- 1 Department of Neurobiology, Weizmann Institute of Science, Rehovot, Israel
- 2 Department of Anatomy and Neurobiology, Washington University School of Medicine, St. Louis, MO, USA
- 3 Center for Neuroscience, University of California at Davis, Davis, CA, USA
- 4 Department of Molecular and Cell Biology, University of California at Berkeley, Berkeley, CA, USA
- 5 Department of Biomedical Engineering, Columbia University, New York, NY, USA
- 6 Department of Physiology, Feinberg School of Medicine, Northwestern University, Chicago, IL, USA
- 7 Department of Psychology, University of Western Ontario, London, ON, Canada
This article was motivated by the conference entitled “Perception & Action – An Interdisciplinary Approach to Cognitive Systems Theory,” which took place September 14–16, 2010 at the Santa Fe Institute, NM, USA. The goal of the conference was to bring together an interdisciplinary group of neuroscientists, roboticists, and theorists to discuss the extent and implications of action–perception integration in the brain. The motivation for the conference was the realization that it is a widespread approach in biological, theoretical, and computational neuroscience to investigate sensory and motor function of the brain in isolation from one another, while at the same time, it is generally appreciated that sensory and motor processing cannot be fully separated. Our article summarizes the key findings of the conference, provides a hypothetical model that integrates the major themes and concepts presented at the conference, and concludes with a perspective on future challenges in the field.
Introduction
A widespread approach in biological, theoretical, and computational neuroscience has been to investigate and model sensory function of the brain in isolation from motor function, and vice-versa. At the same time, it is generally appreciated that sensory and motor processing cannot be fully separated; indeed, recent findings in neuroscience suggest that the sensory and motor functions of the brain might be significantly more integrated than previously thought. The purpose of the workshop on Perception and Action, held in September 2010 at the Santa Fe Institute in Sante Fe, New Mexico, was to bring together a multidisciplinary group of researchers to discuss the extent and implications of action–perception integration in the brain.
The workshop was organized by Nihat Ay, Ray Guillery, Bruno Olshausen, Murray Sherman, and Fritz Sommer. In addition to the workshop organizers a diverse group of researchers spoke at the workshop: Ehud Ahissar, Josh Bongard, Andy Clark, Carol Colby, Ralf Der, Keyan Ghazi-Zahedi, Jeff Hawkins, Christopher Moore, Kevin O’Regan, Daniel Polani, Marc Sommer, and Naftali Tishby. The attendance of graduate students and post-doctoral researchers, who are the authors of this article, was supported by travel grants from the Santa Fe Institute. A complete list of participants and presentations can be found on the conference web site: http://tuvalu.santafe.edu/events/workshops/index.php/Perception_and_Action_-_an_Interdisciplinary_Approach_to_Cognitive_Systems_Theory
In this article, we summarize key findings presented at the workshop with a focus on (1) biological evidence for a strong integration of perception and action in the brain and (2) the implications of closed action–perception loops for computational modeling. We also highlight some of the recurring themes that were presented and discussed at the workshop and conclude with a perspective on future challenges in the field.
Biological Evidence for Integration of Perception and Action in the Brain
Foundational anatomical and physiological studies have provided substantial evidence for the integration of motor and sensory functions in the brain (for a recent review, see Guillery and Sherman, 2011). Regarding the neuroanatomy of the thalamus and cortex, Guillery and Sherman have noted that most, if not all, ascending axons reaching the thalamus for relay to the cortex have collateral branches that innervate the spinal cord and motor nuclei of the brainstem (see Guillery, 2005). Similarly, those cortico-cortical connections that are relayed via higher-order thalamic structures, such as the pulvinar nucleus, also branch to innervate brainstem motor nuclei. Guillery and Sherman thus hypothesize that a significant portion of the driving inputs to thalamic relay nuclei are “efference copies” of motor instructions sent to subcortical motor centers, suggesting a more pervasive ambiguity between sensory and motor signals than has previously been acknowledged.
“Efference Copies” (von Holst and Mittelstaedt, 1950), or alternatively termed “Corollary Discharges” (Sperry, 1950), have long been hypothesized to establish sensorimotor contingencies in perception (for review see, e.g., Poulet and Hedwig, 2007). While the work of Guillery and Sherman demonstrates the prominence of collateral connections in the anatomy of motor and sensory pathways, it does not directly implicate these connections in the encoding of sensorimotor contingencies. Neurophysiological evidence for motor feedback in sensory processing can be found in the remapping of visual receptive fields (RFs) across several visual and association cortices. For example, during directed saccadic eye movement tasks, the spatial location of visual RFs of Frontal Eye Field (FEF) neurons have been demonstrated to shift, prior to saccade, to the projected post-saccadic target (Sommer and Wurtz, 2008). Building on these results, Marc Sommer presented evidence that the superior colliculus (SC), a brain stem nucleus known to play an important role in the generation of eye movements, sends projections to the FEF via the mediodorsal (MD) thalamus. Consistent with the hypothesis that this SC–MD–FEF pathway may be carrying efference copies of saccade motor commands required for accurate spatial remapping of RFs in FEF, Sommer found that inactivation of the MD nucleus during behavioral tasks greatly diminishes such remapping (Crapse and Sommer, 2009). Carol Colby has reported that efference copy-based remapping is a widespread phenomenon in the visual system, occurring in the lateral intraparietal area (LIP), V4, V3A, V2, and possibly even as early in the visual system as the primary visual cortex (V1; Berman and Colby, 2009). These results suggest that efference copies find widespread use throughout the cortex, including early visual cortices.
A clear example of the role of motor pathways in the representation of external objects came from the lab of Ehud Ahissar. Analyzing the vibrissae system of rats and its role in object localization, Ahissar showed how this inherently active sensory modality is anatomically organized to form a nested set of hierarchical feedback loops including sensory and motor circuitry of the brainstem, thalamus, SC, and the cortex (Yu et al., 2006). His lab has provided behavioral and neurophysiological evidence that object localization is encoded in the steady-state activation of an entire sensory-motor loop pathway, with convergence emerging over approximately four whisking cycles (Knutsen and Ahissar, 2009). This demonstrates, for one sensory modality, the critical importance of putting motor action into perception.
Implications of Closed Action-Perception Loops for Computational Modeling
Two common themes emerged with regard to the implications of action-perception loops for computational modeling. The first theme was how embodiment, the opportunities, and constraints imposed by an agent’s body on motor action and perception, can shape learning, and facilitate information processing (Pfeifer and Bongard, 2006). The second theme was that action strategies, through their interaction with the external environment, can shape the flow of information being processed by an active, embodied agent.
Josh Bongard argued for an embodied approach to building robots that explicitly highlights the role of active motor exploration and morphological factors for aiding or scaffolding sensorimotor learning, computation, cognitive development, and biological evolution (Bongard et al., 2006). Bongard’s lab has designed and constructed robots capable of autonomously generating internal models for motor control through adaptive exploration of their own bodily sensorimotor contingencies. Experiments centered on the these robots have demonstrated that gradual changes to a simulated robot’s physical form across “developmental/ontogenetic” timescales can facilitate learning of sensorimotor contingencies by guiding the learning process along a gradual trajectory toward its mature state. Remarkably, by tying the learning process directly to exploration of the sensorimotor space, his robots demonstrated resilience to major morphological changes and were capable of relearning and adapting their internal self-models after drastic changes such as damage or loss of limbs.
To achieve an effective internal model, Bongard’s robots generate a set of “hypotheses” and then choose actions that yield differing expectations under those “hypotheses.” Thus, the actions of the robot determine the information it receives and uses to improve its internal model. This idea of action strategies determining the flow of information was reiterated frequently during the theoretical neuroscience and cognitive philosophy sessions of the workshop. Each researcher, however, presented different objectives in the regulation of information flow by action strategy. Andy Clark, for example, presented a unifying theory of the predictive brain, in which all of the brain’s operations can be understood as being optimized to reduce prediction error (Lee and Mumford, 2003; Friston, 2005). Ralf Der, in contrast, suggested that minimization of post-diction error, not prediction error, represents a fundamental objective of behavior (Hesse et al., 2009). Der presented simulated robots that learned to self-organize toward minimizing post-diction error, an objective that achieved homeokinesis and yielded a vast range of coherent and playful behaviors.
Nihat Ay and Keyan Ghazi-Zahedi integrated the themes of embodiment and control of information flow by suggesting that a learning agent can export much of its behavioral information to the external world (Der et al., 2008; Zahedi et al., 2010). Drawing from the field of Information Theory, they showed that maximization of predictive information, the mutual information between past and future senses, yielded explorative behaviors across a variety of simulated robots. Interestingly, it has been shown that this principle of maximizing predictive information proposed by Ay is mathematically equivalent to the principle of minimization of post-diction error articulated by Der, thus tying together several information-theoretic approaches.
While the unified principle presented by Der, Ay, and Ghazi-Zahedi operated on the information flow within a single model of the world and converged to a single optimal action strategy, Fritz Sommer, presenting work done in collaboration with Daniel Little, introduced a different principle that operated on the flow of information between changing internal models of the world. As Bongard’s robots choose actions that help them to distinguish between competing hypotheses, Sommer postulated that choosing action to maximize the expected gain in internal model accuracy was a fundamental feature of exploratory learning. Using value-iteration to predict information gains multiple time steps into the future, he showed that embodied agents could achieve, under this objective function, efficient learning of the world dynamics (the transition probabilities of a Controllable Markov Chain). He also demonstrated that this efficient learning allowed the agents to become better navigators of their world.
Daniel Polani further supported the primacy of Information Theory in modeling action-perception loops in the brain, arguing that Shannon information is the proper currency of brain function as it is measured in unitless bits and is coordinate-free. He showed that, for a given scenario, the total information to be gained or exploited is invariant, but its actual accumulation can be spread between sensors or over time. He further suggested that information can measure not only the knowledge to be obtained about one’s external world (a gain) but also the complexity of the action strategy utilized to exploit the world (a cost). Incorporating this cost on decision-making, Polani defined relevant information as the minimal amount of information required to achieve a certain goal. In doing so, Polani effectively linked the emerging information-theoretic approaches to the more classic studies of reinforcement learning. He also presented the concept of empowerment as an information-theoretic measure of the impact that an action choice has on the external world. In simulations, strategies for achieving high empowerment gave rise to unique behaviors driving agents toward critical states (such as a pendulum arm balancing in an unstable upright vertical position; Klyubin et al., 2008).
Concluding the conference, Naftali Tishby further attempted to unify Information Theory with value-seeking decision principles. Like Sommer, Tishby argued that information itself is a “value” and that actions can generate information across a wide range of timescales, from the immediate to the very distant future. In his words, “Life is exploiting the predictability of the environment.” Tishby however considered the value of information within the context of specific tasks. He suggested that action strategies should balance the increase of environmental predictability with the maximization of the objective value of a specific task. To that effect he offered a model that integrates information gain with externally defined value functions and formulated the “Info-Bellman” equation (Tishby and Polani, 2010), which is an extension of the iterative Bellman equation from the field of reinforcement learning.
Interestingly, when it comes to predicting and integrating value (defined by Information Theory) over time, these three researchers – Sommer, Polani, and Tishby – shared a common approach: By casting their respective objective functions in terms of the classic Bellman equations, they were able to use Control Theory to identify optimal action policies. Indeed, Tishby coined the term “Info-Bellman” to emphasize how his approach merges Information Theory and Control Theory.
On the Necessity of Action in Perception
As experimental neuroscientists provide increasing evidence for the integration of motor and sensory systems in the brains of both vertebrates (see results described in the previous paragraphs, and many more examples such as: Liberman, 1996, 2010; Eliades and Wang, 2003; Rauschecker, 2011; Scheich et al., 2011) and invertebrates (e.g., Chiappe et al., 2010; Haag et al., 2010; Maimon et al., 2010; Tang and Juusola, 2010), and as computational neuroscientists continue studying the theoretical benefits of directly incorporating actions into computational models of perception, a fundamental question remains: how inherent are actions in perception? That is, to what extent can the neural mechanisms of perception be understood free of its behavioral context? A lively debate arose at the conference around this issue. Arguing that actions are necessary for perception, Kevin O’Regan proposed that perception arises only from identification of sensorimotor contingencies (O’Regan, 2010). In support of this hypothesis, he provided evidence that the perception of space and of color can both be explained as identification of motor invariants of sensory inputs (Philipona et al., 2003; Philipona and O’Regan, 2006).
In contrast, Jeff Hawkins argued that much of perception can potentially be understood without direct consideration of actions. Toward this end, he presented Hierarchical Temporal Memory (HTM) networks, inspired by neocortical organization, that were capable of implementing temporal sequence learning, prediction, and causal inference all free of any behavioral context (George and Hawkins, 2009). While he acknowledged the potential information content of actions, he argued that it did not fundamentally change the basic computational principles of the neocortex. Instead, Hawkins argued that the motor pathways are one of several means by which critical temporal information is transferred to the brain, thereby enhancing the information flow from sensory pathways. One conclusion to be drawn from Hawkins’ HTM network model is that sensorimotor interaction with the environment, is, strictly speaking, unnecessary for learning, perception, and prediction, because the temporal information required for such capacities is already included in the sequence of sensory events. Nevertheless, it remains an important open question whether HTM networks can accurately model information processing as it is performed by the neocortex.
Future Perspective: Toward Understanding Action–Perception Loops in the Brain
During the workshop, Ray Guillery pointed out that “frogs can catch flies,” to emphasize the important fact that the mammalian cortex evolved in the context of a brain that was already capable of closing the action–perception loop. This, along with Guillery’s demonstration that nearly all levels of sensory processing receive efferent motor commands, suggest that understanding the hierarchy of nested sensorimotor pathways present in the mammalian brain may provide valuable insights into their function. Studying how such loops integrate sensation and movement at the lowest level of the hierarchy, across diverse species, may therefore lead to an understanding of the foundations upon which more complex sensorimotor loops, and perhaps even higher level cognitive capacities, are built.
Goren Gordon suggested that the research lines presented at the workshop could be categorized along an action–perception–prediction axis, and along an axis describing the degree of hierarchical complexity. Under this framework, the biologically oriented presentations (e.g., Ahissar, Colby, Guillery, Sherman, M. Sommer, Colby), captured the hierarchical nature of action–perception loops, yet lacked predictive capacities. On the other hand, the computational and theoretical models discussed in the workshop (e.g., Ay, Clark, Der, Ghazi-Zehadi, Polani, F. Sommer, Tishby, and O’Regan) were prediction-oriented, but failed to model more than a single instantiation of the action–perception loop. Hawkins’ HTM model was an exception that incorporated both a hierarchical structure and predictive capabilities, but it did so while ignoring the role of actions in prediction and perception. Intriguingly, a multi-hierarchical action–perception–prediction model remains to be explored in both the biological and computational communities. Toward this goal, empirical data are needed regarding the neural substrates underlying the predictive capacities posited by computational and information-theoretic models, as are testable hypotheses generated in action–perception–prediction models implementing the hierarchical architecture observed by empirical studies.
Unification and Progression
The hypothetical model outlined in Figure 1 is an attempt to integrate the major themes and concepts presented at the conference. It provides a framework to consolidate some of the key insights: the basic constituents are forward and inverse models (Shadmehr and Krakauer, 2008). A forward model receives the current state and an efference copy of an action and predicts the subsequent state. It can thus generate a prediction error, a concept discussed in the information-theoretic talks. Higher level forward models predict more complex states. An inverse model, on the other hand, receives a desired goal and generates a motor command that attempts to achieve that goal. It is equivalent to the policy described in reinforcement learning and to the anatomical motor regions. Lower inverse models serve as motor primitives for higher inverse models. The latter’s “motor command” (solid arrow) is then the activation of these primitives.
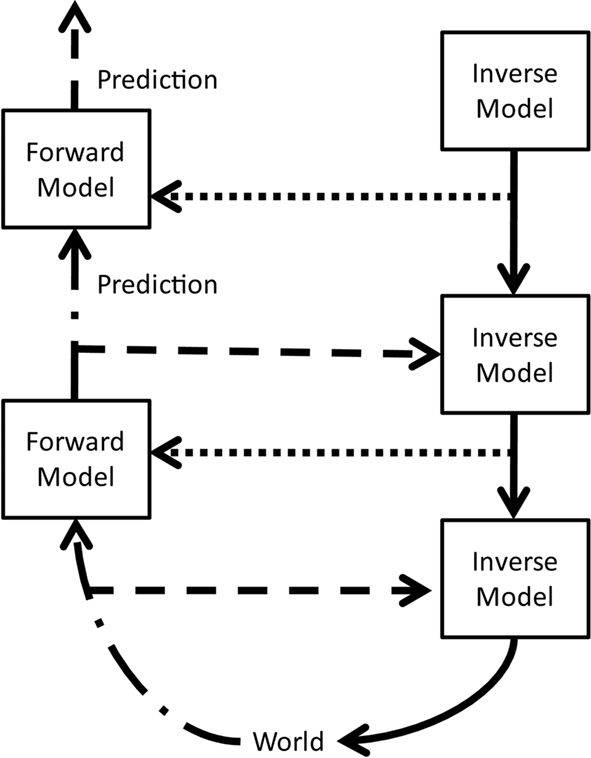
Figure 1. A hypothetical model of hierarchical action–perception loops. Forward models receive the current state (dash-dot arrows) and an efference copy (dotted arrows) from lower loops and send their predicted state to higher loops; inverse models receive a copy of the current state (dashed arrows) and the goal state (solid arrows) from higher loops and send the motor command to lower loops. The same model can also be interpreted in a different manner: higher motor regions (inverse models) send motor commands (solid arrows) to lower motor regions and collateral efference copies (dotted arrows) to sensory regions (forward models). Lower sensory regions send predictions (dash-dot arrows) to higher areas and collateral motor commands (dashed arrows) to motor regions. Hence the ascending predictions (dash-dot arrows) can be viewed as efference copies of their collaterals (dashed arrows). The two views emphasize the inability to separate sensory/perception from motor/action in such hierarchical loops.
The concept of hierarchies or multiple nested loops, suggested by Ahissar and Hawkins, is represented in that lower loops send information to higher ones (dash-dot arrows), whereas the higher loops send goals or commands to the lower ones (solid arrows). Efference copies of motor commands from higher motor regions to lower ones, as suggested by Colby and M. Sommer, are represented by dotted arrows. Information sent from lower forward models to higher ones (dash-dot arrows) also travels via collaterals (dashed arrows) to motor regions, i.e., inverse models, and hence can be considered as efference copies as suggested by Guillery and Sherman.
As forward models are predictors, their output can correspond to the prediction error described by Ay, Clark, Polani, F. Sommer, and Tishby. They influence the policy regions, here described by the inverse models. Furthermore, changing the policy via the info-Bellman equation per Tishby, empowerment per Polani, or information-seeking exploration per Sommer, corresponds to modifying parameters of the inverse model.
Taken together, this hypothetical model corresponds to the concept suggested by O’Regan and Ahissar, namely that perception is sensorimotor contingency, or convergence of sensorimotor loops.
Open Questions
The conference was a step toward bridging disciplines that are divided in their conceptual frameworks and methodologies, yet many open questions remain. In particular, substantial discussion revolved around how an understanding of the relationship between action and perception can inform and constrain (1) experimental design in sensory and motor neuroscience, (2) future theoretical work to model neural systems, and (3) the construction and design of intelligent machines. The breadth of these applications alerts us that common metrics must be developed in order to facilitate direct communication between these different fields. Here we briefly consider several other open questions, which we believe to be of critical importance.
• Is action required for perception? O’Regan suggested that perception requires knowledge of sensorimotor contingencies formed through motor interaction with the environment, and that our perception of space is itself shaped by the potential actions one might perform in space. Tishby raised the contrasting proposition that perception lies in the hypothesis generation and testing done by information gain maximization and hence does not necessarily require motor action. Also in contrast stood Hawkins’ model for passively generating sequence-memories to learn prediction. Is passive exposure to temporally varying stimuli sufficient to produce a percept, or is voluntary action mandatory?
• When are sensory and motor signals no longer distinct? Guillery hypothesized that thalamic inputs to all cortical areas carry efference copies of motor commands intertwined with sensory information from receptors. O’Regan suggested perception might be the enactment of sensorimotor contingencies.
• Which timescales are relevant for the integration of action and perception? Ahissar proposed that perception in whisking rats arises after four whisking cycles, i.e., after several hundred milliseconds. Bongard suggested that ontogenetic timescales are required to build the infrastructure for action–perception loops How much does our perception depend on the active experience of evolutionary ancestors (phylogenetic timescale), and how much does it depend on the experience of an individual (ontogenetic timescale)?
• Is the relationship between Action and Perception invariable? There are actions that could be considered “directly mapped,” such as a hand movement whose direction is based on the sensed location of a target such as a coin. However, there are also responses that could be considered “symbolically mapped,” such as a hand movement whose direction is tied to the sensed symbolic value of coin (e.g., left movement if it is heads, right movement if it is tails). It is known that different brain areas are involved in the computation of different perceptual attributes, such as location and identity for example (Mishkin and Ungerleider, 1982), and this might translate as differences in the brain areas involved in different kinds of responses (Goodale and Milner, 1992). The question arises if the same perceptual and motor contingencies apply for directly vs. symbolically mapped responses.
• Is prediction central to the action–perception framework? Clark proposed this explicitly, and many others tacitly argued for this idea. Prediction, predictive coding/remapping (“future response fields” per Colby and Sommer), and predictive information figured prominently in many of the theoretical models, especially those incorporating considerations from Markov Decision theory (Ay, Ghazi-Zahedi, Polani, Tishby, F. Sommer), and in robotics (Bongard). What are the neural correlates of these predictive mathematical constructs?
• What are the implications of embodiment on action and perception? Polani showed that the Acrobot, a two-degrees-of-freedom simulated robotic arm driven only by empowerment, reaches the uniquely unstable inverted point, often defined as the goal for optimal control problems. O’Regan presented a mathematical model able to learn the dimensionality of real space only by finding a unique compensable subspace within the highly dimensional sensorimotor manifold (Philipona et al., 2003). This suggests that sensorimotor contingencies can teach the agent about physical space. Can a generalized relationship between information-driven sensorimotor contingencies and embodiment be formulated?
• How much can be learned from constrained experiments and simplified simulations? Experimental studies of the mammalian brain often rely on reduced preparations or unnaturally constrained behavior. Likewise, theoretical treatments and computational simulations often make many simplifying assumptions. In both cases, the hope is that careful study of limited systems will result in findings that are relevant to the full system of interest. We believe that sensory and motor functions are highly interdependent, that the brain is a complex network of interconnected circuits, and that embodiment is vital to interaction with the environment. These beliefs stand in contrast to the approximations made in most conventional lines of study. Perhaps collaboration across disciplines and generations of researchers can push our studies closer to real, intricate, complex phenomena.
We believe that the interaction between experimental-, computational-, and robotic-oriented researchers can facilitate the understanding of brain function. Roboticists have used inspiration from neurobiological systems to construct complex controllers for embodied agents. How might their insights inform neurobiological experiments? Experimental neuroanatomy and neurophysiology have provided evidence of sensorimotor loops and efference copies throughout the brain. Can these findings further inform theoreticians about how to model closed action–perception loops? Theoreticians have proposed several elegant models of action–perception–prediction loops as the basis of animal behaviors. With more regular dialog, could theoretical models be formulated to generate testable experimental predictions?
The recent workshop on Perception and Action at the Santa Fe Institute has awakened a welcome dialog between researchers from a variety of disciplines, and has emphasized the important interplay between perception and action. Many open questions still remain, and the field will undoubtedly benefit from further collaboration between these different disciplines, and possibly others. In our view, the defining accomplishment of the meeting was to initiate interdisciplinary discussion among modelers, roboticists, and experimental neuroscientists, and to identify questions and themes for future exploration.
Conflict of Interest Statement
The authors declare that the research was conducted in the absence of any commercial or financial relationships that could be construed as a potential conflict of interest.
Acknowledgments
We thank Nihat Ay, Ray Guillery, Bruno Olshausen, Murray Sherman, and Fritz Sommer for organizing the conference and for encouraging the authors to collaborate on this article. We thank the conference attendees for many insightful discussions, and Bruce Bertram for help with conference logistics. This work was supported by grants from the Santa Fe Institute for student travel and publication fees. David M. Kaplan was supported by NEI-EY012135. Benjamin S. Lankow was funded by NIH grant EY013588. Daniel Y. Little was supported by the Redwood Center for Theoretical and Computational Neuroscience Graduate Student Endowment. Jason Sherwin was supported by DARPA contract #N10PC20050. Benjamin A. Suter was supported by the NIH under the Neuroscience in the Early Years T32 Training Program. Lore Thaler was supported by a Postdoctoral Fellowship from the Canadian Ministry of Research and Innovation (Ontario).
Conference Website Links
List of presentations and workshop agenda
List of participants
References
Bongard, J., Zykov, V., and Lipson, H. (2006). Resilient machines through continuous self-modeling. Science 314, 1118–1121.
Chiappe, M., Seelig, J., Reiser, M., and Jayaraman, V. (2010). Walking Modulates Speed Sensitivity in Drosophila Motion. Vis. Curr. Biol. 20, 1470–1475.
Crapse, T. B., and Sommer, M. A. (2009). Frontal eye field neurons with spatial representations predicted by their subcortical input. J. Neurosci. 29, 5308–5318.
Der, R., Güttler, F., and Ay, N. (2008). “Predictive information and emergent cooperativity in a chain of mobile robots,” in Artificial Life XI: Proceedings of the Eleventh International Conference on the Simulation and Synthesis of Living Systems, eds S. Bullock, J. Noble, R. Watson, and M. A. Bedau (Cambridge, MA: MIT Press), 166–172.
Eliades, S. J., and Wang, X. (2003). Sensory-Motor Interaction in the Primate Auditory Cortex During Self-Initiated Vocalizations. J. Neurophysiol. 89, 2194–2207.
Friston, K. (2005). A theory of cortical responses. Philos. Trans. R. Soc. Lond., B, Biol. Sci. 360, 815–836.
George, D., and Hawkins, J. (2009). Towards a mathematical theory of cortical micro-circuits. PLoS Comput. Biol. 5, e1000532. doi: 10.1371/journal.pcbi.1000532
Goodale, M. A., and Milner, A. D. (1992). Separate visual pathways for perception and action. Trends Neurosci. 15, 20–25.
Guillery, R. W. (2005). Anatomical pathways that link perception and action. Prog. Brain Res. 149, 235–256.
Guillery, R. W., and Sherman, S. M. (2011). Branched thalamic afferents: what are the messages that they relay to the cortex? Brain Res. Rev. 66, 205–219.
Haag, J., Wertz, A., and Borst, A. (2010). Central gating of fly optomotor response. Proc. Natl. Acad. Sci. 107, 20104–20109.
Hesse, F., Der, R., and Herrmann, J. M. (2009). Modulated exploratory dynamics can shape self-organized behavior. Adv. Comp. Syst. 12, 273–291.
Klyubin, A. S., Polani, D., and Nehaniv, C. L. (2008). Keep your options open: an information-based driving principle for sensorimotor systems. PLoS ONE 3, e4018. doi: 10.1371/journal.pone.0004018
Knutsen, P. M., and Ahissar, E. (2009). Orthogonal coding of object location. Trends Neurosci. 32, 101–109.
Lee, T. S., and Mumford, D. (2003). Hierarchical Bayesian inference in the visual cortex. J. Opt. Soc. Am. A 20, 1434–1448.
Lisberger, S. G. (2010). Visual guidance of smooth-pursuit eye movements: sensation, action, and what happens in between. Neuron 66, 477–491.
Maimon, G., Straw, A., and Dickinson, M. (2010). Active flight increases the gain of visual motion processing in Drosophila. Nat. Neurosci. 13, 393–399.
Mishkin, M., and Ungerleider, L. G. (1982). Contribution of striate inputs to the visuospatial functions of parieto-preoccipital cortex in monkeys. Behav. Brain Res. 6, 57–77.
O’Regan, J. K. (2010). “Explaining what people say about sensory qualia,” in Perception, Action, and Consciousness: Sensorimotor Dynamics and Two Visual Systems, eds N. Gangopadhyay, M. Madary, and F. Spicer (Oxford: Oxford University Press). 31–50.
Pfeifer, R., and Bongard, J. (2006). How the Body Shapes the Way We Think: A New View of Intelligence. Cambridge, MA: Bradford Books, The MIT Press.
Philipona, D., O’Regan, J. K., and Nadal, J. P. (2003). Is there something out there?: Inferring space from sensorimotor dependencies. Neural. Comput. 15, 2029–2049.
Philipona, D. L., and O’Regan, J. K. (2006). Color naming, unique hues, and hue cancellation predicted from singularities in reflection properties. Vis. Neurosci. 23(3–4), 331–339.
Poulet, J. F. A., and Hedwig, B. (2007). New insights into corollary discharges mediated by identified neural pathways. Trends Neurosci. 30, 14–21.
Rauschecker, J. P. (2011). An expanded role for the dorsal auditory pathway in sensorimotor control and integration. Hear. Res. 271, 16–25.
Scheich, H., Brechmann, A., Brosch, M., Budinger, E., Ohl, F. W., Selezneva, E., Stark, H., Tischmeyer, W., and Wetzel, W. (2011). Behavioral semantics of learning and crossmodal processing in auditory cortex: the semantic processor concept. Hear. Res. 271, 3–15.
Shadmehr, R., and Krakauer, J. W. (2008). A computational neuroanatomy for motor control. Exp. Brain Res. 185, 359–381.
Sommer, M. A., and Wurtz, R. H. (2008). Brain circuits for the internal monitoring of movements. Annu. Rev. Neurosci. 31, 317–338.
Sperry, R. W. (1950). Neural basis of the spontaneous optokinetic response produced by visual inversion. J. Comp. Physiol. Psychol. 43, 482–489.
Tang, S., and Juusola, M. (2010). Intrinsic activity in the fly brain gates visual information during behavioral choices. PLoS ONE 5, e14455. doi: 10.1371/journal.pone.0014455
Tishby, N., and Polani, D. (2010). “Information theory of decisions and actions,” in Perception-Action Cycle: Models, Architectures and Hardware, eds V. Cutsuridis, A. Hussain, and J. G. Taylor (New York, NY: Springer). 601–636.
von Holst, E., and Mittelstaedt, H. (1950). Das Reafferenzprinzip: Wechselwirkungen zwischen Zentralnervensystem und Peripherie. Naturwissenschaften 37, 464–476.
Keywords: conference, embodiment, interdisciplinary, robotics, sensorimotor, perception, action
Citation: Gordon G, Kaplan DM, Lankow B, Little DY-J, Sherwin J, Suter BA and Thaler L (2011) Toward an integrated approach to perception and action: conference report and future directions. Front. Syst. Neurosci. 5:20. doi: 10.3389/fnsys.2011.00020
Received: 02 February 2011; Accepted: 07 April 2011;
Published online: 25 April 2011.
Edited by:
Andrew J. Parker, University of Oxford, UKReviewed by:
Björn Brembs, Freie Universität Berlin, GermanyMichael Brosch, Leibniz Institute for Neurobiology, Germany
Copyright: © 2011 Gordon, Kaplan, Lankow, Little, Sherwin, Suter and Thaler. This is an open-access article subject to a non-exclusive license between the authors and Frontiers Media SA, which permits use, distribution and reproduction in other forums, provided the original authors and source are credited and other Frontiers conditions are complied with.
*Correspondence: Benjamin A. Suter, Department of Physiology, Feinberg School of Medicine, Northwestern University, 303 E. Chicago Avenue, Chicago, IL 60611, USA. e-mail:YmVuc3V0ZXJAdS5ub3J0aHdlc3Rlcm4uZWR1