resonance imaging (fMRI) or magnetoencephalography (for recent reviews on concept and techniques, see Fingelkurts and Kahkonen, 2005; Li et al., 2009; Nolte and Muller, 2010; Van Den Heuvel and Pol, 2010; Deco et al., 2011). In particular, fMRI resting-state activity (i.e., without any explicit stimulus or task) has been used in numerous recent studies to characterize potential networks of information exchange (e.g., Cohen et al., 2008; Zhang et al., 2008). Here, however, we focus on effective ways to obtain approximations for long-range anatomical connectivity using diffusion magnetic resonance imaging (dMRI) and diffusion tractography, and we discuss previous achievements and current perspectives in using this technique for generating functional–anatomical maps of the cerebral cortex.
Functional Integration and Brain Networks
From the above, it follows that mapping of brain function onto the cortical surface results in a high degree of functional–anatomical segregation, meaning that different functions are localized in separate cortical areas. Segregation is one important organizational principle of the brain, resulting in the notion of functional localization and the occurrence of sensory feature maps (Op De Beeck et al., 2008). Segregation also appears to be relevant at a wide range of spatial levels, from minicolumns comprising only 100–200 neurons (Mountcastle, 1997; Buxhoeveden and Casanova, 2002), to macroscopic areas covering several square centimeters of cortex (Zilles, 2004). It is complemented by the second essential feature of brain architecture, that is, functional integration (Varela et al., 2001), denoting interaction and information exchange between segregated parts of the brain (for an introduction to the foundation of functional–anatomical segregation and integration, see Zeki and Shipp, 1988; Tononi et al., 1994). Both segregation and integration motivate the concept of brain networks, where the nodes of these networks describe functionally distinct brain areas (Bullmore and Sporns, 2009; Sporns, 2010). Brain areas are distinct if they specifically contribute to different brain functions, which are, to a large extent, determined by their input and output channels (i.e., by their connectivity with other areas). The connections, in turn, form the edges of a network allowing the exchange of information and thereby embody the principle of integration. Hence, connectivity is the decisive criterion for defining brain networks, not only with respect to network edges, but also with respect to the definition of network nodes. Consequently, a specific network node can be understood as a brain area whose connectivity to other brain areas is (relatively) homogeneous and definitively distinct from other areas.
In the context of brain function, connectivity may be understood in terms of information transfer, which is quantified as the causal influence the functional state of a brain structure has on the functional state of another one. This concept is referred to as effective connectivity (Friston, 1994; Aertsen and Preißl, 1999; Büchel et al., 1999). In practice, effective connectivity is often not directly accessible. To provide a causal relationship would usually require a specific experimental perturbation of the system. This could be done, for example, either by creating a (transient or permanent) lesion in one brain structure and observing how this effects functional activity in other structures, or by pharmacological intervention. In particular in human subjects, this is often not possible for ethical reasons. Instead, functional connectivity (Friston et al., 1993) is measured. This describes a correlative relationship between brain activities and is sensitive but not specific to effective connectivity. For example, the activity in two homolog areas of the two retinas is functionally connected (i.e., correlated), although there is certainly no causal relationship between them – instead, common input from the environment causes the correlation. By using models one can infer effective connectivity from functional connectivity, for example within the dynamic causal modeling (DCM) framework (David et al., 2002; Friston et al., 2003). The validity of this inference then depends on the correctness of the model assumptions.
Likewise, anatomical or structural connectivity subsumes the entire physical basis for information transfer between neurons, neuronal populations or brain areas. It involves a multitude of physiological and anatomical parameters, such as number and properties of axons; number, distribution, and properties of synapses; type and abundance of neurotransmitters and neuroreceptors and many more (Figure 2). Generally, these aspects of structural connectivity form a processing chain, where each link crucially limits the entire process. For example, the membrane potential of one neuron can directly influence the state of another neuron only if there are axons running between these neurons, if synapses are attached to the target neuron, if neurotransmitters are released and if receptors are present that respond to the neurotransmitters. Therefore, in many cases, it might be already fairly revealing to consider one of these aspects, such as the course of axons, which is referred to as axonal connectivity.
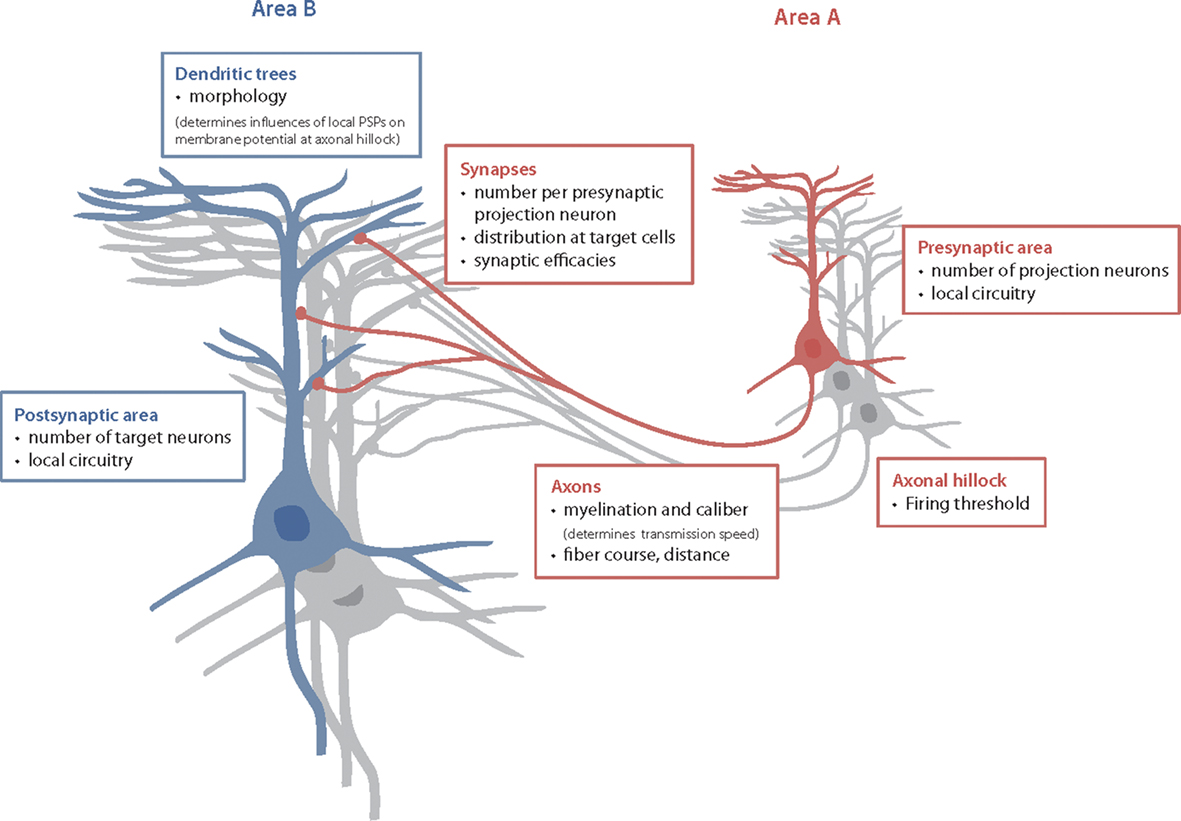
Figure 2. Contributing factors for anatomical connectivity. One possible quantitative definition of anatomical connectivity is the potential influence of the mean membrane potential in one brain area onto the mean membrane potential of another area (Yo et al., 2009). In this line, the anatomical connectivity from area A to area B depends on: (1) the number of cells in the presynaptic area A, the local circuitry there and the number of axons leaving this area for the respective target area B (= number of projection neurons in A); (2) the distribution of axonal diameter and myelination of the axons as well as the distance between A and B and the exact course of the fibers – all these factors determine the distribution of transmission speeds; (3) the number of synapses made with cells in area B, their distribution over the target cells and the synaptic efficacies, which depend on numerous factors, including abundance of neurotransmitters and receptors, (4) the number of cells in area B and the local circuitry there.
Axons densely connect neurons within a cortical area, while different cortical areas are linked through much more specific fiber pathways, which originate and terminate in specific layers (Barbas and Rempel-Clower, 1997). Hence, cortical areas are not broadly interconnected in an all-to-all fashion (Kaas, 1989) – only 40% of all possible cortico-cortical connections are estimated to actually exist (Felleman and Van Essen, 1991). As a consequence, structural brain networks are locally dense and globally sparse, i.e., they have small-world properties, supporting efficient parallel information transfer at relatively low physical connection cost (Kaiser and Hilgetag, 2006; Achard and Bullmore, 2007; Sporns, 2011). It is important to note that the specific choice of the network nodes strongly influences quantitative estimates of organizational parameters – e.g., small-worldness, clustering, path length, and efficiency – although it leaves qualitative network properties – e.g., whether the network exhibits small-worldness or not – relatively unaffected (Zalesky et al., 2010). From a network analysis point of view, it therefore does matter for global network properties how the functional units of the brain are defined.
Besides these organizational principles underlying anatomical connectivity, an emerging body of evidence reveals that cognitive function also derives from large-scale networks (Bressler and Menon, 2010). The actual existence of such large-scale networks has been established by neuroanatomical studies as distributed cortical areas interconnected by fiber pathways (anatomical connectivity), as well as by neurophysiological studies demonstrating the functional co-activation (i.e., functional connectivity) of multiple areas (Bressler, 2002). Processing in a large-scale network may proceed concurrently at multiple levels, with information shared across levels.
Structure–Function Relationship in Modeling and Imaging
Even if we eventually see brain function in its entirety as a product of the complete brain structure and its interaction with the external environment, this appears very different in practice when interpreting observed structural data and modeling brain function. The reason is that it is only possible to access and account for some aspects of structure and function. Consequently, we cannot say any more that function (as we measure it) is fully determined by structure (given the aperture of the measurement), and the influence of structure on function should be described as a constraint rather than as a determinant. In particular, it is generally accepted that anatomical (more specifically, axonal) connectivity provides important constraints to effective connectivity. For example, it has been shown that the functional repertoire of a cortical area (the “functional fingerprint”) is closely related to the pattern of its anatomical connections (the “connectional fingerprint,” Passingham et al., 2002). Similarly, network analyses of anatomical connectivity (Hilgetag et al., 2000; Markov et al., 2010) and functional interactions in the Macaque cortex (Stephan et al., 2000) have revealed similar clustering in the cortical network and indicated that, both from an anatomical and functional perspective, the Macaque cortex possesses small-world properties. Finally, a number of analytical and simulation studies have recently started to explore how different types of structural network topologies are linked to different types of neuronal dynamics (Strogatz, 2001; Sporns et al., 2002; Honey et al., 2007; Ghosh et al., 2008; Bullmore and Sporns, 2009; Deco et al., 2009). An interesting review on this topic with a specific focus on resting-state activity is provided by Deco et al. (2011).
It is important to stress that axonal connectivity constrains, but does not exhaustively determine, effective connectivity. It is worthwhile discussing some of the reasons for this, which are all rooted in the above-mentioned incompleteness of functional and structural information. First, axonal connectivity is only one aspect of structural connectivity (see Figure 2). For example, the number, distribution, and microanatomical structure of synapses also play a role. Moreover, the function of a synapse depends on its recent history, i.e., in the absence of any structural changes of the synapse per se, marked facilitation or depression of synaptic transmission can occur at a timescale of milliseconds (Zucker and Regehr, 2002). Various mechanisms exist by which synaptic connections can be enabled or disabled in a dynamic fashion at the timescale of milliseconds. These mechanisms include gating and gain control mechanisms, which render synaptic transmission dependent on the current membrane potential and the history of other synaptic inputs nearby. These transient and non-linear effects are important for explaining the dynamics of functional interactions among neuronal populations (Friston, 1995; McIntosh, 2000; Salinas and Sejnowski, 2001; Stephan et al., 2008). A second, and probably more important, reason why there is no one-to-one mapping between axonal and effective connectivity is that the structural presence, and even the molecular structure, of a particular synaptic connection does not determine whether it will be engaged during a particular functional process. This depends on whether the presynaptic structure is activated in the first place, which is determined by the network as whole. Although one might argue that, in the end, all these phenomena depend on structural properties of the brain as a whole, in practical brain imaging and modeling, this leads to an apparent “extrastructural” component in the determination of brain function.
In any case, anatomical connectivity is important to guide the construction of biologically realistic models of effective connectivity; and recently the degree to which these models actually profit from anatomical constraints has been formally investigated (Stephan et al., 2009). In the following section, we will discuss how anatomical connectivity can be estimated non-invasively in humans and highlight the strengths and limitations of the available techniques.
On the other hand, measures of functional connectivity, such as resting-state fMRI (Cohen et al., 2008; Zhang et al., 2008), may also reflect (but not uniquely determine) effective connectivity patterns. The comparison between structural and functional connectivity patterns could therefore shed further light onto the structure–function relationship, in particular, when it comes to test hypotheses based on models of effective connectivity.
Characterization of Structural Connectivity
To date, anatomical connectivity information has been revealed mostly from animal models. These focus on the measurement of degenerating axons subsequent to lesion (Nauta and Gygax, 1951; Blackstad, 1965) or active transport of tracers that are injected while the animal is still alive (cf., e.g., Schmahmann and Pandya, 2006). Although powerful, active axonal transport techniques have seen little or no application in the human brain because they are limited to invasive studies.
In principle, post mortem tracer application also allows tracing of tracts in aldehyde-fixed (Haber, 1988) as well as in unfixed (McConnell et al., 1989) tissue, but only for distances of about 10 mm (Mufson et al., 1990). Longer distance connections can only be investigated by series of histological sections (Axer et al., 2002), blunt dissection, freeze-thaw dissection (Klingler and Gloor, 1960), or indirect evidence from anterograde degeneration due to brain lesions (Di Virgilio and Clarke, 1997). Due to these restrictions, information about long-range connectivity in the normal human has been difficult to obtain and limited in scope (Clarke and Miklossy, 1990; Miklossy et al., 1991; Crick and Jones, 1993). The respective techniques require high levels of effort and skill, cannot be applied to an individual living human subject, and are not suited for obtaining an exhaustive connectivity pattern of the whole brain, or even of a certain region in the brain. Furthermore, tract variability is difficult to assess since only a limited set of connections can be traced per specimen.
With dMRI, a technique has emerged that allows in vivo and non-invasive characterization of long-range axonal connectivity in the brain (Johansen-Berg and Behrens, 2009; Johansen-Berg and Rushworth, 2009; Hagmann et al., 2010). This technique probes the direction-dependent mobility of water molecules by measuring dephasing of spins of protons in the presence of a spatially varying magnetic field (“gradient”) at a certain time after excitation. As microstructural barriers influence the mobility of the molecules, it is possible to infer certain direction-dependent aspects of the microanatomy, such as fiber directions. This allows the reconstruction of white matter fiber pathways, referred to as diffusion tractography (Mori and Van Zijl, 2002), and the estimation of anatomical connectivity. Both sensitivity and specificity of the technique toward aspects of anatomical connectivity estimation crucially depend on signal-to-noise ratio and sampling resolution in space (voxel dimensions), gradient orientation (number of probed diffusion directions), diffusion time, and gradient strength. These latter two parameters usually determine the so-called b-value, which characterizes the sensitivity of the dMRI protocol toward characteristic length scales in microstructural features. All of these parameters are limited on practical grounds, leading to the necessity for strong model assumptions and simplifications. Recently, important progress has been made to overcome these limitations. The use of high angular resolution diffusion imaging (HARDI) in conjunction with advanced local modeling methods (Jansons and Alexander, 2003; Tournier et al., 2004, 2007; Tuch, 2004; Ozarslan et al., 2006; Kaden et al., 2007; Descoteaux et al., 2009) allows the reconstruction of fiber orientation profiles within each voxel and permits the resolution of complex fiber layouts, such as crossings, branching, and twisting.
The important problem of spatial resolution can be tackled, for example, using ultrahigh field strength in conjunction with reduced field of view and parallel imaging. For instance, images with isotropic resolution of 800 μm, sufficient signal-to-noise ratio and high angular resolution have revealed surprising detail on small fiber bundles and, most important for connectivity analysis, the gray–white matter interface (Heidemann et al., 2010). The use of multiple b-values can be utilized to further enhance the angular resolution, as in diffusion spectral imaging (DSI; Wedeen et al., 2005), or to separately estimate compartments of restricted (intra-axonal) and hindered (extra-axonal) diffusion, thereby estimating important parameters, such as axonal density, as in the so-called “composite hindered and restricted model of diffusion” (CHARMED; Assaf and Basser, 2005). If diffusion time and gradient strength, which are usually combined in the b-value, can be varied independently, it is possible to reveal additional important connectivity parameters, such as the axonal caliber distribution (Assaf et al., 2008; Alexander et al., 2010).
In spite of these promising technological advances, an exact quantification of axonal connectivity remains difficult. For a comprehensive and critical review on this issue, refer to Jones (2010). On the other hand, while dMRI may not be sufficiently specific for the quantification of axonal connectivity, it is still very sensitive to differences of this. In other words, even without an exact quantitative estimate of connectivity patterns, we might still be able to represent the similarity structure of the connectivity of the cortex (i.e., to quantify how similar or dissimilar the connectivity patterns of two pieces of cortex are). In this respect it needs to be discussed whether this similarity structure can be used to find functionally meaningful parcellations of the cortex.
Parcellation and Similarity Mapping Based on Connectivity
As argued above, functional and structural feature spaces map onto the cortex obeying the principle of smoothness. In this line, one way to represent the structural (and thereby also the functional) similarity structure of the cortex is by means of parcellation. Parcellation means the subdivision of the cortical surface into compact areas, which are internally relatively homogeneous and distinct from one another, with respect to the considered structural criteria. Cortex parcellation has proven very useful for studying the organizational principles of the brain and its ontogenetic and phylogenetic development (Bystron et al., 2008; Rakic, 2009). In practice, it also plays a prominent role in the structural interpretation of functional imaging results (Eickhoff et al., 2005), but it needs to be stressed that a unique parcellation of the entire cortex does not exist. Instead the parcellation of choice depends on a number of factors: (1) the required level of detail (i.e., the magnitude of difference that is just considered relevant for the particular purpose), (2) the achievable level of detail (i.e., the magnitude of difference that just can be reliably detected by the available technique), and (3) the particular structural criteria the parcellation is based upon. The last point, in particular, has led to considerable diversity in parcellation results of the human cortex. In the past, cortex parcellation has been most often based on local tissue properties. As one of the first, Brodmann (1909) considered cytoarchitecture, which reflects the specific variation in size and packing density of cell bodies across the depth profile of the cortical sheet. Since then this approach has been adapted by a number of researchers to produce cytoarchitectonic maps of specific cortical areas (e.g., Amunts et al., 2007; Zilles and Amunts, 2010). Later researchers have also extended the concept of cortical arealization to other local microstructural parameters, such as layering, distribution, and amount of intracortical myelinated fibers (Vogt, 1910, 1911; Braitenberg, 1962; Zilles, 2004) and the density of certain neurotransmitters receptors (Zilles et al., 2004; Zilles and Amunts, 2009; Amunts et al., 2010). However, functional–anatomical models based on these local tissue properties must remain somewhat limited for the following reasons.
First, differences between the microarchitectures of cortical areas are often quite subtle (Kaas and Catania, 2002). Second, the understanding that higher cognitive functions are preferentially based on widespread networks, rather than isolated cortical areas, leads to the conclusion that the long-range connectional pattern of cortex and sub-cortical structures must be an indispensible structural parameter in terms of functional relevance. In this line, we concluded above that structural connectivity (in particular, axonal connectivity) is a strong constraint for effective connectivity forming functional networks. Therefore, the anatomical characterization of brain areas just by their internal microstructure, neglecting their connections to other brain areas must remain incomplete. Consequently, the identification of functionally relevant units should consider axonal connectivity as an important criterion.
Although parcellations based on local cytoarchitecture and long-range connectivity have been shown to bear some congruence in a number of publications (e.g., Jones and Burton, 1976; Eickhoff et al., 2010), none of these studies demonstrated a one-to-one relationship. Instead of the notion that long-range (extrinsic) connectivity is simply a more easy-to-access indicator for cytoarchitectonic areas (see, e.g., Behrens and Johansen-Berg, 2005), we propose that both structural traits are, at least to some degree, complementary. Only the coexistence of a multitude of complimentary structural maps provides the structural diversity necessary to support the functional diversity needed for the brain to operate robustly.
Fundamental to the concept of connectivity-based parcellation is the idea that individual brain regions maintain individual connection profiles, which are relatively invariant within and distinct between areas. Current approaches, and in particular those relying on diffusion tractography, are based on the following principles (see Figure 3):
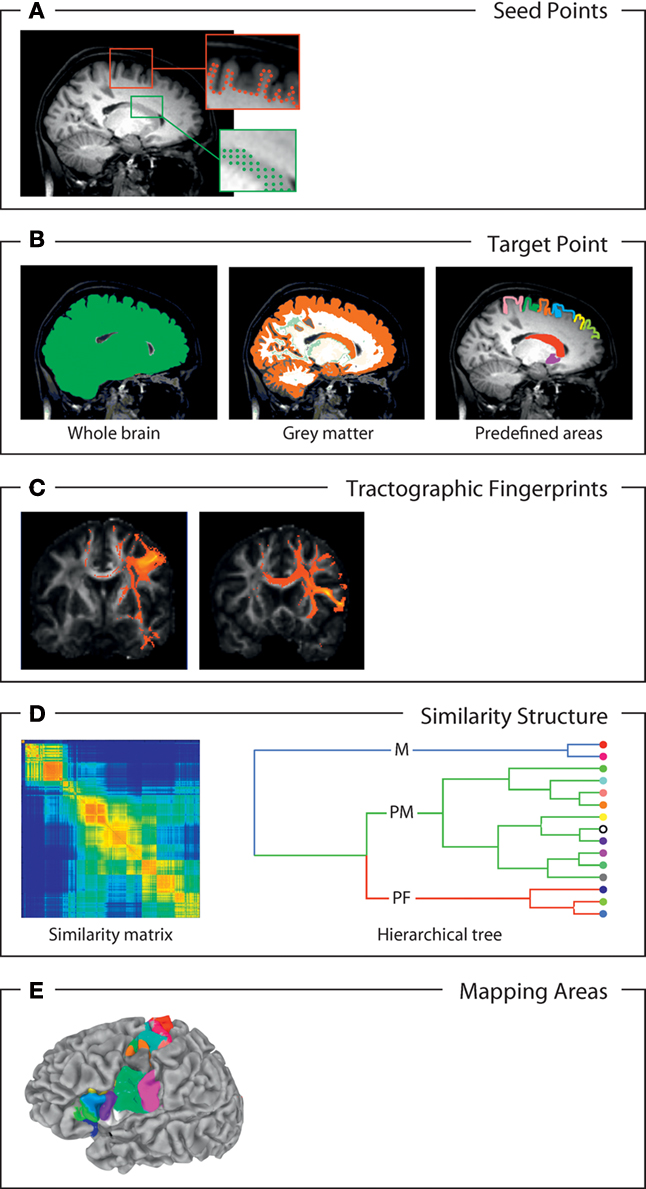
Figure 3. Schematic overview of the main operations involved in connectivity-based gray matter parcellation. (A) Definition of seed points. The seed points discretize the region of interest to be parcellated. This may, for example, be the cortical mantle or a section thereof, represented by the points at the gray–white matter interface (red dots, e.g., Anwander et al., 2007), or a sub-cortical structure like the caudate. As such sub-cortical structures are penetrated by fibers much more than the cortex, the use of all voxels as seed points is warranted (green dots, e.g., Behrens et al., 2003). (B) Definition of the target space. The target space determines to which voxels or regions the connectivity from the seed voxels will be computed. For example, one may simply use the entire brain (top, e.g., Johansen-Berg et al., 2004), or just a certain part of the brain (e.g., Bach et al., 2011), such as the cortex or all gray matter (middle) or a collection of predefined regions (e.g., Behrens et al., 2003b), e.g., based on macroscopic landmarks (bottom). (C) Connectional fingerprints: the fingerprints comprise the connectivity of each seed voxel with all target voxels or regions. They can be estimated in various ways. Here, as an example, probabilistic tractograms connecting the seed voxel with all other voxels of the brain are depicted. (D) Similarity structure: similarity between different connectional fingerprints can be measured in different ways, for example, as correlation, as Euclidean distance (after normalization) or as mutual information. It can be represented as similarity matrix (top) or hierarchical cluster tree (bottom). (E) Parcellation mapped on the brain: clustering or partitioning algorithms extract sensible parcellations from the similarity structure, which can then be mapped on the brain. Here, we show a cortical parcellation computed with an agglomerative clustering method (Gorbach et al., 2010).
(a) Within the resolution provided by the available imaging data, a connectional fingerprint characterizes each point on the region to be parcelled. This fingerprint may be sensitive to the connectivity to the rest of the brain (e.g., Johansen-Berg et al., 2004; Anwander et al., 2007; Tomassini et al., 2007; Schubotz et al., 2010; Mars et al., 2011), or only to a preselected collection of target areas (Behrens et al., 2003b; Johansen-Berg et al., 2005). It is important to stress that the fingerprint does not need to accurately reflect structural connectivity in a quantitative sense – it only needs to be sensitive to connectivity differences.
(b) Connectional fingerprints are mutually compared, either exhaustively or only between neighboring cortical points. The comparison can be done using various similarity measures, which need to be chosen in a problem-specific manner. This way, the similarity structure of the cortical connectivity is established. It can be expressed, for instance, in terms of a similarity matrix or as a hierarchical cluster tree.
(c) From the similarity structure, a parcellation is derived; clustering or grouping can achieve this. The parcellation does not reflect the similarity structure in its entirety any more, but embodies an information reduction based on certain prior assumptions. The clustered similarity matrix, for instance, expresses similarity of connectional fingerprints within each cluster, whereas the hierarchical tree reflects cluster similarity.
In the following, we will discuss these operations, the respective possibilities for their implementation, the implicit assumptions associated with these choices and the consequences thereof.
Computation of Connectional Fingerprints in vivo
Connectional fingerprints approximate the connectivity profile of small areas (seed areas; usually single MRI voxels) with other parts of the brain. As dMRI is the only method currently available that allows non-invasive, exhaustive, and in vivo characterization of structural connectivity of the human cortex, we will restrict ourselves here to approaches using diffusion tractography.
A convenient way to characterize anatomical connectivity of one seed point to the entire brain is the computation of a probabilistic tractogram. Here, streamline tractography or random particle walks are repeated many times with different samples drawn from the local fiber orientation distributions, and each voxel in the brain is labeled according to the number of times it has been reached by a fiber pathway (Koch et al., 2002; Behrens et al., 2003a; Anwander et al., 2007). The result is a vector with one number per brain voxel, which can be interpreted (with some reservations, see Jones, 2010) as probability of connection between this voxel and the seed voxel. These tractograms can be used as is (e.g., Anwander et al., 2007) or binarized with a certain threshold (e.g., Johansen-Berg et al., 2004; Devlin et al., 2006). The use of such high-dimensional connectivity signatures allows a very fine-grained characterization of the cortical similarity structure, possible beyond the real information content of the data. A reduction in size can be reached by only considering target voxels in certain parts of the brain, such as the cortex, or by defining way points, where the fiber pathways of interest do not pass (Bach et al., 2011).
Alternatively, one may use probabilistic or deterministic tractography to quantify the connectivity strength between the voxels of the seed region and a limited number of predefined target regions (Behrens et al., 2003b; Traynor et al., 2010). Such connectional fingerprints are more low dimensional and incorporate additional knowledge by focusing only on connections considered relevant.
Naturally, accuracy and precision of connectional fingerprints based on tractography depend crucially on the quality and the properties of the underlying data, as well as the techniques employed for local (voxel-wise) modeling and tract tracing (see previous section). To date, the majority of the parcellation studies have used local models assuming one principal fiber direction in each voxel, such as the (sharpened) diffusion tensor (Anwander et al., 2007; Schubotz et al., 2010) or the single direction ball-and-stick model (Behrens et al., 2003b; Johansen-Berg et al., 2004, 2005; Devlin et al., 2006). These have been fitted to high angular resolution diffusion data measured at low b-values (around 1000 s/mm2), with voxel sizes between 1.5 and 2.5 mm. Recently, more sophisticated local models accounting for more complicated fiber layouts have been introduced into tractography-based parcellation. For example, Bach et al. (2011) and O’Muircheartaigh et al. (2011) used a compartment model (ball-and-stick) with two possible fiber directions per voxel (Behrens et al., 2007) to parcel the human amygdala and thalamus, respectively. Likewise, another compartment model implementing multiple tensors within the framework of the probabilistic index of connectivity (PICo; Parker et al., 2003; Parker and Alexander, 2005) was used to segment the human basal ganglia and thalamus (Draganski et al., 2008).
Further improvements can be expected from advances in MRI technology and associated local modeling techniques. One important parameter is the spatial resolution. The current voxel size of more than 1.5 mm causes finer details to be averaged out and prevents, for example, exact detection of small fiber paths connecting the large white matter bundles with their cortical and sub-cortical targets. Preliminary studies have shown that the use of ultrahigh field MRI (7 T) in conjunction with zoomed and parallel imaging can reduce the resolution to below 1 mm and thereby reveal fine details of the gray–white matter interface (Heidemann et al., 2010). Another direction of improvement concerns the fact that axonal connectivity, being only part of structural connectivity in the first place, is by itself a multiparametric entity. A more complete characterization would, for example, include information on the density and the diameter of axons. Such additional information may be extracted by techniques that vary the sensitivity of the measurement to particular diffusion length (by gradient strength) and the time the particles are given to diffuse, thereby probing the tissue microstructure in more detail and at different spatial scales (Assaf and Basser, 2005; Assaf et al., 2008; Alexander et al., 2010).
Quantification of the Connectional Similarity Structure
An obvious next step toward a connectivity-based parcellation of cortical areas is the definition of the similarity structure of the cortical regions of interest in terms of their global connectivity. In this way, we obtain criteria according to which seed voxels can be classified as belonging to the same or different areas. As a result, one can derive meaningful subdivisions of the regions of interest and extract connectional signatures of the resulting subareas (e.g., as average or prototypical connectional fingerprints).
Target-based parcellation
If the connectional fingerprints are low dimensional and reflect the connectivity between the seed voxels and a number of predefined target areas, the simplest method is to categorize the seed voxels according to the most probable target region. This method is often applied to sub-cortical structures, where clear hypotheses exist for the target areas of the potential subnuclei. For example, Behrens et al. (2003b) applied this technique to segment the thalamus according to its connectivity to large-scale cortical regions. Their results appear to be very similar to previous histological and tracer findings in humans and non-human primates, not only in terms of the arrangement of the subregions, but also in terms of the pathways represented by their connectional signatures. The findings were later validated by a comparison with fMRI measurements (Johansen-Berg et al., 2005). Powerful as it is, this method has the disadvantage that it depends on strong assumptions. The underlying model assumes regions in the seed area, where each region is required to connect preferentially with one target area. Moreover, the target areas may be difficult to delineate, in particular in the cortex, where the boundaries of cytoarchitectonic areas only limitedly correspond to macroscopic landmarks (Amunts et al., 1999).
Supervised clustering
A more data-driven approach compares all connectional fingerprints, which can be based on predefined target regions or simply the voxels of the brain or a subregion thereof (e.g., cortex). Here, it is no longer assumed that the connectivity of the resulting parcels is uniform to one target region. To date, two different types of supervised clustering algorithms have been used to perform cortex parcellation; spectral reordering (Johansen-Berg et al., 2004) and k-means clustering (Anwander et al., 2007; Klein et al., 2007). Both of these approaches employ correlation as a predefined similarity measure and thus explicitly rely on the strength of linear dependency between tractograms in order to form clusters, but in general any mathematically suitable similarity measure could be applied for supervised clustering.
Johansen-Berg et al. (2004) quantified the similarity between the connectional fingerprints of the voxels of medial frontal cortex using correlation, applied spectral reordering to the resulting similarity matrix, and then identified homogeneous clusters by inspection. They found two areas with distinct connectivity profiles preferentially projecting to motor and prefrontal areas, respectively, which could be identified with SMA proper and preSMA. These results were further substantiated by a very close agreement between this connectivity-based parcellation and functional parcellation using fMRI localizer paradigms. A similar approach was also used to separate the lateral and medial geniculate nuclei, which bear very distinct connections to the visual and auditory cortices, respectively (Devlin et al., 2006).
Anwander et al. (2007) clustered a similarity matrix with a k-means approach to subdivide the posterior inferior frontal cortex (Broca’s area). Although this method is less subjective than mere visual inspection, it still requires the user to select the number of clusters. Here, the authors opted for three clusters, which was the largest number that still yielded topologically consistent results in all subjects. By comparison with the cytoarchitectonic maps published by Amunts et al. (1999), the three regions were argued to coincide with Brodmann areas 44 and 45 as well as with the deep frontal operculum. A k-means approach was also used by other researchers (Klein et al., 2007; Tomassini et al., 2007), and was investigated in detail with respect to its reproducibility by Nanetti et al. (2009).
It is important to stress that using a k-means approach for clustering in these studies, a partitioning is forced (i.e., gradations or soft transitions between areas are not accounted for).
Unsupervised, hierarchical clustering
A major limitation of the above-mentioned clustering studies is that they assume that the number of clusters is known. However, in practice, the a priori determination of the number of clusters may not be possible. This problem motivates so-called unsupervised clustering.
Another limitation of the above-mentioned clustering techniques is that they force a parcellation of the region of interest that is unique and complete (i.e., each voxel belongs to exactly one subarea). This might not entirely reflect anatomical reality. The existence of a transition in cortical architecture, no matter how robust or consistent, does not necessarily signify a boundary between distinct cortical “areas”. An architectonic transition may instead reflect gradients or trends across the full extent of a given area. In fact, it is well known that such directional changes of cytoarchitectonic, receptorarchitectonic, or myeloarchitectonic properties of adjacent cortical fields can occur (Sanides, 1962; Lewis and Van Essen, 2000). A broad transition region may reflect biologically genuine gradations, such that neurons within the transition region have anatomical and/or physiological characteristics intermediate between the neighboring subdivisions. Hence, an important issue with parcellation is to assess the spatial extent over which such architectonic transitions occur.
Moreover, especially with respect to connectivity, brain networks are more appropriately conceived of as forming nested modules (Bassett and Gazzaniga, 2011), each with a characteristic connectivity – i.e., modular hierarchies (Kaiser and Hilgetag, 2007, 2010). Therefore, further properties of cortical subunits such as their possible nested hierarchical structure and transitional borders need to be investigated. The notion of a hierarchically modular organization of cortical subunits (Meunier et al., 2010) stems from the idea that cortical subunits themselves are nested into further modular structures at higher topological scales due to their similarity to one another with respect to anatomical connectivity. The question is therefore at which topological scales such nested modular structures exist.
With respect to the requirement specification of clustering methods, this means that cluster boundaries may be fuzzy and several parcellations at different hierarchical levels might coexist. It might be that areas with homogeneous and mutually distinct properties can sensibly describe only portions of the region of interest. In other words, the connectivity similarity arrangement of the cortex might be structured such that it is only insufficiently reflected by a single unique and complete parcellation.
A first step toward a more realistic, unsupervised clustering approach was realized by Jbabdi et al. (2009) with the application of a Dirichlet process mixture model. While this study still focuses on partitioning (i.e., no soft transitions between areas) of cortical areas and there is no underlying hierarchically modular organization, the technique embodies a mixture of Gaussian distributions as a hierarchical model to describe the data. An obvious next step would be to drop the requirements of uniqueness and completeness, and allow both fuzzy boundaries between areas and a modular hierarchy. Therefore, we have recently suggested a hierarchical information-based clustering method (Gorbach et al., 2010) that was face validated using the example of the posterior inferior prefrontal cortex and also applied to the anterior lateral prefrontal cortex (Sujazow et al., 2010). With this approach, the similarity matrix was constructed using mutual information. An agglomerative hierarchical reformulation of information-based clustering (Slonim et al., 2005) was initialized by adaptive affinity propagation (Frey and Dueck, 2007; Leone et al., 2008) and cluster validity was assessed as the minimum of a variational Bayes problem. Note that this technique explicitly allows fuzzy boundaries.
An attempt to represent the similarity structure of the entire cortical mantle was presented by Moreno et al. (2011), who used agglomerative hierarchical clustering based on the correlations between probabilistic tractograms, with and without the additional assumption of topographical compactness of the areas.
Since hierarchical cluster trees can accommodate the complete similarity structure of cortical connectivity, these methods might represent a promising route toward more meaningful structural characterization of the brain (cf., also Averbeck and Seo, 2008; Averbeck et al., 2009). In particular, these methods allow the representation of entire series of interrelated parcellations at different levels of detail and thereby, at least partially, eliminate the need to choose one desired level of detail (e.g., by selecting the number of clusters).
Population-based clustering
Individual variability is an important issue in anatomical studies because any given area (even a primary sensory area) can vary in size by twofold or more (Filiminoff, 1932; Maunsell and Van Essen, 1987; Uylings et al., 2005; Van Essen and Dierker, 2007) and because the consistency with which each area is located with respect to topographic boundaries has important implications for physiological and neuroimaging studies. In this respect, a meaningful parcellation should be assumed to exist in all subjects with similar location, shape and connectivity pattern. Multi-subject approaches are therefore expected to stabilize parcellation results. The simplest approach to multi-subject analysis is to use post hoc statistics in order to filter out the results with a high level of consistency between subjects (Nanetti et al., 2009). However, in order to fully exploit the potential of inter-subject consistency constraint, it would be necessary to directly implement this assumption into the clustering procedure. An interesting approach was proposed by O’Muircheartaigh et al. (2011), who used independent components analysis (ICA) to identify prototypical tractograms and the associated seed areas (independent components) in the thalamus of multiple brains. The method assumes consistency of both tracts and seed areas across subjects. The pattern of the contributions of each seed voxel to a particular component can then be used to define clusters in the seed region. These clusters are potentially overlapping, which might be interesting in the light of partial volume effects underlying the diffusion imaging data (one voxel might belong to more than one area). Note that there are still some subjective choices to be made, in particular regarding the number of components to be considered.
An entirely more difficult problem arises if it is not inter-subject consistency that should be analyzed, but inter-subject variability. Variable aspects of cortical organization, such as the size and connections of a cortical area, can generate differences in behavior (cf., Christoff et al., 2003; Kanai and Rees, 2011). Thus, to understand such phenotypic variation it is necessary to identify types of variation that exists in different cortical areas of individuals within a population. A prominent example of inter-subject variability is the prefrontal cortex, where some areas not only vary tremendously in spatial extent as well as in topographic location, but where the general arrangement of areas might be quite different (e.g., Rajkowska and Goldman-Rakic, 1995). For instance, in some subjects, there may be a clear anatomical distinction between areas 46 and 9, whereas in others, some additional areas, such as 9/46d and 9/46v, seem to exist (Petrides, 2005). One might even argue here for phenotypic diversity and relate this to evolutionary aspects in cortical area formation (Krubitzer and Kaas, 2005; Karlen and Krubitzer, 2006; Schoenemann, 2006).
From a methodological point of view, the statistical assessment of area variability requires a population-based clustering approach, which even needs to cope with (at least some degree of) topological variability among clusters. This is mathematically demanding, and such methods might be subject of future research.
Conclusion and Perspective
We have argued for long-range axonal connectivity being an important indicator of the functional–anatomical organization of the cortex. Thereby we discussed that this criterion might be partly reflective of and partly complementary to other indicators of structural organization, such as intrinsic connectivity (local neural circuits), cyto- and myelo-architecture, and receptorarchitecture. Moreover, with respect to mapping of functional and structural feature spaces onto the cortical sheet, the functional–anatomical organization of the cortex (somewhat in contrast to sub-cortical gray matter areas) is inter-individually quite variable and correlated with behavioral variables. This correlation is potentially very informative of the general relationship between structure and function in the brain and therefore of major scientific interest. In this light, recently evolved techniques for non-invasive estimation of axonal connectivity from dMRI deserve special interest in spite of the fact that they also have some limitations. Based on this motivation, we focused on techniques that apply diffusion tractography in order to estimate long-range connectivity and use this information to reveal the functional–anatomical organization of these areas.
So far, the structural organization of the cortex has mostly been described in terms of a parcellation, that is, the subdivision into a number of areas, which are, according to the criteria at hand, relatively homogeneous and mutually distinct. This tradition goes back to the seminal work of Korbinian Brodmann and has been adhered to ever since by many researchers who produced maps based on various local structural criteria. However, while some of these areas are indeed very homogeneous and separated from neighboring areas by sharp and undisputed boundaries, this seems to not be true in other cases, leading to great variability across different maps. Likewise, the homogeneity of areas is always relative and subareas are often postulated later using finer criteria. In general, the definition “relatively homogenous and mutually distinct” raises the question of what is “relatively homogeneous.” The answer to this question has a subjective and a methodological component – it depends on what magnitude of difference is just considered high enough in the particular context to warrant the definition of an area boundary, and on what magnitude of difference the methodology used is just able to reliably detect.
Therefore, we argue that more universal representations of the connectional similarity structure of gray matter areas are needed in order to achieve convergence between different methods and to successfully characterize the structure–function relationship. This can be realized by series of interrelated parcellations at different levels of detail based on hierarchical cluster trees, for example. Another road to more realistic subdivisions may be built on fuzzy clustering approaches, allowing the representation of distinct areas with architectonic transitions between them. Of course the quality of the connectivity estimate itself has a decisive influence on the validity of connectivity-based models of functional–anatomical organization. New developments in MR technology allow higher sampling in voxel space and diffusion orientation, as well as for using multiple diffusion lengths and times. Together with improved analysis methods, this will lead to more reliable reconstructions of finer details of the fiber pathways and provide access to additional parameters of axonal connectivity, such as axonal density and diameter.
So far, due to technical limitations, most studies on connectivity-based parcellation have focused on a comparatively small, circumscribed region of the cortex or on a particular sub-cortical structure. Especially in the case of the cortex, the definition of such regions is rather difficult and has the potential to bias for the parcellation result. As the computational capacities increase, the simultaneous analysis of the whole cortex, for example, will become feasible. Moreover, this development will also enable the statistical interpretation of multiple-subject approaches, which may, at least in a number of cases, make parcellations more reliable and representative, or yield much better information about variability in a population.
To summarize, the use of non-invasively acquired connectivity estimates to characterize the functional–anatomical organization of the brain is a valid, relevant, and necessary endeavor. Current and future developments in MR technology, tractography algorithms and models of the similarity structure hold great potential for a substantial improvement and enrichment of the results of the technique.
Finally, much work needs to be done in the fields of validation and comparison with other techniques, including functional connectivity measures. Also, clinical implications need to be carefully addressed. As many neurological and psychiatric disorders are likely to be associated with altered anatomical connectivity (Mesulam, 2005), the study of connectivity-based parcellation may yield further understanding of the disease process: most probably with respect to degeneration or reorganization, disconnection or dislocation, or congenital manifestations of malformation. However, to date, these links are yet to be established.
Conflict of Interest Statement
The authors declare that the research was conducted in the absence of any commercial or financial relationships that could be construed as a potential conflict of interest.
Acknowledgments
The authors wish to thank D. Y. von Cramon for his endurance in numerous discussions about brain anatomy as well as for his remarks on a previous version of the manuscript. The work of Marc Tittgemeyer is supported by the German Ministry of Education and Research (grant number 01GW0612, 01GW0772). The work of Thomas R. Knösche was supported in part by the “CONNECT” project financed by the Future and Emerging Technologies (FET) program within the Seventh Framework Program for Research of the European Commission, under FET-Open grant number: 238292.
References
Achard, S., and Bullmore, E. (2007). Efficiency and cost of economical brain functional networks. PLoS Comput. Biol. 3, e17. doi: 10.1371/journal.pcbi.0030017
Aertsen, A., and Preißl, H. (1999). Dynamics of Activity and Connectivity in Physiological Neuronal Networks. New York:VCH.
Alexander, D. C., Hubbard, P. L., Hall, M. G., Moore, E. A., Ptito, M., Parker, G. J. M., and Dyrby, T. B. (2010). Orientationally invariant indices of axon diameter and density from diffusion MRI. Neuroimage 52, 1374–1389.
Amunts, K., Lenzen, M., Friederici, A. D., Schleicher, A., Morosan, P., Palomero-Gallagher, N., and Zilles, K. (2010). Broca’s region: novel organizational principles and multiple receptor mapping. PLoS Biol. 8, e1000489. doi: 10.1371/journal.pbio.1000489
Amunts, K., Schleicher, A., Burgel, U., Mohlberg, H., Uylings, H., and Zilles, K. (1999). Broca’s region revisited: cytoarchitecture and intersubject variability. J. Comp. Neurol. 412, 319–341.
Amunts, K., Schleicher, A., and Zilles, K. (2007). Cytoarchitecture of the cerebral cortex – more than localization. Neuroimage 37, 1061–1065; discussion 1066–1068.
Anwander, A., Tittgemeyer, M., Von Cramon, D. Y., Friederici, A. D., and Knösche, T. R. (2007). Connectivity-based parcellation of Broca’s area. Cereb. Cortex 17, 816–825.
Assaf, Y., and Basser, P. (2005). Composite hindered and restricted model of diffusion (charmed) MR imaging of the human brain. Neuroimage 27, 48–58.
Assaf, Y., Blumenfeld-Katzir, T., Yovel, Y., and Basser, P. J. (2008). AxCaliber: a method for measuring axon diameter distribution from diffusion MRI. Magn. Reson. Med. 59, 1347–1354.
Averbeck, B. B., Battaglia-Mayer, A., Guglielmo, C., and Caminiti, R. (2009). Statistical analysis of parieto-frontal cognitive-motor networks. J. Neurophysiol. 102, 1911–1920.
Averbeck, B. B., and Seo, M. (2008). The statistical neuroanatomy of frontal networks in the macaque. PLoS Comput. Biol. 4, e1000050. doi: 10.1371/journal.pcbi.1000050
Axer, H., Leunert, M., Mürköster, M., Grässel, D., Larsen, L., Griffin, L. D., and Graf VKeyserlingk, D. (2002). A 3D fiber model of the human brainstem. Comput. Med. Imaging Graph. 26, 439–444.
Bach, D., Behren, S. T., Garrido, L., Weiskopf, N., and Dolan, R. (2011). Deep and superficial amygdala nuclei projections revealed in vivo by probabilistic tractography. J. Neurosci. 31, 618–623.
Barbas, H., and Rempel-Clower, N. (1997). Cortical structure predicts the pattern of corticocortical connections. Cereb. Cortex 7, 635–646.
Barone, P., Batardiere, A., Knoblauch, K., and Kennedy, H. (2000). Laminar distribution of neurons in extrastriate areas projecting to visual areas V1 and V4 correlates with the hierarchical rank and indicates the operation of a distance rule. J. Neurosci. 20, 3263–3281.
Bassett, D. S., and Gazzaniga, M. S. (2011). Understanding complexity in the human brain. Trends Cogn. Sci. (Regul. Ed.) 15, 200–209.
Behrens, T. E., Woolrich, M. W., Jenkinson, M., Johansen-Berg, H., Nunes, R. G., Clare, S., Matthews, P. M., Brady, J. M., and Smith, S. M. (2003a). Characterization and propagation of uncertainty in diffusion-weighted MR imaging. Magn. Reson. Med. 50, 1077–1088.
Behrens, T. E. J., Johansen-Berg, H. J., Woolrich, M. W., Smith, S. M., Wheeler-Kingshott, C. A. M., Boulby, P. A., Barker, G. J., Sillery, E. L., Sheehan, K., Ciccarelli, O., Thompson, A. J., Brady, M., and Matthews, P. M. (2003b). Non-invasive mapping of connections between human thalamus and cortex using diffusion imaging. Nat. Neurosci. 6, 750–757.
Behrens, T. E. J., Berg, H. J., Jbabdi, S., Rushworth, M. F. S., and Woolrich, M. W. (2007). Probabilistic diffusion tractography with multiple fibre orientations: what can we gain? Neuroimage 34, 144–155.
Behrens, T. E. J., and Johansen-Berg, H. (2005). Relating connectional architecture to grey matter function using diffusion imaging. Philos. Trans. R. Soc. Lond. B Biol. Sci. 360, 903–911.
Blackstad, T. W. (1965). Mapping of experimental axon degeneration by electron microscopy of Golgi preparations. Z. Zellforsch. Mikrosk. Anat. 67, 819–834.
Bressler, S. L. (2002). Understanding cognition through large-scale cortical networks. Curr. Dir. Psychol. Sci. 11, 58–61.
Bressler, S. L., and Menon, V. (2010). Large-scale brain networks in cognition: emerging methods and principles. Trends Cogn. Sci. (Regul. Ed.) 14, 277–290.
Brodmann, K. (1909). Vergleichende Lokalisationslehre der Großhirnrinde in ihren Prinzipien dargestellt auf Grund des Zellaufbaues. Leipzig: Barth.
Büchel, C., Coull, J. T., and Friston, K. J. (1999). The predictive value of changes in effective connectivity for human learning. Science 283, 1538–1541.
Bullmore, E., and Sporns, O. (2009). Complex brain networks: graph theoretical analysis of structural and functional systems. Nat. Rev. Neurosci. 10, 186–198.
Buxhoeveden, D. P., and Casanova, M. F. (2002). The minicolumn hypothesis in neuroscience. Brain 125, 935–951.
Bystron, I., Blakemore, C., and Rakic, P. (2008). Development of the human cerebral cortex: Boulder Committee revisited. Nat. Rev. Neurosci. 9, 110–122.
Chklovskii, D. B., and Koulakov, A. A. (2004). Maps in the brain: what can we learn from them? Annu.Rev. Neurosci. 27, 369–392.
Christoff, K., Ream, J. M., Geddes, L. P. T., and Gabrieli, J. D. E. (2003). Evaluating self-generated information: anterior prefrontal contributions to human cognition. Behav. Neurosci. 117, 1161–1168.
Clarke, S., and Miklossy, J. (1990). Occipital cortex in man: organization of callosal connections, related myelo- and cytoarchitecture, and putative boundaries of functional visual areas. J. Comp. Neurol. 298, 188–214.
Cohen, A. L., Fair, D. A., Dosenbach, N. U. F., Miezin, F. M., Dierker, D., Van Essen, D. C., Schlaggar, B. L., and Petersen, S. E. (2008). Defining functional areas in individual human brains using resting functional connectivity MRI. Neuroimage 41, 45–57.
David, O., Garnero, L., Cosmelli, D., and Varela, F. J. (2002). Estimation of neural dynamics from MEG/EEG cortical current density maps: application to the reconstruction of large-scale cortical synchrony. IEEE Trans. Biomed. Eng. 49, 975–987.
Deco, G., Jirsa, V., McIntosh, A. R., Sporns, O., and Kötter, R. (2009). Key role of coupling, delay, and noise in resting brain fluctuations. Proc. Natl. Acad. Sci. U.S.A. 106, 10302–10307.
Deco, G., Jirsa, V. K., and McIntosh, A. R. (2011). Emerging concepts for the dynamical organization of resting-state activity in the brain. Nat. Rev. Neurosci. 12, 43–56.
Descoteaux, M., Deriche, R., Knösche, T. R., and Anwander, A. (2009). Deterministic and probabilistic tractography based on complex fibre orientation distributions. IEEE Trans. Med. Imaging 28, 269–286.
Devlin, J. T., Sillery, E. L., Hall, D. A., Hobden, P., Behrens, T. E. J., Nunes, R. G., Clare, S., Matthews, P. M., Moore, D. R., and Johansen-Berg, H. (2006). Reliable identification of the auditory thalamus using multi-modal structural analyses. Neuroimage 30, 1112–1120.
Di Virgilio, G., and Clarke, S. (1997). Direct interhemispheric visual input to human speech areas. Hum. Brain Mapp. 5, 347–354.
Draganski, B., Kherif, F., Kloppel, S., Cook, P. A., Alexander, D. C., Parker, G. J. M., Deichmann, R., Ashburner, J., and Frackowiak, R. S. J. (2008). Evidence for segregated and integrative connectivity patterns in the human basal ganglia. J. Neurosci. 28, 7143–7152.
Durbin, R., and Mitchison, G. (1990). A dimension reduction framework for understanding cortical maps. Nature 343, 644–647.
Eickhoff, S. B., Jbabdi, S., Caspers, S., Laird, A. R., Fox, P. T., Zilles, K., and Behrens, T. E. J. (2010). Anatomical and functional connectivity of cytoarchitectonic areas within the human parietal operculum. J. Neurosci. 30, 6409–6421.
Eickhoff, S. B., Stephan, K. E., Mohlberg, H., Grefkes, C., Fink, G. R., Amunts, K., and Zilles, K. (2005). A new SPM toolbox for combining probabilistic cytoarchitectonic maps and functional imaging data. Neuroimage 25, 1325–1335.
Felleman, D. J., and Van Essen, D. C. (1991). Distributed hierarchical processing in the primate cerebral cortex. Cereb. Cortex. 1, 1–47.
Filiminoff, I. (1932). über die Variabilitat der Grosshirnrindenstruktur. Mitteilung II. Regio occipitalis beim erwachsenen Menschen. J. Psychol. Neurol. 44, 1–96.
Fingelkurts, A. A., and Kahkonen, S. (2005). Functional connectivity in the brain – is it an elusive concept? Neurosci.Biobehav. Rev. 28, 827–836.
Frey, B. J., and Dueck, D. (2007). Clustering by passing messages between data points. Science 315, 972–976.
Friston, K. J. (1994). Functional and effective connectivity in neuroimaging: a synthesis. Hum. Brain Mapp. 2, 56–78.
Friston, K. J., Frith, C. D., Liddle, P. F., and Frackowiak, R. S. (1993). Functional connectivity: the principal-component analysis of large (PET) data sets. J. Cereb. Blood Flow Metab. 13, 5–14.
Friston, K. J., Harrison, L., and Penny, W. D. (2003). Dynamic causal modelling. Neuroimage 19, 1273–1302.
Ghosh, A., Rho, Y., Mcintosh, A. R., Kötter, R., and Jirsa, V. K. (2008). Noise during rest enables the exploration of the brain’s dynamic repertoire. PLoS Comput. Biol. 4, e1000196. doi: 10.1371/journal.pcbi.1000196
Gierer, A., and Müller, C. M. (1995). Development of layers, maps and modules. Curr. Opin. Neurobiol. 5, 91–97.
Goodhill, G. J. (2007). Contributions of theoretical modeling to the understanding of neural map development. Neuron 56, 301–311.
Gorbach, N., Schütte, C., Melzer, C., Amunts, K., Douglas, T., and Tittgemeyer, M. (2010). “Hierarchical clustering for connectivity-based cortex parcellation,” in 16th Annual Meeting of the Organisation for Human Brain Mapping,Barcelona, 1371, MT–AM.
Graziano, M. (2006). The organization of behavioral repertoire in motor cortex. Annu. Rev. Neurosci. 29, 105–134.
Graziano, M. S. A., and Aflalo, T. N. (2007a). Mapping behavioral repertoire onto the cortex. Neuron 56, 239–251.
Graziano, M. S. A., and Aflalo, T. N. (2007b). Rethinking cortical organization: moving away from discrete areas arranged in hierarchies. Neuroscientist 13, 138–147.
Haber, S. (1988). Tracing intrinsic fiber connections in postmortem human brain with WGA-HRP. J. Neurosci. 23, 15–22.
Hagmann, P., Cammoun, L., Gigandet, X., Gerhard, S., Ellen Grant, P., Wedeen, V., Meuli, R., Thiran, J.-P., Honey, C. J., and Sporns, O. (2010). MR connectomics: principles and challenges. J. Neurosci. 194, 34–45.
Heidemann, R. M., Porter, D. A., Anwander, A., Feiweier, T., Heberlein, K., Knösche, T. R., and Turner, R. (2010). Diffusion imaging in humans at 7T using readout-segmented EPI and GRAPPA. Magn. Reson. Med. 64, 9–14.
Hilgetag, C. C., Burns, G. A., O’Neill, M. A., Scannell, J. W., and Young, M. P. (2000). Anatomical connectivity defines the organization of clusters of cortical areas in the macaque monkey and the cat. Philos. Trans. R. Soc. Lond. B Biol. Sci. 355, 91–110.
Honey, C. J., Kötter, R., Breakspear, M., and Sporns, O. (2007). Network structure of cerebral cortex shapes functional connectivity on multiple time scales. Proc. Natl. Acad. Sci. U.S.A. 104, 10240–10245.
Jansons, K. M., and Alexander, D. C. (2003). Persistent angular structure: new insights from diffusion MRI data. Dummy version. Inf. Process. Med. Imaging 18, 672–683.
Jbabdi, S., Woolrich, M. W., and Behrens, T. E. J. (2009). Multiple-subjects connectivity-based parcellation using hierarchical Dirichlet process mixture models. Neuroimage 44, 373–384.
Johansen-Berg, H., Behrens, T., Sillery, E., Ciccarelli, O., Thompson, A., Smith, S., and Matthews, P. (2005). Functional-anatomical validation and individual variation of diffusion tractography-based segmentation of the human thalamus. Cereb. Cortex 15, 31–39.
Johansen-Berg, H., Behrens, T. E., Robson, M. D., Drobnjak, I., Rushworth, M. F., Brady, J. M., Smith, S. M., Higham, D. J., and Matthews, P. M. (2004). Changes in connectivity profiles define functionally distinct regions in human medial frontal cortex. Proc. Natl. Acad. Sci. U.S.A. 101, 13335–13340.
Johansen-Berg, H. J., and Rushworth, M. (2009). Using diffusion imaging to study human connectional anatomy. Annu. Rev. Neurosci. 32, 75–94.
Jones, D. (2010). Challenges and limitations of quantifying brain connectivity in vivo with diffusion MRI. Imaging Med. 2, 341–355.
Jones, E. G., and Burton, H. (1976). Areal differences in laminar distribution of thalamic afferents in cortical fields of insular, parietal and temporal regions of primates. J. Comp. Neurol. 168, 197–247.
Kaas, J. H., and Catania, K. C. (2002). How do features of sensory representations develop? Bioessays 24, 334–343.
Kaas, J. H., and Hackett, T. A. (2000). Subdivisions of auditory cortex and processing streams in primates. Proc. Natl. Acad. Sci. U.S.A. 97, 11793–11799.
Kaden, E., Knösche, T. R., and Anwander, A. (2007). Parametric spherical deconvolution: inferring anatomical connectivity using diffusion MR imaging. Neuroimage 37, 474–488.
Kaiser, M., and Hilgetag, C. C. (2006). Nonoptimal component placement, but short processing paths, due to long-distance projections in neural systems. PLoS Comput. Biol. 2, e95. doi: 10.1371/journal.pcbi.0020095
Kaiser, M., and Hilgetag, C. C. (2007). Development of multi-cluster cortical networks by time windows for spatial growth. Neurocomputing 70, 1829–1832.
Kaiser, M., and Hilgetag, C. C. (2010). Optimal hierarchical modular topologies for producing limited sustained activation of neural networks. Front. Neuroinform. 4:8. doi: 10.3389/fninf.2010.00008
Kanai, R., and Rees, G. (2011). The structural basis of inter-individual differences in human behaviour and cognition. Nat. Rev. Neurosci. 12, 231–242.
Karlen, S. J., and Krubitzer, L. (2006). Phenotypic diversity is the cornerstone of evolution: variation in cortical field size within short-tailed opossums. J. Comp. Neurol. 499, 990–999.
Klein, J. C., Behrens, T. E. J., Robson, M. D., Mackay, C. E., Higham, D. J., and Johansen-Berg, H. (2007). Connectivity-based parcellation of human cortex using diffusion MRI: establishing reproducibility, validity and observer independence in BA 44/45 and SMA/pre-SMA. Neuroimage 34, 204–211.
Klingler, J., and Gloor, P. (1960). The connections of the amygdala and of the anterior temporal cortex in the human brain. J. Comp. Neurol. 115, 333–369.
Koch, M. A., Norris, D. G., and Hund-Georgiadis, M. (2002). An investigation of functional and anatomical connectivity using magnetic resonance imaging. Neuroimage 16, 241–250.
Krubitzer, L., and Kaas, J. (2005). The evolution of the neocortex in mammals: how is phenotypic diversity generated? Curr. Opin. Neurobiol. 15, 444–453.
Leone, M., Sumedha, and Weigt, M. (2008). Unsupervised and semi-supervised clustering by message passing: soft-constraint affinity propagation. Eur. Phys. J. B 66, 125–135.
Lewis, J. W., and Van Essen, D. C. (2000). Corticocortical connections of visual, sensorimotor, and multimodal processing areas in the parietal lobe of the macaque monkey. J. Comp. Neurol. 428, 112–137.
Li, K. M., Guo, L., Nie, J. X., Li, G., and Liu, T. M. (2009). Review of methods for functional brain connectivity detection using fMRI. Comput. Med. Imaging Graph. 33, 131–139.
Markov, N. T., Misery, P., Falchier, A., Lamy, C., Vezoli, J., Quilodran, R., Gariel, M. A., Giroud, P., Ercsey-Ravasz, M., Pilaz, L. J., Huissoud, C., Barone, P., Dehay, C., Toroczkai, Z., Van Essen, D. C., Kennedy, H., and Knoblauch, K. (2010). Weight consistency specifies regularities of macaque cortical networks. Cereb. Cortex. 21, 1254–1272.
Mars, R. B., Jbabdi, S., Sallet, J., O’Reilly, J. X., Croxson, P. L., Olivier, E., Noonan, M. P., Bergmann, C., Mitchell, A. S., Baxter, M. G., Behrens, T. E. J., Johansen-Berg, H., Tomassini, V., Miller, K. L., and Rushworth, M. F. S. (2011). Diffusion-weighted imaging tractography-based parcellation of the human parietal cortex and comparison with human and macaque resting-state functional connectivity. J. Neurosci. 31, 4087–4100.
Maunsell, J. H., and Van Essen, D. C. (1987). Topographic organization of the middle temporal visual area in the macaque monkey: representational biases and the relationship to callosal connections and myeloarchitectonic boundaries. J. Comp. Neurol. 266, 535–555.
McConnell, S. K., Ghosh, A., and Shatz, C. J. (1989). Subplate neurons pioneer the first axon pathway from the cerebral cortex. Science 245, 978–982.
Meier, J. D., Aflalo, T. N., Kastner, S., and Graziano, M. S. A. (2008). Complex organization of human primary motor cortex: a high-resolution fMRI study. J. Neurophysiol. 100, 1800–1812.
Mesulam, M. (2005). Imaging connectivity in the human cerebral cortex: the next frontier? Ann.Neurol. 57, 5–7.
Meunier, D., Lambiotte, R., and Bullmore, E. T. (2010). Modular and hierarchically modular organization of brain networks. Front. Neurosci. 4:200. doi: 10.3389/fnins.2010.00200
Miklossy, J., Clarke, S., and Van Der Loos, H. (1991). The long distance effects of brain lesions: visualization of axonal pathways and their terminations in the human brain by the Nauta method. J. Neuropathol. Exp. Neurol. 50, 595–614.
Miller, R. (1996). Axonal Conduction Time and Human Cerebral Laterality: A Psychobiological Theory. Amsterdam: Harwood Academic Publisher.
Moreno, D., Anwander, A., Schurade, R., and Knösche, T. R. (2011). “Connectivity-based whole-brain hierarchical parcellation of the human brain,” in 17th Annual Meeting of the Organization for Human Brain Mapping, Quebec.
Mori, S., and Van Zijl, P. C. M. (2002). Fiber tracking: principles and strategies – a technical review. NMR Biomed. 15, 468–480.
Mufson, E. J., Brady, D. R., and Kordower, J. H. (1990). Tracing neuronal connections in postmortem human hippocampal complex with the carbocyanine dye DiI. Neurobiol. Aging 11, 649–653.
Nanetti, L., Cerliani, L., Gazzola, V., Renken, R., and Keysers, C. (2009). Group analyses of connectivity-based cortical parcellation using repeated k-means clustering. Neuroimage 47, 1666–1677.
Nauta, W. J. H., and Gygax, P. A. (1951). Silver impregnation of degenerating axon terminals in the central nervous system: (1) technic. (2) Chemical notes. Stain Technol. 26, 5–11.
Nolte, G., and Muller, K. R. (2010). Localizing and estimating causal relations of interacting brain rhythms. Front. Hum. Neurosci. 4:209. doi: 10.3389/fnhum.2010.00209
Obermayer, K., Ritter, H., and Schulten, K. (1990). A principle for the formation of the spatial structure of cortical feature maps. Proc. Natl. Acad. Sci. U.S.A. 87, 8345–8349.
O’Muircheartaigh, J., Vollmar, C., Traynor, C., Barker, G., Kumari, V., Symms, M., Thompson, P., Duncan, J., Koepp, M., and Richardson, M. (2011). Clustering probabilistic tractograms using independent component analysis applied to the thalamus. Neuroimage 54, 2020–2032.
Op De Beeck, H. P., Haushofer, J., and Kanwisher, N. G. (2008). Interpreting fMRI data: maps, modules and dimensions. Nat. Rev. Neurosci. 9, 123–135.
Ozarslan, E., Shepherd, T. M., Vemuri, B. C., Blackband, S. J., and Mareci, T. H. (2006). Resolution of complex tissue microarchitecture using the diffusion orientation transform (DOT). Neuroimage 31, 1086–1103.
Parker, G. J. M., and Alexander, D. C. (2005). Probabilistic anatomical connectivity derived from the microscopic persistent angular structure of cerebral tissue. Philos. Trans. R. Soc. Lond. B Biol. Sci. 360, 893–902.
Parker, G. J. M., Haroon, H. A., and Wheeler-Kingshott, C. A. M. (2003). A framework for a streamline-based probabilistic index of connectivity (PICo) using a structural interpretation of MRI diffusion measurements. J. Magn. Reson. Imaging 18, 242–254.
Passingham, R. E., Stephan, K. E., and Kötter, R. (2002). The anatomical basis of functional localization in the cortex. Nat. Rev. Neurosci. 3, 606–616.
Petkov, C. I., Kayser, C., Augath, M., and Logothetis, N. K. (2006). Functional imaging reveals numerous fields in the monkey auditory cortex. PLoS Biol. 4, e215. doi: 10.1371/journal.pbio.0040215
Petrides, M. (2005). Lateral prefrontal cortex: architectonic and functional organization. Philos. Trans. R. Soc. Lond. B Biol. Sci. 360, 781–795.
Ragsdale, C. W., and Grove, E. A. (2001). Patterning the mammalian cerebral cortex. Curr. Opin. Neurobiol. 11, 50–58.
Rajkowska, G., and Goldman-Rakic, P. S. (1995). Cytoarchitectonic definition of prefrontal areas in the normal human cortex: II. Variability in locations of areas 9 and 46 and relationship to the Talairach coordinate system. Cereb. cortex 5, 323–337.
Rakic, P. (2009). Evolution of the neocortex: a perspective from developmental biology. Nat. Rev. Neurosci. 10, 724–735.
Saarinen, J., and Kohonen, T. (1985). Self-organized formation of colour maps in a model cortex. Perception 14, 711–719.
Salinas, E., and Sejnowski, T. J. (2001). Correlated neuronal activity and the flow of neural information. Nat. Rev. Neurosci. 2, 539–550.
Sanides, F. (1962). Architectonics of the human frontal lobe of the brain. With a demonstration of the principles of its formation as a reflection of phylogenetic differentiation of the cerebral cortex. Monogr. Gesamtgeb. Neurol. Psychiatr. 98, 1–201.
Schmahmann, J. D., and Pandya, D. N. (2006). Fibre Pathways of the Brain. Oxford: Oxford University Press.
Schoenemann, P. T. (2006). Evolution of the size and functional areas of the human brain. Annu. Rev. Anthropol. 35, 379–406.
Schubotz, R. I., Anwander, A., Knösche, T. R., Von Cramon, D. Y., and Tittgemeyer, M. (2010). Anatomical and functional parcellation of the human lateral premotor cortex. Neuroimage 50, 396–408.
Silver, M. A., and Kastner, S. (2009). Topographic maps in human frontal and parietal cortex. Trends Cogn. Sci. (Regul. Ed.) 13, 488–495.
Slonim, N., Atwal, G. S., Tkacik, G., and Bialek, W. (2005). Information-based clustering. Proc. Natl. Acad. Sci. U.S.A. 102, 18297–18302.
Smith, S. L., and Häusser, M. (2010). Parallel processing of visual space by neighboring neurons in mouse visual cortex. Nat. Neurosci. 13, 1144–1149.
Sporns, O., Tononi, G., and Edelman, G. M. (2002). Theoretical neuroanatomy and the connectivity of the cerebral cortex. Behav. Brain Res. 135, 69–74.
Stephan, K. E., Hilgetag, C. C., Burns, G. A., O’Neill, M. A., Young, M. P., and Kötter, R. (2000). Computational analysis of functional connectivity between areas of primate cerebral cortex. Philos. Trans. R. Soc. Lond. B Biol. Sci. 355, 111–126.
Stephan, K. E., Riera, J., Deco, G., and Horwitz, B. (2008). The brain connectivity workshops: moving the frontiers of computational systems neuroscience. Neuroimage 42, 1–9.
Stephan, K. E., Tittgemeyer, M., Knösche, T. R., Moran, R. J., and Friston, K. J. (2009). Tractography-based priors for dynamic causal models. Neuroimage 47, 1628–1638.
Sujazow, O., Schütte, C., Derrfuss, J., Melzer, C., Vogeley, K., Amunts, K., Von Cramon, D. Y., and Tittgemeyer, M. (2010). “Connectivity-based cortex parcellation of the anterior prefrontal cortex,” in 16th Annual Meeting of the Organisation for Human Brain Mapping, Barcelona, 1492 WTh-PM.
Toga, A. W., Thompson, P. M., Mori, S., Amunts, K., and Zilles, K. (2006). Towards multimodal atlases of the human brain. Nat. Rev. Neurosci. 7, 952–966.
Tomassini, V., Jbabdi, S., Klein, J. C., Behrens, T. E. J., Pozzilli, C., Matthews, P. M., Rushworth, M. F. S., and Johansen-Berg, H. (2007). Diffusion-weighted Imaging tractography-based parcellation of the human lateral premotor cortex identifies dorsal and ventral subregions with anatomical and functional specializations. J. Neurosci. 27, 10259–10269.
Tononi, G., Sporns, O., and Edelman, G. M. (1992). Reentry and the problem of integrating multiple cortical areas: simulation of dynamic integration in the visual system. Cereb. Cortex 2, 310–335.
Tononi, G., Sporns, O., and Edelman, G. M. (1994). A measure for brain complexity: relating functional segregation and integration in the nervous system. Proc. Natl. Acad. Sci. U.S.A. 91, 5033–5037.
Tournier, J.-D., Calamante, F., and Connelly, A. (2007). Robust determination of the fibre orientation distribution in diffusion MRI: non-negativity constrained super-resolved spherical deconvolution. Neuroimage 35, 1459–1472.
Tournier, J.-D., Calamante, F., Gadian, D. G., and Connelly, A. (2004). Direct estimation of the fiber orientation density function from diffusion-weighted MRI data using spherical deconvolution. Neuroimage 23, 1176–1185.
Traynor, C., Heckemann, R. A., Hammers, A., O’Muircheartaigh, J., Crum, W. R., Barker, G. J., and Richardson, M. P. (2010). Reproducibility of thalamic segmentation based on probabilistic tractography. Neuroimage 52, 69–85.
Tuch, D. S., Wisco, J. J., Khachaturian, M. H., Ekstrom, L. B., Kötter, R., and Vanduffel, W. (2005). Q-ball imaging of macaque white matter architecture. Philos. Trans. R. Soc. Lond. B Biol. Sci. 360, 869–879.
Uylings, H., Rajkowska, G., Sanz-Arigita, E., Amunts, K., and Zilles, K. (2005). Consequences of large interindividual variability for human brain atlases: converging macroscopical imaging and microscopical neuroanatomy. Anat. Embryol. (Berl.) 210, 423–431.
Van Den Heuvel, M. P., and Pol, H. E. H. (2010). Exploring the brain network: a review on resting-state fMRI functional connectivity. Eur. Neuropsychopharmacol. 20, 519–534.
Van Essen, D. C., and Dierker, D. (2007). On navigating the human cerebral cortex: response to “in praise of tedious anatomy”. Neuroimage 37, 1050–1054; discussion 1066–1058.
Varela, F., Lachaux, J. P., Rodriguez, E., and Martinerie, J. (2001). The brainweb: phase synchronization and large-scale integration. Nat. Rev. Neurosci. 2, 229–239.
Vogt, O. (1910). Die myeloarchitektonische Felderung des menschlichen Stirnhirns. J. Psychol. Neurol. 15, 221–232.
Wedeen, V. J., Hagmann, P., Tseng, W.-Y. I., Reese, T. G., and Weisskoff, R. M. (2005). Mapping complex tissue architecture with diffusion spectrum magnetic resonance imaging. Magn. Reson. Med. 54, 1377–1386.
Yo, T., Anwander, A., Descoteaux, M., Fillard, P., Poupon, C., and Knösche, T. R. (2009). Quantify brain connectivity: a comparative study of tractography. Lect. Notes Comput. Sci. 5761, 886–893.
Zalesky, A., Fornito, A., Harding, I. H., Cocchi, L., Yucel, M., Pantelis, C., and Bullmore, E. T. (2010). Whole-brain anatomical networks: does the choice of nodes matter? Neuroimage 50, 970–983.
Zhang, D., Snyder, A. Z., Fox, M. D., Sansbury, M. W., Shimony, J. S., and Raichle, M. E. (2008). Intrinsic functional relations between human cerebral cortex and thalamus. J. Neurophysiol. 100, 1740–1748.
Zilles, K. (2004). “Architecture of the human cerebral cortex – regional and laminar organisation,” in The Human Nervous System, eds J. K. Mai and G. Praxinas (Amsterdam: Academic Press), 997–1055.
Zilles, K., and Amunts, K. (2009). Receptor mapping: architecture of the human cerebral cortex. Curr. Opin. Neurol. 22, 331–339.
Zilles, K., and Amunts, K. (2010). Centenary of Brodmann’s map – conception and fate. Nat. Rev. Neurosci. 11, 139–145.
Zilles, K., Palomero-Gallagher, N., and Schleicher, A. (2004). Transmitter receptors and functional anatomy of the cerebral cortex. J. Anat. 205, 417–432.
Keywords: anatomical connectivity, functional connectivity, diffusion tractography, cortex area, connectivity-based parcellation
Citation: Knösche TR and Tittgemeyer M (2011) The role of long-range connectivity for the characterization of the functional–anatomical organization of the cortex. Front. Syst.Neurosci. 5:58. doi: 10.3389/fnsys.2011.00058
Received: 25 March 2011; Paper pending published: 20 April 2011;
Accepted: 25 June 2011; Published online: 07 July 2011.
Edited by:
Robert Turner, Max Planck Institute for Human Cognitive and Brain Sciences, GermanyCopyright: © 2011 Knösche and Tittgemeyer. This is an open-access article subject to a non-exclusive license between the authors and Frontiers Media SA, which permits use, distribution and reproduction in other forums, provided the original authors and source are credited and other Frontiers conditions are complied with.
*Correspondence: Marc Tittgemeyer, Max Planck Institute for Neurological Research, Gleueler Str. 50, D-50931 Cologne, Germany. e-mail:bWFyYy50aXR0Z2VtZXllckBuZi5tcGcuZGU=