- 1Section on Cellular and Synaptic Physiology, NICHD - Eunice Kennedy Shriver National Institute of Child Health and Human Development, National Institutes of Health (NIH), Bethesda, MD, United States
- 2Newcastle University Biosciences Institute, Newcastle University, Newcastle upon Tyne, United Kingdom
Recent studies have implicated impaired Parvalbumin Fast-Spiking Interneuron (PVIN) function as a precipitating factor underlying abnormalities in network synchrony, oscillatory rhythms, and cognition associated with Alzheimer’s disease (AD). However, a complete developmental investigation of potential gamma deficits, induced by commonly used carbachol or kainate in ex vivo slice preparations, within AD model mice is lacking. We examined gamma oscillations using field recordings in acute hippocampal slices from 5xFAD and control mice, through the period of developing pathology, starting at 3 months of age, when there is minimal plaque presence in the hippocampus, through to 12+ months of age, when plaque burden is high. In addition, we examined PVIN participation in gamma rhythms using targeted cell-attached recordings of genetically-reported PVINs, in both wild type and mutant mice. In parallel, a developmental immunohistochemical characterisation probing the PVIN-associated expression of PV and perineuronal nets (PNNs) was compared between control and 5xFAD mice. Remarkably, this comprehensive longitudinal evaluation failed to reveal any obvious correlations between PVIN deficits (electrical and molecular), circuit rhythmogenesis (gamma frequency and power), and Aβ deposits/plaque formation. By 6–12 months, 5xFAD animals have extensive plaque formation throughout the hippocampus. However, a deficit in gamma oscillatory power was only evident in the oldest 5xFAD animals (12+ months), and only when using kainate, and not carbachol, to induce the oscillations. We found no difference in PV firing or phase preference during kainate-induced oscillations in younger or older 5xFAD mice compared to control, and a reduction of PV and PNNs only in the oldest 5xFAD mice. The lack of a clear relationship between PVIN function, network rhythmicity, and plaque formation in our study highlights an unexpected resilience in PVIN function in the face of extensive plaque pathology associated with this model, calling into question the presumptive link between PVIN pathology and Alzheimer’s progression.
Introduction
Alzheimer’s disease (AD) is a progressive, degenerative, neurological condition which has devastating effects on memory, personality and ultimately other cognitive and physical functions. The disease is most notably characterised by the presence of extracellular amyloid beta (Aβ) plaques and intracellular neurofibrillary tangles (NFT) containing hyperphosphorylated tau, and in later stages, extensive cell death leading to brain atrophy (Ogomori et al., 1989; Braak and Braak, 1991; Serrano-Pozo et al., 2011; Selkoe and Hardy, 2016; Lane et al., 2018).
In recent years there has been an interest in examining how different types of rhythmic brain network activities are affected in AD, especially in relation to cognitive decline seen in the disease. Gamma frequency oscillations (30–90 Hz) are of particular interest given that they have been recorded during cognitively demanding tasks in humans and other animals (Lisman and Idiart, 1995; Fries et al., 2001; Bauer et al., 2007; Colgin and Moser, 2010); and are thought to serve as a metric for higher-order cortical functions, and as a possible mechanism for cortical “binding” of the activity of distinct neuronal populations in regards to a common object of attention (Gray and Singer, 1989; Whittington et al., 1995, 2011; Singer, 1999; Draguhn and Buzsáki, 2004).
There have been a small number of studies which have looked at gamma oscillatory deficits in human patients with AD (Spydell and Sheer, 1983; Ribary et al., 1991; Başar et al., 2016); however, the majority of work has been done using mouse models of the disease. Gamma power has been reported to be reduced in a number of mouse models of AD in vivo: PVJ20 mice and 8-month-old J20 mice displayed reduced CA1 gamma power, recorded in awake behaving mice (Rubio et al., 2012; Etter et al., 2019); similarly, sharp-wave ripple (SWR)-associated decreases in gamma power were recorded in the CA1 of 3-month-old 5xFAD mice in vivo (Iaccarino et al., 2016), and the dentate of aged ApoE4 mice (Gillespie et al., 2016). Ex vivo slice experiments have also shown evidence of network dysfunction in AD with both kainate- and carbachol-induced gamma frequency power reductions seen in brain slices from transgenic mice, and in control brain slices exposed to different forms of Aβ (Driver et al., 2007; Kurudenkandy et al., 2014; Xiao et al., 2017; Arroyo-García et al., 2021).
In the CA3 region of the hippocampus, it is well established that the generation and coordination of gamma frequency oscillations is largely due to the activity of fast-spiking, PV-expressing interneurons (PVINs; Cobb et al., 1995; Whittington et al., 1995, 2011; McBain, 2001; Bartos et al., 2002; Gulyás et al., 2010). Impaired PVIN activity can result in disrupted brain network activity and hypersynchrony in various disorders including AD (Palop and Mucke, 2016). In vitro hippocampal slices from 3-month-old 5xFAD mice displayed increased SWRs and reduced firing frequency of PV-expressing basket cells within CA1 during these network events (Caccavano et al., 2020). PVINs recorded in the parietal cortex of hAPP J20 AD mice expressed lower levels of the voltage-gated Na channel, NaV1.1, and displayed smaller amplitude action potentials and impaired inhibitory synaptic transmission onto layer 2/3 parietal pyramidal cells. This loss in PVIN function also affected network dynamics, yielding reduced gamma frequency activity and coincident network hyperexcitability in vivo, which was restored by rescuing levels of NaV1.1 within PVINs (Verret et al., 2012). Similarly, transplants of NaV1.1-overexpressing PVINs enhanced AP kinetics and firing frequency, reduced hyperexcitability, and restored behaviour-modulated gamma oscillations in hAPP J20 mice (Martinez-Losa et al., 2018). Furthermore, it is widely accepted that gamma frequency stimulation of PVINs is sufficient to induce gamma oscillations, whilst their targeted inhibition suppresses it, showcasing their important role in this form of network activity (Cardin et al., 2009; Sohal et al., 2009). This has led to a number of studies using optogenetic or auditory gamma frequency stimulation of PVINs to improve network and behavioural deficits and reduce plaque burden in a number of AD mouse models, highlighting the importance of studying PVINs with regard to network dysfunction in AD (Iaccarino et al., 2016; Lee et al., 2018; Etter et al., 2019; Martorell et al., 2019; Zhang et al., 2020).
Despite these findings, there is still a lack of knowledge concerning the onset or development of gamma deficits in the disease in relation to plaque burden, and limited comparison of gamma oscillatory activity induced by different mechanisms. Here we provide an in-depth, longitudinal assessment of gamma frequency network disruption, in a well characterised ex vivo brain slice model, in relation to PVIN deficits and plaque deposition. All of the experiments described in this manuscript were done using the 5xFAD Tg-6799 (5xFAD) model (Oakley et al., 2006). Although no model completely recapitulates the human phenotype of the disease, this multi-knock-in model exhibits accelerated plaque pathology, cognitive memory deficits, and synapse/cellular loss (Oakley et al., 2006; Jawhar et al., 2012; Schneider et al., 2014; Martorell et al., 2019). We recorded kainate (KA)- and carbachol (Cch)-induced gamma oscillations within mid-ventral hippocampal slices, as well as PVIN spiking activity during KA, from young and old, 5xFAD and control mice. Brain slices were then processed for Aβ, PV and perineuronal net (PNN) quantification using immunohistochemistry. Our findings do not support a clear relationship between hippocampal plaque presence and impaired gamma oscillatory network activity as a result of PVIN dysfunction. Plaque formation developed as previously described for this model (Oakley et al., 2006), with minimal hippocampal plaque presence at 3–4 months progressing to a much higher plaque burden by 6–12 months throughout the hippocampus. However, despite extensive tissue pathology only KA- and not Cch-induced gamma frequency oscillations in the oldest 5xFAD mice were found to be significantly impaired. PVIN firing during KA was not different between 5xFAD and control mice, with the only differences observed between older and younger animals. Thus, the link between plaque pathology and network dysfunction is more complex than previously thought and this model demonstrates a surprising resilience against plaque pathology with regard to PVIN function and network rhythmogenesis.
Materials and Methods
Animals
All experiments were conducted in accordance with animal protocols approved by the National Institutes of Health.
For all electrophysiology and immunohistochemistry experiments, both male and female transgenic 5xFAD mice were used [Jax MMRRC stock #34840, B6SJL-Tg (APPSwFlLon, PSEN1*M146L*L286V) 6799Vas]. Three to four month-old animals were used for the “young” timepoint. These were chosen due to the minimal hippocampal plaque development present at this stage (Oakley et al., 2006). For the “old” timepoints, 12–19-month-old animals were used (except with cell-attached, patch-clamp experiments where 10–13-month-old animals were used). At this stage, plaque deposition was extensive throughout the cortex and hippocampus. All animals were group-housed in individually ventilated cages kept at room temperature with a 12 h/12 h light/dark cycle and provided with food and water ad libitum.
All control experiments were done using littermate controls or PV-tdTomato mice for patching experiments. To label PV cells for targeted patch clamp experiments, 5xFAD mice were crossed with PV-tdTomato mice maintained on a C57BL/6 background [Stock #027395, C57BL/6-Tg (Pvalb-tdTomato) 15 Gfng/J]. Mouse genotype was confirmed pre and post experiment by tail biopsy using Transnetyx genotyping services.
Antibodies
Mouse anti-PV (1:5,000; Sigma-Aldrich, MO, USA Cat# P3088, RRID:AB_477329), mouse anti-beta amyloid (1:1,000; BioLegend, CA, USA Cat# 803004, RRID:AB_2715854) were used as indicated below. For PNN labelling biotinylated WFA (1:500, Vector Laboratories, CA, USA Cat#B-1355-2) was used as a primary and, Streptavidin Alexa 488 or 555 was used as a secondary (1:1,000; Thermo Fisher Scientific, MA, USA). Similarly, for the PV and beta amyloid, secondary antibodies were conjugated with Alexa Fluor dyes 488 or 555 (1:1,000; Thermo Fisher Scientific, MA, USA).
Immunohistochemistry (IHC) on Drop Fixed Tissue
All immunohistochemical staining was performed on hippocampal slices used for the electrophysiology experiments. When the experimental recordings finished, slices (350 μm) were taken from the interface chamber and immediately drop fixed in 4% PFA for 4–24 h before being transferred to a 30% sucrose solution for >24 h. The slices were then re-sectioned using a freezing microtome to 70 μm and stored in a cryoprotective solution (25% glycerol, 30% ethylene glycol in PBS) at −20°C until required.
For immunostaining, sections were rinsed in PBS at room temperature then blocked for 2 h in 10% normal goat serum with 0.5% Triton X-100 then incubated in the primary antibody at 4°C for 24–48 h. Sections were then rinsed with PBS and incubated in secondary antibodies (1:1,000) for 2 h at room temperature. All antibodies were diluted in a carrier solution consisting of PBS with 1% BSA, 1% normal goat serum, and 0.5% Triton X-100. Sections were then rinsed, mounted on Superfrost glass slides, and cover slipped using prolong gold antifade mounting medium (Thermo Fisher Scientific; Cat# P-36931) and 1.5 mm cover glasses.
Image Acquisition and Analysis
Confocal images were taken using a Zeiss 780 confocal microscope. Amyloid plaque density analysis was done using Imaris software (Oxford Instruments, RRID:SCR_007370). Plaque density was measured using the surfaces tool in Imaris on stitched, Z-stack images which were segmented into regions containing either the CA1 subfield or the dentate/CA3 with reference to the Paxinos and Franklin mouse brain atlas (Franklin and Paxinos, 2008). Plaque density was quantified as the volume of plaques per volume of analysed region and presented as a percentage density within CA1 or dentate/CA3. Quantitative analysis of PNN and PV+ cell density in the hippocampus was performed using ImageJ software (NIH, Bethesda, MD, USA, RRID:SCR_003070). The number of PV cells with/without PNNs, and PNNs without PV cells were counted and again normalised to the volume of the inspected region.
Slice Preparation and Electrophysiology
Mice were anaesthetised with isoflurane until pedal withdrawal reflex was lost and then immediately decapitated. The brains were dissected in ice-cold sucrose artificial cerebrospinal fluid (SaCSF) containing the following (in mM): 90 sucrose, 80 NaCl, 3.5 KCl, 24 NaHCO3, 1.25 NaH2PO4, 4.5 MgCl2, 0.5 CaCl2, and 10 glucose, saturated with 95% O2 and 5% CO2. Horizontal brain slices (350 μm) were cut to obtain transverse sections of the mid-ventral hippocampus using a VT-1200S vibratome (Leica Microsystems, Wetzlar, Germany) and incubated in an interface-style chamber in normal aCSF solution containing (in mM): 130 NaCl, 3.5 KCl, 24 NaHCO3, 1.25 NaH2PO4, 2 MgCl2, 2 CaCl2 at 32–34°C for 30 min and then maintained at room temperature until use. This area was chosen for our experiments as it better preserves the structural hippocampal connectivity after slicing, thus enabling the generation of more robust gamma network activity than sections from the dorsal hippocampus (Steullet et al., 2010; Whitebirch, 2020). Slices were incubated for at least 1 h before conducting electrophysiological recordings.
For gamma oscillation experiments, all slices were transferred to a modified interface-style recording chamber (BSC-1, Scientific Systems design Inc., ON, Canada) and perfused with aCSF at 1.5–2 ml/min, 32–34°C. The field potentials were recorded using borosilicate glass micropipettes (World Precision Instruments, FL, USA) pulled to a resistance of 3–5 MΩ using a vertical pipette puller (Narishige, PP-830) and filled with aCSF and placed in CA3 stratum pyramidale and CA1 stratum radiatum. Signals were acquired at 10 kHz using a MultiClamp 700 A amplifier, filtered at 3 kHz (Molecular Devices, CA, USA), digitised using a Digidata1322A (Molecular Devices, CA, USA), and captured on a computer running pClamp9.2 (Molecular Devices, CA, USA). After a baseline of 5 min, gamma oscillations were induced by 10 μM carbachol (Sigma, MO, USA) or 400 nM kainate (Sigma, MO, USA cat# K0250).
For cell-attached recordings of PV cells, slices were transferred to a submerged recording chamber (Warner Instruments, CT, USA) with an upright microscope (Zeiss Axioskop) placed between two custom-made harps to allow perfusion above and below the slice with aCSF at 2–3 ml/min at 32°C. Similar to the interface recording set-up, the field potential was recorded from CA3 stratum pyramidale with a glass micropipette (3–5 MΩ). Individual PV cells were visualised using a 40× objective using fluorescence and IR-DIC camera microscopy. PV+ cells were located within the CA3 region and cell-attached recordings were made using 3–5 MΩ resistance microelectrodes filled with aCSF. Recordings were made using a Multiclamp 700 A amplifier (Molecular Devices, MO, USA), and signals were digitised at 10 kHz (Digidata 1322A, filtered at 3 kHz) for collection on a PC computer equipped with pClamp 9.2 or 10.4 software (Molecular Devices, CA, USA RRID:SCR_011323) Gamma oscillations were induced in submerged recording configuration using application of 100 nM KA (Sigma, MO, USA cat# K0250).
Data Analysis
Pre-processing of electrophysiology data was done in Clampfit 10.5/11.2 (Molecular Devices, RRID:SCR_011323).
For gamma oscillations recorded from the interface chamber, all analysis was performed during the first 5 min during washout (where oscillations yielded the greatest power) after at least 30 min of drug application to allow time for the oscillation to build up. The signals were bandpass filtered between 5 and 120 Hz and then 10 s epochs were used for calculating the power spectrum, autocorrelation, and cross-correlation between CA3 and CA1. Slices were only included for analysis if the frequency at peak power (calculated from the power spectrum analysis) was >19 Hz and <80 Hz. Similarly, recordings were only included for analysis if the peak gamma power was >1 × 100 μV2/Hz for CA3 and >1 × 10−1μV2/Hz for CA1. Recordings with significant mains noise contamination (~60 Hz) were also discarded. Peak gamma power, frequency at peak power, and bandwidth power (between 20 and 80 Hz) were extracted from power spectrum output. Although the frequency range for gamma oscillations is normally reported between 30 and 90 Hz, the optimal recording temperature for our experimental set-up was 5–7°C lower than body temperature and due to the linear relationship between recording temperature and oscillation frequency, we shifted the limits for our bandwidth power calculations to 20–80 Hz to account for this (Dickinson et al., 2003; Schneider et al., 2015). Cross-correlation peak and cross-correlation delay between CA3 and CA1 were obtained from the Clampfit cross-correlation output. The rhythmicity of the gamma oscillations was determined by calculating the coefficient of rhythmicity from the autocorrelograms (Cangiano and Grillner, 2003; Balleza-Tapia et al., 2018). Briefly, the value was defined as Cr = (α−β)/(α+β) where α and β are the height of the second peak and the first trough in the normalised autocorrelogram, respectively. The values ranged from 0 to 1 with all values for our data found to be ≥0.01 and therefore considered rhythmic. The higher the Cr values the better the quality of the oscillations.
Pre-processing of PVIN spike data was done using the threshold detection tool in Clampfit. Three minute epochs were selected for analysis at baseline, during kainate, and in drug washout. Spike analysis from the threshold detection was exported along with the respective section of the abf file trace and used for phase timing analysis in MATLAB using custom-written code (Caccavano et al., 2020). For slices that developed ictal-like activity, abf files were analysed using MATLAB to examine PVIN spike half-width and frequency during interictal bursting.
Statistical Analysis
All data were assessed for normality and then tested with appropriate parametric or non-parametric tests using GraphPad Prism (GraphPad Prism 9.1.0, RRID:SCR_002798) or MATLAB (MATLAB R_2020, RRID:SCR_001622). Where data displayed lognormal distribution, they were log-transformed prior to running any statistical tests. Plots are represented as box and whisker plots with the median, 25th–75th percentile, and range of data shown. Several results are presented in a two-way design, with age and genotype as the main factors. These were analysed by a two-way ANOVA including the interaction. For consistency between endpoints, the post-hoc tests were kept consistent and based on a priori hypotheses (specifically: young control vs. young 5xFAD, old control vs. old 5xFAD, old control vs. young control, and old 5xFAD vs. young 5xFAD). For transparency, all results from the two-way ANOVA and post-hoc tests are presented in the Supplementary Stats File. PVIN firing data were analysed by fitting a mixed model, as implemented in GraphPad Prism 9.0, instead of a repeated measures ANOVA which cannot handle missing values. This mixed model used a compound symmetry covariance matrix fit using Restricted Maximum Likelihood (REML). Circular data from the phase-locking analysis were analysed in MATLAB. First-order cellular data were tested for non-uniformity using Raleigh’s test to determine the significance of phase-locking with the Circular Statistics Toolbox for MATLAB (RRID:SCR_016651; Berens, 2009). Second-order circular data of the population of cells (including those not significantly phase-locked) were analysed with a custom MATLAB implementation of Hotelling’s and Batschelet’s methods to compute the weighted mean vector and significance of one and two-sample means (Sections 26.9, 27.9, 27.11 of Zar, 2010). All data points on box plots indicate the individual or average values for a single slice or single cell. P values displayed in plots in bold or with * indicate significance. *p < 0.05, **p < 0.01, ***p < 0.001 and ****p < 0.0001. Figures were made in MATLAB, GraphPad Prism, and Inkscape (Inkskape 1.0, RRID:SCR_014479).
Results
Plaque Presence in 5xFAD Model
5xFAD mice display accelerated Aβ plaque development with extracellular deposits, appearing in deep cortical layers and subiculum by 2 months, and spreading to other brain regions, including the hippocampus, by 4 months (Oakley et al., 2006). We confirmed the presence of extracellular Aβ deposits at 3 and 4 months within the cortex and subiculum as well as some minor plaque deposition in the hippocampus (Figure 1). Whilst labelling of Aβ deposits was seen throughout the subiculum at 3–4 months, within the hippocampus labelling was sparser, with no deposits in the pyramidal and granule cell layers and most plaque presence occurring in the surrounding layers e.g., stratum oriens and stratum radiatum of CA1 and CA3, and the hilar region of the dentate (Figure 1A). At 6 months, plaque density significantly increased, particularly in the CA1 subfield (Figures 1B,D). In tissue from animals 12 months or older, Aβ plaque deposition occurred throughout the hippocampal formation with plaques encroaching on the previously spared granule and pyramidal cell layers (Figures 1C,D).
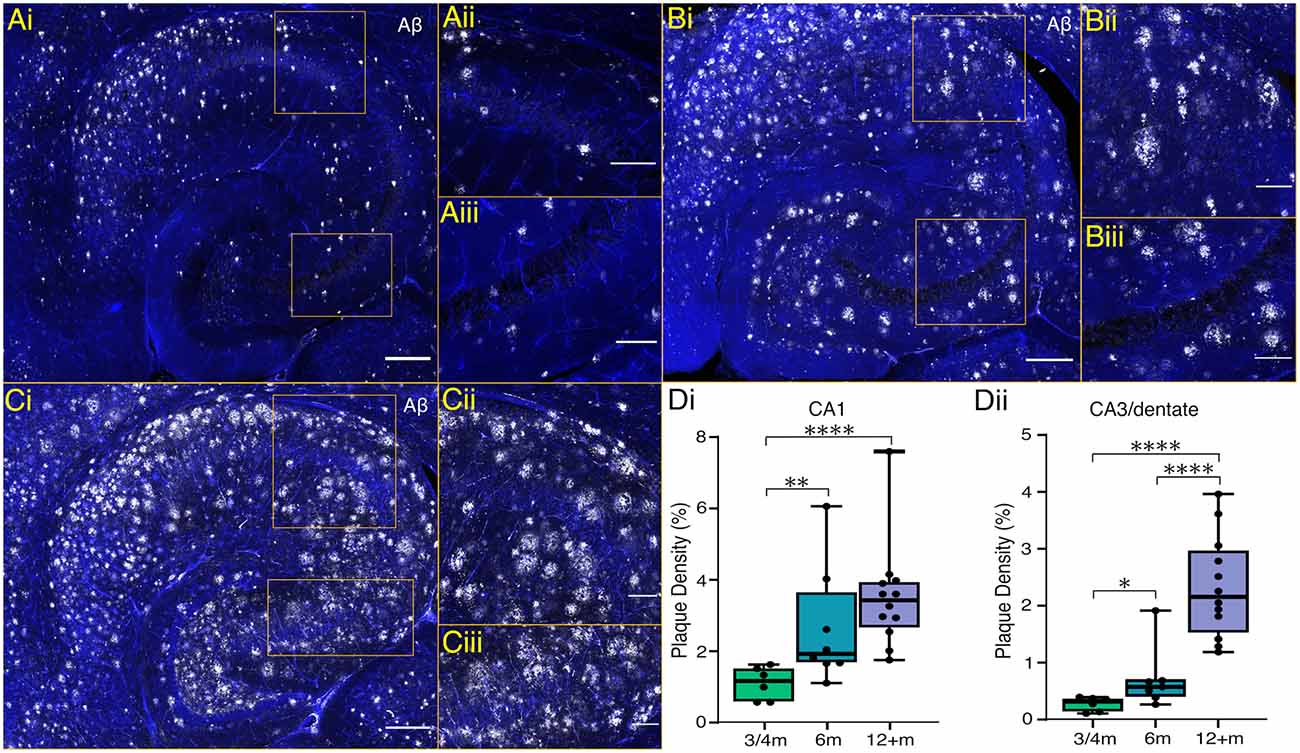
Figure 1. Hippocampal plaque development in 5xFAD mice. (Ai,Bi,Ci) Representative maximum intensity projection images of ventral hippocampal slices processed with an anti-amyloid beta antibody (Aβ; white) and DAPI stain (blue) from 4, 6 and 12-month-old mice, respectively (scale bar = 250 μm). (Aii,Bii,Cii) Enlarged sections of CA1 plaque presence and (Aiii,Biii,Ciii) of CA3 (scale bar = 100 μm). (D) The plaque densities as a percentage of measured volumes are shown from CA1 (Di) and CA3/dentate (Dii) across timepoints. Box plots show the median as a black line, boxed region as the interquartile range, and whiskers as the range with individual points representing data from one brain slice. 3–4 m: n = 6 slices from four mice (two female); 6 m: n = 8 slices from four mice (two females); 12+ m: n = 12 slices from six mice (three females). *p < 0.05, **p < 0.01, ****p < 0.0001 (one-way ANOVA with Šidák correction).
Carbachol (Cch)-Induced Gamma Frequency Oscillations
To study the effect of developing plaque presence on network activity within the hippocampus, we compared gamma frequency oscillations in ex vivo brain slices from 5xFAD and control mice (Figure 2A). This form of rhythmic activity is commonly induced by the perfusion of the cholinergic agonist, carbachol (Cch), which yields persistent oscillatory activity around 40 Hz within CA3, and propagates to CA1 (Fisahn et al., 1998). Figure 2 shows that the application of 10 μM Cch successfully induced CA3 and CA1 gamma frequency oscillations in young and old control and 5xFAD brain slices (Figures 2B,C). The peak CA3-recorded gamma frequency did not differ between control slices and 5xFAD brain slices from young or old mice (Figure 2Di). Gamma power (Figure 2Ei) and bandwidth power (Figure 2F) were also not different between control and 5xFAD brain slices, however, the two-way ANOVA showed significant age effects with post-hoc tests indicating significant increases in CA3 power and bandwidth power in older 5xFAD brain slices (Figures 2Ei,Fi).
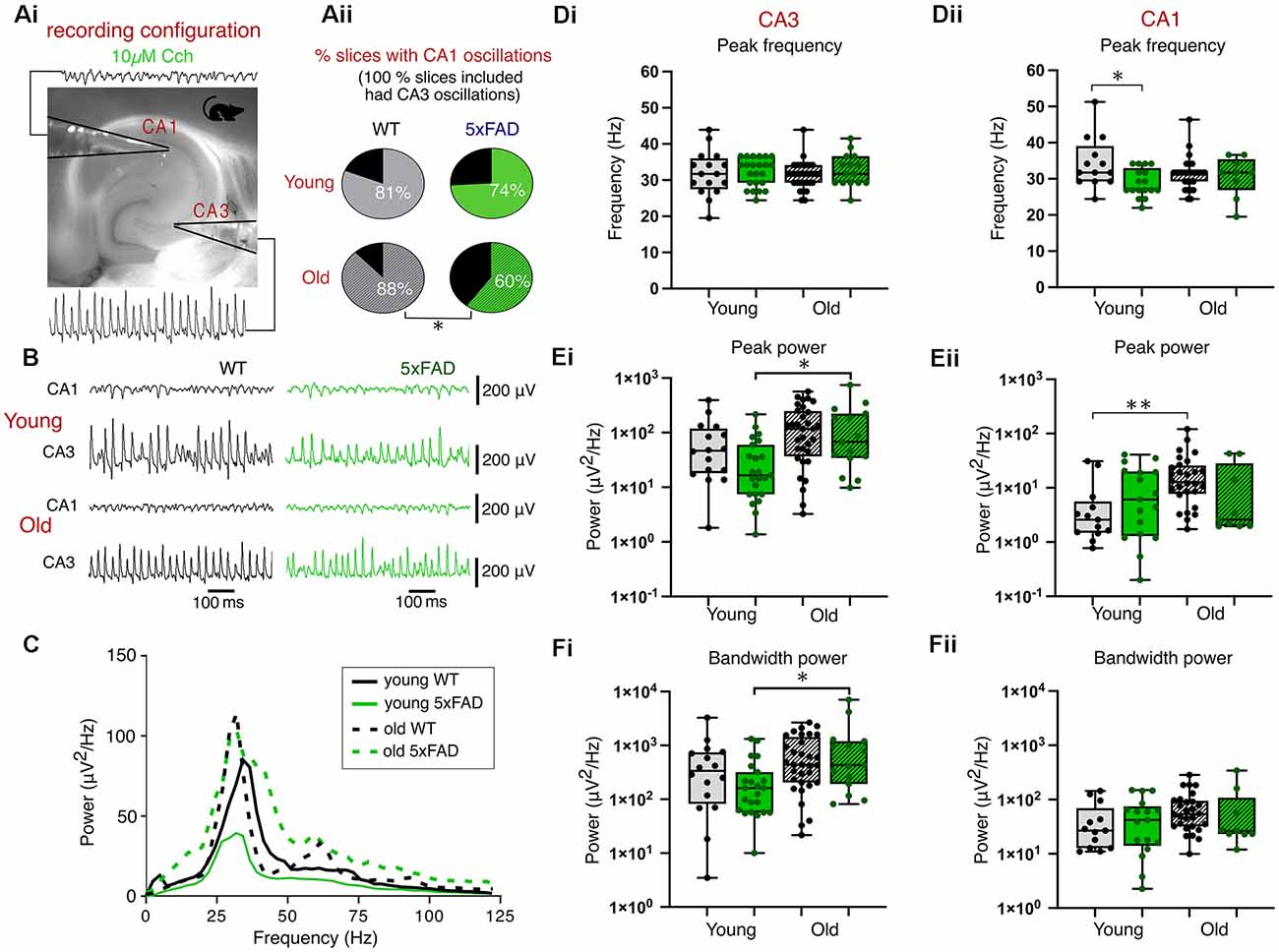
Figure 2. Carbachol-induced gamma oscillations. (Ai) Experimental configuration used for recording gamma oscillations in an interface recording chamber. (Aii) Pie charts indicating the percentage of slices with coincident CA1 and CA3 gamma oscillations (all slices included in analysis exhibited CA3 oscillations). (B) Representative traces of carbachol-induced gamma oscillations. (C) Average CA3 power spectrums of young and old, control and 5xFAD slices during carbachol-induced oscillations. (D,E,F) Group data boxplots of the peak frequency, peak gamma power and bandwidth power respectively from young and old, control and 5xFAD animals in CA3 (Di,Ei,Fi) and CA1 (Dii,Eii,Fii). Box plots show the median as the black line, the boxed region as the interquartile range, and the whiskers as the range with each data point representing a single slice. Young WT: n = 16/13 (CA3/CA1), eight mice (three females); Young 5xFAD: n = 23/17 (CA3/CA1), eight mice (four females); Old WT: n = 32/28 (CA3/CA1), six mice (one female); Old 5xFAD: n = 15/9 (CA3/CA1), five mice (two females). *p < 0.05, **p < 0.01 (Aii: χ2 test; D,E,F: two-way ANOVA with Šidák correction for multiple comparisons).
The presence of cholinergically-induced gamma oscillations in the CA1 is dependent upon intact circuitry and communication from CA3 via the Schaffer collaterals and so we also recorded from CA1 stratum radiatum, where it is possible to detect gamma oscillations, albeit at lower power than those of CA3 (Fisahn et al., 1998; Zemankovics et al., 2013). All slices used in our analysis exhibited CA3 gamma oscillations, however, we found a difference between WT and 5xFAD mice in the proportion of slices which exhibited coincident CA1 gamma oscillations. In slices from younger mice, 82% of control slices compared with 74% of 5xFAD slices had both CA3 and CA1 gamma frequency activity (p = 0.59, χ2 test; Figure 2Aii). In slices from the older cohort of mice, this difference was more pronounced with 88% of control slices exhibiting gamma oscillations in both CA3 and CA1 as opposed to only 60% of 5xFAD slices (p = 0.03, χ2test; Figure 2Aii). Despite differences in the proportion of slices exhibiting coincident CA1 gamma frequency oscillatory activity between 5xFAD and control tissue, in slices that did show CA1 gamma oscillations, the results of the two-way ANOVA showed no significant effect of genotype on CA1 power (Figure 2Eii) or bandwidth power (Figure 2Fii). There was a significant effect of genotype on CA1 frequency, which after post-hoc tests showed a significant decrease in peak gamma frequency in young 5xFAD slices (Figure 2Dii). The two-way ANOVA also showed a significant age effect for CA1 gamma power which, after post-hoc tests, revealed a significant increase in CA1 power in older compared to young WT slices (Figure 2Eii).
Rhythmicity and Synchronicity of Cch-Induced Gamma Oscillations
We calculated the coefficient of rhythmicity for CA3 gamma oscillations in young and old 5xFAD and control tissue to investigate the quality of the generated gamma oscillations (see Section “Materials and Methods”). The average CA3 autocorrelograms for each condition are shown in Figure 3A and despite a significant age-effect indicated by the two-way ANOVA, post-hoc tests revealed no differences in rhythmicity between young and old, control or 5xFAD oscillating brain slices (Figure 3B).
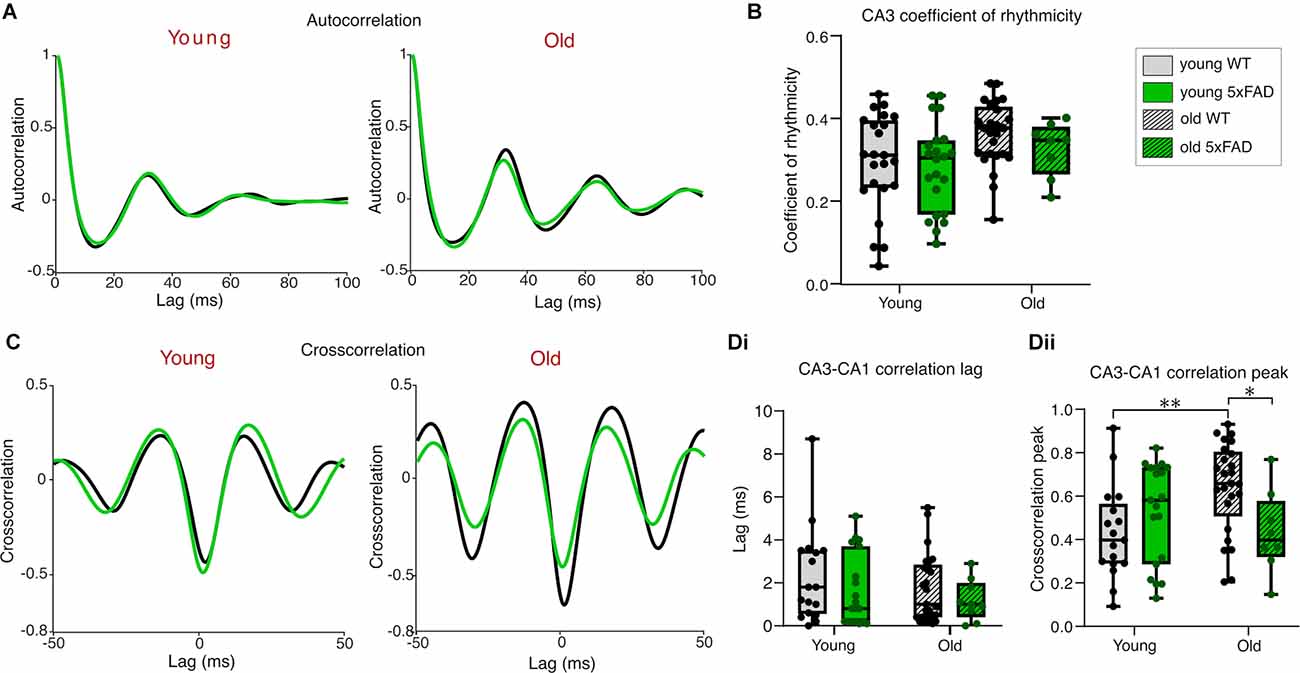
Figure 3. Rhythmicityof carbachol-induced gamma oscillations. (A) Mean autocorrelograms from CA3 recorded gamma oscillations. (B)Boxplots of the calculated coefficient of rhythmicity of the oscillations (see Section “Materials and Methods” for details of calculation). (C) Mean cross correlograms of CA3-CA1. (D) Group data cross correlation lags (Di) and peaks (Dii) from CA3 to CA1. Young WT: n = 23/17 (A,B/C,D), eight mice (three females); Young 5xFAD: n = 23/19 (A,B/C,D), eight mice (four females); Old WT: n = 25/25 (A,B/C,D), six mice (one female); Old 5xFAD: n = 8/9 (A,B/C,D), five mice (two females). *p < 0.05, **p < 0.01 (two-way ANOVA with Šidák correction for multiple comparisons).
The precise coordination of activity between different subregions of the hippocampus is crucial for proper information transfer and memory consolidation (Kanak et al., 2013). For this reason, we examined the cross-correlation between CA3 and CA1 in the young and old 5xFAD and control mouse tissue (Figures 3C,D). The phase lags measured from the cross-correlation peaks were no different between young and old, or control and 5xFAD slices (Figure 3Di), suggesting that the Schaffer collateral pathway is not selectively impaired in 5xFAD slices from young or old mice. A two-way ANOVA comparing the peak cross-correlation values between CA3 and CA1 showed a significant interaction effect with post-hoc testing revealing higher cross-correlation in older compared to younger WT slices. This age-related increase in CA3-CA1 coordination was not seen in the 5xFAD brain slices, where post-hoc testing showed significantly lower peak cross correlation than in age-matched WT brain slices (Figure 3Dii). This decrease in the peak cross-correlation in older 5xFAD slices, without any genotype difference in the propagation delay between CA3 and CA1, may reflect impaired post-synaptic responsiveness or synchronicity within CA1 to CA3 rhythmic input despite intact Schaffer collateral afferents in the 5xFAD tissue.
Kainate (KA)-Induced Gamma Frequency Oscillations
Despite extensive plaque burden at 12 months and older, 5xFAD slices exhibited remarkable resilience in their ability to generate robust Cch-induced gamma oscillations, with only modest impairments in rhythmicity and CA1 participation. There are many ways of inducing gamma frequency oscillations in brain slices, all of which function to increase excitability within pyramidal cells and/or interneurons (Fisahn, 2005). However, different methods work through specific targets and exhibit distinct properties. For example, while cholinergically-induced oscillations work through targeting M1 muscarinic acetylcholine receptors and require AMPA and GABAA receptors (Fisahn et al., 1998, 2002), glutamatergically-induced gamma frequency oscillations, using kainate (KA), require KA and GABAA receptors but not AMPARs (Fisahn et al., 2004; Fisahn, 2005). This demonstrates that whilst different pharmacological methods can produce similar network activity, they do so through different mechanisms and possibly involving different cell ensembles. Therefore, we next investigated if the network resiliency of 5xFAD mice extends to KA-induced gamma oscillations.
Notably, the application of 400 nM KA-induced higher power gamma oscillations than Cch in all conditions (Figures 4B,C). The frequency of CA3 gamma oscillations in KA was no different between control and 5xFAD, or between age groups (Figure 4Di). Similarly, the CA3 power and bandwidth power did not differ between any groups (Figures 4Ei,Fi). However, in contrast to oscillations induced by Cch, KA-induced oscillations showed significant genotype effects for CA3 power and CA3 bandwidth power. After post-hoc testing, this revealed significant reductions in CA3 power and bandwidth power in older 5xFAD brain slices compared to controls (Figures 4Ei,Fi).
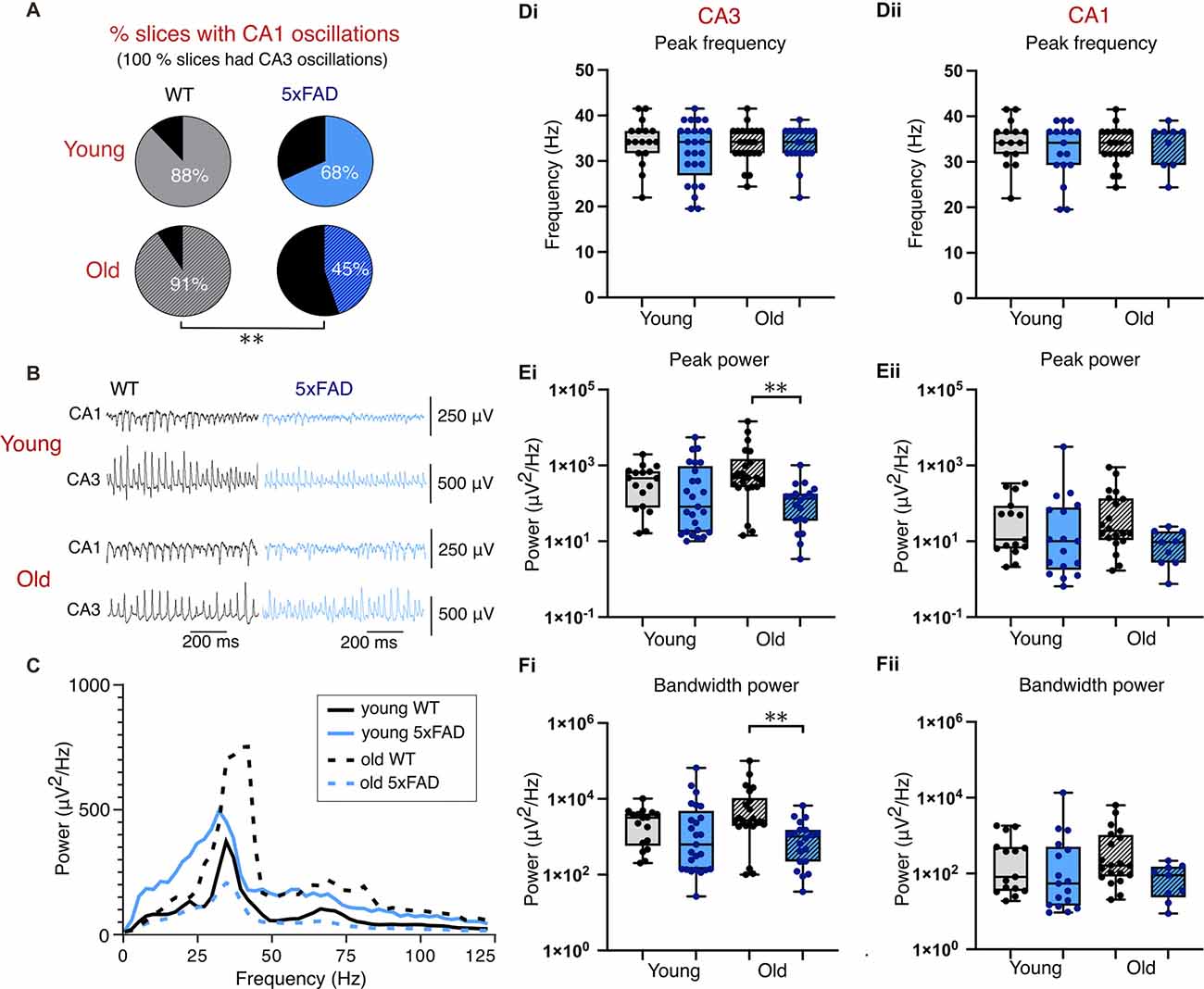
Figure 4. Kainate-induced gamma oscillations. (A) Pie charts indicating the percentage of slices with coincident CA1 and CA3 oscillations (all slices included in analysis exhibited CA3 gamma oscillations). (B) Representative traces of kainate-induced gamma oscillations. (C) Average CA3 power spectrums of young and old, control and 5xFAD slices during kainate-induced oscillations. (D,E,F) Group data boxplots of the peak frequency, peak gamma power and bandwidth power, respectively, from young and old control and 5xFAD animals in CA3 (Di,Ei,Fi) and CA1 (Dii,Eii,Fii). Each data point represents a single slice. Young WT: n = 17/15 (CA3/CA1), eight mice (three female); Young 5xFAD: n = 25/17 (CA3/CA1), eight mice (four females); old WT: n = 22/20 (CA3/CA1), eight animals (three females); old 5xFAD: n = 20/9 (CA3/CA1), seven mice (three females). **p < 0.01 (A: χ2 test; D,E,F: two-way ANOVA with Šidák correction for multiple comparisons).
Once again, 100% slices used in the analysis exhibited CA3 gamma oscillations and we found that, as with slices exposed to Cch, young and old 5xFAD brain slices exposed to KA displayed lower CA1 participation in gamma frequency oscillations (Figure 4A). In slices from younger mice, 88% of control slices compared with 68% of 5xFAD slices had coincident CA3 and CA1 gamma oscillatory activity (p = 0.085, χ2 test; Figure 4A). In slices from the older cohort of mice, the difference in CA1 participation was even more substantial with 91% of control slices exhibiting gamma oscillations in both CA3 and CA1 as opposed to only 45% of 5xFAD slices (p = 0.001, χ2 test; Figure 4A). Despite lower CA1 participation in 5xFAD brain slices, those which did exhibit CA1 gamma oscillations exhibited no difference in frequency, power, or bandwidth power after post-hoc testing, despite a significant effect of genotype in CA1 power (Figures 4Dii,Fii).
Rhythmicity and Synchronicity of KA-Induced Gamma Oscillations
Figure 5A shows the average autocorrelograms for KA-induced gamma oscillations in control and 5xFAD slices, for young and old mice. The coefficient of rhythmicity (Cr) was similar across age groups; however, a significant genotype effect showed the Cr in young 5xFAD slices to be significantly lower compared to controls after post-hoc testing (Figure 5B).
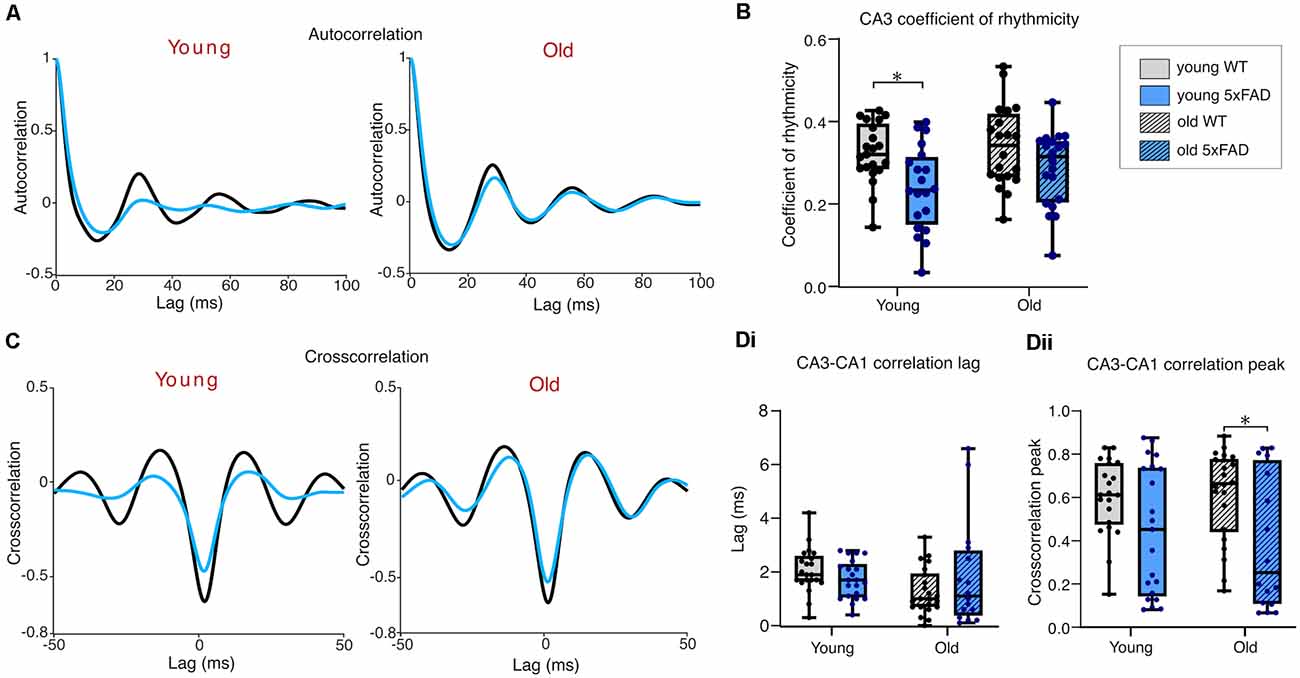
Figure 5. Rhythmicity of kainate-induced gamma oscillations. (A) Mean autocorrelograms from CA3 recorded gamma oscillations. (B) Boxplots of the calculated coefficient of rhythmicity of the oscillations. (C) Mean crosscorrelograms of CA3-CA1. (D) Group data cross correlation lags (Di) and peaks (Dii) from CA3 to CA1. Young WT: n = 21/21 (A,B/C,D), eight mice (three females); Young 5xFAD: n = 20/21 (A,B/C,D), eight mice (four females); old WT: n = 20/22 (A,B/C,D), eight mice (three females); old 5xFAD: n = 20/16 (A,B/C,D), seven mice (three females). *p < 0.05 (two-way ANOVA with Šidák correction for multiple comparisons).
Cross-correlation phase lag between CA3 and CA1 was not different between 5xFAD and control in either young or old brain slices (Figures 5C,Di). However, there was a significant genotype effect on peak cross-correlation, with old 5xFAD slices exhibiting significantly reduced CA3-CA1cross-correlation compared to controls, after post-hoc testing (Figure 5Dii). In summary, our KA-induced gamma findings reveal poorer quality CA3 gamma oscillations and less CA1 participation, and at later stages, impaired synchrony between CA3 and CA1 and compromised gamma power in 5xFAD mice.
PVIN Firing Properties During KA-Induced Gamma Oscillations
As outlined above, the generation and coordination of gamma frequency oscillations are critically dependent upon the activity of fast-spiking PV-expressing interneurons (PVINs). Thus, based on the deficits observed in KA-induced oscillations in 5xFAD slices, we chose to investigate PVIN function during KA-induced gamma oscillations in slices from young and old, 5xFAD, and control mice.
In submerged slices (see Section “Materials and Methods” and Hájos et al., 2009), application of 100 nM KA initiated gamma oscillations and results from the mixed model analysis and post-hoc testing showed significantly increased PVIN firing frequency in all groups upon kainate application (Figures 6A,B). This analysis also showed no effect of genotype on firing frequency (Figure 6Ci). Similarly, the results from two-way ANOVAs showed no genotype effect for inter-spike interval (ISI) or PVIN spike half width during KA-induced gamma oscillations (Figure 6Cii,iii). Interestingly, despite no observed genotype-specific differences in the behaviour of PVIN firing during KA application, the mixed model analysis revealed a significant effect of age as well as condition on PVIN firing frequencies. There was a non-significant trend for older PVINs from both genotypes to fire at higher frequencies during KA application, and a failure to return to lower levels of firing during the washout period, which was significantly different between young and old 5xFAD slices after post-hoc tests (Figures 6B,Ci). The inter-spike interval also showed a significant effect of age during kainate application, with a significant reduction in old compared to young 5xFAD PVINs after post-hoc tests (Figure 6Cii).
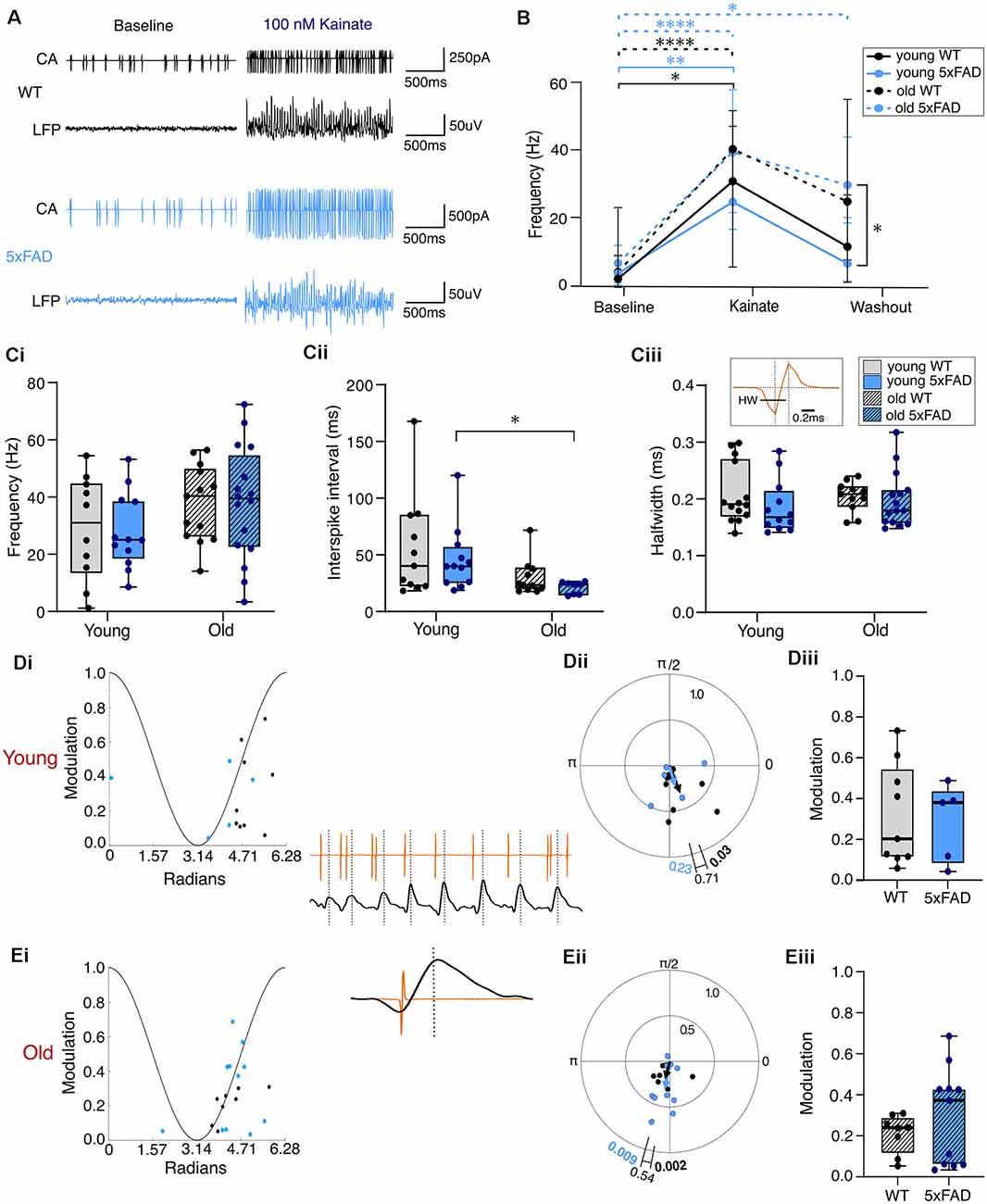
Figure 6. PVIN firing properties during kainate-induced gamma oscillations. (A) Representative traces of simultaneously recorded PVIN firing (cell-attached, CA, recordings) and extracellular CA3 local field potential (LFP) in baseline and then during kainate application. (B) Progressive change in PVIN firing frequency during the wash in and wash out of kainate plotted as the median with error bars indicating the 95% confidence interval, solid line for young, and dashed line for old data. (Ci,Cii,Ciii) Spike frequency, inter-spike interval, and spike halfwidth of PVINs during kainate, respectively. The inset in (Ciii) shows a representative PVIN action potential with the halfwidth shown with scale bar for reference. (D,E) Phase-locking of the PVIN spikes in relation to the gamma oscillation with the peak of the cycle at 0 and trough at π in young (D) and old slices (E). The angles of individual data points in (Di) and (Ei) represent the mean phase angle for a single PV cell. The vector length/modulation is a value between 0 and 1 indicating the degree of phase-locking with a value of 1 meaning the cell always fires at the same phase and a value of 0 meaning the cell fires randomly with no phase-locking. The lines on the polar plots (Dii,Eii) indicate the weighted average degree of phase locking for the population of cells (see Section “Materials and Methods” for details). The p values shown at the end of each line on the polar plot indicate the results from Hotelling’s test of one-sample weighted means and the p-value shown above the bracket indicates the result of Batschelet’s test between the group population means. The insets show a representative trace of PVIN phase-locked spiking (in orange) to the oscillating LFP (black) with an enlarged view of a single phase-locked PVIN spike shown below. (Diii,Eiii) Box plots of the population data of the strength of phase modulation (median = black line, boxed region = interquartile range, and whiskers = full range). (B,C) Young WT: n = 10 cells, four mice (two female); Young 5xFAD: n = 12 cells, three mice (all males); Old WT: n = 13 cells, four mice (all males); Old 5xFAD: = 16 cells, three mice (two females). (D,E) Young WT: n = 9 cells, three mice (one female); Young 5xFAD: n = 5 cells, two mice (all males); Old WT: n = 8 cells, three mice (all male); Old 5xFAD: n = 11 cells, three mice (two females). *p < 0.01, **p < 0.01, ****p < 0.0001 [for (B) we used Repeated-measures Mixed-model approach with Šidák correction for multiple comparisons; (C,D) used two-way ANOVA with Šidák correction for multiple comparisons].
Phase-Locking of PVINs During Kainate-Induced Gamma Oscillations
As the KA-induced gamma deficits in 5xFAD brain slices appear unrelated to differences in the overall firing rates of PVINs, we probed for differences in phase-locking of PVINs to the gamma rhythm. Impaired phase-locking of fast-spiking interneurons (FSINs) to gamma frequency oscillations has been reported in APPNL-G-F AD mice prior to plaque formation as well as in FSINs after exposure to Aβ42 application (Andrade-Talavera et al., 2020; Chung et al., 2020; Arroyo-García et al., 2021). Furthermore, recordings at reciprocal PC-PVIN synapses show both reduced EPSCs onto PVINs and reduced IPSCs and increased paired pulse depression at PVIN-PC synapses after treatment with Aβ1–42 oligomers (Chung et al., 2020; Park et al., 2020).
Despite this evidence of Aβ-induced synaptic disruption at both PC-PVIN and PVIN-PC synapses, we did not find any noticeable deficit in PVIN-gamma phase-locking in brain slices from 5xFAD mice. Of the subset of PVIN recordings with detectable LFP gamma oscillations (submerged recording configuration), almost all PVINs fired with an average phase corresponding to the rising phase of the gamma cycle (Figures 6D,E). A first order analysis of PVIN spiking activity revealed significant phase-locking for most cells (young control: eight of nine; young 5xFAD: five of five; old control: seven of eight; old 5xFAD: nine of 11; p < 0.05, Raleigh’s test). A second order analysis of the population found significant gamma phase-locking of PVINs from young control mice at 5.070 rad/290° (p = 0.0266, Hotelling’s test), but no significant phase-locking of PVINs from young 5xFAD brain slices, which displayed an average phase of 4.958 rad/284° (p = 0.2349, Hotelling’s test). However, this is likely related to low sampling in the mutant rather than a real-genotype related loss of the ability of PVINs to significantly phase-lock to gamma. There was no genotype difference between the mean phase angles (p = 0.7123, Batschelet’s test; Figure 6Dii). Older PVINs from both control and 5xFAD brain slices were significantly phase-locked to the gamma oscillation (Control = 4.450 rad/255°; p = 0.0085, Hotelling’s test. 5xFAD = 4.539 rad/260°; p = 0.0221, Hotelling’s test). There was no genotype difference between the mean phase angles (p = 0.5402, Batschelet’s test; Figure 6Eii). There was also no significant difference between young or old 5xFAD and control PVINs with regards to the strength of modulation (Figures 6Diii,Eiii). However, the modulation variance was significantly greater in PVINs from older 5xFAD mice (p = 0.0242, F(10, 7) = 6.19), which could potentially point to differential alterations in PVIN subpopulations. To further explore this, a Kolmogorov-Smirnov analysis of the strength of modulation was conducted but revealed no significant alterations in the underlying distributions in either young (p = 0.9461, D = 0.244) or old (p = 0.1271, D = 0.5455) mice.
KA-Induced Hyperexcitability
Network hyperexcitability is commonly comorbid with AD (Bakker et al., 2012; Palop and Mucke, 2016; Ambrad Giovannetti and Fuhrmann, 2019). Likewise, different AD models exhibit network hyperexcitability and increased predisposition to ictal-like activity (Busche et al., 2008; Sanchez et al., 2012; Verret et al., 2012; Siwek et al., 2015; Ciccone et al., 2019). We were therefore interested in evaluating any differences between 5xFAD and control slices in their propensity for hyperexcitability. A number of slices from the older mice developed ictal-like activity during KA application in the submerged recording configuration (Figures 7A,B). There was no difference in the percentage of slices that developed ictal-like activity (3/10 of WT recordings, 30%; 3/9 of 5xFAD recordings, 33.3%). There was also no significant difference in the PVIN firing frequency or spike half width during interictal events (IIEs), despite a trend towards lower frequency firing in the 5xFAD PVINs. However, this could also be due to the low sampling size, as only a subset of the slice recordings exhibiting ictal-like activity had paired PVIN activity and could be used for analysis of IIE-spike properties.
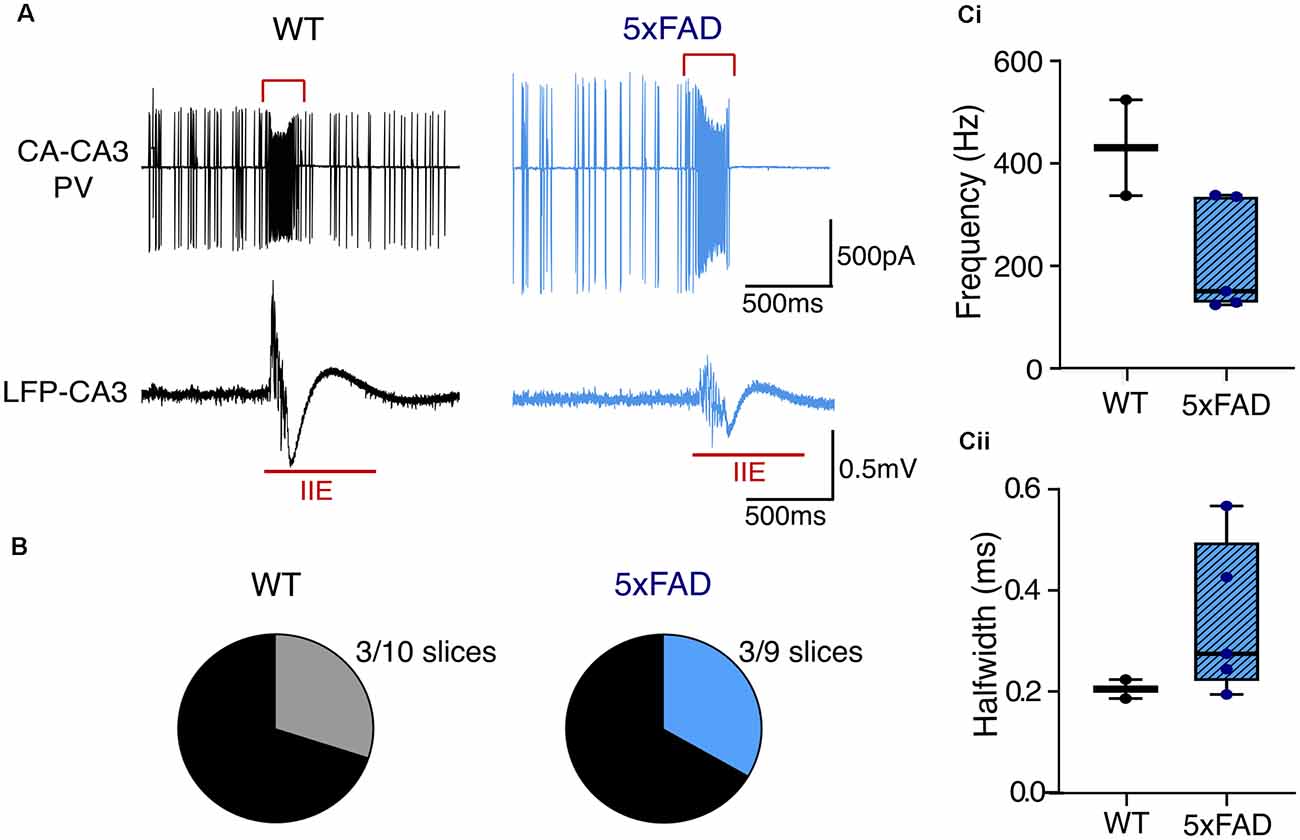
Figure 7. Ictal-like activity patterns in 5xFAD and control slices during kainateapplication. (A) Representative traces from brain slice recordings in kainate that developed ictal-like activity. The top traces show the cell-attached PVIN recordings, and the bottom traces show the simultaneously recorded LFP during an inter-ictal event (shown in red)—the red square bracket in the PVIN recording indicates the section used for the analysis of spike properties during the IIE. (B) The total number of slices in submerged configuration from older WT and 5xFAD mice that displayed interictal-like activity. WT: n = 10 slices, three mice (zero female); 5xFAD: n = 9 slices, three mice (two females). (C) Boxplots of the PVIN firing frequency (Ci) and spike halfwidth (Cii) during the IIEs, from a subset of recordings which displayed both interictal activity and PVIN spiking. WT: n = 2 slices, one male mouse; 5xFAD: n = 5 slices, three mice (one female). The median is shown by the black line and the boxed region indicates the interquartile range with the range shown by the whiskers [Data in (C) Mann-Whitney U test].
PV and Perineuronal Net (PNN) Presence in Young and Aged 5xFAD and Control Mice
Accounts of whether interneuron numbers are affected in AD are controversial, with many contradicting reports (Morawski et al., 2010; Mahar et al., 2017; Cattaud et al., 2018; Ambrad Giovannetti and Fuhrmann, 2019; Hongo et al., 2020). Likewise, there is uncertainty as to whether or not there is any disease-related deficit in perineuronal nets (PNNs), the extracellular matrix structures which preferentially surround PVINs and promote their functioning (Morawski et al., 2010; Cattaud et al., 2018; Hayani et al., 2018; Crapser et al., 2020). We compared numbers of PVINs with and without PNNs, in control and 5xFAD mice throughout disease progression, to determine if the mutants exhibited any differences that correlated with the oscillatory deficits observed (Figures 8A–D). The results of a two-way ANOVA showed a significant effect of age on PVIN numbers, and of genotype on PNN numbers. After post-hoc testing, this revealed a significant reduction in the total numbers of PVINs and PNNs from older 5xFAD brain slices (Figure 8G), with no differences in younger WT and 5xFAD mice (Figures 8E,F). This appears to be due to a non-significant reduction in older 5xFAD PVINs and their associated PNNs (Figure 8H).
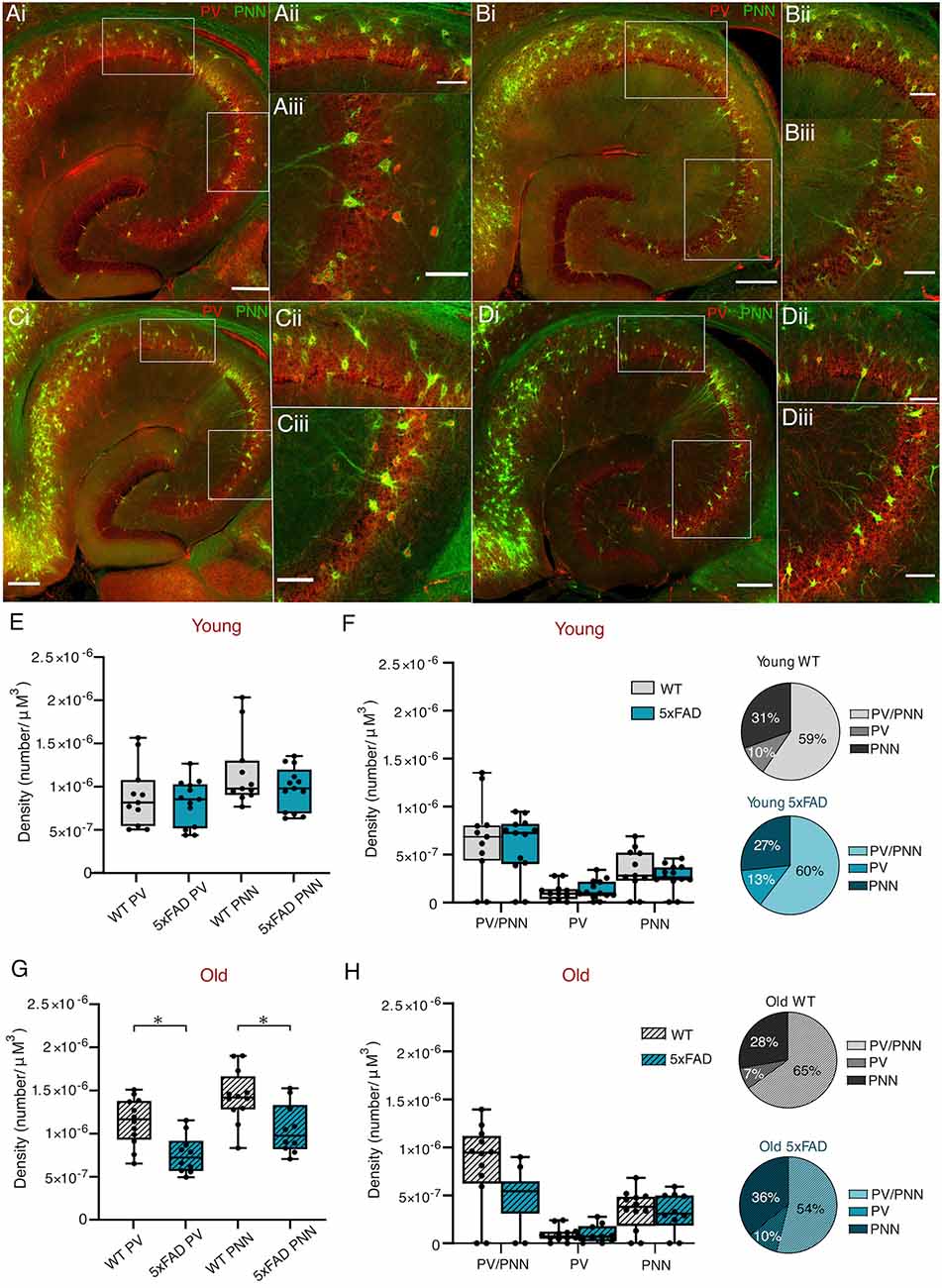
Figure 8. PVIN and PNNdensities in young and old 5xFAD and control mice.(Ai–Di) Representative maximum intensityprojection images of ventral hippocampal slices processed withanti-PV and biotinylated WFA antibodies to label PV cells(red) and perineuronal nets (PNNs; green), respectively (scalebar = 250 μm). Young control (A), Young 5xFAD(B), Old control (C), Old 5xFAD(D). Enlarged images of the CA1(Aii–Dii) and CA3(Aiii–Diii) regions highlighted in the boxed region on the main image (Scale bar = 100 μm). (E,G) Total PV numbers and PNN numbers in young and old slices within CA1 and CA3. (F,H) Combined CA1/CA3 density of PV cells with PNN, PV cells without PNN, and PNN without PV cells in the young and old slices, respectively, with the proportions shown additionally as pie charts. Box plots show the median as the black line, the boxed region indicates the interquartile range, and the whiskers indicate the range. The individual data points represent slices. Young WT: n = 11 slices, four mice (two females); Young 5xFAD: n = 13, three mice (two females); old WT: n = 12 slices, six mice (three females); old 5xFAD: n = 10 slices, six mice (three females). *p <0.05 (two-way ANOVA with Šidák correction for multiple comparisons).
Discussion
In this study, we investigated potential correlations in the hippocampal plaque development and network activity over time in the 5xFAD model of AD focusing on two distinct time points (3–4 months and 12+ months), each with distinct hippocampal plaque burdens (Figure 1). This allowed for an in-depth approach to investigating network and PVIN function in relation to plaque pathology using widely published models of in vitro gamma oscillations. We found that gamma frequency oscillations are differentially affected in brain slices from 5xFAD mice depending on the pharmacological approach used to initiate the oscillations. KA-induced oscillations showed significant power deficits in older 5xFAD slices (Figure 4) and impaired rhythmicity in young (3–4 months) and old (12+ months) 5xFAD slices (Figure 5). In contrast, Cch-induced oscillations showed no power or frequency deficits (Figure 2) and only minor rhythmicity deficits in the oldest 5xFAD slices (Figure 3). We additionally looked at the function of PVINs during gamma frequency oscillations and found that their activity remained remarkably stable when compared between 5xFAD and control mice (Figure 6). However, there was a trend towards increased PVIN excitability in older tissue, with older PVINs firing more frequently during KA application and washout compared to younger PVINs (Figure 6). Although PVIN function was conserved, numbers of PVINs and their associated PNNs (supportive extracellular matrix structures around PVINs) were significantly reduced in older 5xFAD brain slices (Figure 8). Further work is required to ascertain whether the PVIN loss is Aβ plaque-mediated or caused by other disease mechanisms such as excitotoxicity. Regardless, impaired network activity was only seen during KA-induced oscillations, despite significant reductions in PVINs and extensive plaque deposition revealing a remarkable degree of network level resilience for patterned activity in the 5xFAD mouse model.
Gamma Frequency Disruption in Alzheimer’s Disease
Gamma oscillation deficits have often been implicated in the cognitive and memory decline seen in AD patients (Spydell and Sheer, 1983; Ribary et al., 1991; Hermann et al., 2009; Başar et al., 2016). AD mouse models have provided some potential insights into the network mechanisms underlying gamma frequency impairments observed in human EEG recordings. Indeed, a number of studies in various AD mouse models have also reported gamma deficits in ex vivo slice preparations, and in vivo recordings (Driver et al., 2007; Iaccarino et al., 2016; Etter et al., 2019; Zhang et al., 2020; Arroyo-García et al., 2021). We add to this literature by demonstrating that KA-driven gamma oscillations in brain slices from 5xFAD mice reveal deficits at an earlier stage of disease progression, and of greater impact than cholinergically-driven gamma oscillations. As mentioned previously, the triggering mechanisms behind KA- and Cch-driven gamma oscillations are distinct involving unique receptors with differing expression profiles within the hippocampus (Buhl et al., 1998; Fisahn et al., 2002, 2004; Fisahn, 2005). It is also possible that the two approaches engage distinct populations of cells, which are differently affected by evolving AD pathology. This has previously been proposed in work comparing cholinergic, and metabotropic glutamatergic oscillations (Pálhalmi et al., 2004). Additionally, gamma oscillations induced by KA were significantly higher power than those induced by Cch. Thus, our findings could reflect a requisite minimum threshold level of cellular participation to reveal deficits in pharmacologically induced gamma oscillations associated with AD pathology. If only certain subpopulations of cells are affected in AD, then perhaps the gamma deficit phenotype does not reveal itself unless the network is driven particularly hard, as happens with the network activity induced by KA.
5xFAD mice also yielded a lower percentage of slices with detectable CA1 gamma oscillations, and impairments in synchrony between CA3 and CA1, manifested as reduced peak cross-correlations (Figures 3, 5). Despite this, in slices with coupled CA3-CA1 gamma oscillations, there was no difference in the phase lag between regions, suggesting a deficit in CA1 recruitment independent of severe Schaffer collateral impairment. The CA1 deficits instead could reflect weaker population drive or coordination of CA1, through post-synaptic deficits, or CA1 circuit cell-specific excitability impairments. A prior study investigating synaptic function in behaving 5xFAD mice found impaired inhibitory interneuronal connections onto CA1 pyramidal cells and disrupted sharp-wave ripples (Prince et al., 2021). A different study similarly found altered SWRs and PV basket cell (PVBC) activity in the CA1 of 3 month 5xFAD brain slices, with reduced excitatory input to PVBCs during SWRs, and reduced SWR-associated firing of PVBCs (Caccavano et al., 2020). Although the two studies reported contrasting findings with regards to SWR frequency and amplitude, they both implicated PVIN dysfunction in their findings. Moreover, J20-Tg-AD mice exhibit subicular/CA1 PVIN dysfunction with reduced maximal firing and impaired input/output functions, that alter fast and slow gamma-theta cross-frequency coupling (Mondragón-Rodríguez et al., 2018). These studies support the possibility that reduced CA1 recruitment during gamma oscillations is caused by impaired CA1 PVIN activity, and/or CA3-CA1 communication deficits.
Hyperexcitability in Alzheimer’s Disease
Many studies have reported hyperexcitability and altered inhibition/excitation ratios in the brains of AD patients and mouse models (Bakker et al., 2012; Sanchez et al., 2012; Verret et al., 2012; Palop and Mucke, 2016; Styr and Slutsky, 2018; Ciccone et al., 2019). As KA acts to increase neuronal excitability and is commonly used experimentally to induce ictal activity (Lévesque and Avoli, 2013; Falcón-Moya et al., 2018), it was expected that some slices would exhibit ictal-like activity (Figure 7). However, there was no increased tendency towards ictal development in the 5xFAD tissue. We did find that PVINs in aged tissue fired with higher frequency during KA compared to younger tissue and continued firing at higher frequencies following removal of KA (Figure 6B). This difference was only significant between young and old 5xFAD PVINs during the KA washout. Although a similar trend was seen in the control tissue, this may reflect enhanced PVIN excitability in older 5xFAD slices.
PVIN Dysfunction in Alzheimer’s Disease
PVINs are known to be tightly phase-locked to gamma frequency oscillations (Buzśaki and Wang, 2012). We observed PVIN phase-locking to the rising phase of the gamma cycle, in agreement with prior studies (Varga et al., 2014; Andrade-Talavera et al., 2020; Caccavano et al., 2020; Chung et al., 2020; Arroyo-García et al., 2021). Disrupted PVIN phase-locking in different models of AD has been proposed to underly impaired gamma oscillations. Application of Aβ42 peptide to acute brain slices during kainate-induced gamma oscillations promoted increased PVIN firing and interfered with PVIN-gamma phase locking (Andrade-Talavera et al., 2020). Similarly, the application of Aβ1–42 oligomers to brain slices showed both impaired inhibition from PVIN-PC synapses, with lower IPSCs and increased paired pulse depression; and impaired excitatory drive onto PVINs, with reduced amplitude PVIN-recorded EPSCs (Chung et al., 2020; Park et al., 2020). However, we did not see any difference in the phase or degree of phase-locking, indicating unique cellular mechanisms for different AD models evaluated in rodent brain slices. Direct, acute application of purified Aβ42, even at physiological concentrations, could have a stronger effect on slice network function compared to modest deficits observed in slices from 5xFAD mice with unknown and variable levels of Aβ. Slices from 5xFAD mice also had a greater opportunity to undergo compensatory, homeostatic circuit reorganisation to cope with disease progression, perhaps blunting the gamma deficits compared to acute Aβ42 challenge. However, another recent study reported that APPNL-G-F mice exhibited impaired phase-gamma coupling of fast-spiking interneurons with reductions in phase modulation, but not phase angle, as early as 2 months prior to plaque formation, which could indicate a difference between AD mouse models which do, or do not, overexpress hAPP (Saito et al., 2014; Arroyo-García et al., 2021). Finally, several studies have investigated the contribution of different interneuron subtypes toward gamma frequency oscillations such as CGE-derived trilaminar interneurons, which are strongly phase-locked to gamma oscillations in CA1 (Craig and McBain, 2015); and somatostatin-positive interneurons, which have been implicated in the generation of low-frequency gamma oscillations in the visual cortex in vivo and hippocampus in vitro (Chen et al., 2017; Veit et al., 2017; Antonoudiou et al., 2020). KA- and Cch- application could differentially recruit these interneuron subtypes, based on their receptor expression profiles, and thus AD-associated deficits in other interneuronal populations could be contributing to the KA-specific gamma deficit we describe.
Cell Loss in Alzheimer’s Disease
The 5xFAD model is characterised by its early onset pathology, with regards to plaque onset and behavioural deficits (Oakley et al., 2006). As gamma power will be affected by the number of participating cells, neuronal loss within the hippocampus could be the cause of reduced gamma power. Although we did not measure overall cell density within different hippocampal subfields, 5xFAD mice reportedly have significant cell loss in the deep cortical cell layers and subiculum by 9–12 months (Jawhar et al., 2012; Eimer and Vassar, 2013); and cell loss in the hippocampus and cortex by 8–9 months, with synapse loss as early as 4 months in the neocortex (Kang et al., 2021). In particular, PVIN loss could impair the rhythmicity of, and/or the ability of the network to generate gamma oscillatory activity. However, reports of PVIN loss in AD are inconclusive. There are reports of significant PVIN loss in patients and mouse models of AD (Brady and Mufson, 1997; Mahar et al., 2017; Crapser et al., 2020; Giesers and Wirths, 2020; Hongo et al., 2020), whereas no GABAergic cell loss was found in 2, 8 or 18 month APP-J20 AD mice (Rubio et al., 2012). There are even reports of increased PV immunoreactivity in 3-month APPPS1 mice (Hollnagel et al., 2019). We examined the hippocampal PVIN density in brain slices from 3 to 4-month and 12+-month-old 5xFAD mice and found a significant decrease in the total number of PVINs in old 5xFAD tissue (Figure 8G). While this may in part explain the impaired power and rhythmicity observed, it was striking that no deficits were observed for Cch-induced oscillations and that PVIN function appeared preserved in 5xFAD mice.
PVINs and Perineuronal Nets (PNNs) in Alzheimer’s Disease
Perineuronal nets (PNNs) are extracellular matrix structures which form during development and signal the end of the critical period for synaptic plasticity (Sorg et al., 2016). They are thought to be involved in determining levels of plasticity and in stabilising and securing synaptic connections. They have been shown to preferentially surround PVINs (Härtig et al., 1999); and there is evidence for their role in memory impairment in AD (Sorg et al., 2016; Wen et al., 2018). Due to their role in protecting against oxidative stress, some studies argue that impaired PNNs leave cells more susceptible to damage and cell death (Morawski et al., 2004). On the other hand, digesting PNNs with chondroitinase ABC may reopen plasticity, to permit structural remodelling such as axonal sprouting to bypass damaged synapses in AD (Romberg et al., 2013; Yang et al., 2015). There is also evidence for PNN disruption altering the activity of PVINs and PVIN-dependent forms of network activity such as sharp wave ripples and gamma frequency oscillations (Favuzzi et al., 2017; Lensjø et al., 2017; Sun et al., 2018).
The literature is divided on whether or not AD affects PNNs (Morawski et al., 2010; Cattaud et al., 2018; Crapser et al., 2020). Our results show a reduction in total PNNs in older 5xFAD tissue. However, further work will be necessary to determine whether the loss in PNNs is upstream or downstream of PVIN loss.
Possible Contribution of Metabolic Stress Towards Impaired Network Activity in AD
Another possible explanation for the gamma deficits uncovered during larger power, KA-driven activity, could be the role of cellular metabolism. This is of particular relevance, considering the strong association between increased metabolic stress and Alzheimer’s disease pathophysiology (de Felice and Lourenco, 2015; Chan et al., 2016). It has been well documented that oxygen consumption and expression of mitochondrial proteins increase with gamma oscillatory activity in the hippocampal slices in an activity-dependent manner (Kann et al., 2011; Chan et al., 2016). Metabolic stress has also been shown to reduce gamma oscillations in vitro, particularly in the ventral hippocampus, whilst leaving gamma frequency unchanged, similar to our results (Steullet et al., 2010; Elzoheiry et al., 2020). It is, therefore, possible that deficits in 5xFAD network activity are only present when driven by kainate as these oscillations are larger power and consequently more metabolically demanding. In this case, metabolic dysfunction within the network would be exacerbated by the intensity of the network activity.
Although one might expect the impact of metabolic stress to manifest primarily in PVINs, due to their energy-demanding physiology, we see no change in PVIN firing properties in brain slices from 5xFAD mice. This could be attributed to specific PVIN properties intended to manage this energy requirement, such as higher densities of mitochondria with greater cytochrome-c content, increased expression of the mitochondrial transcriptional co-activator PGC-1α, and fast-gating KV3 channels (Tansey et al., 2002; Cowell et al., 2007; Kann et al., 2014; Kann, 2016). Additionally, recruitment and synchronisation of cell ensembles during cholinergically-induced gamma oscillations were compromised by rotenone-mediated inhibition of complex 1, whereas individual cell activity was unaffected (Elzoheiry et al., 2020). This suggests that mild metabolic stress, whilst not impacting individual cell activity patterns, could impair the regulation of neuronal ensembles in network gamma activity, possibly through subtle alterations in synaptic function. Indeed, as previously mentioned, the application of Aβ1–42 oligomers to hippocampal slices disrupted synaptic transmission at reciprocal PC-PVIN synapses and impaired network oscillations (Kurudenkandy et al., 2014; Chung et al., 2020; Park et al., 2020).
In line with these recent findings, there is interest in exploring antioxidant treatment for AD pathophysiology, to target metabolic stress (Kapogiannis and Mattson, 2011; Hongo et al., 2020; Yan et al., 2020). Likewise, there has also been a lot of focus on developing therapies to boost gamma oscillatory function in order to reduce AD pathology and improve cognitive function (Iaccarino et al., 2016; Lee et al., 2018; Adaikkan et al., 2019; Etter et al., 2019; Jones et al., 2019; Martorell et al., 2019; Strüber and Herrmann, 2020; Zhang et al., 2020). However, it may also be useful to consider issues such as metabolic stress, impaired synaptic transmission, and disrupted gamma frequency activity as overlapping pathologies each with the ability to influence one another (Kapogiannis and Mattson, 2011). In this way, potential combinatorial approaches towards AD treatment may be developed.
Conclusion
We have described here that KA-driven network activity in the 5xFAD mouse is impaired; whilst Cch-driven activity is relatively spared in the model, despite extensive plaque burden and loss of PVINs. It has already been acknowledged that plaque burden does not correlate well with the level of cognitive decline in AD (Hardy and Selkoe, 2002). Our work suggests that plaque-levels and even cell loss are not enough to fully predict the extent of network-level deficits in AD, and that the network is strikingly resilient to these pathological changes. In conclusion, this highlights the need for a more nuanced approach towards thinking of Alzheimer’s disease pathology in order to improve understanding of disease aetiology, diagnosis, and treatment options.
Data Availability Statement
The raw data supporting the conclusions of this article will be made available by the authors, without undue reservation.
Ethics Statement
The animal study was reviewed and approved by National Institutes of Health (ASP# 17-045).
Author Contributions
CM and KP conceived and designed research. CM-G, KP, and AC performed electrophysiology experiments. CM-G and AC analysed electrophysiology data. CM-G, ML, KB, and NB performed/contributed to immunohistochemistry experiments or analysis. DA maintained and managed transgenic mouse colonies. CM, KP, AC, and CM-G interpreted results of experiments. AC and AT provided code for electrophysiology analysis. CM-G prepared figures and drafted manuscript. KP, AC, AT, and CM edited and revised manuscript. All authors approved final version of manuscript. All authors contributed to the article and approved the submitted version.
Funding
This work was supported by the NICHD Intramural Research Program (CM) and a Wellcome Trust-NIH PhD studentship 205944/Z/17/Z (CM-G).
Conflict of Interest
The authors declare that the research was conducted in the absence of any commercial or financial relationships that could be construed as a potential conflict of interest.
Publisher’s Note
All claims expressed in this article are solely those of the authors and do not necessarily represent those of their affiliated organizations, or those of the publisher, the editors and the reviewers. Any product that may be evaluated in this article, or claim that may be made by its manufacturer, is not guaranteed or endorsed by the publisher.
Acknowledgments
We would like to thank Xiaoqing Yuan and Steven Hunt for technical assistance with immunohistochemistry and genotyping. We also would like to thank Vincent Schram and Lynne Holtzclaw of the NICHD Microscopy and Imaging Core for their imaging support.
Supplementary Material
The Supplementary Material for this article can be found online at: https://www.frontiersin.org/articles/10.3389/fnsyn.2022.857608/full#supplementary-material.
References
Adaikkan, C., Middleton, S. J., Marco, A., Pao, P. C., Mathys, H., Kim, D. N. W., et al. (2019). Gamma entrainment binds higher-order brain regions and offers neuroprotection. Neuron 102, 929–943.e8. doi: 10.1016/j.neuron.2019.04.011
Ambrad Giovannetti, E., and Fuhrmann, M. (2019). Unsupervised excitation: GABAergic dysfunctions in Alzheimer’s disease. Brain Res. 1707, 216–226. doi: 10.1016/j.brainres.2018.11.042
Andrade-Talavera, Y., Arroyo-García, L. E., Chen, G., Johansson, J., and Fisahn, A. (2020). Modulation of Kv3.1/Kv3.2 promotes gamma oscillations by rescuing Aβ-induced desynchronization of fast-spiking interneuron firing in an AD mouse model in vitro. J. Physiol. 598, 3711–3725. doi: 10.1113/JP279718
Antonoudiou, P., Tan, Y. L., Kontou, G., Upton, A. L., and Mann, E. O. (2020). Systems/circuits parvalbumin and somatostatin interneurons contribute to the generation of hippocampal gamma oscillations. J. Neurosci. 40, 7668–7687. doi: 10.1523/JNEUROSCI.0261-20.2020
Arroyo-García, L. E., Isla, A. G., Andrade-Talavera, Y., Balleza-Tapia, H., Loera-Valencia, R., Alvarez-Jimenez, L., et al. (2021). Impaired spike-gamma coupling of area CA3 fast-spiking interneurons as the earliest functional impairment in the App NL-G-F mouse model of Alzheimer’s disease. Mol. Psychiatry 26, 5557–5567. doi: 10.1038/s41380-021-01257-0
Bakker, A., Krauss, G. L., Albert, M. S., Speck, C. L., Jones, L. R., Stark, C. E., et al. (2012). Reduction of hippocampal hyperactivity improves cognition in amnestic mild cognitive impairment. Neuron 74, 467–474. doi: 10.1016/j.neuron.2012.03.023
Balleza-Tapia, H., Crux, S., Andrade-Talavera, Y., Dolz-Gaiton, P., Papadia, D., Chen, G., et al. (2018). TrpV1 receptor activation rescues neuronal function and network gamma oscillations from Aβ-induced impairment in mouse hippocampus in vitro. eLife 7:e37703. doi: 10.7554/eLife.37703
Bartos, M., Vida, I., Frotscher, M., Meyer, A., Monyer, H., Rg, J., et al. (2002). Fast synaptic inhibition promotes synchronized gamma oscillations in hippocampal interneuron networks. Proc. Natl. Acad. Sci. U S A 99, 13222–13227. doi: 10.1073/pnas.192233099
Başar, E., Emek-Savaş, D. D., Güntekin, B., and Yener, G. G. (2016). Delay of cognitive gamma responses in Alzheimer’s disease. Neuroimage Clin. 11, 106–115. doi: 10.1016/j.nicl.2016.01.015
Bauer, E. P., Paz, R., and Paré, D. (2007). Gamma oscillations coordinate amygdalo-rhinal interactions during learning. J. Neurosci. 27, 9369–9379. doi: 10.1523/JNEUROSCI.2153-07.2007
Berens, P. (2009). CircStat: a MATLAB toolbox for circular statistics. J. Stat. Softw. 31, 1–21. doi: 10.18637/jss.v031.i10
Braak, H., and Braak, E. (1991). Neuropathological stageing of Alzheimer-related changes. Acta Neuropathol. 82, 239–259). doi: 10.1007/BF00308809
Brady, D. R., and Mufson, E. J. (1997). Parvalbumin-immunoreactive neurons in the hippocampal formation of Alzheimer’s diseased brain. Neuroscience 80, 1113–1125. doi: 10.1016/s0306-4522(97)00068-7
Buhl, E. H., Tamás, G., and Fisahn, A. (1998). Cholinergic activation and tonic excitation induce persistent gamma oscillations in mouse somatosensory cortex in vitro. J. Physiol. 513, 117–126. doi: 10.1111/j.1469-7793.1998.117by.x
Busche, M. A., Eichhoff, G., Adelsberger, H., Abramowski, D., Wiederhold, K. H., Haass, C., et al. (2008). Clusters of hyperactive neurons near amyloid plaques in a mouse model of Alzheimer’s disease. Science 321, 1686–1689. doi: 10.1126/science.1162844
Buzśaki, G., and Wang, X. J. (2012). Mechanisms of gamma oscillations. Annu. Rev. Neurosci. 35, 203–225). doi: 10.1146/annurev-neuro-062111-150444
Caccavano, A., Lorenzo Bozzelli, P., Forcelli, P. A., Pak, D. T. S., Wu, J. Y., Conant, K., et al. (2020). Inhibitory parvalbumin basket cell activity is selectively reduced during hippocampal sharp wave ripples in a mouse model of familial Alzheimer’s disease. J. Neurosci. 40, 5116–5136. doi: 10.1523/JNEUROSCI.0425-20.2020
Cangiano, L., and Grillner, S. (2003). Fast and slow locomotor burst generation in the hemispinal cord of the lamprey. J. Neurophysiol. 89, 2931–2942. doi: 10.1152/jn.01100.2002
Cardin, J. A., Carlén, M., Meletis, K., Knoblich, U., Zhang, F., Deisseroth, K., et al. (2009). Driving fast-spiking cells induces gamma rhythm and controls sensory responses. Nature 459, 663–667. doi: 10.1038/nature08002
Cattaud, V., Bezzina, C., Rey, C. C., Lejards, C., Dahan, L., and Verret, L. (2018). Early disruption of parvalbumin expression and perineuronal nets in the hippocampus of the Tg2576 mouse model of Alzheimer’s disease can be rescued by enriched environment. Neurobiol. Aging 72, 147–158. doi: 10.1016/j.neurobiolaging.2018.08.024
Chan, F., Lax, N. Z., Davies, C. H., Turnbull, D. M., and Cunningham, M. O. (2016). Neuronal oscillations: A physiological correlate for targeting mitochondrial dysfunction in neurodegenerative diseases. Neuropharmacology 102, 48–58. doi: 10.1016/j.neuropharm.2015.10.033
Chen, G., Zhang, Y., Li, X., Zhao, X., Ye, Q., Lin, Y., et al. (2017). Distinct inhibitory circuits orchestrate cortical beta and gamma band oscillations. Neuron 96:1403. doi: 10.1016/j.neuron.2017.11.033
Chung, H., Park, K., Jang, H. J., Kohl, M. M., and Kwag, J. (2020). Dissociation of somatostatin and parvalbumin interneurons circuit dysfunctions underlying hippocampal theta and gamma oscillations impaired by amyloid β oligomers in vivo. Brain Struct. Funct. 225, 935–954. doi: 10.1007/s00429-020-02044-3
Ciccone, R., Franco, C., Piccialli, I., Boscia, F., Casamassa, A., de Rosa, V., et al. (2019). Amyloid β-induced upregulation of Nav1.6 underlies neuronal hyperactivity in Tg2576 Alzheimer’s disease mouse model. Sci. Rep. 9:13592. doi: 10.1038/s41598-019-50018-1
Cobb, S. R., Buhl, E. H., Halasy, K., Paulsen, O., and Somogyi, P. (1995). Synchronization of neuronal activity in hippocampus by individual GABAergic interneurons. Nature 378, 75–78. doi: 10.1038/378075a0
Colgin, L. L., and Moser, E. I. (2010). Gamma oscillations in the hippocampus. Physiology (Bethesda) 25, 319–329. doi: 10.1152/physiol.00021.2010
Cowell, R. M., Blake, K. R., and Russell, J. W. (2007). Localization of the transcriptional coactivator PGC-1α to GABAergic neurons during maturation of the rat brain. J. Comp. Neurol. 502, 1–18. doi: 10.1002/cne.21211
Craig, M. T., and McBain, C. J. (2015). Systems/circuits fast gamma oscillations are generated intrinsically in CA1 without the involvement of fast-spiking basket cells. J. Neurosci. 35, 3616–3624. doi: 10.1523/JNEUROSCI.4166-14.2015
Crapser, J. D., Spangenberg, E. E., Barahona, R. A., Arreola, M. A., Hohsfield, L. A., and Green, K. N. (2020). Microglia facilitate loss of perineuronal nets in the Alzheimer’s disease brain. EBioMedicine 58:102919. doi: 10.1016/j.ebiom.2020.102919
de Felice, F. G., and Lourenco, M. V. (2015). Brain metabolic stress and neuroinflammation at the basis of cognitive impairment in Alzheimer’s disease. Front. Aging Neurosci. 7:94. doi: 10.3389/fnagi.2015.00094
Dickinson, R., Awaiz, S., Whittington, M. A., Lieb, W. R., and Franks, N. P. (2003). The effects of general anaesthetics on carbachol-evoked gamma oscillations in the rat hippocampus in vitro. Neuropharmacology 44, 864–872. doi: 10.1016/s0028-3908(03)00083-2
Draguhn, A., and Buzsáki, G. (2004). Neuronal oscillations in cortical networks. Science 304, 1926–1930. doi: 10.1126/science.1099745
Driver, J. E., Racca, C., Cunningham, M. O., Towers, S. K., Davies, C. H., Whittington, M. A., et al. (2007). Impairment of hippocampal gamma (γ)-frequency oscillations in vitro in mice overexpressing human amyloid precursor protein (APP). Eur. J. Neurosci. 26, 1280–1288. doi: 10.1111/j.1460-9568.2007.05705.x
Eimer, W. A., and Vassar, R. (2013). Neuron loss in the 5XFAD mouse model of Alzheimer’s disease correlates with intraneuronal Aβ42 accumulation and Caspase-3 activation. Mol. Neurodegener. 8:2. doi: 10.1186/1750-1326-8-2
Elzoheiry, S., Lewen, A., Schneider, J., Both, M., Hefter, D., Boffi, J. C., et al. (2020). Mild metabolic stress is sufficient to disturb the formation of pyramidal cell ensembles during gamma oscillations. J. Cereb. Blood Flow Metab. 40:2401. doi: 10.1177/0271678X19892657
Etter, G., van der Veldt, S., Manseau, F., Zarrinkoub, I., Trillaud-Doppia, E., and Williams, S. (2019). Optogenetic gamma stimulation rescues memory impairments in an Alzheimer’s disease mouse model. Nat. Commun. 10:5322. doi: 10.1038/s41467-019-13260-9
Falcón-Moya, R., Sihra, T. S., and Rodríguez-Moreno, A. (2018). Kainate receptors: role in epilepsy. Front. Mol. Neurosci. 11:217. doi: 10.3389/fnmol.2018.00217
Favuzzi, E., Marques-Smith, A., Deogracias, R., Winterflood, C. M., Sánchez-Aguilera, A., Mantoan, L., et al. (2017). Activity-dependent gating of parvalbumin interneuron function by the perineuronal net protein brevican. Neuron 95, 639–655.e10. doi: 10.1016/j.neuron.2017.06.028
Fisahn, A. (2005). Kainate receptors and rhythmic activity in neuronal networks: hippocampal gamma oscillations as a tool. J. Physiol. 562:65. doi: 10.1113/jphysiol.2004.077388
Fisahn, A., Contractor, A., Traub, R. D., Buhl, E. H., Heinemann, S. F., and McBain, C. J. (2004). Distinct roles for the kainate receptor subunits GluR5 and GluR6 in kainate-induced hippocampal gamma oscillations. J. Neurosci. 24, 9658–9668. doi: 10.1523/JNEUROSCI.2973-04.2004
Fisahn, A., Pike, F. G., Buhl, E. H., and Paulsen, O. (1998). Cholinergic induction of network oscillations at 40 Hz in the hippocampus in vitro. Nature 394, 186–189. doi: 10.1038/28179
Fisahn, A., Yamada, M., Duttaroy, A., Gan, J. W., Deng, C. X., McBain, C. J., et al. (2002). Muscarinic induction of hippocampal gamma oscillations requires coupling of the M1 receptor to two mixed cation currents. Neuron 33, 615–624. doi: 10.1016/s0896-6273(02)00587-1
Franklin, K., and Paxinos, G. (2008). The Mouse Brain in Stereotaxic Coordinates Compact, 3rd Edn. New York: Academic Press
Fries, P., Reynolds, J. H., Rorie, A. E., and Desimone, R. (2001). Modulation of oscillatory neuronal synchronization by selective visual attention. Science 291, 1560–1563. doi: 10.1126/science.1055465
Giesers, N. K., and Wirths, O. (2020). Loss of hippocampal calretinin and parvalbumin interneurons in the 5XFAD mouse model of Alzheimer’s disease. ASN Neuro 12:1759091420925356. doi: 10.1177/1759091420925356
Gillespie, A. K., Jones, E. A., Lin, Y. H., Karlsson, M. P., Kay, K., Yoon, S. Y., et al. (2016). Apolipoprotein E4 causes age-dependent disruption of slow gamma oscillations during hippocampal sharp-wave ripples. Neuron 90, 740–751. doi: 10.1016/j.neuron.2016.04.009
Gray, C. M., and Singer, W. (1989). Stimulus-specific neuronal oscillations in orientation columns of cat visual cortex. Proc. Nat. Acad. Sci. U S A 86, 1698–1702. doi: 10.1073/pnas.86.5.1698
Gulyás, A. I., Szabó, G. G., Ulbert, I., Holderith, N., Monyer, H., Erdélyi, F., et al. (2010). Parvalbumin-containing fast-spiking basket cells generate the field potential oscillations induced by cholinergic receptor activation in the hippocampus. J. Neurosci. 30, 15134–15145. doi: 10.1523/JNEUROSCI.4104-10.2010
Hájos, N., Ellender, T. J., Zemankovics, R., Mann, E. O., Exley, R., Cragg, S. J., et al. (2009). Maintaining network activity in submerged hippocampal slices: importance of oxygen supply. Eur J. Neurosci. 29, 319–327. doi: 10.1111/j.1460-9568.2008.06577.x
Hardy, J., and Selkoe, D. J. (2002). The amyloid hypothesis of Alzheimer’s disease: progress and problems on the road to therapeutics. Science 297, 353–356. doi: 10.1126/science.1072994
Härtig, W., Derouiche, A., Welt, K., Brauer, K., Grosche, J., Mäder, M., et al. (1999). Cortical neurons immunoreactive for the potassium channel Kv3.1b subunit are predominantly surrounded by perineuronal nets presumed as a buffering system for cations. Brain Res. 842, 15–29. doi: 10.1016/s0006-8993(99)01784-9
Hayani, H., Song, I., and Dityatev, A. (2018). Increased excitability and reduced excitatory synaptic input into fast-spiking CA2 interneurons after enzymatic attenuation of extracellular matrix. Front. Cell Neurosci. 12:149. doi: 10.3389/fncel.2018.00149
Hermann, D., Both, M., Ebert, U., Gross, G., Schoemaker, H., Draguhn, A., et al. (2009). Synaptic transmission is impaired prior to plaque formation in amyloid precursor protein-overexpressing mice without altering behaviorally-correlated sharp wave-ripple complexes. Neuroscience 162, 1081–1090. doi: 10.1016/j.neuroscience.2009.05.044
Hollnagel, J. O., Elzoheiry, S., Gorgas, K., Kins, S., Beretta, C. A., Kirsch, J., et al. (2019). Early alterations in hippocampal perisomatic GABAergic synapses and network oscillations in a mouse model of Alzheimer’s disease amyloidosis. PLoS One 14:e0209228. doi: 10.1371/journal.pone.0209228
Hongo, N., Takamura, Y., Nishimaru, H., Matsumoto, J., Tobe, K., Saito, T., et al. (2020). Astaxanthin ameliorated parvalbumin-positive neuron deficits and Alzheimer’s disease-related pathological progression in the hippocampus of AppNL-G-F/NL-G-F mice. Front. Pharmacol. 11:307. doi: 10.3389/fphar.2020.00307
Iaccarino, H. F., Singer, A. C., Martorell, A. J., Rudenko, A., Gao, F., Gillingham, T. Z., et al. (2016). Gamma frequency entrainment attenuates amyloid load and modifies microglia. Nature 540, 230–235. doi: 10.1038/nature20587
Jawhar, S., Trawicka, A., Jenneckens, C., Bayer, T. A., and Wirths, O. (2012). Motor deficits, neuron loss and reduced anxiety coinciding with axonal degeneration and intraneuronal Aβ aggregation in the 5XFAD mouse model of Alzheimer’s disease. Neurobiol. Aging 33, 196.e29–196.e40. doi: 10.1016/j.neurobiolaging.2010.05.027
Jones, M., McDermott, B., Oliveira, B. L., O’Brien, A., Coogan, D., Lang, M., et al. (2019). Gamma band light stimulation in human case studies: groundwork for potential Alzheimer’s disease treatment. J. Alzheimer’s Dis. 70, 171–185. doi: 10.3233/JAD-190299
Kanak, D. J., Rose, G. M., Zaveri, H. P., and Patrylo, P. R. (2013). Altered network timing in the CA3-CA1 circuit of hippocampal slices from aged mice. PLoS One 8:e61364. doi: 10.1371/journal.pone.0061364
Kang, S., Kim, J., and Chang, K.-A. (2021). Spatial memory deficiency early in 6xTg Alzheimer’s disease mouse model. Sci. Rep. 11:334. doi: 10.1038/s41598-020-79344-5
Kann, O. (2016). The interneuron energy hypothesis: implications for brain disease. Neurobiol. Dis. 90, 75–85. doi: 10.1016/j.nbd.2015.08.005
Kann, O., Huchzermeyer, C., Kovács, R., Wirtz, S., and Schuelke, M. (2011). Gamma oscillations in the hippocampus require high complex I gene expression and strong functional performance of mitochondria. Brain 134, 345–358. doi: 10.1093/brain/awq333
Kann, O., Papageorgiou, I. E., and Draguhn, A. (2014). Highly energized inhibitory interneurons are a central element for information processing in cortical networks. J. Cereb. Blood Flow Metab. 34, 1270–1282. doi: 10.1038/jcbfm.2014.104
Kapogiannis, D., and Mattson, M. P. (2011). Perturbed energy metabolism and neuronal circuit dysfunction in cognitive impairment. Lancet Neurol. 10, 187–198. doi: 10.1016/S1474-4422(10)70277-5
Kurudenkandy, F. R., Zilberter, M., Biverstål, H., Presto, J., Honcharenko, D., Strömberg, R., et al. (2014). Amyloid-β-induced action potential desynchronization and degradation of hippocampal gamma oscillations is prevented by interference with peptide conformation change and aggregation. J. Neurosci. 34, 11416–11425. doi: 10.1523/JNEUROSCI.1195-14.2014
Lane, C. A., Hardy, J., and Schott, J. M. (2018). Alzheimer’s disease. Eur. J. Neurol. 25, 59–70. doi: 10.1111/ene.13439
Lee, J., Ryu, S., Kim, H.-J., Jung, J., Lee, B., and Kim, T. (2018). 40 Hz acoustic stimulation decreases amyloid beta and modulates brain rhythms in a mouse model of Alzheimer’s disease. BioRxiv [Preprint]. doi: 10.1101/390302
Lensjø, K. K., Lepperød, M. E., Dick, G., Hafting, T., and Fyhn, M. (2017). Removal of perineuronal nets unlocks juvenile plasticity through network mechanisms of decreased inhibition and increased gamma activity. J. Neurosci. 37, 1269–1283. doi: 10.1523/JNEUROSCI.2504-16.2016
Lévesque, M., and Avoli, M. (2013). The kainic acid model of temporal lobe epilepsy. Neurosci. Biobehav. Rev. 37, 2887–2899. doi: 10.1016/j.neubiorev.2013.10.011
Lisman, J. E., and Idiart, M. A. P. (1995). Storage of 7 ± 2 short-term memories in oscillatory subcycles. Science 267, 1512–1515. doi: 10.1126/science.7878473
Mahar, I., Albuquerque, M. S., Mondragon-Rodriguez, S., Cavanagh, C., Davoli, M. A., Chabot, J. G., et al. (2017). Phenotypic alterations in hippocampal NPY- and PV-expressing interneurons in a presymptomatic transgenic mouse model of Alzheimer’s disease. Front. Aging Neurosci. 8:327. doi: 10.3389/fnagi.2016.00327
Martinez-Losa, M., Tracy, T. E., Ma, K., Verret, L., Clemente-Perez, A., Khan, A. S., et al. (2018). Nav1.1-overexpressing interneuron transplants restore brain rhythms and cognition in a mouse model of Alzheimer’s disease. Neuron 98, 75–89.e5. doi: 10.1016/j.neuron.2018.02.029
Martorell, A. J., Paulson, A. L., Suk, H. J., Abdurrob, F., Drummond, G. T., Guan, W., et al. (2019). Multi-sensory gamma stimulation ameliorates Alzheimer’s-associated pathology and improves cognition. Cell 177, 256–271.e22. doi: 10.1016/j.cell.2019.02.014
Mondragón-Rodríguez, S., Gu, N., Manseau, F., and Williams, S. (2018). Alzheimer’s transgenic model is characterized by very early brain network alterations and β-CTF fragment accumulation: reversal by β-secretase inhibition. Front. Cell. Neurosci. 12:121. doi: 10.3389/fncel.2018.00121
Morawski, M., Brückner, M. K., Riederer, P., Brückner, G., and Arendt, T. (2004). Perineuronal nets potentially protect against oxidative stress. Exp. Neurol. 188, 309–315. doi: 10.1016/j.expneurol.2004.04.017
Morawski, M., Pavlica, S., Seeger, G., Grosche, J., Kouznetsova, E., Schliebs, R., et al. (2010). Perineuronal nets are largely unaffected in Alzheimer model Tg2576 mice. Neurobiol. Aging 31, 1254–1256. doi: 10.1016/j.neurobiolaging.2008.07.023
Oakley, H., Cole, S. L., Logan, S., Maus, E., Shao, P., Craft, J., et al. (2006). Intraneuronal β-amyloid aggregates, neurodegeneration and neuron loss in transgenic mice with five familial Alzheimer’s disease mutations: potential factors in amyloid plaque formation. J. Neurosci. 26, 10129–10140. doi: 10.1523/JNEUROSCI.1202-06.2006
Ogomori, K., Kitamoto, T., Tateishi, J., Sato, Y., Suetsugu, M., and Abe, M. (1989). β-protein amyloid is widely distributed in the central nervous system of patients with Alzheimer’s disease. Am. J. Pathol. 134, 243–251.
Pálhalmi, J., Paulsen, O., Freund, T. F., and Hájos, N. (2004). Distinct properties of carbachol- and DHPG-induced network oscillations in hippocampal slices. Neuropharmacology 47, 381–389. doi: 10.1016/j.neuropharm.2004.04.010
Palop, J. J., and Mucke, L. (2016). Network abnormalities and interneuron dysfunction in Alzheimer disease. Nat. Rev. Neurosci. 17, 777–792. doi: 10.1038/nrn.2016.141
Park, K., Lee, J., Jang, H. J., Richards, B. A., Kohl, M. M., and Kwag, J. (2020). Optogenetic activation of parvalbumin and somatostatin interneurons selectively restores theta-nested gamma oscillations and oscillation-induced spike timing-dependent long-term potentiation impaired by amyloid β oligomers. BMC Biol. 18:7. doi: 10.1186/s12915-019-0732-7
Prince, S. M., Paulson, A. L., Jeong, N., Zhang, L., Amigues, S., and Singer, A. C. (2021). Alzheimer’s pathology causes impaired inhibitory connections and reactivation of spatial codes during spatial navigation. Cell Rep. 35:109008. doi: 10.1016/j.celrep.2021.109008
Ribary, U., Ioannides, A. A., Singh, K. D., Hasson, R., Bolton, J. P. R., Lado, F., et al. (1991). Magnetic field tomography of coherent thalamocortical 40-Hz oscillations in humans. Proc. Natl. Acad. Sci. U S A 88, 11037–11041. doi: 10.1073/pnas.88.24.11037
Romberg, C., Yang, S., Melani, R., Andrews, M. R., Horner, A. E., Spillantini, M. G., et al. (2013). Depletion of perineuronal nets enhances recognition memory and long-term depression in the perirhinal cortex. J. Neurosci. 33, 7057–7065. doi: 10.1523/JNEUROSCI.6267-11.2013
Rubio, S. E., Vega-Flores, G., Martínez, A., Bosch, C., Pérez-Mediavilla, A., Del Río, J., et al. (2012). Accelerated aging of the GABAergic septohippocampal pathway and decreased hippocampal rhythms in a mouse model of Alzheimer’s disease. FASEB J. 26, 4458–4467. doi: 10.1096/fj.12-208413
Saito, T., Matsuba, Y., Mihira, N., Takano, J., Nilsson, P., Itohara, S., et al. (2014). Single App knock-in mouse models of Alzheimer’s disease. Nat. Neurosci. 17, 661–663. doi: 10.1038/nn.3697
Sanchez, P. E., Zhu, L., Verret, L., Vossel, K. A., Orr, A. G., Cirrito, J. R., et al. (2012). Levetiracetam suppresses neuronal network dysfunction and reverses synaptic and cognitive deficits in an Alzheimer’s disease model. Proc. Natl. Acad. Sci. U S A 109, E2895–E2903. doi: 10.1073/pnas.1121081109
Schneider, F., Baldauf, K., Wetzel, W., and Reymann, K. G. (2014). Behavioral and EEG changes in male 5xFAD mice. Physiol. Behav. 135, 25–33. doi: 10.1016/j.physbeh.2014.05.041
Schneider, J., Lewen, A., Ta, T. T., Galow, L. V., Isola, R., Papageorgiou, I. E., et al. (2015). A reliable model for gamma oscillations in hippocampal tissue. J. Neurosci. Res. 93, 1067–1078. doi: 10.1002/jnr.23590
Selkoe, D. J., and Hardy, J. (2016). The amyloid hypothesis of Alzheimer’s disease at 25 years. EMBO Mol. Med. 8, 595–608. doi: 10.15252/emmm.201606210
Serrano-Pozo, A., Frosch, M. P., Masliah, E., and Hyman, B. T. (2011). Neuropathological alterations in Alzheimer disease. Cold Spring Harb. Perspect. Med. 1:a006189. doi: 10.1101/cshperspect.a006189
Singer, W. (1999). Neuronal synchrony: a versatile code for the definition of relations? Neuron, 24, 49–65, 111–125. doi: 10.1016/S0896-6273(00)80821-1
Siwek, M. E., Müller, R., Henseler, C., Trog, A., Lundt, A., Wormuth, C., et al. (2015). Altered theta oscillations and aberrant cortical excitatory activity in the 5XFAD model of Alzheimer’s disease. Neural Plast. 2015:781731. doi: 10.1155/2015/781731
Sohal, V. S., Zhang, F., Yizhar, O., and Deisseroth, K. (2009). Parvalbumin neurons and gamma rhythms enhance cortical circuit performance. Nature 459, 698–702. doi: 10.1038/nature07991
Sorg, B. A., Berretta, S., Blacktop, J. M., Fawcett, J. W., Kitagawa, H., Kwok, J. C. F., et al. (2016). Casting a wide net: role of perineuronal nets in neural plasticity. J. Neurosci. 36, 11459–11468. doi: 10.1523/JNEUROSCI.2351-16.2016
Spydell, J. D., and Sheer, D. E. (1983). Forty hertz EEC activity in Alzheimer’s type dementia. Psychophysiology 20, 313–319. doi: 10.1111/j.1469-8986.1983.tb02160.x
Steullet, P., Cabungcal, J.-H., Kulak, A., Kraftsik, R., Chen, Y., Dalton, T. P., et al. (2010). Redox dysregulation affects the ventral but not dorsal hippocampus: impairment of parvalbumin neurons, gamma oscillations and related behaviors. J. Neurosci. 30, 2547–2558. doi: 10.1523/JNEUROSCI.3857-09.2010
Strüber, D., and Herrmann, C. S. (2020). Modulation of gamma oscillations as a possible therapeutic tool for neuropsychiatric diseases: a review and perspective. Int. J. Psychophysiol. 152, 15–25. doi: 10.1016/j.ijpsycho.2020.03.003
Styr, B., and Slutsky, I. (2018). Imbalance between firing homeostasis and synaptic plasticity drives early-phase Alzheimer’s disease. Nat. Neurosci. 21, 463–473. doi: 10.1038/s41593-018-0080-x
Sun, Z. Y., Bozzelli, P. L., Caccavano, A., Allen, M., Balmuth, J., Vicini, S., et al. (2018). Disruption of perineuronal nets increases the frequency of sharp wave ripple events. Hippocampus 28, 42–52. doi: 10.1002/hipo.22804
Tansey, E. P., Chow, A., Rudy, B., and McBain, C. J. (2002). Developmental expression of potassium-channel subunit Kv3.2 within subpopulations of mouse hippocampal inhibitory interneurons. Hippocampus 12, 137–148. doi: 10.1002/hipo.1104
Varga, C., Oijala, M., Lish, J., Szabo, G. G., Bezaire, M., Marchionni, I., et al. (2014). Functional fission of parvalbumin interneuron classes during fast network events. eLife 3:e04006. doi: 10.7554/eLife.04006
Veit, J., Hakim, R., Jadi, M. P., Sejnowski, T. J., and Adesnik, H. (2017). Cortical gamma band synchronization through somatostatin interneurons. Nat. Neurosci. 20, 951–959. doi: 10.1038/nn.4562
Verret, L., Mann, E. O., Hang, G. B., Barth, A. M. I., Cobos, I., Ho, K., et al. (2012). Inhibitory interneuron deficit links altered network activity and cognitive dysfunction in Alzheimer model. Cell 149, 708–721. doi: 10.1016/j.cell.2012.02.046
Wen, T. H., Binder, D. K., Ethell, I. M., and Razak, K. A. (2018). The perineuronal ‘Safety’ Net? Perineuronal net abnormalities in neurological disorders. Front. Mol. Neurosci. 11:270. doi: 10.3389/fnmol.2018.00270
Whitebirch, A. C. (2020). Acute mouse brain slicing to investigate spontaneous hippocampal network activity. J. Vis. Exp. doi: 10.3791/61704
Whittington, M. A., Cunningham, M. O., LeBeau, F. E. N., Racca, C., and Traub, R. D. (2011). Multiple origins of the cortical gamma rhythm. Dev. Neurobiol. 71, 92–106. doi: 10.1002/dneu.20814
Whittington, M. A., Traub, R. D., and Jefferys, J. G. R. (1995). Synchronized oscillations in interneuron networks driven by metabotropic glutamate receptor activation. Nature 373, 612–615. doi: 10.1038/373612a0
Xiao, M. F., Xu, D., Craig, M. T., Pelkey, K. A., Chien, C. C., Shi, Y., et al. (2017). NPTX2 and cognitive dysfunction in Alzheimer’s Disease. eLife 6:e23798. doi: 10.7554/eLife.23798
Yan, X., Hu, Y., Wang, B., Wang, S., and Zhang, X. (2020). Metabolic dysregulation contributes to the progression of Alzheimer’s disease. Front. Neurosci. 14:530219. doi: 10.3389/fnins.2020.530219
Yang, S., Cacquevel, M., Saksida, L. M., Bussey, T. J., Schneider, B. L., Aebischer, P., et al. (2015). Perineuronal net digestion with chondroitinase restores memory in mice with tau pathology. Exp. Neurol. 265, 48–58. doi: 10.1016/j.expneurol.2014.11.013
Zemankovics, R., Veres, J. M., Oren, I., and Hájos, N. (2013). Feedforward inhibition underlies the propagation of cholinergically induced gamma oscillations from hippocampal CA3 to CA1. J. Neurosci. 33, 12337–12351. doi: 10.1523/JNEUROSCI.3680-12.2013
Keywords: gamma oscillations, 5xFAD, parvalbumin interneurons, Alzheimer’s disease, carbachol, kainate
Citation: Mackenzie-Gray Scott CA,Pelkey KA, Caccavano AP, Abebe D, Lai M, Black KN, Brown ND, Trevelyan AJ and McBain CJ (2022) Resilient Hippocampal Gamma Rhythmogenesis and Parvalbumin-Expressing Interneuron Function Before and After Plaque Burden in 5xFAD Alzheimer’s Disease Model. Front. Synaptic Neurosci. 14:857608. doi: 10.3389/fnsyn.2022.857608
Received: 18 January 2022; Accepted: 14 April 2022;
Published: 11 May 2022.
Edited by:
Maria Clara Gravielle, University of Buenos Aires, ArgentinaReviewed by:
Oliver Kann, Heidelberg University, GermanyFlorence P. Varodayan, Binghamton University - SUNY, United States
Copyright © 2022 Mackenzie-Gray Scott, Pelkey, Caccavano, Abebe, Lai, Black, Brown, Trevelyan and McBain. This is an open-access article distributed under the terms of the Creative Commons Attribution License (CC BY). The use, distribution or reproduction in other forums is permitted, provided the original author(s) and the copyright owner(s) are credited and that the original publication in this journal is cited, in accordance with accepted academic practice. No use, distribution or reproduction is permitted which does not comply with these terms.
*Correspondence: Connie A. Mackenzie-Gray Scott, Yy5tYWNrZW56aWUtZ3JheS1zY290dDJAbmV3Y2FzdGxlLmFjLnVr; Chris J. McBain, bWNiYWluY0BtYWlsLm5paC5nb3Y=