- 1 Department of Veterans Affairs Northern California Health Care System, Center for Aphasia and Related Disorders, Martinez, CA, USA
- 2 Neurology Department, University of California Davis, Davis, CA, USA
While traditional models of language comprehension have focused on the left posterior temporal cortex as the neurological basis for language comprehension, lesion and functional imaging studies indicate the involvement of an extensive network of cortical regions. However, the full extent of this network and the white matter pathways that contribute to it remain to be characterized. In an earlier voxel-based lesion-symptom mapping analysis of data from aphasic patients (Dronkers et al., 2004), several brain regions in the left hemisphere were found to be critical for language comprehension: the left posterior middle temporal gyrus, the anterior part of Brodmann’s area 22 in the superior temporal gyrus (anterior STG/BA22), the posterior superior temporal sulcus (STS) extending into Brodmann’s area 39 (STS/BA39), the orbital part of the inferior frontal gyrus (BA47), and the middle frontal gyrus (BA46). Here, we investigated the white matter pathways associated with these regions using diffusion tensor imaging from healthy subjects. We also used resting-state functional magnetic resonance imaging data to assess the functional connectivity profiles of these regions. Fiber tractography and functional connectivity analyses indicated that the left MTG, anterior STG/BA22, STS/BA39, and BA47 are part of a richly interconnected network that extends to additional frontal, parietal, and temporal regions in the two hemispheres. The inferior occipito-frontal fasciculus, the arcuate fasciculus, and the middle and inferior longitudinal fasciculi, as well as transcallosal projections via the tapetum were found to be the most prominent white matter pathways bridging the regions important for language comprehension. The left MTG showed a particularly extensive structural and functional connectivity pattern which is consistent with the severity of the impairments associated with MTG lesions and which suggests a central role for this region in language comprehension.
Introduction
The brain regions that subserve verbal comprehension are traditionally associated with Wernicke’s and, to some extent, Broca’s areas. Following the seminal work of Wernicke (1874), who described neurological patients with trouble understanding spoken language, it has been assumed that the posterior superior temporal cortex in the left hemisphere is primarily responsible for language comprehension (Geschwind, 1970, 1972; Goodglass, 1993). Broca’s area has also been implicated in sentence comprehension in that patients with Broca’s aphasia have difficulty processing the syntactic rules that affect sentence meaning. However, this position is gradually giving way to a network perspective, which emphasizes the contribution of several brain regions organized into a large-scale network via long-distance association pathways (Mesulam, 1990; Damasio, 1991). Lesion–deficit correlation findings with aphasic patients have led to an appreciation of how several other brain regions within and outside the left temporal lobe also contribute to language comprehension. It has been shown in several studies that language comprehension deficits can be produced by lesions in several posterior and frontal cortical areas and subcortical structures (Kertesz et al., 1979, 1982, 1993; Naeser et al., 1982, 1987; Alexander et al., 1989; Damasio, 1992; Dronkers et al., 1994, 2000b, 2004; Caplan et al., 1996, 2007; Damasio and Damasio, 2002; Bates et al., 2003; Binder, 2003; Tyler and Marslen-Wilson, 2008). Functional neuroimaging investigations have also highlighted distributed activation patterns in the healthy brain during the performance of tasks that involve language comprehension and the processing of lexical-semantic information (Demonet et al., 1992; Demb et al., 1995; Binder et al., 1997, 2009; Price, 2000, 2010; Bookheimer, 2002; Friederici, 2002; Vigneau et al., 2006; Ferstl et al., 2008).
Thus, an extensive left-lateralized network for language comprehension is indicated by both lesion–symptom correlations in aphasia and functional imaging findings in the healthy brain. This network is distributed throughout association areas in the left peri-sylvian cortex and neighboring regions, including parts of the posterior middle temporal gyrus (MTG), inferior temporal regions, the inferior parietal lobe (IPL), inferior frontal gyrus (IFG), as well as other frontal regions not directly involved in language but facilitating comprehension through working memory and cognitive control operations (Dronkers et al., 2004). The anatomical extent of this network is consistent with the notion that language comprehension is a complex process which, like other complex behaviors, is the product of the coordinated activity of several brain regions, each contributing to several subprocesses of comprehension. The functioning of the overall network can be disrupted by brain lesions damaging different cortical nodes and disconnecting the white matter (WM) pathways that subserve their interactions. Better understanding of the functional anatomy of the language comprehension network and its disorders requires a detailed characterization of the underlying connectional architecture that allows the functional integration of the brain structures that comprise the language network.
The specific contributions of individual brain regions to the large-scale networks specialized for different aspects of cognition are determined by their connectivity patterns (Mesulam, 1990, 1998, 2005; Schmahmann and Pandya, 2008). Each brain region acquires its particular function in a brain network by virtue of how it interacts with other regions in the brain. This highlights the need to delineate the WM pathways that interconnect distinct components of the language network in order to elucidate how each part is functionally integrated to the overall network and makes specific contributions to language processing, and to understanding why lesions in different brain regions cause the particular symptoms observed in aphasic patients. Behavioral deficits can also be produced by WM lesions that disconnect healthy brain regions, and the resulting deficits can be as severe as those produced by damage to the areas that subserve cortical information processing (Lichtheim, 1885; Geschwind, 1965a,b; Catani and Mesulam, 2008a,b). Reorganization of the architecture of functional networks is a key factor for the recovery of function following neurological injury, and this process might be mediated by the connections that allow intact regions to acquire new functions and enable the surviving sections of damaged regions to be reintegrated into functional networks (Heiss et al., 2003; Dancause et al., 2005; Ward, 2005; Marsh and Hillis, 2006; Murphy and Corbett, 2009). Thus, better knowledge of the connectional anatomy of the language network is essential for understanding the consequences of the disruption of WM pathways due to brain injury, and the contributions of these pathways to functional recovery.
While the arcuate fasciculus (AF) has traditionally been viewed as the single critical WM pathway that provides the scaffolding for the language network (Dronkers et al., 2000a; Catani and Mesulam, 2008a; Friederici, 2009), the growing knowledge of WM anatomy indicates that the language network is subserved by a richer network of fiber systems than originally appreciated. The arcuate itself has been shown to be composed not of a single arc, but at least three segments, including a direct pathway between temporal and frontal regions, and an indirect segment involving inferior parietal regions (Catani et al., 2005). Histological tracing studies of the homologues of human language areas in the monkey brain have shown the existence of a distinct parieto-temporal pathway, the middle longitudinal fasciculus (MdLF), that interconnects the superior temporal and inferior parietal cortices (Seltzer and Pandya, 1984, 1994; Schmahmann et al., 2007), and long fibers that pass through the extreme capsule (EmC) to link posterior superior temporal regions with the IFG (Petrides and Pandya, 1988, 2009). These connections have also been characterized in the human brain using in vivo imaging techniques (Frey et al., 2008; Makris and Pandya, 2009; Makris et al., 2009). The inferior occipito-frontal fasciculus, a major pathway extending from frontal cortex to temporal, parietal, and occipital cortices, has been implicated in semantic processing by subcortical stimulation data from neurosurgical patients (Duffau et al., 2005, 2008). It has also been proposed, on anatomical grounds, that semantic processing might be subserved by a two-step pathway involving the inferior longitudinal fasciculus (ILF), which runs along the whole length of the temporal lobe, and the uncinate fasciculus (UF), which connects the temporal pole with the inferior frontal regions (Vigneau et al., 2006). It is important to examine these new findings in relation to the lesion anatomy in aphasia in order to appreciate the functional contributions of these pathways.
Here, we investigated the structural and functional connectivity of the brain regions found to be critical for auditory sentence comprehension in an earlier investigation from our laboratory (Dronkers et al., 2004). Sentence comprehension is a complex function that engages many components of the language network and other brain regions that support language, such as working memory and cognitive control systems (Friederici, 2002; Dronkers et al., 2004). Thus, sentence comprehension is an ideal task for exploring the language comprehension network. In our previous investigation, auditory sentence comprehension deficits were analyzed in relation to lesion anatomy in 64 chronic stage aphasic patients who had suffered focal left hemisphere damage due to stroke. Sentence comprehension was assessed by the Curtiss-Yamada Comprehensive Language Evaluation, Receptive Language Test (CYCLE-R; Curtiss and Yamada, 1988). Lesions that significantly alter sentence comprehension performance were mapped on a standard template using voxel-based lesion-symptom mapping (VLSM, Bates et al., 2003). Subgroups of patients whose lesions encompassed each of these regions were also examined separately, using their scores on each CYCLE-R subtest, and their performance on the Western Aphasia Battery (WAB; Kertesz, 1982). Five brain regions were highlighted in this analysis as being critical for language comprehension: the MTG and underlying WM (hereafter referred to as “MTG”), the anterior superior temporal gyrus (“anterior STG/BA22”), a region including parts of the posterior superior temporal sulcus (STS) and the angular gyrus (“STS/BA39”), Brodmann’s area 47 in pars orbitalis of the IFG (“BA47”), and a part of Brodmann’s area 46 in the middle frontal gyrus (“BA46”; Figures 1,2).
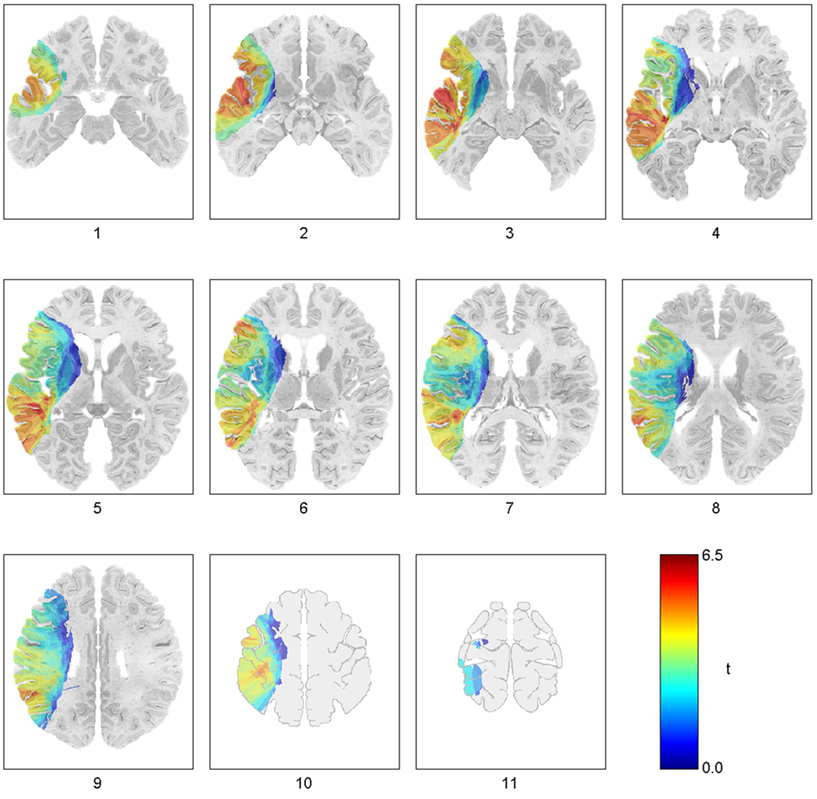
Figure 1. Voxel-based lesion-symptom mapping findings for the regions critical for auditory sentence comprehension, as assessed by the CYCLE-R. The t-map is visualized on 11 slices from the DeArmond et al. (1989) brain atlas, which was used as the template for lesion reconstructions. Significant voxels from this map comprised five distinct regions, each associated with a different pattern of performance (see text). (Reprinted with permission from Dronkers et al., 2004).
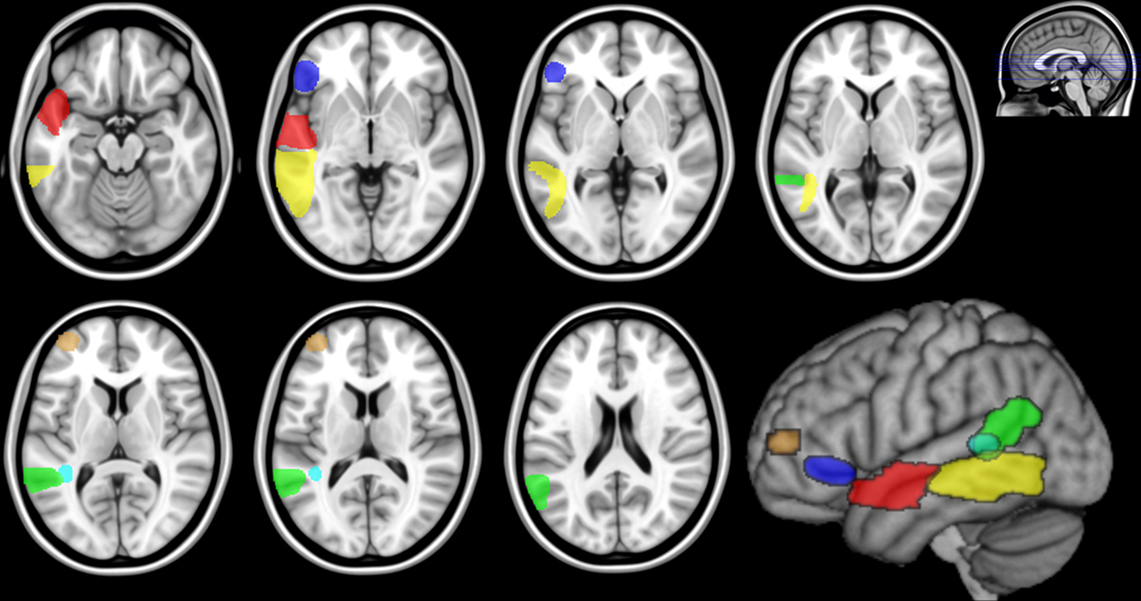
Figure 2. Regions of interest in MNI space based on the lesion-symptom mapping findings for the posterior MTG (), anterior STG/BA22 (
), BA47 (
), BA46 (
), STS/BA39 (
), and white matter underlying the STS (
). Slice positions are marked on a mid-sagittal view of the MNI template on the upper right side.
Patients with lesions in MTG and adjacent white matter, including those classified with a severe Wernicke’s aphasia, were impaired in all but the simplest sentences, indicating a word-level deficit, which was also confirmed by data from the WAB Auditory Comprehension subtests. Lesions in other areas affected sentence level processing as difficulty level increased (Dronkers et al., 2004,Figure 5), suggesting higher-level contributions to language comprehension. For example, the performance of patients with anterior STG/BA22 lesions declined when the syntactic structure of test items influenced the interpretation of the sentence, suggesting a role for this region in basic morphosyntactic processing. Patients with lesions in frontal regions BA46 and BA47 were impaired only for the most complex sentence structures. The BA47 finding was interpreted as reflecting the impairment of a working memory system for semantic information, whereas the BA46 involvement was attributed to deficits in general cognitive control processes. Finally, posterior STS/BA39 involvement was attributed to auditory short-term memory impairments, as patients with lesions in this region were most impaired on items that relied on auditory rehearsal. Patients whose lesions spared all of these regions showed only minor deficits on the most difficult items. Critically, lesions in the two regions which have traditionally been considered to be at the core of language functioning in the brain, Broca’s area (IFG pars opercularis and pars triangularis) and posterior superior temporal gyrus, the cortical zone commonly attributed to Wernicke’s area, were not found to be associated with significant language comprehension deficits in this investigation. A small WM region medial to the posterior STS was also identified as being critical for sentence comprehension, suggesting that not only cortical damage but a disconnection produced by a lesion in WM adjacent to posterior STG and MTG might also produce language comprehension deficits.
In order to investigate how the above brain regions might participate in a larger network of interconnected areas, we analyzed brain imaging data from healthy subjects using two new magnetic resonance imaging (MRI) techniques. The first, diffusion tensor imaging (DT-MRI), examines the fiber pathways that structurally link brain regions to each other (Basser et al., 1994; Pierpaoli et al., 1996). We used this technique to determine which pathways were associated with the regions found to be critical for auditory sentence comprehension from our previous lesion analysis. The second technique, resting-state functional MRI (RS-fMRI), reveals functional brain networks by correlating blood oxygenation levels across brain regions over time to identify which parts of the brain are simultaneously active (Lowe et al., 1998; Greicius et al., 2003; Biswal et al., 2010; Van Dijk et al., 2010). We applied the second method to identify brain areas that were functionally connected with each of the regions critical for auditory sentence comprehension. We used these two approaches, described below, with data from healthy subjects to examine the structural and functional architecture of the brain regions associated with sentence comprehension deficits as previously found in aphasic patients.
Diffusion tensor magnetic resonance imaging shows the microstructural organization of white matter in the living brain. With this information, it is possible to perform tractography analysis and determine the trajectories of long-distance pathways by tracing the dominant orientation of fiber groups at different points in brain white matter (Conturo et al., 1999; Basser et al., 2000; Catani et al., 2002; Mori et al., 2002). DT-MRI tractography has been used to examine the anatomical organization of the pathways that support language in the brain, to determine which pathways interconnect specific brain regions, and to interpret the functions subserved by individual pathways based on their cortical termination patterns (Catani et al., 2005, 2007; Croxson et al., 2005; Makris et al., 2005, 2009; Parker et al., 2005; Anwander et al., 2007; Vernooij et al., 2007; Frey et al., 2008; Glasser and Rilling, 2008; Saur et al., 2008; Makris and Pandya, 2009; Kaplan et al., 2010; Brauer et al., 2010). In this investigation, we used the regions of interest (ROIs) from the lesion findings which were described earlier to constrain whole-brain streamline tractography results, in order to isolate the fiber pathways associated with the WM contained in each ROI. We then identified the major WM structures associated with these fibers using digital WM atlases (Wakana et al., 2004; Burgel et al., 2006; Catani and Thiebaut de Schotten, 2008; Mori et al., 2008).
The analysis of interregional correlations in RS-fMRI data is another technique for mapping the connectivity profiles of individual brain regions as well as complete functional circuits (Biswal et al., 1995, 2010; Lowe et al., 1998; Greicius et al., 2003; Damoiseaux et al., 2006; Buckner et al., 2009; Van Dijk et al., 2010). Correlated fluctuations of spontaneous brain activity, as assessed by the blood oxygenation-level dependent (BOLD) functional magnetic resonance imaging (fMRI) signal, provide information about which regions are physiologically coupled, either via direct structural connections, or indirect polysynaptic links. Thus, high correlations between the amount of oxygen consumed simultaneously by multiple regions are believed to mirror which brain areas typically interact with each other. Several investigations have shown that the functional organization of large-scale brain networks can be assessed by mapping with this approach the regions that show coherent resting-state activity (Fox et al., 2005; Damoiseaux et al., 2006; Dosenbach et al., 2007; He et al., 2007; Vincent et al., 2007; Buckner et al., 2009; Biswal et al., 2010), including those that may be related to language (other than comprehension; Hampson et al., 2002; Kelly et al., 2010; Koyama et al., 2010; Xiang et al., 2010). In order to examine the language comprehension network, we used the cortical ROIs derived from the previous lesion analysis findings and computed whole-brain correlation maps for each ROI.
By combining the information from these two connectivity analyses, we sought to determine the relationships among the brain regions identified as being important for auditory sentence comprehension in our previous lesion analysis with aphasic patients (Dronkers et al., 2004). This was achieved by identifying in the healthy brain (1) the major pathways associated with these regions, and (2) the functional connectivity of these regions with other cortical areas.
In this way we explored how these regions might fit into a network that supports language comprehension. We also examined the implications of our findings in the context of the lesion anatomy of comprehension deficits in aphasia.
Materials and Methods
Structural and functional connectivity patterns of the regions that were found to be critical for language comprehension in our brain lesion analysis were investigated using diffusion tensor imaging and fMRI data. Streamline tractography was applied to the DTI data from 25 healthy subjects to trace the principal WM pathways associated with each of the regions. The functional connectivity profile for each region was assessed using resting-state fMRI data from another group of 25 healthy subjects. These data processing and analysis steps are described in detail in the following sections.
Definition of Regions of Interest for the Language Comprehension Network Analysis
The regions identified by the VLSM analysis of sentence comprehension deficits (Figure 1; Dronkers et al., 2004) were transformed from the DeArmond et al. (1989) atlas space, in which the original results were produced, to the Montreal Neurological Institute (MNI) space. A mapping from the DeArmond et al. atlas space to the MNI space was determined by the following steps. The MNI anatomical template was aligned to the atlas plates by a 7° rotation of the axial plane. Eleven slices were identified on the re-oriented MNI template that best match the axial photographic images of the brain in the DeArmond et al. atlas. The corresponding anatomical landmarks were marked by manually selecting several control points on each slice and used for 2D non-linear warping of the DeArmond et al. slices to MNI template slices using the Image Processing Toolbox for MATLAB1. The DeArmond et al. atlas slices were then realigned to the MNI space orientation and linearly interpolated to obtain a 2-mm inter-slice distance. A software implementation of this atlas transformation is included as part of the VLSM 1.6 package for MATLAB2.
Region of interests in MNI space were then obtained from the VLSM results for the lesion–symptom analysis in DeArmond et al. (1989) atlas space (Figure 1; Dronkers et al., 2004, Figures 3 and 4) using the same procedure. A smoothing filter (8 mm FWHM Gaussian) was applied to each ROI in order to compensate for registration errors and the residual anatomical variability that remains after the spatial normalization of the healthy subject datasets to MNI space. These ROIs for the MTG, anterior STG/BA22, BA47, BA46, STS/BA39, and the WM subjacent to the STS were saved as binary mask images for the subsequent analyses (Figure 2). It should be noted that the VLSM findings include WM regions as well as the cortical areas listed in Table 3 of Dronkers et al. (2004). Tractography analysis of structural connectivity revealed the WM pathways associated with each ROI, while the functional connectivity analysis was restricted to the cortical gray matter (GM) contained within each ROI.
Assessment of Structural Connectivity with Diffusion Imaging Tractography
Diffusion tensor imaging data
Diffusion tensor imaging datasets from 25 healthy subjects were randomly selected from the publicly-available ICBM DTI-81 database3,4, which includes diffusion imaging data from young and middle-aged healthy right-handed subjects (Oishi et al., 2008). The subject demographics, data acquisition protocols and the probabilistic maps of major pathways developed using these data have been described in publications by the ICBM researchers (Hua et al., 2009; Mori et al., 2008; Oishi et al., 2008; Zhang et al., 2008). The diffusion imaging data were acquired on Siemens 1.5T MRI scanners at the MNI and University of California Los Angeles using single-shot echo-planar imaging (EPI) sequences with sensitivity encoding and a parallel imaging factor of 2.0, 96 × 96 imaging matrix, field of view (FOV) = 240 mm × 240 mm, 60 transverse sections (2.5 mm slice thickness) parallel to the anterior commissure–posterior commissure line (AC–PC), two repetitions of a sequence of five images with minimal diffusion weighting and 30 diffusion-weighted (1000 s/mm2) images with 30 gradient orientations (Mori et al., 2008).
DTI post-processing
First, an anatomical reference image was produced from the EPI images with minimal diffusion weighting (B0 images) by correcting for head motion by rigid-body alignment in SPM8, and averaging over the realigned scans. All diffusion-weighted images were co-registered to this reference image. Using SPM8, a brain mask was derived from the reference image by applying a tissue segmentation algorithm and then applied to the other scans to exclude non-brain tissue. The reference image was also normalized to MNI space for the ROI-based analyses. The diffusion datasets were resampled to 2.0 mm3 resolution and averaged over the repeated series to improve signal-to-noise ratio. Using the methods implemented in the MedInria software package5 (Fillard et al., 2007), the diffusion tensor was computed (Basser, 1995), and streamline tractography was applied to derive fiber pathways following the principal diffusion direction at each voxel. The default settings in MedInria were used for this analysis. The tractography results were then imported into the TrackVis package6 (Wang et al., 2007) for visualization and the isolation of WM tracts for the structural connectivity analyses.
The ROIs described in the preceding section were transformed to each subject’s native dataset space for isolating the tractography-defined fibers associated with each ROI. For this purpose, the deformation fields produced by the normalization of the reference (mean B0) image were inverted using the Deformations utility in SPM8, and the resulting reverse mapping was applied to each ROI defined in MNI space to identify the corresponding regions in each subject’s brain. The fiber groups intersecting these reverse-normalized ROIs were isolated and manually segmented into fiber bundles corresponding to known anatomy and published diffusion imaging atlases (Wakana et al., 2004; Burgel et al., 2006; Catani and Thiebaut de Schotten, 2008; Hua et al., 2009; Lawes et al., 2008; Mori et al., 2008; Oishi et al., 2008). For this purpose, additional ROIs were used to delineate several major pathways, consistent with the published guidelines for identifying and isolating fiber bundles using the multiple-ROI approach (Wakana et al., 2007; Catani and Thiebaut de Schotten, 2008; Hua et al., 2008). These additional ROIs for isolating individual pathways were first drawn in MNI space on a color-coded principal diffusion direction map derived from the ICBM DTI-81 group-averaged tensor map. They were then reverse-normalized to native space datasets for tract identification. Custom MATLAB code was used to extract the fiber groups associated with the ROIs. The extracted bundles were compared against the ICBM DTI-81 probabilistic maps and tractography publications to verify their identity. The two authors independently completed this process. Fiber tract findings are reported in the results section if at least 10 tractography streamlines were found in at least 20 (80%) of the 25 subjects.
Assessment of Functional Connectivity with Resting-State fMRI Data
Functional magnetic resonance imaging
Resting-state fMRI data from 25 healthy subjects were acquired from a publicly available database of functional and structural imaging data7 (Biswal et al., 2010). We chose to use the NYU Test Retest Reliability dataset from the 1000 Functional Connectomes Project database, as the subjects in this study were scanned on three different dates and the stability of the functional connectivity patterns have been demonstrated (Shehzad et al., 2009). The image acquisition protocol (NYU Test Retest Reliability dataset, as described in previous publications that presented findings from these data (Margulies et al., 2009; Shehzad et al., 2009; Kelly et al., 2010), was: EPI on a Siemens Allegra 3.0 Tesla scanner with repetition time (TR) = 2000 ms, echo time (TE) = 25 ms; flip angle = 90°, matrix = 64 × 64; FOV = 192 mm; voxel size = 3 mm × 3 mm × 3 mm, 39 axial slices, 197 functional volumes, and a high-resolution T1-weighted anatomical image (magnetization-prepared gradient echo sequence with 1 mm × 1 mm × 1 mm resolution). During each functional imaging session, subjects were instructed to rest with their eyes open. Signed informed consent, approved by the institutional review boards of the New York University School of Medicine and New York University, was obtained from each subject prior to participation.
fMRI data pre-processing
Functional magnetic resonance imaging datasets were pre-processed using the standard SPM88 protocol which includes slice-timing correction, correction for head movements using rigid-body realignment to the mean EPI image from each session, co-registration of EPI data to the anatomical scan for each subject, tissue segmentation and normalization of anatomical scans to standard (MNI) space with the unified segmentation and normalization algorithm implemented in SPM8 (Ashburner and Friston, 2005). The EPI datasets were transformed to standard space using the normalization parameters for anatomical scans, with a final spatial resolution of 2.5 mm3 isotropic voxels. A Gaussian smoothing filter (6.125 mm full width at half maximum) was applied to enhance the signal-to-noise ratio. Tissue segmentation of the anatomical images isolated the GM, WM, and cerebrospinal fluid (CSF) compartments, which were used in the functional connectivity analysis described below.
Resting-state functional connectivity analysis
The Functional Connectivity Toolbox9 for SPM8 was used for deriving the functional connectivity maps for each ROI. The BOLD time-series for each of the ROIs was extracted, averaging over the GM voxels (derived from the tissue segmentation of anatomical images) included in the ROI. Resting fMRI data need to be corrected for artifacts of non-neural origin associated with physiological (respiratory and cardiac) events, instrument noise, and head movements, which can induce spurious correlations into the connectivity analysis. Confounding signals were estimated from the WM and CSF segments using the CompCor method (Behzadi et al., 2007). These signals together with movement-related covariates were removed from the fMRI data using regression. Next, the residual BOLD time-series were band-pass filtered over a low-frequency window of interest (0.008 Hz < f < 0.08 Hz). Correlation maps for each ROI in each session and each subject were then produced by computing the Pearson’s correlation coefficients between the ROI time-series and the time courses for all brain voxels. The correlation maps for all subjects were converted to normally-distributed scores using Fischer’s transform and submitted to one-sample t-tests to compute the group-level maps of functional connectivity for each ROI. Only positive correlations were mapped for this investigation. The resultant statistical parametric maps after testing for statistical significance using a voxel-wise threshold of p < 0.01, corrected for multiple comparisons (family-wise error rate across all brain voxels), and a cluster extent threshold of 100 mm3 (40 contiguous voxels). Only the cortical extents of these maps are reported in the present manuscript. The anatomical distributions for the functional connectivity maps were assessed in relation to digital atlases for cortical areas and gyral anatomy (Harvard–Oxford probabilistic atlas, Desikan et al., 2006; AAL atlas, Tzourio-Mazoyer et al., 2002), distributed with the FSL10 and MRICron11 software packages.
In addition to the whole-brain resting-state functional connectivity maps for the five cortical ROIs, the pair-wise interregional correlations between these regions were also examined. The mean BOLD time-series for all the GM voxels contained in each ROI was obtained, after performing band-pass filtering and artifact correction as described above. Pearson’s correlation coefficients were computed for each pair of ROIs in each subject, and analyzed across subjects with one-sample t-tests (p < 0.01, corrected for multiple comparisons).
Results
In the following sections, the structural and functional connectivity patterns associated with each of the five ROIs are reported, followed by a summary of the findings. The structural connectivity findings are illustrated in figures for two representative subjects, showing the distinct fiber pathways associated with each ROI. Each pathway was reliably traced in at least 80% (20 out of 25) of the DTI datasets. Functional connectivity maps have been rendered on a semi-inflated view of the single-subject cortical surface reconstruction provided in SPM8.
Posterior Middle Temporal Gyrus (MTG ROI)
Structural connectivity
Streamline tractography revealed that the white matter contained within the MTG ROI contains fibers from several major pathways, which were consistently identified in at least 80% of subjects (Figure 3). These pathways were: the direct (temporo-frontal) and indirect (temporo-parietal) segments of the AF; the IOFF; the MdLF; the ILF; and posterior corpus callosum fibers, consistent with the temporo-temporal interhemispheric connections through the tapetum.
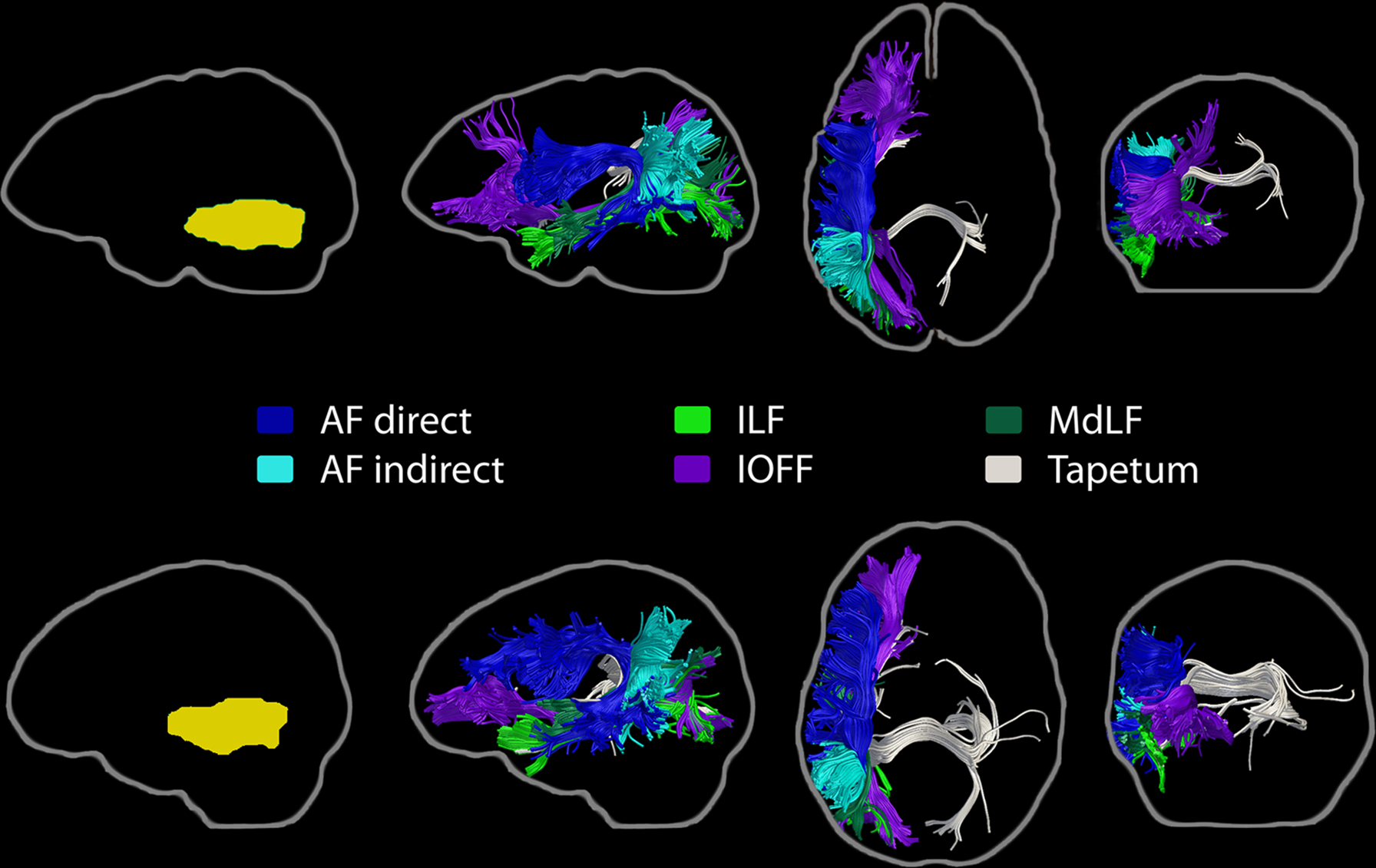
Figure 3. Major pathways associated with the left MTG region of interest. Streamline tractography results from two subjects are presented as exemplars. Each row depicts the individual subject’s ROI warped to their own native space (left, yellow), followed by sagittal, axial, and coronal perspectives of the fiber bundles involved. The direct and indirect segments of the arcuate fasciculus, the inferior occipito-frontal fasciculus, the middle longitudinal fasciculus, the inferior longitudinal fasciculus, and transcallosal projections, consistent with the tapetum, are shown.
As for the fiber bundles linking the MTG ROI with the other ROIs (Figure 4), those passing through the MTG ROI and reaching the BA47 ROI were identified as being part of the IOFF. Short fibers connecting the MTG with the STS/BA39 ROI were identified as fibers of the indirect segment of the AF. Those passing adjacent to the MTG and reaching the anterior STG/BA22 ROI were recognized as MdLF fibers, based on their trajectory12. Connections with the BA46 ROI could not be consistently traced, for reasons further discussed in the section entitled Anterior Middle Frontal Gyrus (BA46 ROI).
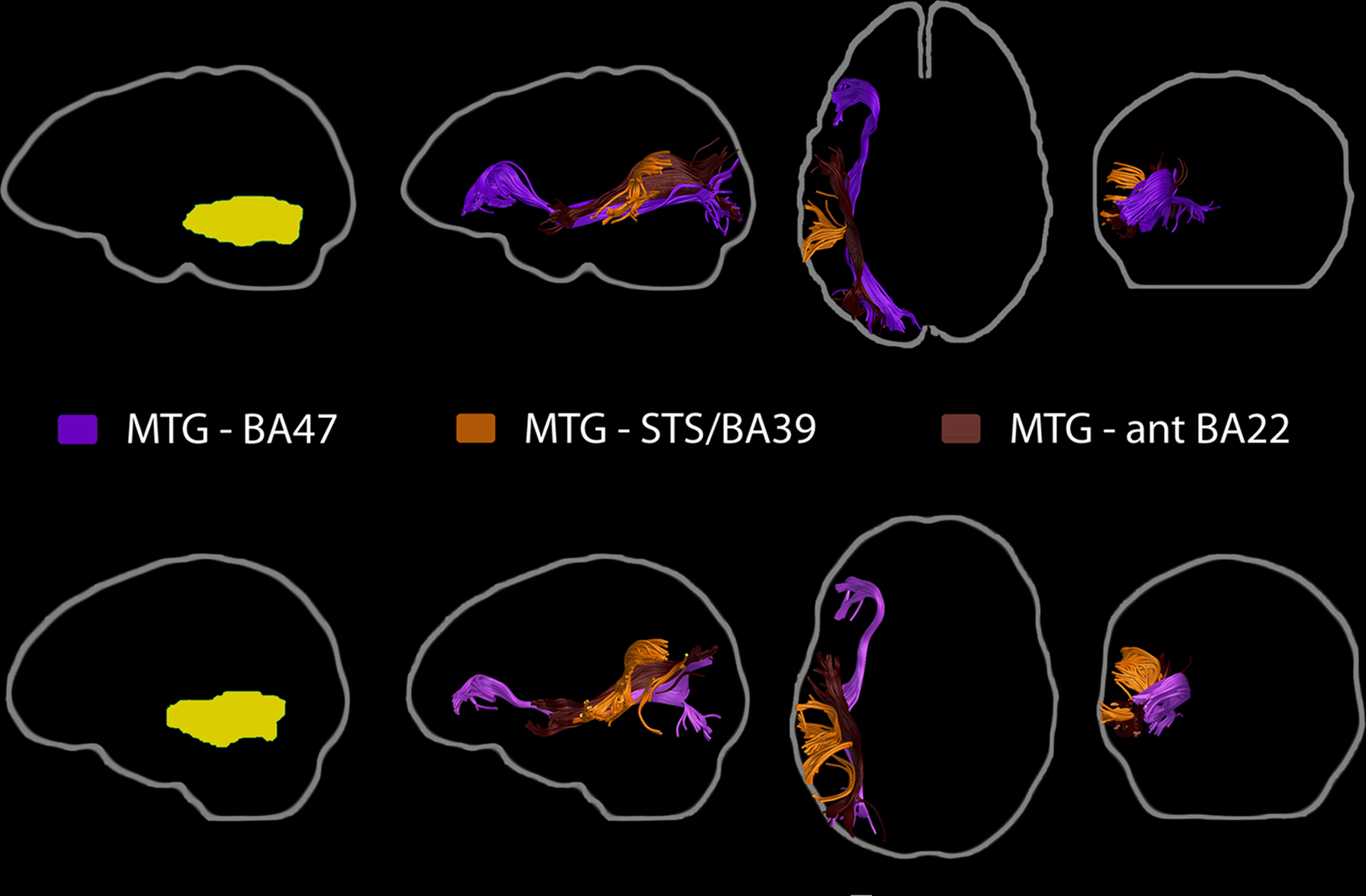
Figure 4. Pathways linking the posterior MTG region of interest with the other ROIs: BA47, STS/BA39, and anterior STG/BA22. Each row again depicts the findings for each sampled individual, with the ROI and fiber bundles depicted in the subject’s native space (left, yellow). Fibers passing between the MTG and BA47 were identified as part of the IOFF. Those connecting MTG with STS/BA39 were consistent with fibers of the indirect segment of the AF. The pathway likely to connect the MTG ROI with the anterior STG/BA22 ROI was identified as the MdLF.
Resting-state functional connectivity
The whole-brain resting functional connectivity map for the MTG ROI revealed an extensive network of cortical association areas, importantly, several peri-sylvian and neighboring regions that have been implicated in language, and their right hemisphere homologues (Figure 5). Because of the broad extent of this map, we also identified the regions that show the strongest correlations at a more stringent threshold (t > 12.13, cluster extent > 62.5 mm3), which are reported in Table 1. In the left lateral temporal lobe, we identified a zone running from the temporal pole to the temporo-parietal and temporo-occipital regions, extending into the inferior temporal cortex (BA 20 and BA 37), the STS, and parts of the STG (BA 22), but excluding the primary auditory cortex (BA 41 and 42), which was mirrored in the right temporal lobe. The parietal regions showing the strongest correlations with the MTG were the left angular gyrus (BA 39) and superior parietal lobe (BA 7). A medial posterior parietal region was also functionally connected with the MTG. Frontal lobe functional connectivity was bilateral (more extensive in the left hemisphere) and comprised three zones: a broad cortical swath running along the superior–inferior direction on the lateral prefrontal cortex, including parts of the middle frontal gyrus and the precentral gyrus dorsally, continuing through the pars opercularis (BA44) and pars triangularis (BA45) of the IFG and ending inferiorly into the pars orbitalis (BA47); a dorso-medial zone including parts of BA6, BA8, BA9, and BA 32; and the gyrus rectus (BA11) in the ventromedial frontal cortex. Regions of interest that showed functional connectivity with the MTG included STS/BA39, BA47, and parts of anterior STG/BA 22, but not BA46.
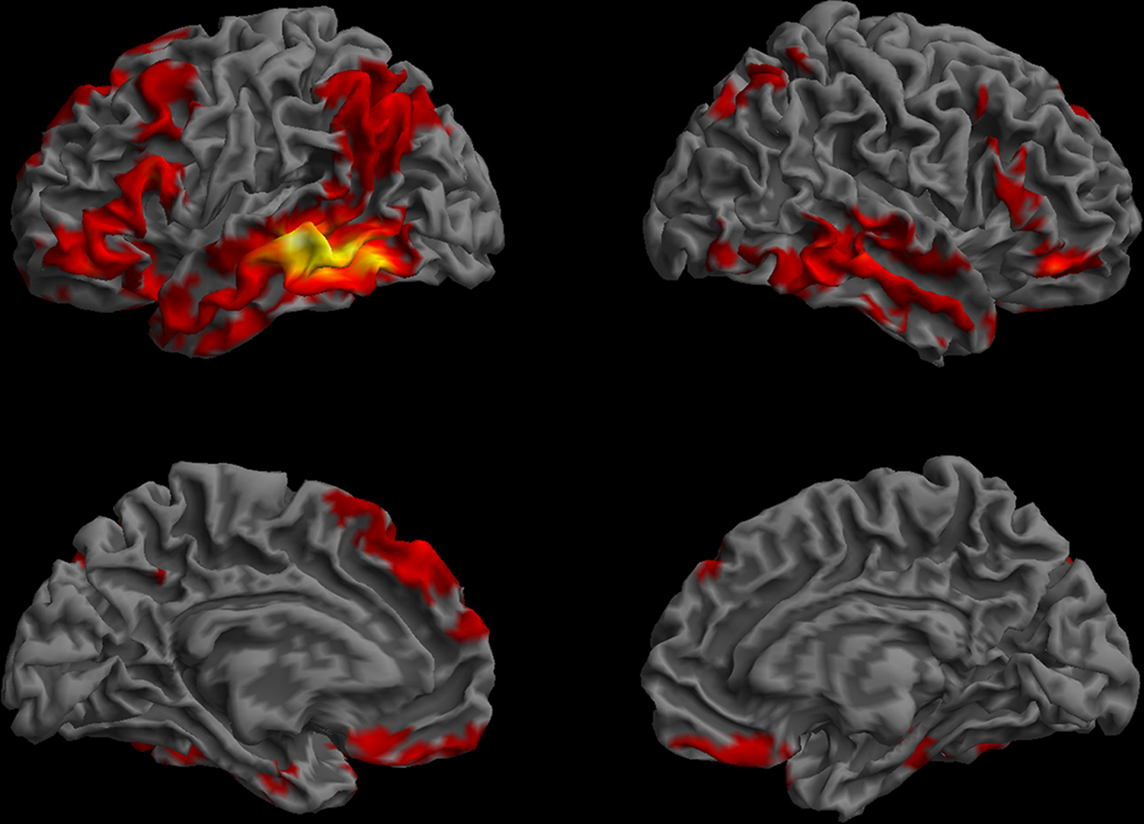
Figure 5. Functional connectivity profile of the left posterior middle temporal region that was previously found to be critical for the core processes supporting sentence comprehension (Dronkers et al., 2004). The regions that showed highly correlated (p < 0.01, corrected, cluster extent > 100 mm3) spontaneous activity with the left MTG seed are shown on a semi-inflated view of the cortical surface. The left and right hemispheres are shown on the left and right columns, respectively. The upper row shows the lateral surface, and the lower row, the medial surface of the cerebrum. Colors indicate t-values (dark red = lowest, yellow-white = highest, with the voxels within the ROI showing the highest correlation).
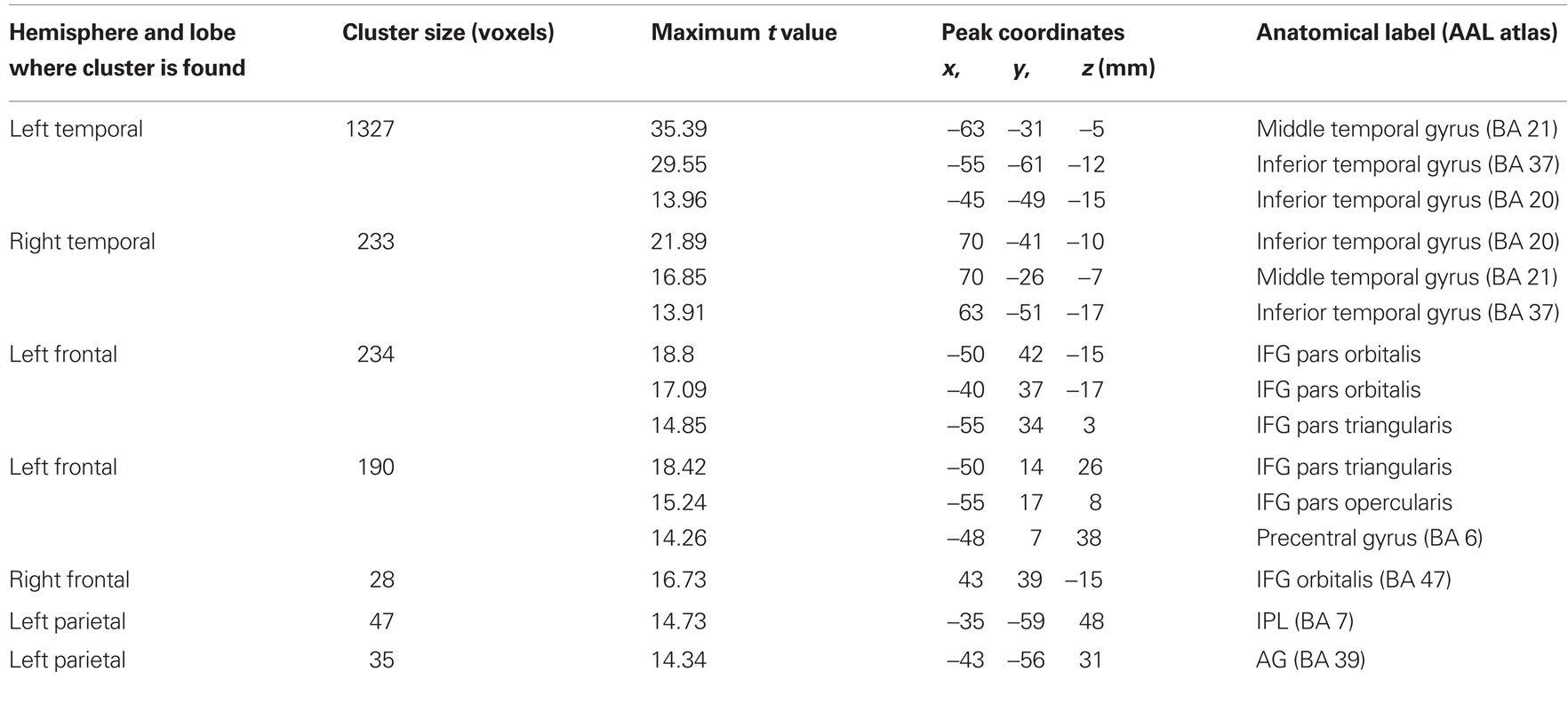
Table 1. Cortical areas found to be functionally connected to the left MTG seed region at a stringent threshold for significance (t > 12.13, cluster extent > 62.5 mm3).
Anterior Brodmann’s Area 22 in the Superior Temporal Gyrus (Anterior STG/BA22 ROI)
Structural connectivity
The middle longitudinal fasciculus and UF fibers were associated with the anterior STG/BA22 ROI in all subjects (Figure 6). Fibers passing adjacent to this ROI and the MTG ROI were identified as belonging to the MdLF. Pathways between the anterior STG/BA22 and STS/BA39, BA47, and BA46 ROIs could not be found consistently across subjects.
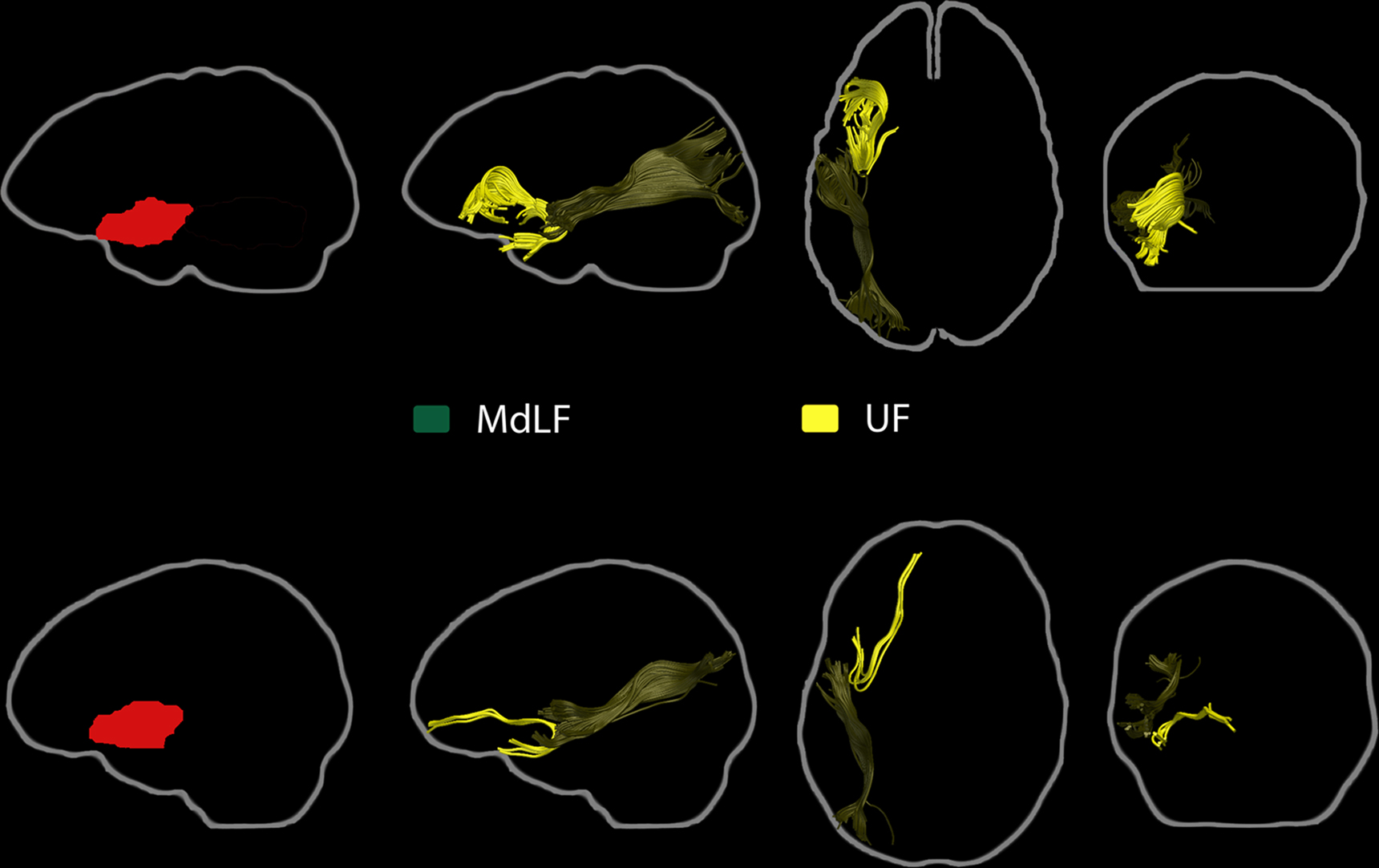
Figure 6. Pathways associated with the anterior STG/BA22 region of interest (left, red). Streamline tractography results from two subjects are presented as exemplars. The middle longitudinal fasciculus and the uncinate fasciculus were found to be associated with this ROI.
Resting-state functional connectivity
The functional connectivity map for the anterior STG/BA22 ROI included the whole extent of the superior temporal gyrus and neighboring sections of the MTG, BA 37, the temporal pole, the rolandic operculum, IFG pars orbitalis (BA 47) and the anterior cingulate cortex (BA 24), the supplementary motor area, the precentral gyrus (BA 6), and the insula (Figure 7). All of these regions were identified in both hemispheres. Other ROIs that showed functional connectivity with the anterior STG/BA22 ROI were parts of the MTG and BA47.
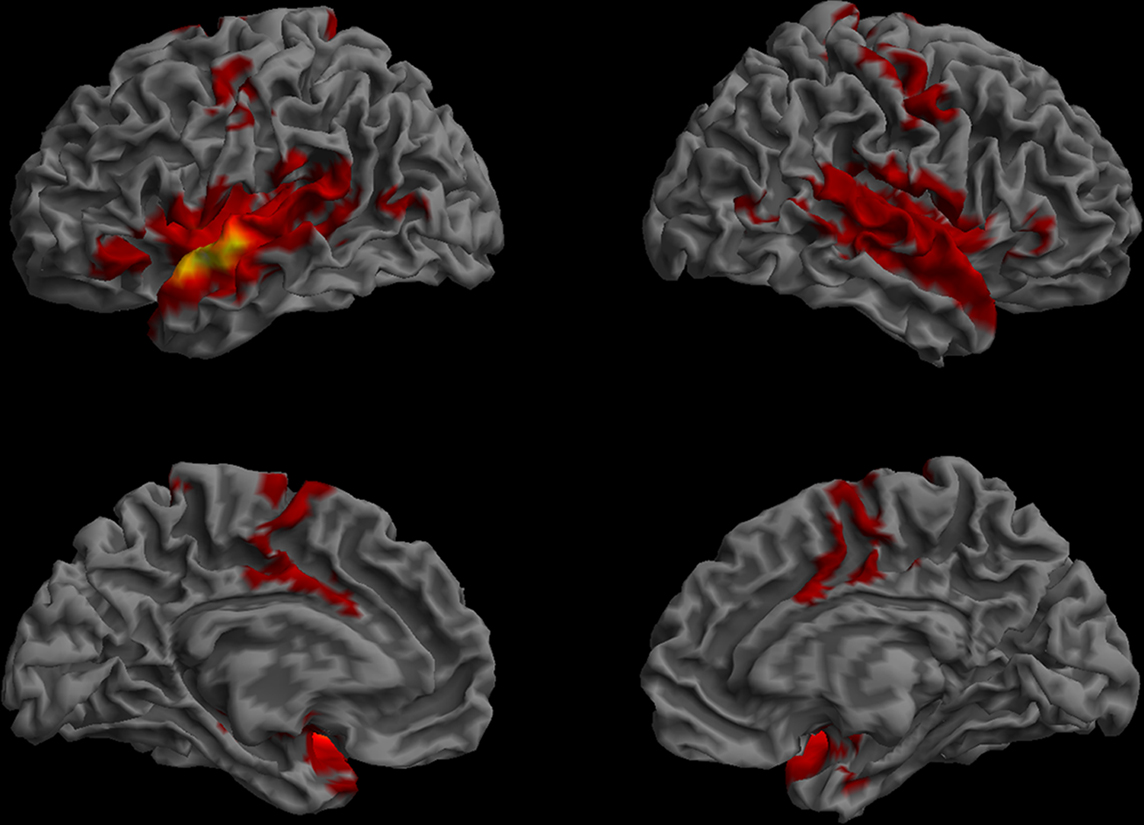
Figure 7. Resting-state functional connectivity of the left anterior STG/BA22 ROI. (Lateral and medial views of the left and right hemispheres, p < 0.01, corrected, cluster extent > 100 mm3, colors indicate t-values, dark red = lowest, yellow-white = highest, with the voxels within the ROI showing the highest correlation).
Pars Orbitalis of the Inferior Frontal Gyrus (BA47 ROI)
Structural connectivity
The BA47 ROI was consistently found to be associated with the inferior occipito-frontal fasciculus (Figure 8). In all subjects, streamlines consistent with the IOFF passed through both the BA47 and MTG ROIs.
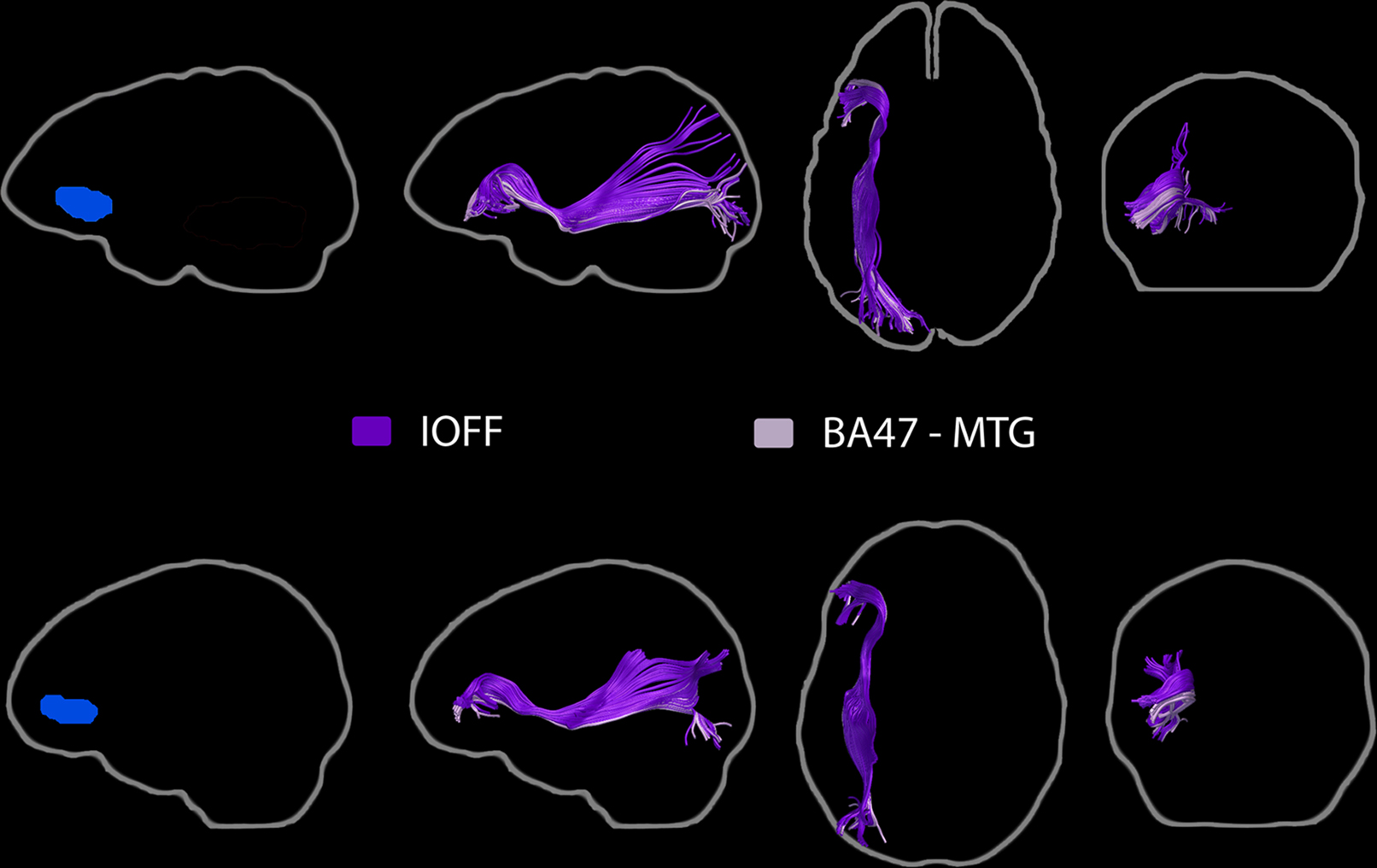
Figure 8. Pathways associated with the BA47 ROI (left, blue). Streamline tractography results from two subjects are presented as exemplars. The inferior occipito-frontal fasciculus as well as a group of IOFF fibers associated with the posterior MTG ROI were identified.
Resting-state functional connectivity
The functional connectivity map for BA47 consisted of six clusters (Figure 9). The largest cluster was centered in the IFG, extending to pars opercularis (BA 44), inferior precentral gyrus (BA 6), and the temporal pole (BA 38). Other clusters were in the MTG bilaterally, the right IFG, a left posterior region which included parts of the left angular gyrus (BA 39) and the superior temporal gyrus (BA 22, but not anterior STG/BA22), and the left medial frontal cortex (SMA, BA 6, 8, and 9).
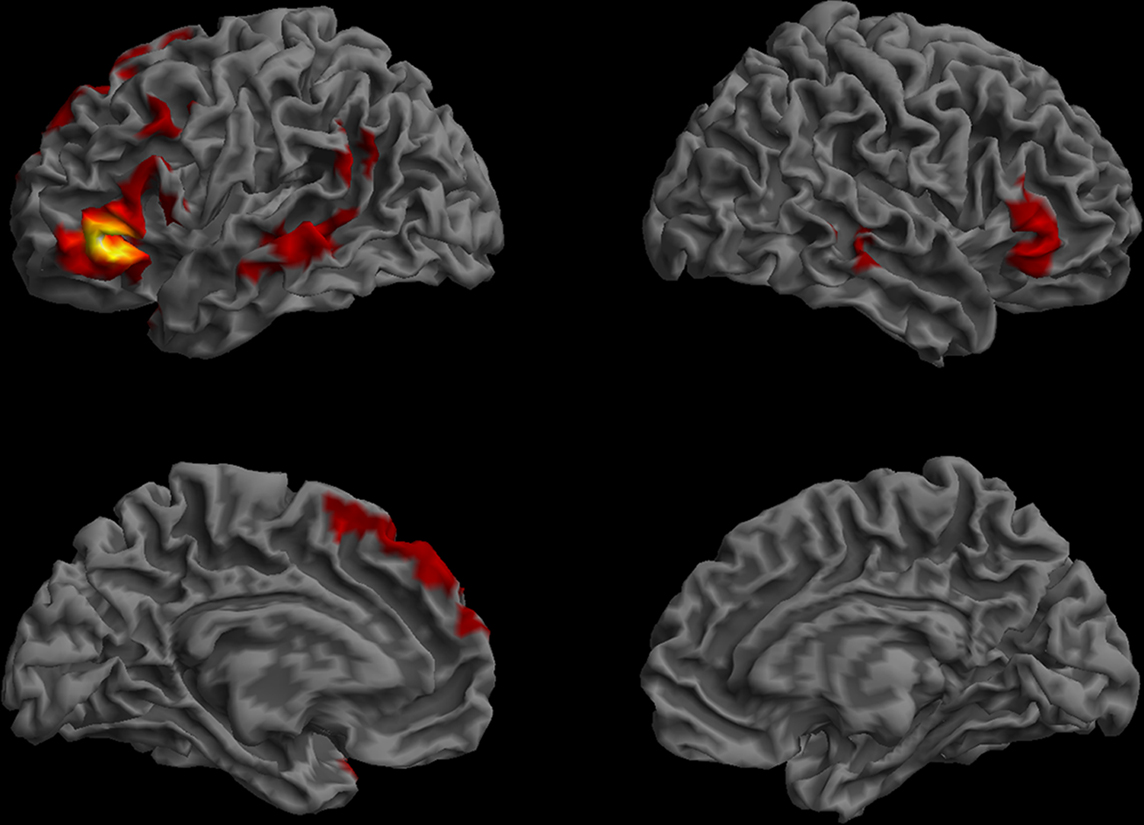
Figure 9. Resting-state functional connectivity map of the BA 47 region of interest. (Lateral and medial views of the left and right hemispheres, p < 0.01, corrected, cluster extent > 100 mm3, colors indicate t-values, dark red = lowest, yellow-white = highest, with the voxels within the ROI showing the highest correlation).
Anterior Middle Frontal Gyrus (BA46 ROI)
Structural connectivity
Tractography analysis of the BA46 ROI did not reveal a consistent pattern across subjects, possibly due to the smaller size of this ROI, intersubject anatomical variability that affected registration accuracy, and/or limitations of streamline tractography. It is important to note the BA46 ROI does not cover the entire extent of Brodmann’s area 46 and that an analysis of the entire region would undoubtedly reveal an extensive connectivity pattern.
Resting-state functional connectivity
The functional connectivity map for the BA46 ROI included bilateral middle frontal gyri, small sections of the supramarginal gyrus (SMG), and anterior cingulate cortex (BA 24, 32) as well as the left anterior insula (Figure 10).
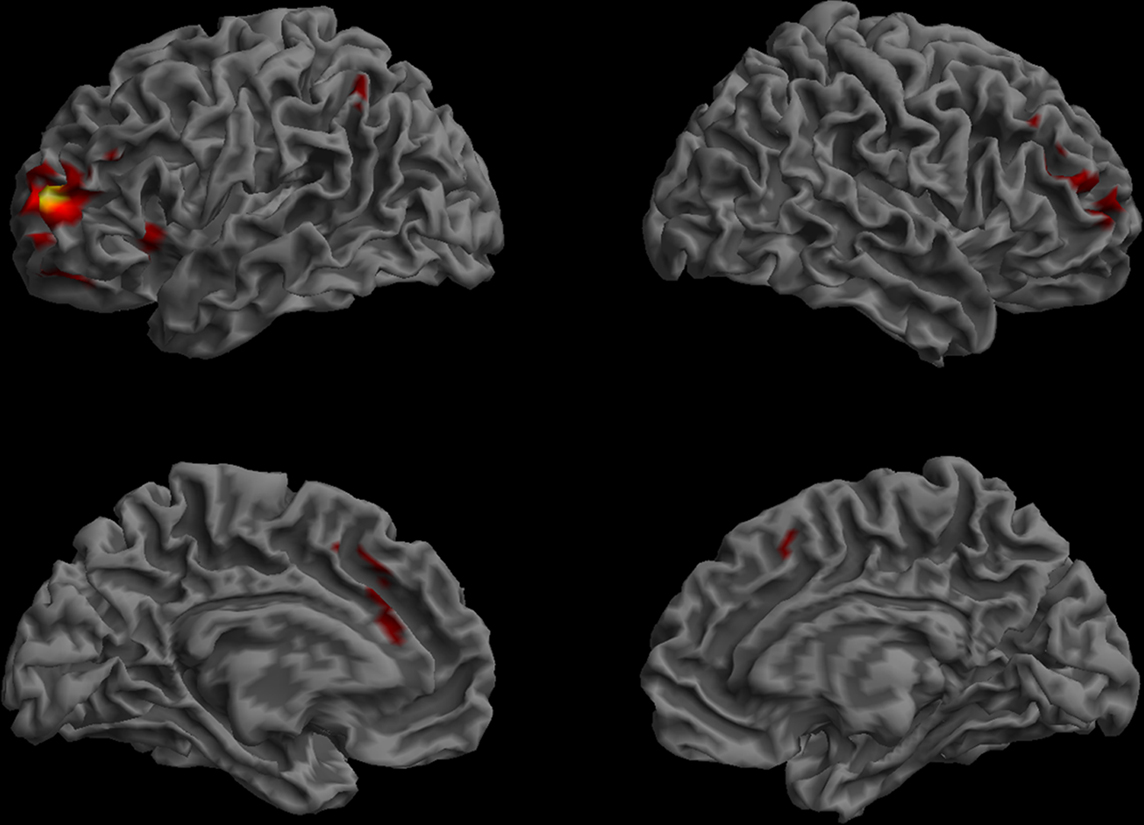
Figure 10. Functional connectivity of the BA46 ROI. (Lateral and medial views of the left and right hemispheres, p < 0.01, corrected, cluster extent > 100 mm3, colors indicate t-values, dark red = lowest, yellow-white = highest, with the voxels within the ROI showing the highest correlation).
Posterior Superior Temporal Sulcus and Brodmann’s Area 39 (STS/BA39 ROI)
Structural connectivity
Fibers associated with the direct and indirect segments of the AF were found to pass through the WM region included in the posterior STS/BA39 ROI (Figure 11). Short, vertically-oriented fibers interconnecting the MTG and STS/BA39 ROIs were also found in all subjects. Also of note was a robust bundle of short fibers connecting the STS/BA39 ROI with other parts of the angular gyrus. Fibers between STS/BA39 and BA47 or anterior STG/BA22 could not be consistently identified across subjects.
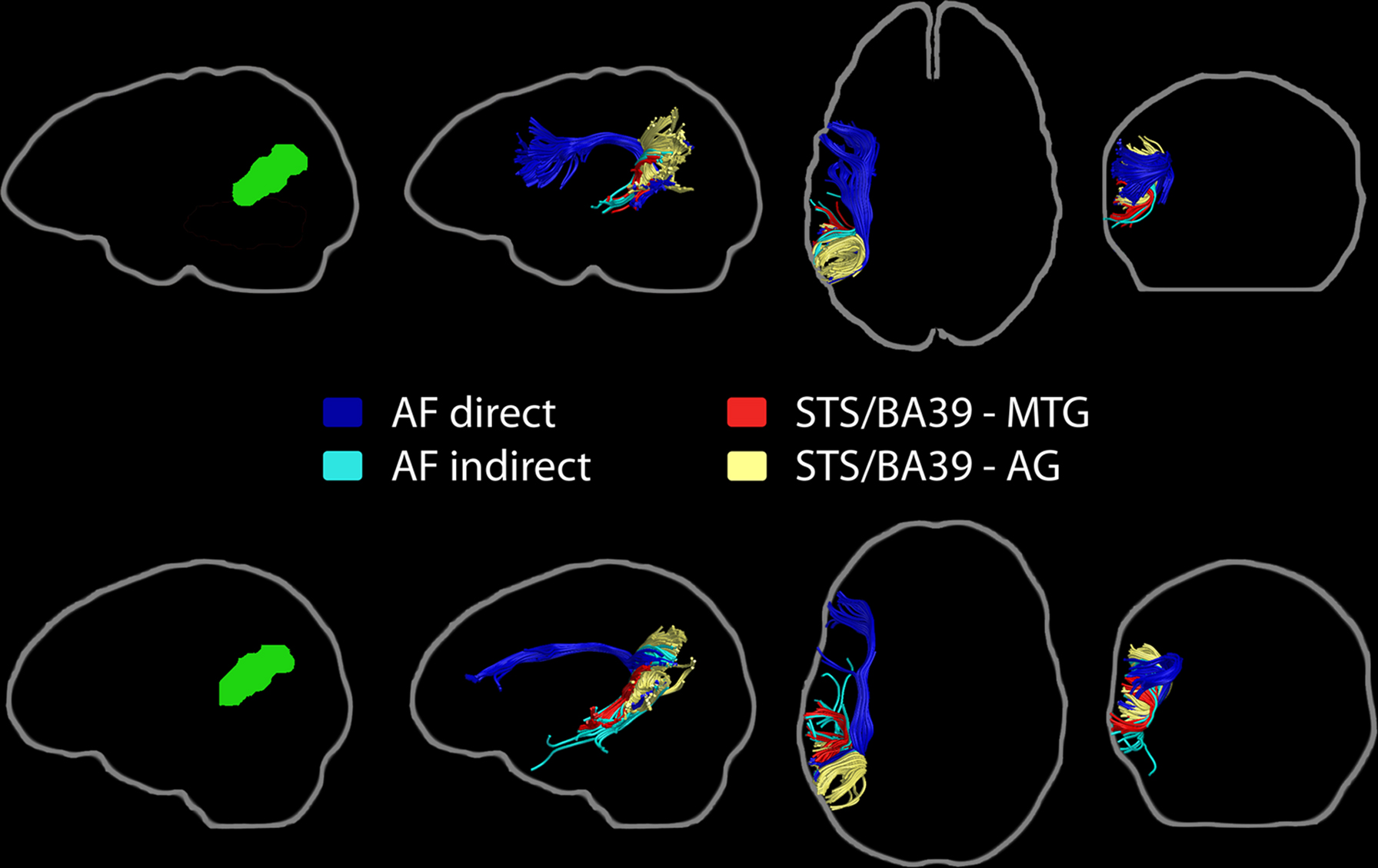
Figure 11. Pathways associated with the left STS/BA39 region of interest (left, green). Streamline tractography results from two subjects are presented as exemplars. The primary fiber pathway associated with this ROI is the arcuate fasciculus, with its direct and indirect compartments shown. Short fiber bundles connecting this ROI to the MTG ROI and the angular gyrus are also represented.
Resting-state functional connectivity
The functional connectivity pattern of the STS/BA39 ROI included two large clusters running along the MTG bilaterally, forming a bridge between the temporal pole and the angular gyrus, and extending into the superior and inferior temporal gyri (Figure 12). Other functionally-connected regions were in the left lateral prefrontal cortex, including the pars orbitalis (BA 47) and pars triangularis, the left middle frontal gyrus, bilateral dorso-medial frontal regions, right BA 47, the gyrus rectus, mid-cingulate cortex, and the medial posterior parietal cortex, bilaterally. Other ROIs that showed functional connectivity with the STS/BA39 ROI included parts of the MTG, BA47, and anterior STG/BA22 ROIs, but not the BA46 ROI.
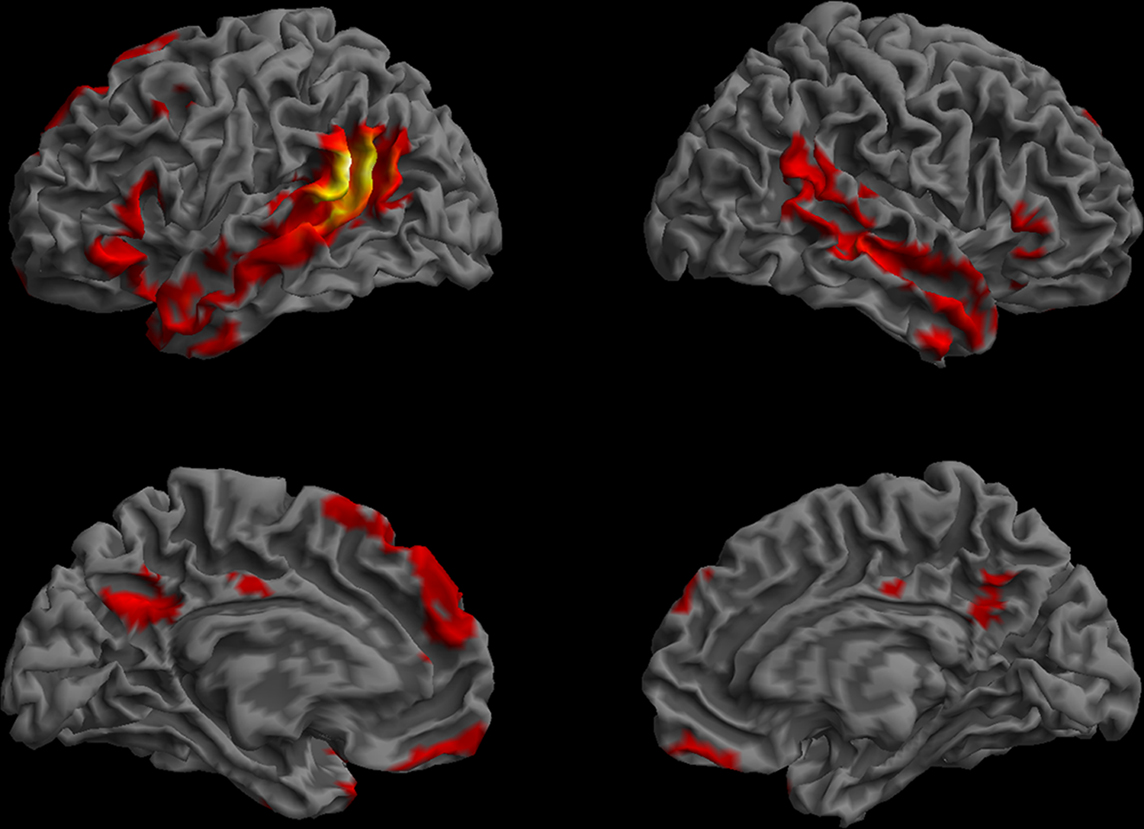
Figure 12. Resting-state functional connectivity pattern for the posterior STS/BA39 region of interest. (Lateral and medial views of the left and right hemispheres, p < 0.01, corrected, cluster extent > 100 mm3, colors indicate t-values, dark red = lowest, yellow-white = highest, with the voxels within the ROI showing the highest correlation).
White Matter Underlying the Superior Temporal Sulcus (STS WM ROI)
The fiber composition of a WM region subjacent to the STS was also analyzed. This region was reported in our lesion–symptom mapping analysis as part of the STS/BA39 ROI (Dronkers et al., 2004), but it was also noted that it could represent a distinct functional unit on its own (Dronkers et al., 2004, p. 159, Figure 4, slice 7, footnote 2). This small region was found to contain fibers associated with five fiber systems: the direct (temporo-frontal) and indirect (temporo-parietal) segments of the AF, the IOFF, the MdLF, and transcallosal fibers consistent with the tapetum (Figure 13).
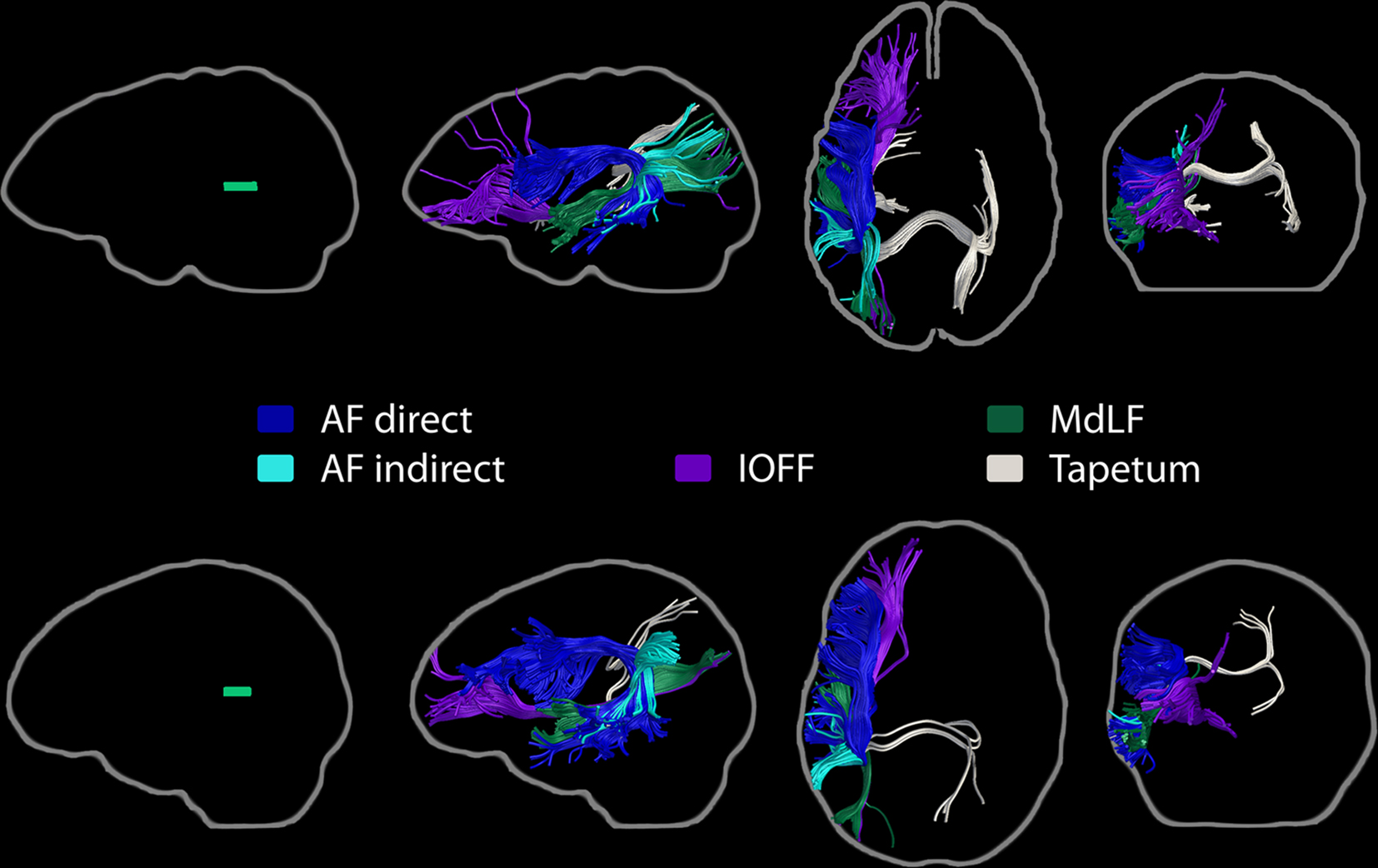
Figure 13. Fiber pathways passing through the white matter underlying the superior temporal sulcus. Five different fiber bundles were found to contribute fibers to this small white matter region (left, green). Direct and indirect segments of the arcuate fasciculus, the inferior occipito-frontal fasciculus, the middle longitudinal fasciculus, and the tapetum are shown for the two subjects chosen as exemplars.
Additional Analyses
Subdivisions of the posterior MTG
The extensive connectivity pattern of the MTG ROI was a surprising result. It is possible that ROI size, rather than location, may have contributed to this novel finding, as this ROI covers the whole extent of the MTG along the anterior–posterior axis (y = −18 to −70 mm) and also goes deeply into WM. For this reason, we subdivided the MTG ROI into subregions of equal length along the anterior–posterior axis, and analyzed their connectional anatomy patterns. Resting functional connectivity maps revealed an anterior-to-posterior gradient, with the compartments in the anterior half of the MTG showing more widely distributed functional connectivity patterns than those in the posterior half (Figure 14). The second quarter of the MTG showed a distribution that best matched the extent of the original MTG functional connectivity profile. This subregion is consistent with the main MTG termination of the ascending section of the AF, and the STS WM ROI [the subsection entitled White Matter Underlying the Superior Temporal Sulcus (STS WM ROI)] is situated superior to this section of the MTG. The IOFF, AF, MdLF, ILF and fibers of the tapetum all course through the WM underlying this segment of the MTG, so that a lesion in this region alone can affect all five fiber systems.
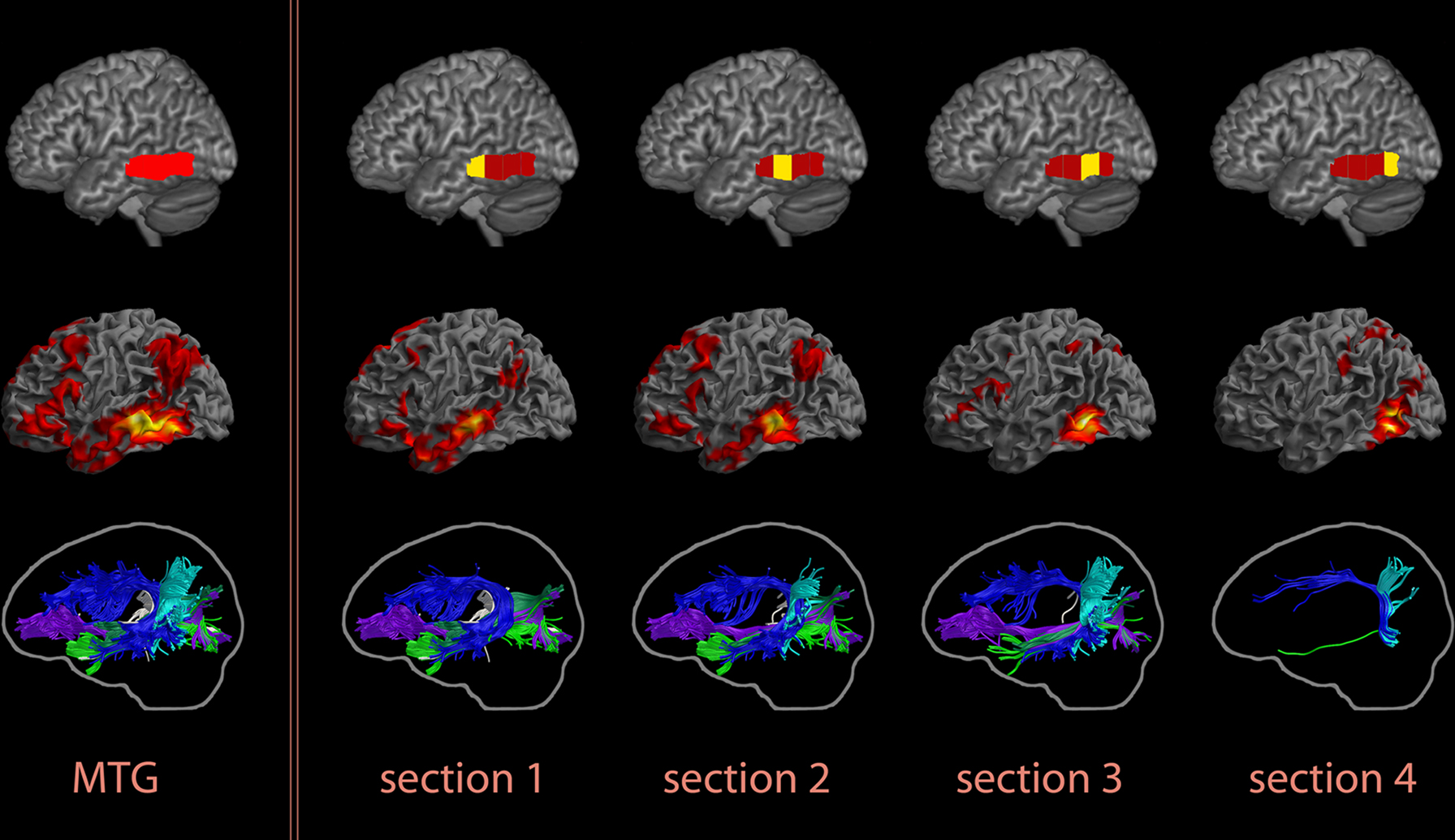
Figure 14. Resting functional and structural connectivity profiles of subregions of the posterior MTG, divided into four parts of equal length along its anterior–posterior extent (y = −18 to −70 mm). An anterior-to-posterior gradient was observed in these maps, with the two anterior subregions showing more widely-distributed functional connectivity and richer structural connectivity patterns. The individual compartments are indicated on the top row, functional connectivity maps are presented in the middle row, and tractography results for one subject chosen as an exemplar are in the bottom row. The results for the whole MTG ROI are shown in the first column, followed by the findings for the individual subregions. In the functional connectivity maps, colors indicate t-values (dark red = lowest, yellow-white = highest, with the voxels within the ROI showing the highest correlation).
“Wernicke’s area”
Although Wernicke’s area has traditionally been associated with language comprehension, our 2004 lesion–symptom mapping results did not associate this region with language comprehension impairments. To test if functional connectivity analysis could offer additional information regarding the role of this area in the language network, Harvard–Oxford atlas-based ROIs were used. The functional connectivity maps for the planum temporale and the posterior SMG – regions associated with Wernicke’s area in some models (Geschwind, 1972; Bogen and Bogen, 1976) – showed distinctly different patterns compared to our MTG map, suggesting that these regions belong to separate networks (Figure 15).
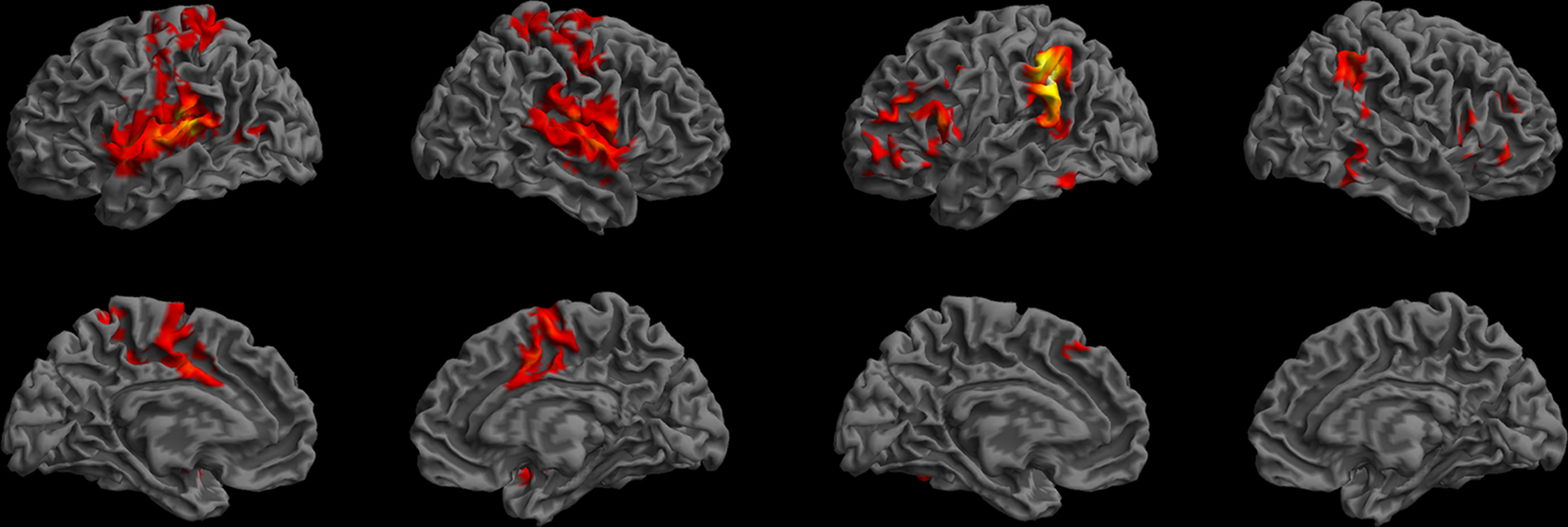
Figure 15. Resting-state functional connectivity maps for the left planum temporale (left) and the left posterior supramarginal gyrus (right) according to the Harvard–Oxford cortical atlas. These maps suggest that the network associated with the posterior MTG is distinct from the networks that include the planum temporale and the posterior SMG. Colors indicate t-values (dark red = lowest, yellow-white = highest, with the voxels within the ROI showing the highest correlation).
Structural and Functional Connections Linking the Regions of Interest: Summary of Findings
Table 2 summarizes the structural and functional connectivity patterns for the five cortical regions of interest. The MTG ROI was linked with the anterior STG/BA22 and STS/BA39 and BA47 ROIs via several major pathways, although cortical terminations could not be ascertained in each case. In addition, the MdLF fibers associated with the anterior STG/BA22 ROI were found pass through a WM region subjacent to the STS, and course medially to the STS/BA39 ROI, suggesting that these two ROIs could be linked via the MdLF. Functional connectivity analysis of the ROI-to-ROI correlations were found to be significant for all pair-wise combinations of the MTG, anterior STG/BA22 and STS/BA39 and BA47 ROIs, whereas the BA46 ROI was only correlated with the MTG ROI (p < 0.01, corrected).
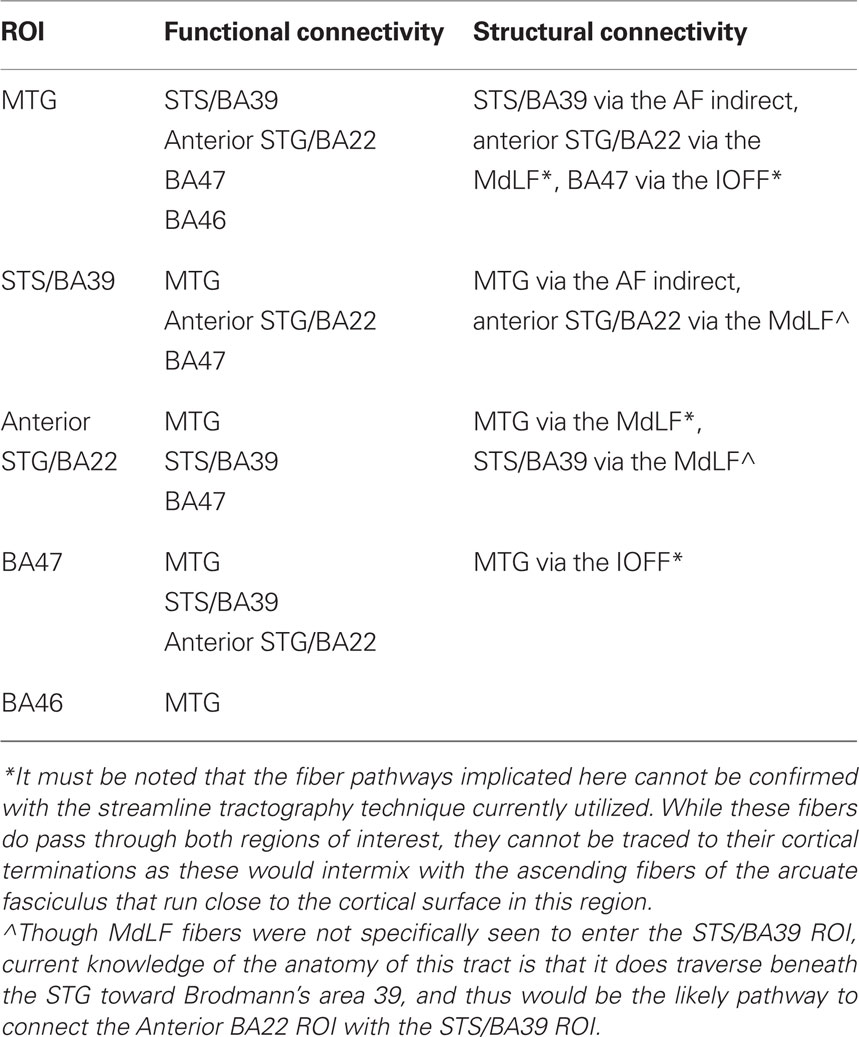
Table 2. Summary of the structural and functional connectivity findings for each region of interest in relation to the other ROIs.
Discussion
Our analysis of the structural and functional connectivity of the key regions implicated in auditory sentence comprehension by our previous work (Dronkers et al., 2004) revealed a bilateral temporo-parieto-frontal network supported by numerous WM pathways and interacting with several other brain regions outside the traditionally-recognized language areas.
The structural connectivity analyses revealed that six long distance fiber pathways were associated with these regions: the AF, the IOFF, the ILF, the MdLF, the UF, and the tapetum. Functional connectivity analyses revealed an extensive network of cortical association areas within the left hemisphere, extending to homologous regions of the right hemisphere. The extent of this network is consistent with the notion that a complex process such as understanding spoken sentences is not mediated by a single region or pathway alone, but requires the integrated functioning of a broadly-distributed constellation of regions interacting via multiple routes within and outside the language areas (Mesulam, 1998; Dronkers, 2000; Dronkers et al., 2000a; Damasio and Damasio, 2002).
One important implication of the present findings is that the left MTG holds a key position within this network for language comprehension, indicated by the richness of its structural and functional connectivity as described here. This region appears to function as a focal point with no less than five major fiber pathways passing beneath it and a broadly distributed set of cortical association areas functionally connected with it. It is not surprising that injury to this region, rich in connections, results in severe and persisting language comprehension impairments. This observation also highlights an important notion. Regions such as the MTG and its underlying white matter, where multiple fiber pathways converge, could serve as the backbone of a complex network, but such regions also become the most vulnerable part of the whole network when neurological damage occurs.
Another major finding supports the proposal (Duffau et al., 2005) that the IOFF, a pathway that is not part of the canonical language network, might play an important role in the comprehension and production of meaningful speech. We found that the IOFF fibers pass through the temporal white matter region highlighted in our lesion analysis, providing a bridge between the middle temporal region and inferior prefrontal area 47, which has been implicated in high-level language comprehension by several findings, including our lesion analysis (Bookheimer, 2002; Dronkers et al., 2004; Hagoort et al., 2004; Binder et al., 2009). Our functional connectivity findings also support a direct link between the MTG and BA47 that is mediated by the fibers that travel along the IOFF.
Finally, the AF was found to have extensive connections within the MTG, consistent with other recent findings (Powell et al., 2006; Glasser and Rilling, 2008; Rilling et al., 2008). Traditional models of language processing have previously considered this tract to connect the posterior superior temporal gyrus with inferior frontal regions. However it is important to remember that the terminations of this tract are not restricted to the superior temporal gyrus but reach the MTG as well. Thus, future models of language processing must also take into account the vast connections of this important fiber pathway including those that extend into the MTG. The present findings and their implications for understanding comprehension impairments in aphasia are discussed in more detail in the following sections.
The Extended Network for Language Comprehension
The present findings indicate the involvement of a large-scale network in language comprehension, including the left MTG, anterior STG/BA22, BA47, and STS/BA39, which had been identified in our earlier lesion analysis findings (Dronkers et al., 2004). Several long-distance white matter pathways are associated with the lesion-symptom mapping findings: the direct and indirect segments of the AF, the IOFF, the ILF, the MdLF, the UF, and the tapetum. The left MTG, with the most extensive structural and functional connectivity profile, emerged as a central component of the language comprehension network. Of the six major pathways implicated in the present study, only the UF was not associated with the MTG. Regions of functional connectivity for the MTG included large territories in temporal, parietal, and frontal cortices in the two hemispheres. The anterior STG/BA22 ROI included fibers from the MdLF, consistent with its functional connectivity pattern extending posteriorly along the superior temporal gyrus toward the supramarginal and angular gyri, as well as fibers of the UF, which link anterior sections of the temporal lobe with the IFG. However, direct pathways linking this region with the MTG, STS/BA39, and BA47 could not be consistently identified. The STS/BA39 ROI was associated with fibers from the AF and had a functional connectivity pattern that bridged the middle temporal and the angular gyri. The structural and functional connectivity findings for the BA46 ROI did not link this area with the other regions of interest, which could be due to the technical limitations of the small ROI size and high inter-individual anatomical variability in this location. BA47 was found to be part of a lateral and dorso-medial prefrontal network, as indicated by its functional connectivity pattern, and was structurally connected to posterior brain regions via the IOFF.
The overall pattern is remarkably consistent with a recent characterization of the functional anatomy of the verbal semantic system based on a meta-analysis of 120 functional neuroimaging investigations (Binder et al., 2009). The congruence between the two sets of findings, as seen in Figure 16, provides further support for the notion that language comprehension is subserved by an extensive network of regions distributed throughout the left hemisphere.
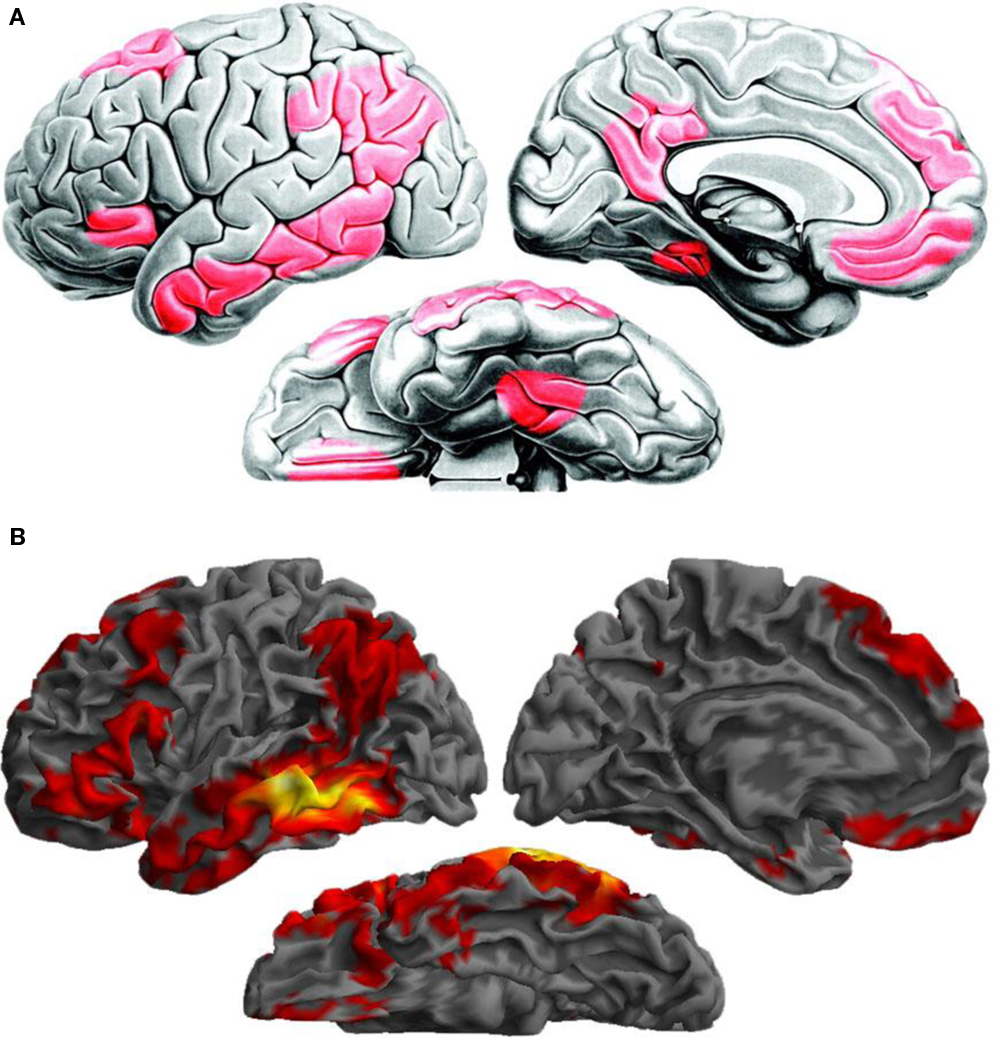
Figure 16. (A) A large-scale network for verbal semantic processing identified by a meta-analysis of 120 functional neuroimaging studies, and the underlying structural connections inferred from tracing studies of the homologous regions in the macaque from (Reprinted with permission from Binder et al., 2009, page 2779, Figure 7). (B) Resting functional connectivity pattern for the left posterior MTG ROI, assessed in the present investigation, is largely consistent with the meta-analysis findings.
Structural and Functional Connectivity of the Left Middle Temporal Gyrus: Implications for Understanding the Role of the MTG in Language and of MTG Lesions in Aphasia
The left MTG’s rich pattern of structural and functional connectivity suggests a core function in the language comprehension network. This pattern can also help explain the severe nature of the comprehension deficits produced by lesions affecting the MTG and underlying white matter (Dronkers et al., 1995, 2004; Binder et al., 2003). We found that fibers from five major pathways are included in the white matter adjacent to the MTG. These pathways, the inferior occipito-frontal fasciculus, the AF, the inferior and middle longitudinal fasciculi, and the tapetum, mediate long-distance interactions between temporal, parietal and frontal regions and temporo-temporal interhemispheric communication. Consistent with its structural connectivity pattern, the functional connectivity profile of the MTG includes a broad network of left peri-sylvian association cortical areas known to be involved in language, homologous regions in the right hemisphere, as well as several association areas that extend beyond the classical canonical language network. This extensive functional connectivity pattern also accords with a resting-state functional connectivity mapping study of the brain regions involved in reading, which found the left MTG as a common node in a conjunction analysis of six different networks investigated (Koyama et al., 2010). A recent resting-state fMRI found that the MTG is among the most highly connected regions in the cerebral cortex (“cortical hubs”; Buckner et al., 2009). The left MTG peak reported by Buckner and colleagues (−62, −38, −12 mm, Buckner et al., 2009, Table 3) is also consistent with the MTG subdivision we have found to have the most extensive functional connectivity profile (Figure 14; second section of the MTG ROI).
It is perhaps not surprising that a region that supports core lexical-semantic processes should be highly integrated with broadly distributed brain networks. That the MTG is involved even in word-level comprehension is indicated by our previous lesion-symptom mapping analysis (Dronkers et al., 2004). Other investigations on the effects of brain lesions (Hart and Gordon, 1990; Hillis et al., 1999; Boatman et al., 2000; Bates et al., 2003) and functional neuroimaging findings with healthy individuals also support this conclusion (Binder et al., 1997, 2009; Bookheimer, 2002; Vigneau et al., 2006; Price, 2010). Binder (2003) has also reviewed the neurological evidence implicating the MTG in comprehension deficits in Wernicke’s aphasia. Mapping lexical representations to their concepts relies upon extensive links between distributed representations throughout the cerebrum, including regions that support sensory and motor experiences, such that associations can be formed between words and their meanings.
The extensive connectivity of the left MTG also suggests a role that Mesulam (1990, 1998) describes as a neural epicenter. Mesulam introduced a framework for thinking about large-scale functional networks which are widely distributed but also include core regions that serve as transmodal gateways. This concept also finds a parallel in Damasio’s convergence zones model positing that highly connected brain regions serve to bind together distributed representations (Damasio, 1989). Mesulam further predicted that lesions affecting such gateways would produce multiple disconnections, disrupting upstream, and downstream network functions. For the language network, the MTG could be such a critical neural epicenter.
A brain lesion affecting the MTG and underlying white matter would disrupt the functionally and structurally connected network that we found here in the healthy brain. For example, such a lesion could produce a disconnection between left temporal and frontal regions, as both the AF and the inferior occipito-frontal fasciculus would be affected. Communication across the length of the temporal lobe would be disrupted because of damage to the ILF. Lateral temporal and inferior parietal regions would be disconnected because of damage to the indirect segment of the AF and the MdLF. Temporo-temporal interhemispheric communication would also be affected by the loss of tapetum fibers. Thus, even a small lesion in a strategic place such as the MTG would have far more serious ramifications than a comparable lesion elsewhere in the network.
A Semantic Working Memory Pathway Via the Inferior Occipito-Frontal Fasciculus?
We found that the left MTG and BA47 were functionally connected, and that their interaction was most likely to be mediated through a direct pathway via the IOFF. It has been proposed that the larger Brodmann’s area 47, together with parts of the adjacent BA 45, might support higher-level processes in comprehension that are required only when processing demands are high (Bookheimer, 2002; Friederici, 2002; Dronkers et al., 2004). Proposals for BA 47 functions include working memory for semantic features and thematic structure (Friederici, 2002), the unification of individual semantic features into an overall representation at the multi-word level, and the controlled aspects of the retrieval, selection, and evaluation of semantic information (Thompson-Schill et al., 1997; Gabrieli et al., 1998; Poldrack et al., 1999). In our earlier lesion-symptom mapping investigation, patients whose lesions included BA47 were only impaired when presented with the most syntactically-complex sentences. This finding is consistent the view that BA 47 is engaged only when successful performance depends on the ability to keep track of several pieces of information and to resolve between the alternative interpretations of sentence components. This could be achieved by reciprocal interactions between BA 47 and the MTG, so that the appropriate lexical-semantic representations can be selected, sustained in short-term memory throughout sentence processing, and integrated into the overall context. The structural substrate for these functional interactions could be provided by a direct connection between the two regions via the IOFF.
Evidence for a direct link between BA 47 and mid-lateral temporal cortex comes from tract tracing work in the monkey brain (Petrides and Pandya, 1988, 2006; Schmahmann et al., 2007). Walker’s area 12, the monkey homolog of BA 47, was found to connect to the STS and adjacent dorsal inferotemporal cortex, the likely evolutionary precursor of the MTG (Rilling and Seligman, 2002). In our investigation, streamline tractography revealed a pathway with terminations in BA47, running toward posterior regions via the white matter underlying the MTG. This pattern was consistent with the trajectory of the inferior occipito-frontal fasciculus as identified in other diffusion imaging tractography investigations (Catani et al., 2002; Mori et al., 2002, 2008; Catani and Thiebaut de Schotten, 2008). Even though its existence has been disputed in the monkey brain (Schmahmann and Pandya, 2007), several recent post-mortem investigations have described this tract in the human brain (Ture et al., 2000; Kier et al., 2004; Burgel et al., 2006; Fernandez-Miranda et al., 2008; Lawes et al., 2008; Martino et al., 2010). While streamline tractography did not show IOFF terminations in the MTG, we did observe that IOFF fibers approached within a centimeter of the MTG cortex. It is possible that streamline tractography failed to resolve IOFF fibers entering the MTG by crossing the other major pathways in this region; high-angular resolution diffusion imaging techniques designed to trace fibers through regions with complex fiber architecture might be best suited for this task (Wedeen et al., 2008). Thus, it will be important to better characterize the relationship between the IOFF and the MTG in future investigations using new techniques.
A role for the IOFF in language has also been proposed by Duffau and colleagues (Duffau et al., 2005; Duffau, 2008) after finding that semantic paraphasias could be induced by electrical stimulation of the IOFF while patients undergoing neurosurgery performed a picture naming task. Their interpretation of this finding is that the IOFF may serve as the principal pathway for a ventral semantic system. We propose an extension of this idea. It is possible that the top-down control signals from BA 47 that modulate temporal lobe semantic selection processes are disrupted during IOFF stimulation, causing the semantic misnaming errors observed by Duffau and colleagues. Others have reported comprehension deficits in patients with thalamic infarcts that affect the temporal isthmus and adjacent peri-ventricular white matter (Naeser et al., 1982, 1987; Alexander et al., 1989). An interesting possibility, which can be tested with diffusion imaging data from patients with focal white matter lesions, is that the IOFF is affected as well in such cases, since IOFF fibers pass through the temporal isthmus, between the acoustic and optic radiations (Burgel et al., 2006).
Before reaching the frontal lobe, the IOFF passes between the insula and the putamen at the inferior level of the extreme and external capsules (Kier et al., 2004; Burgel et al., 2006; Fernandez-Miranda et al., 2008; Martino et al., 2010). Histological tracing studies in the monkey brain have revealed a pathway between the posterior superior temporal gyrus and monkey homologues of BA 44 and BA 45 in the IFG (Schmahmann and Pandya, 2006). These were later traced in the human brain with high-angular resolution diffusion imaging (Frey et al., 2008). Makris and Pandya (2009) also examined the trajectory of the frontal–posterior long association fibers through the extreme capsule in the human brain with streamline tractography, and found fibers extending to temporal, parietal, and occipital regions (Makris and Pandya, 2009, Figures 1C, 3 and 4). Saur et al. (2008, 2010) used probabilistic tractography to show a pathway through the EmC that links BA 47 and BA 45 with the most posterior extension of the MTG. These studies all describe fiber systems connecting temporal and frontal regions via an inferior pathway, which may contribute to language processing, Though they may not have been labeled as such, we believe all of the above investigations may have demonstrated fibers consistent with the IOFF, as their shape and trajectory are consistent with numerous fiber atlases and post-mortem examinations of the human brain (Ture et al., 2000; Catani et al., 2002; Mori et al., 2002, 2008; Kier et al., 2004; Burgel et al., 2006; Catani and Thiebaut de Schotten, 2008; Fernandez-Miranda et al., 2008; Lawes et al., 2008; Martino et al., 2010).
The Arcuate Fasciculus and Language Comprehension
The AF fibers that we found to be associated with the MTG are consistent with the emerging knowledge of this tract’s anatomy; diffusion imaging investigations of the human brain indicate a considerably more complex architecture than traditional language models presume. In the temporal lobe, AF fibers have been found not only in the superior temporal gyrus but in the MTG as well (Powell et al., 2006; Glasser and Rilling, 2008; Rilling et al., 2008). Temporal projections to and from the parietal lobe have been identified via an indirect AF segment (Catani et al., 2005). Frontal terminations have extended beyond BA 44 and 45 into BA 47, BA 9, BA 46, and BA 6 (Rilling et al., 2008). Traditional language models that only consider the AF as connecting Broca’s and Wernicke’s areas overlook the other possible ways in which AF fibers could support language and related cognitive processes.
One of these alternative functions could fall in the realm of semantic integration. In our previous study, lesions in the MTG and underlying white matter were associated with severe lexical comprehension impairments (Dronkers et al., 2004). In the present study, the MTG ROI was found to include the AF, specifically the direct and indirect segments as described by Catani et al. (2005). Whether these middle temporal AF fibers are critical for lexical comprehension cannot be determined from the existing data. However, the present findings do suggest that fibers of this tract connecting to the MTG could support the integration of lexical-semantics with other cognitive and linguistic mechanisms that are also involved in language comprehension. Lexical–semantic integration is certainly not the only function of this tract. Among other functions, the AF has been proposed as a dorsal route for auditory-motor mapping (Saur et al., 2008, 2010) in accordance with a dual-stream model which segregates phonological and semantic processing (Hickok and Poeppel, 2004). Most likely, the AF is a composite structure with multiple compartments specializing in transmitting different types of information. Whether this hypothesis is true is a question for future investigations.
Other Observations and Some Questions for Future Research
The present study identified numerous major fiber pathways and functional connections that may form the backbone of the language comprehension network. Some of these structural and functional pathways and the cortical regions associated with them might be uniquely dedicated to auditory sentence comprehension. Another possibility is that each component of the language comprehension network might play multiple roles in the large-scale distributed neural systems that support different aspects of language and cognition. This possibility is suggested by the overlapping but distinct connectivity profiles that we found for each of the regions of interest.
What is specific to language comprehension might be the coordinated recruitment of these components to form a functional network to process verbal information. Thus, there are two important questions to be addressed in future investigations. One question is the precise delineation of the functional roles of individual fiber pathways and cortical regions in the language comprehension process. The other question is the characterization of the organizing principles that shape the overall architecture of the language comprehension network.
While several white matter pathways and cortical regions were found to be associated with the regions identified as being critical for comprehension by our earlier lesion-symptom analysis (Dronkers et al., 2004), the present findings do not establish which of these play essential roles. For instance, our findings suggest the involvement of the MdLF and the UF in language comprehension. However, surgical removal of parts of these pathways does not appear to elicit permanent language deficits (Duffau et al., 2009; De Witt Hamer et al., 2010). The fact that these structures were associated with our regions of interest does not necessarily mean that they support language comprehension per se. More information regarding the roles of these pathways may come from studies involving neurological patients.
Our functional connectivity findings implicate the right hemisphere homologues of key left hemisphere areas in the language comprehension network. Our tractography findings indicate that left hemisphere lesions that produce comprehension deficits affect temporo-temporal interhemispheric connections via the tapetum. Right hemisphere involvement in the language comprehension network (Just et al., 1996; Jung-Beeman, 2005) is another important question for future investigations.
Detailed analysis of neurological patient data with advanced imaging protocols will provide important clues as to the organization of this system, how it is affected by well-circumscribed lesions to its cortical and WM components, and the specific roles of each of these components in the overall functioning of the network. Combined analysis of data from healthy subjects and neurological patients will also play an important role (Turken et al., 2008). Further insights will also be gained by computer simulations of the effects of lesions in different parts of the language network to predict the functional impact of well-circumscribed brain lesions and to examine where the network might be most vulnerable to neurological damage (Achard et al., 2006; Kaiser et al., 2007; Nomura et al., 2010). Finally, better characterization of the language network and the relationship between lesion anatomy and behavioral disturbances will also allow a better characterization of patterns of recovery in aphasia, which is one of the key questions in behavioral neurology (Price, 2010).
Limitations
Each of the methods presented here naturally has its own limitations. The regions of interest that served as our starting point reflect the lesion-symptom mapping findings from only one study. While this study included a large a number of aphasic patients, there are certainly other lesion analyses and functional neuroimaging studies that can be used to identify and further refine regions of interest. The lesion findings were also restricted to the vascular territories affected in stroke. Therefore, cortical areas that emerged from the functional connectivity analysis, such as the dorso-medial frontal regions, would not have been identified in the patient findings. Structural and functional connectivity measures derived from in vivo magnetic resonance imaging data need to be interpreted with caution until they are fully validated with histological measures (Mesulam, 2009). While streamline tractography with DTI provides information on the trajectories of the core sections of major tracts, it cannot determine their cortical terminations with certainty (Hagmann et al., 2006). The utilization of established streamline tractography protocols in this investigation allowed us to identify the pathways associated with our earlier lesion findings in relation to DTI-based WM atlases (Catani and Thiebaut de Schotten, 2008; Mori et al., 2008), and to make predictions about the cortical destinations. Future investigations using high-angular resolution diffusion imaging protocols (Hagmann et al., 2008) and probabilistic tractography algorithms (Frey et al., 2008) can provide more precise mapping of these pathways. Resting-state functional connectivity reveals which regions exhibit coherent activity patterns, but cannot distinguish between monosynaptic and polysynaptic connections (Kelly et al., 2010). Despite such limitations, combining findings from neurological patients, with the newly available tools for assessing connectional anatomy of the brain, is a step in the right direction, both for understanding how language is organized in the brain, and for understanding the disorders that are caused by a disruption within the language network.
Conclusion
In this research, we analyzed the structural and functional connectivity of the brain regions found in our earlier investigation with aphasic patients to be critical for sentence comprehension. This analysis revealed a broadly distributed network of peri-sylvian and neighboring cortical association areas residing primarily, but not exclusively, in the left hemisphere and supported by numerous long-distance white matter pathways. These tracts span temporal, parietal, and frontal association cortices and provide a structural backbone for the language comprehension network, integrating the neural computations carried out by key cortical nodes. The additional finding of an extensive connectivity pattern underlying the left MTG answered an earlier question as to why lesions affecting these structures produce such severe and persisting comprehension deficits. The unique trajectories of the IOFF and the MTG compartment of the AF were also seen as novel additions to the network that supports language comprehension. Through the integration of three different methodologies – lesion analysis, white matter tractography, and resting state functional MRI – a vast network supporting language comprehension emerged, one far more extensive than previously thought, and one that may more accurately reflect the complexity of human language.
Conflict of Interest Statement
The authors declare that the research was conducted in the absence of any commercial or financial relationships that could be construed as a potential conflict of interest.
Acknowledgments
This investigation was supported by a Department of Veterans Affairs CSR&D Career Development Award to And U. Turken, a CSR&D Research Career Scientist award to Nina F. Dronkers, and a grant from VA CSR&D Merit Review Program to Nina F. Dronkers and And U. Turken. We would like to thank the International Consortium for Brain Mapping (ICBM) and the Neuroimaging Informatics Tools and Resources Clearinghouse (NITRC) for making the diffusion and fMRI datasets that we have used in this research publicly available. We thank Susan Whitfield-Gabrieli for making the Functional Connectivity Toolbox available and for providing assistance in its use. Finally, we would like to thank members of the Center for Aphasia and Related Disorders, including Juliana Baldo, Shira Katseff, Carl Ludy, David Wilkins, Tim Justus, Analia Aravelo, and Janet Patterson for their input during the preparation of this manuscript, and Drs. Jeffrey R. Binder and Soo-Eun Chang for their insightful comments as reviewers.
Footnotes
- ^www.mathworks.com
- ^http://crl.ucsd.edu/vlsm
- ^http://www.loni.ucla.edu/ICBM
- ^https://www.mristudio.org
- ^http://www.trackvis.org
- ^http://www.nitrc.org/projects/fcon_1000
- ^http://www.fil.ion.ucl.ac.uk/spm/
- ^http://www.nitrc.org/projects/conn
- ^www.fmrib.ox.ac.uk/fsl
- ^www.nitrc.org/projects/mricron
- ^It should be noted that while the MdLF has been shown to interconnect inferior parietal regions with the multimodal areas of the STG in the monkey brain Schmahmann et al. (2007), it is still unknown how this fiber bundle relates to the MTG in the human brain.
References
Achard, S., Salvador, R., Whitcher, B., Suckling, J., and Bullmore, E. (2006). A resilient, low-frequency, small-world human brain functional network with highly connected association cortical hubs. J. Neurosci. 26, 63–72.
Alexander, M. P., Hiltbrunner, B., and Fischer, R. S. (1989). Distributed anatomy of transcortical sensory aphasia. Arch. Neurol. 46, 885–892.
Anwander, A., Tittgemeyer, M., von Cramon, D. Y., Friederici, A. D., and Knosche, T. R. (2007). Connectivity-based parcellation of Broca’s area. Cereb. Cortex 17, 816–825.
Basser, P. J. (1995). Inferring microstructural features and the physiological state of tissues from diffusion-weighted images. NMR Biomed. 8, 333–344.
Basser, P. J., Mattiello, J., and LeBihan, D. (1994). MR diffusion tensor spectroscopy and imaging. Biophys. J. 66, 259–267.
Basser, P. J., Pajevic, S., Pierpaoli, C., Duda, J., and Aldroubi, A. (2000). In vivo fiber tractography using DT-MRI data. Magn. Reson. Med. 44, 625–632.
Bates, E., Wilson, S. M., Saygin, A. P., Dick, F., Sereno, M. I., Knight, R. T., and Dronkers, N. F. (2003). Voxel-based lesion-symptom mapping. Nat. Neurosci. 6, 448–450.
Behzadi, Y., Restom, K., Liau, J., and Liu, T. T. (2007). A component based noise correction method (CompCor) for BOLD and perfusion based fMRI. Neuroimage 37, 90–101.
Binder, J. R. (2003). “Wernicke aphasia: a disorder of central language processing’, in Neurological Foundations of Cognitive Neuroscience, ed. M. E. D’Esposito (Cambridge: The MIT Press), 75–238.
Binder, J. R., Desai, R. H., Graves, W. W., and Conant, L. L. (2009). Where is the semantic system? A critical review and meta-analysis of 120 functional neuroimaging studies. Cereb. Cortex 19, 2767–2796.
Binder, J. R., Frost, J. A., Hammeke, T. A., Cox, R. W., Rao, S. M., and Prieto, T. (1997). Human brain language areas identified by functional magnetic resonance imaging. J. Neurosci. 17, 353–362.
Biswal, B., Yetkin, F. Z., Haughton, V. M., and Hyde, J. S. (1995). Functional connectivity in the motor cortex of resting human brain using echo-planar MRI. Magn. Reson. Med. 34, 537–541.
Biswal, B. B., Mennes, M., Zuo, X. N., Gohel, S., Kelly, C., Smith, S. M., Beckmann, C. F., Adelstein, J. S., Buckner, R. L., Colcombe, S., Dogonowski, A. M., Ernst, M., Fair, D., Hampson, M., Hoptman, M. J., Hyde, J. S., Kiviniemi, V. J., Kotter, R., Li, S. J., Lin, C. P., Lowe, M. J., Mackay, C., Madden, D. J., Madsen, K. H., Margulies, D. S., Mayberg, H. S., McMahon, K., Monk, C. S., Mostofsky, S. H., Nagel, B. J., Pekar, J. J., Peltier, S. J., Petersen, S. E., Riedl, V., Rombouts, S. A., Rypma, B., Schlaggar, B. L., Schmidt, S., Seidler, R. D., Siegle, G. J., Sorg, C., Teng, G. J., Veijola, J., Villringer, A., Walter, M., Wang, L., Weng, X. C., Whitfield-Gabrieli, S., Williamson, P., Windischberger, C., Zang, Y. F., Zhang, H. Y., Castellanos, F. X., and Milham, M. P. (2010). Toward discovery science of human brain function. Proc. Natl. Acad. Sci. U.S.A. 107, 4734–4739.
Boatman, D., Gordon, B., Hart, J., Selnes, O., Miglioretti, D., and Lenz, F. (2000). Transcortical sensory aphasia: revisited and revised. Brain 123(Pt 8), 1634–1642.
Bogen, J. E., and Bogen, G. M. (1976). Wernicke’s region-where is it? Ann. N. Y. Acad. Sci. 280, 834–843.
Bookheimer, S. (2002). Functional MRI of language: new approaches to understanding the cortical organization of semantic processing. Annu. Rev. Neurosci. 25, 151–188.
Brauer, J., Anwander, A., and Friederici, A. D. (2010). Neuroanatomical prerequisites for language functions in the maturing brain. Cereb. Cortex 41, 1484–1492.
Buckner, R. L., Sepulcre, J., Talukdar, T., Krienen, F. M., Liu, H., Hedden, T., Andrews-Hanna, J. R., Sperling, R. A., and Johnson, K. A. (2009). Cortical hubs revealed by intrinsic functional connectivity: mapping, assessment of stability, and relation to Alzheimer’s disease. J. Neurosci. 29, 1860–1873.
Burgel, U., Amunts, K., Hoemke, L., Mohlberg, H., Gilsbach, J. M., and Zilles, K. (2006). White matter fiber tracts of the human brain: three-dimensional mapping at microscopic resolution, topography and intersubject variability. Neuroimage 29, 1092–1105.
Caplan, D., Hildebrandt, N., and Makris, N. (1996). Location of lesions in stroke patients with deficits in syntactic processing in sentence comprehension. Brain 119(Pt 3), 933–949.
Caplan, D., Waters, G., Kennedy, D., Alpert, N., Makris, N., Dede, G., Michaud, J., and Reddy, A. (2007). A study of syntactic processing in aphasia II: neurological aspects. Brain Lang. 101, 151–177.
Catani, M., Allin, M. P., Husain, M., Pugliese, L., Mesulam, M. M., Murray, R. M., and Jones, D. K. (2007). Symmetries in human brain language pathways correlate with verbal recall. Proc. Natl. Acad. Sci. U.S.A. 104, 17163–17168.
Catani, M., Howard, R. J., Pajevic, S., and Jones, D. K. (2002). Virtual in vivo interactive dissection of white matter fasciculi in the human brain. Neuroimage 17, 77–94.
Catani, M., Jones, D. K., and ffytche, D. H. (2005). Perisylvian language networks of the human brain. Ann. Neurol. 57, 8–16.
Catani, M., and Mesulam, M. (2008a). The arcuate fasciculus and the disconnection theme in language and aphasia: history and current state. Cortex 44, 953–961.
Catani, M., and Thiebaut de Schotten, M. (2008). A diffusion tensor imaging tractography atlas for virtual in vivo dissections. Cortex 44, 1105–1132.
Conturo, T. E., Lori, N. F., Cull, T. S., Akbudak, E., Snyder, A. Z., Shimony, J. S., McKinstry, R. C., Burton, H., and Raichle, M. E. (1999). Tracking neuronal fiber pathways in the living human brain. Proc. Natl. Acad. Sci. U.S.A. 96, 10422–10427.
Croxson, P. L., Johansen-Berg, H., Behrens, T. E., Robson, M. D., Pinsk, M. A., Gross, C. G., Richter, W., Richter, M. C., Kastner, S., and Rushworth, M. F. (2005). Quantitative investigation of connections of the prefrontal cortex in the human and macaque using probabilistic diffusion tractography. J. Neurosci. 25, 8854–8866.
Curtiss, S., and Yamada, J. (1988). Curtiss–Yamada Comprehensive Language Evaluation. Unpublished test, UCLA.
Damasio, A. R. (1989). Time-locked multiregional retroactivation: a systems-level proposal for the neural substrates of recall and recognition. Cognition 33, 25–62.
Damasio, A. R. (1991). “Signs of aphasia,” in Acquired Aphasia, 2nd Edn. ed. M. T. Sarno (San Diego, CA: Academic Press), 27–43.
Damasio, A. R., and Damasio, H. (2002). Principles of Behavioral and Cognitive Neurology, 2nd Edn. New York: Oxford University Press.
Damoiseaux, J. S., Rombouts, S. A., Barkhof, F., Scheltens, P., Stam, C. J., Smith, S. M., and Beckmann, C. F. (2006). Consistent resting-state networks across healthy subjects. Proc. Natl. Acad. Sci. U.S.A. 103, 13848–13853.
Dancause, N., Barbay, S., Frost, S. B., Plautz, E. J., Chen, D., Zoubina, E. V., Stowe, A. M., and Nudo, R. J. (2005). Extensive cortical rewiring after brain injury. J. Neurosci. 25, 10167–10179.
De Witt Hamer, P. C., Moritz-Gasser, S., Gatignol, P., and Duffau, H. (2010). Is the human left middle longitudinal fascicle essential for language? A brain electrostimulation study. Hum. Brain Mapp.
DeArmond, S. J., Fusco, M. M., and Dewey, M. M. (1989). Structure of the Human Brain: A Photographic Atlas. New York: Oxford University Press.
Demb, J. B., Desmond, J. E., Wagner, A. D., Vaidya, C. J., Glover, G. H., and Gabrieli, J. D. (1995). Semantic encoding and retrieval in the left inferior prefrontal cortex: a functional MRI study of task difficulty and process specificity. J. Neurosci. 15, 5870–5878.
Demonet, J. F., Chollet, F., Ramsay, S., Cardebat, D., Nespoulous, J. L., Wise, R., Rascol, A., and Frackowiak, R. (1992). The anatomy of phonological and semantic processing in normal subjects. Brain 115(Pt 6), 1753–1768.
Desikan, R. S., Segonne, F., Fischl, B., Quinn, B. T., Dickerson, B. C., Blacker, D., Buckner, R. L., Dale, A. M., Maguire, R. P., Hyman, B. T., Albert, M. S., and Killiany, R. J. (2006). An automated labeling system for subdividing the human cerebral cortex on MRI scans into gyral based regions of interest. Neuroimage 31, 968–980.
Dosenbach, N. U., Fair, D. A., Miezin, F. M., Cohen, A. L., Wenger, K. K., Dosenbach, R. A., Fox, M. D., Snyder, A. Z., Vincent, J. L., Raichle, M. E., Schlaggar, B. L., and Petersen, S. E. (2007). Distinct brain networks for adaptive and stable task control in humans. Proc. Natl. Acad. Sci. U.S.A. 104, 11073–11078.
Dronkers, N., Pinker, S., and Damasio, A. R., (2000a). “Language and the aphasias,” in Principles in Neural Science, 4th Edn. eds E. R. Kandel, J. Schwartz, and T. Jessell (New York: McGraw-Hill), 1169–1187.
Dronkers, N. F., Redfern, B. B., and Knight, R. T., (2000b). “The neural architecture of language disorders,” in The New Cognitive Neurosciences, ed. M. S. Gazzaniga (Cambridge: The MIT Press), 949–958.
Dronkers, N. F., Redfern, B. B., and Ludy, C. A. (1995). Lesion localization in chronic Wernicke’s aphasia. Brain Lang. 51, 62–65.
Dronkers, N. F., Wilkins, D. P., Van Valin, R. D. J., Redfern, B. B., and Jaeger, J. J. (1994). A reconsideration of the brain areas involved in the disruption of morphosyntactic comprehension. Brain Lang. 47, 461–463.
Dronkers, N. F., Wilkins, D. P., Van Valin, R. D. Jr., Redfern, B. B., and Jaeger, J. J. (2004). Lesion analysis of the brain areas involved in language comprehension. Cognition 92, 145–177.
Duffau, H. (2008). The anatomo-functional connectivity of language revisited. New insights provided by electrostimulation and tractography. Neuropsychologia 46, 927–934.
Duffau, H., Gatignol, P., Mandonnet, E., Peruzzi, P., Tzourio-Mazoyer, N., and Capelle, L. (2005). New insights into the anatomo-functional connectivity of the semantic system: a study using cortico-subcortical electrostimulations. Brain 128, 797–810.
Duffau, H., Gatignol, P., Moritz-Gasser, S., and Mandonnet, E. (2009). Is the left uncinate fasciculus essential for language? A cerebral stimulation study. J. Neurol. 256, 382–389.
Duffau, H., Peggy Gatignol, S. T., Mandonnet, E., Capelle, L., and Taillandier, L. (2008). Intraoperative subcortical stimulation mapping of language pathways in a consecutive series of 115 patients with grade II glioma in the left dominant hemisphere. J. Neurosurg. 109, 461–471.
Fernandez-Miranda, J. C., Rhoton, A. L., Jr., Alvarez-Linera, J., Kakizawa, Y., Choi, C., and de Oliveira, E. P. (2008). Three-dimensional microsurgical and tractographic anatomy of the white matter of the human brain. Neurosurgery 62, 989–1026; discussion 1026–1028.
Ferstl, E. C., Neumann, J., Bogler, C., and von Cramon, D. Y. (2008). The extended language network: a meta-analysis of neuroimaging studies on text comprehension. Hum. Brain Mapp. 29, 581–593.
Fillard, P., Pennec, X., Arsigny, V., and Ayache, N. (2007). Clinical DT-MRI estimation, smoothing, and fiber tracking with log-Euclidean metrics. IEEE Trans. Med. Imaging 26, 1472–1482.
Fox, M. D., Snyder, A. Z., Vincent, J. L., Corbetta, M., Van Essen, D. C., and Raichle, M. E. (2005). The human brain is intrinsically organized into dynamic, anticorrelated functional networks. Proc. Natl. Acad. Sci. U.S.A. 102, 9673–9678.
Frey, S., Campbell, J. S., Pike, G. B., and Petrides, M. (2008). Dissociating the human language pathways with high angular resolution diffusion fiber tractography. J. Neurosci. 28, 11435–11444.
Friederici, A. D. (2002). Towards a neural basis of auditory sentence processing. Trends Cogn. Sci. 6, 78–84.
Friederici, A. D. (2009). Pathways to language: fiber tracts in the human brain. Trends Cogn. Sci. 13, 175–181.
Gabrieli, J. D., Poldrack, R. A., and Desmond, J. E. (1998). The role of left prefrontal cortex in language and memory. Proc. Natl. Acad. Sci. U.S.A. 95, 906–913.
Glasser, M. F., and Rilling, J. K. (2008). DTI tractography of the human brain’s language pathways. Cereb. Cortex 18, 2471–2482.
Greicius, M. D., Krasnow, B., Reiss, A. L., and Menon, V. (2003). Functional connectivity in the resting brain: a network analysis of the default mode hypothesis. Proc. Natl. Acad. Sci. U.S.A. 100, 253–258.
Hagmann, P., Cammoun, L., Gigandet, X., Meuli, R., Honey, C. J., Wedeen, V. J., and Sporns, O. (2008). Mapping the structural core of human cerebral cortex. PLoS Biol. 6, e159. doi:10.1371/journal.pbio.0060159
Hagmann, P., Jonasson, L., Maeder, P., Thiran, J. P., Wedeen, V. J., and Meuli, R. (2006). Understanding diffusion MR imaging techniques: from scalar diffusion-weighted imaging to diffusion tensor imaging and beyond. Radiographics 26(Suppl. 1), S205–S223.
Hagoort, P., Hald, L., Bastiaansen, M., and Petersson, K. M. (2004). Integration of word meaning and world knowledge in language comprehension. Science 304, 438–441.
Hampson, M., Peterson, B. S., Skudlarski, P., Gatenby, J. C., and Gore, J. C. (2002). Detection of functional connectivity using temporal correlations in MR images. Hum. Brain Mapp. 15, 247–262.
Hart, J. Jr., and Gordon, B. (1990). Delineation of single-word semantic comprehension deficits in aphasia, with anatomical correlation. Ann. Neurol. 27, 226–231.
He, B. J., Snyder, A. Z., Vincent, J. L., Epstein, A., Shulman, G. L., and Corbetta, M. (2007). Breakdown of functional connectivity in frontoparietal networks underlies behavioral deficits in spatial neglect. Neuron 53, 905–918.
Heiss, W. D., Thiel, A., Kessler, J., and Herholz, K. (2003). Disturbance and recovery of language function: correlates in PET activation studies. Neuroimage 20(Suppl. 1), S42–S49.
Hickok, G., and Poeppel, D. (2004). Dorsal and ventral streams: a framework for understanding aspects of the functional anatomy of language. Cognition 92, 67–99.
Hillis, A. E., Boatman, D., Hart, J., and Gordon, B. (1999). Making sense out of jargon: a neurolinguistic and computational account of jargon aphasia. Neurology 53, 1813–1824.
Hua, K., Oishi, K., Zhang, J., Wakana, S., Yoshioka, T., Zhang, W., Akhter, K. D., Li, X., Huang, H., Jiang, H., van Zijl, P., and Mori, S. (2009). Mapping of functional areas in the human cortex based on connectivity through association fibers. Cereb. Cortex 19, 1889–1895.
Hua, K., Zhang, J., Wakana, S., Jiang, H., Li, X., Reich, D. S., Calabresi, P. A., Pekar, J. J., van Zijl, P. C., and Mori, S. (2008). Tract probability maps in stereotaxic spaces: analyses of white matter anatomy and tract-specific quantification. Neuroimage 39, 336–347.
Jung-Beeman, M. (2005). Bilateral brain processes for comprehending natural language. Trends Cogn. Sci. 9, 512–518.
Just, M. A., Carpenter, P. A., Keller, T. A., Eddy, W. F., and Thulborn, K. R. (1996). Brain activation modulated by sentence comprehension. Science 274, 114–116.
Kaiser, M., Martin, R., Andras, P., and Young, M. P. (2007). Simulation of robustness against lesions of cortical networks. Eur. J. Neurosci. 25, 3185–3192.
Kaplan, E., Naeser, M. A., Martin, P. I., Ho, M., Wang, Y., Baker, E., and Pascual-Leone, A. (2010). Horizontal portion of arcuate fasciculus fibers track to pars opercularis, not pars triangularis, in right and left hemispheres: a DTI study. Neuroimage 52, 436–444.
Kelly, C., Uddin, L. Q., Shehzad, Z., Margulies, D. S., Castellanos, F. X., Milham, M. P., and Petrides, M. (2010). Broca’s region: linking human brain functional connectivity data and non-human primate tracing anatomy studies. Eur. J. Neurosci. 32, 383–398.
Kertesz, A., Harlock, W., and Coates, R. (1979). Computer tomographic localization, lesion size, and prognosis in aphasia and nonverbal impairment. Brain Lang. 8, 34–50.
Kertesz, A., Lau, W. K., and Polk, M. (1993). The structural determinants of recovery in Wernicke’s aphasia. Brain Lang. 44, 153–164.
Kertesz, A., Sheppard, A., and MacKenzie, R. (1982). Localization in transcortical sensory aphasia. Arch. Neurol. 39, 475–478.
Kier, E. L., Staib, L. H., Davis, L. M., and Bronen, R. A. (2004). MR imaging of the temporal stem: anatomic dissection tractography of the uncinate fasciculus, inferior occipitofrontal fasciculus, and Meyer’s loop of the optic radiation. AJNR Am. J. Neuroradiol. 25, 677–691.
Koyama, M. S., Kelly, C., Shehzad, Z., Penesetti, D., Castellanos, F. X., and Milham, M. P. (2010). Reading networks at rest. Cereb. Cortex 10, 2549–2559.
Lawes, I. N., Barrick, T. R., Murugam, V., Spierings, N., Evans, D. R., Song, M., and Clark, C. A. (2008). Atlas-based segmentation of white matter tracts of the human brain using diffusion tensor tractography and comparison with classical dissection. Neuroimage 39, 62–79.
Lowe, M. J., Mock, B. J., and Sorenson, J. A. (1998). Functional connectivity in single and multislice echoplanar imaging using resting-state fluctuations. Neuroimage 7, 119–132.
Makris, N., Kennedy, D. N., McInerney, S., Sorensen, A. G., Wang, R., Caviness, V. S., Jr., and Pandya, D. N. (2005). Segmentation of subcomponents within the superior longitudinal fascicle in humans: a quantitative, in vivo, DT-MRI study. Cereb. Cortex 15, 854–869.
Makris, N., and Pandya, D. N. (2009). The extreme capsule in humans and rethinking of the language circuitry. Brain Struct. Funct. 213, 343–358.
Makris, N., Papadimitriou, G. M., Kaiser, J. R., Sorg, S., Kennedy, D. N., and Pandya, D. N. (2009). Delineation of the middle longitudinal fascicle in humans: a quantitative, in vivo, DT-MRI study. Cereb. Cortex 19, 777–785.
Margulies, D. S., Vincent, J. L., Kelly, C., Lohmann, G., Uddin, L. Q., Biswal, B. B., Villringer, A., Castellanos, F. X., Milham, M. P., and Petrides, M. (2009). Precuneus shares intrinsic functional architecture in humans and monkeys. Proc. Natl. Acad. Sci. U.S.A. 106, 20069–20074.
Marsh, E. B., and Hillis, A. E. (2006). Recovery from aphasia following brain injury: the role of reorganization. Prog. Brain Res. 157, 143–156.
Martino, J., Brogna, C., Robles, S. G., Vergani, F., and Duffau, H. (2010). Anatomic dissection of the inferior fronto-occipital fasciculus revisited in the lights of brain stimulation data. Cortex 46, 691–699.
Mesulam, M. (2005). Imaging connectivity in the human cerebral cortex: the next frontier? Ann. Neurol. 57, 5–7.
Mesulam, M. (2009). Defining neurocognitive networks in the BOLD new world of computed connectivity. Neuron 62, 1–3.
Mesulam, M. M. (1990). Large-scale neurocognitive networks and distributed processing for attention, language, and memory. Ann. Neurol. 28, 597–613.
Mori, S., Kaufmann, W. E., Davatzikos, C., Stieltjes, B., Amodei, L., Fredericksen, K., Pearlson, G. D., Melhem, E. R., Solaiyappan, M., Raymond, G. V., Moser, H. W., and van Zijl, P. C. (2002). Imaging cortical association tracts in the human brain using diffusion-tensor-based axonal tracking. Magn. Reson. Med. 47, 215–223.
Mori, S., Oishi, K., Jiang, H., Jiang, L., Li, X., Akhter, K., Hua, K., Faria, A. V., Mahmood, A., Woods, R., Toga, A. W., Pike, G. B., Neto, P. R., Evans, A., Zhang, J., Huang, H., Miller, M. I., van Zijl, P., and Mazziotta, J. (2008). Stereotaxic white matter atlas based on diffusion tensor imaging in an ICBM template. Neuroimage 40, 570–582.
Murphy, T. H., and Corbett, D. (2009). Plasticity during stroke recovery: from synapse to behaviour. Nat. Rev. Neurosci. 10, 861–872.
Naeser, M. A., Alexander, M. P., Helm-Estabrooks, N., Levine, H. L., Laughlin, S. A., and Geschwind, N. (1982). Aphasia with predominantly subcortical lesion sites: description of three capsular/putaminal aphasia syndromes. Arch. Neurol. 39, 2–14.
Naeser, M. A., Helm-Estabrooks, N., Haas, G., Auerbach, S., and Srinivasan, M. (1987). Relationship between lesion extent in “Wernicke’s area” on computed tomographic scan and predicting recovery of comprehension in Wernicke’s aphasia. Arch. Neurol. 44, 73–82.
Nomura, E. M., Gratton, C., Visser, R. M., Kayser, A., Perez, F., and D’Esposito, M. (2010). Double dissociation of two cognitive control networks in patients with focal brain lesions. Proc. Natl. Acad. Sci. U.S.A. 107, 12017–12022.
Oishi, K., Zilles, K., Amunts, K., Faria, A., Jiang, H., Li, X., Akhter, K., Hua, K., Woods, R., Toga, A. W., Pike, G. B., Rosa-Neto, P., Evans, A., Zhang, J., Huang, H., Miller, M. I., van Zijl, P. C., Mazziotta, J., and Mori, S. (2008). Human brain white matter atlas: identification and assignment of common anatomical structures in superficial white matter. Neuroimage 43, 447–457.
Parker, G. J., Luzzi, S., Alexander, D. C., Wheeler-Kingshott, C. A., Ciccarelli, O., and Lambon Ralph, M. A. (2005). Lateralization of ventral and dorsal auditory-language pathways in the human brain. Neuroimage 24, 656–666.
Petrides, M., and Pandya, D. N. (1988). Association fiber pathways to the frontal cortex from the superior temporal region in the rhesus monkey. J. Comp. Neurol. 273, 52–66.
Petrides, M., and Pandya, D. N. (2006). Efferent association pathways originating in the caudal prefrontal cortex in the macaque monkey. J. Comp. Neurol. 498, 227–251.
Petrides, M., and Pandya, D. N. (2009). Distinct parietal and temporal pathways to the homologues of Broca’s area in the monkey. PLoS Biol. 7, e1000170. doi: 10.1371/journal.pbio.1000170
Pierpaoli, C., Jezzard, P., Basser, P. J., Barnett, A., and Di Chiro, G. (1996). Diffusion tensor MR imaging of the human brain. Radiology 201, 637–648.
Poldrack, R. A., Wagner, A. D., Prull, M. W., Desmond, J. E., Glover, G. H., and Gabrieli, J. D. (1999). Functional specialization for semantic and phonological processing in the left inferior prefrontal cortex. Neuroimage 10, 15–35.
Powell, H. W., Parker, G. J., Alexander, D. C., Symms, M. R., Boulby, P. A., Wheeler-Kingshott, C. A., Barker, G. J., Noppeney, U., Koepp, M. J., and Duncan, J. S. (2006). Hemispheric asymmetries in language-related pathways: a combined functional MRI and tractography study. Neuroimage 32, 388–399.
Price, C. J. (2000). The anatomy of language: contributions from functional neuroimaging. J. Anat. 197(Pt 3), 335–359.
Price, C. J. (2010). The anatomy of language: a review of 100 fMRI studies published in 2009. Ann. N. Y. Acad. Sci. 1191, 62–88.
Rilling, J. K., Glasser, M. F., Preuss, T. M., Ma, X., Zhao, T., Hu, X., and Behrens, T. E. (2008). The evolution of the arcuate fasciculus revealed with comparative DTI. Nat. Neurosci. 11, 426–428.
Rilling, J. K., and Seligman, R. A. (2002). A quantitative morphometric comparative analysis of the primate temporal lobe. J. Hum. Evol. 42, 505–533.
Saur, D., Kreher, B. W., Schnell, S., Kummerer, D., Kellmeyer, P., Vry, M. S., Umarova, R., Musso, M., Glauche, V., Abel, S., Huber, W., Rijntjes, M., Hennig, J., and Weiller, C. (2008). Ventral and dorsal pathways for language. Proc. Natl. Acad. Sci. U.S.A. 105, 18035–18040.
Saur, D., Schelter, B., Schnell, S., Kratochvil, D., Kupper, H., Kellmeyer, P., Kummerer, D., Kloppel, S., Glauche, V., Lange, R., Mader, W., Feess, D., Timmer, J., and Weiller, C. (2010). Combining functional and anatomical connectivity reveals brain networks for auditory language comprehension. Neuroimage 49, 3187–3197.
Schmahmann, J. D., and Pandya, D. N. (2006). Fiber Pathways of the Brain. Oxford; NY: Oxford University Press.
Schmahmann, J. D., and Pandya, D. N. (2007). Cerebral white matter-historical evolution of facts and notions concerning the organization of the fiber pathways of the brain. J. Hist. Neurosci. 16, 237–267.
Schmahmann, J. D., and Pandya, D. N. (2008). Disconnection syndromes of basal ganglia, thalamus, and cerebrocerebellar systems. Cortex 44, 1037–1066.
Schmahmann, J. D., Pandya, D. N., Wang, R., Dai, G., D’Arceuil, H. E., de Crespigny, A. J., and Wedeen, V. J. (2007). Association fibre pathways of the brain: parallel observations from diffusion spectrum imaging and autoradiography. Brain 130, 630–653.
Seltzer, B., and Pandya, D. N. (1984). Further observations on parieto-temporal connections in the rhesus monkey. Exp. Brain Res. 55, 301–312.
Seltzer, B., and Pandya, D. N. (1994). Parietal, temporal, and occipital projections to cortex of the superior temporal sulcus in the rhesus monkey: a retrograde tracer study. J. Comp. Neurol. 343, 445–463.
Shehzad, Z., Kelly, A. M., Reiss, P. T., Gee, D. G., Gotimer, K., Uddin, L. Q., Lee, S. H., Margulies, D. S., Roy, A. K., Biswal, B. B., Petkova, E., Castellanos, F. X., and Milham, M. P. (2009). The resting brain: unconstrained yet reliable. Cereb. Cortex 19, 2209–2229.
Thompson-Schill, S. L., D’Esposito, M., Aguirre, G. K., and Farah, M. J. (1997). Role of left inferior prefrontal cortex in retrieval of semantic knowledge: a reevaluation. Proc. Natl. Acad. Sci. U.S.A. 94, 14792–14797.
Ture, U., Yasargil, M. G., Friedman, A. H., and Al-Mefty, O. (2000). Fiber dissection technique: lateral aspect of the brain. Neurosurgery 47, 417–426; discussion 426–417.
Turken, A., Whitfield-Gabrieli, S., Bammer, R., Baldo, J. V., Dronkers, N. F., and Gabrieli, J. D. (2008). Cognitive processing speed and the structure of white matter pathways: convergent evidence from normal variation and lesion studies. Neuroimage 42, 1032–1044.
Tyler, L. K., and Marslen-Wilson, W. (2008). Fronto-temporal brain systems supporting spoken language comprehension. Philos. Trans. R. Soc. Lond., B, Biol. Sci. 363, 1037–1054.
Tzourio-Mazoyer, N., Landeau, B., Papathanassiou, D., Crivello, F., Etard, O., Delcroix, N., Mazoyer, B., and Joliot, M. (2002). Automated anatomical labeling of activations in SPM using a macroscopic anatomical parcellation of the MNI MRI single-subject brain. Neuroimage 15, 273–289.
Van Dijk, K. R., Hedden, T., Venkataraman, A., Evans, K. C., Lazar, S. W., and Buckner, R. L. (2010). Intrinsic functional connectivity as a tool for human connectomics: theory, properties, and optimization. J. Neurophysiol. 103, 297–321.
Vernooij, M. W., Smits, M., Wielopolski, P. A., Houston, G. C., Krestin, G. P., and van der Lugt, A. (2007). Fiber density asymmetry of the arcuate fasciculus in relation to functional hemispheric language lateralization in both right- and left-handed healthy subjects: a combined fMRI and DTI study. Neuroimage 35, 1064–1076.
Vigneau, M., Beaucousin, V., Herve, P. Y., Duffau, H., Crivello, F., Houde, O., Mazoyer, B., and Tzourio-Mazoyer, N. (2006). Meta-analyzing left hemisphere language areas: phonology, semantics, and sentence processing. Neuroimage 30, 1414–1432.
Vincent, J. L., Patel, G. H., Fox, M. D., Snyder, A. Z., Baker, J. T., Van Essen, D. C., Zempel, J. M., Snyder, L. H., Corbetta, M., and Raichle, M. E. (2007). Intrinsic functional architecture in the anaesthetized monkey brain. Nature 447, 83–86.
Wakana, S., Caprihan, A., Panzenboeck, M. M., Fallon, J. H., Perry, M., Gollub, R. L., Hua, K., Zhang, J., Jiang, H., Dubey, P., Blitz, A., van Zijl, P., and Mori, S. (2007). Reproducibility of quantitative tractography methods applied to cerebral white matter. Neuroimage 36, 630–644.
Wakana, S., Jiang, H., Nagae-Poetscher, L. M., van Zijl, P. C., and Mori, S. (2004). Fiber tract-based atlas of human white matter anatomy. Radiology 230, 77–87.
Wang, R., Benner, T., Sorensen, A. G., and Weeden, V. J. (2007). “Diffusion toolkit: a software package for diffusion imaging data processing and Ttactography,” in Annual Meeting of the International Society for Magnetic Resonance Medicine, Berlin.
Ward, N. S. (2005). Plasticity and the functional reorganization of the human brain. Int. J. Psychophysiol. 58, 158–161.
Wedeen, V. J., Wang, R. P., Schmahmann, J. D., Benner, T., Tseng, W. Y., Dai, G., Pandya, D. N., Hagmann, P., D’Arceuil, H., and de Crespigny, A. J. (2008). Diffusion spectrum magnetic resonance imaging (DSI) tractography of crossing fibers. Neuroimage 41, 1267–1277.
Xiang, H. D., Fonteijn, H. M., Norris, D. G., and Hagoort, P. (2010). Topographical functional connectivity pattern in the perisylvian language networks. Cereb. Cortex 20, 549–560.
Keywords: aphasia, language comprehension, language network, structural connectivity, fiber tracts, resting-state functional connectivity, disconnection, middle temporal gyrus
Citation: Turken AU and Dronkers NF (2011) The neural architecture of the language comprehension network: converging evidence from lesion and connectivity analyses. Front. Syst. Neurosci. 5:1. doi: 10.3389/fnsys.2011.00001
Received: 03 November 2010;
Accepted: 03 January 2011;
Published online: 10 February 2011.
Edited by:
Barry Horwitz, National Institutes of Health, USAReviewed by:
Jeffrey R. Binder, Medical College of Wisconsin, USASoo-Eun Chang, Michigan State University, USA
Copyright: © 2011 Turken and Dronkers. This is an open-access article subject to an exclusive license agreement between the authors and Frontiers Media SA, which permits unrestricted use, distribution, and reproduction in any medium, provided the original authors and source are credited.
*Correspondence: And U. Turken, Veterans Affairs Northern California Health Care System, Research Service R4 151/MTZ, 150 Muir Road, Martinez, CA 94553, USA. e-mail:YW5kLnR1cmtlbkB2YS5nb3Y=; Nina F. Dronkers, Veterans Affairs Northern California Health Care System, Center for Aphasia and Related Disorders (126R), 150 Muir Road, Martinez, CA 94553, USA. e-mail:ZHJvbmtlcnNAdWNkYXZpcy5lZHU=