- Inria Center at the University of Bordeaux/LaBRI, Talence, France
In today's digital information age, human exposure to visual artifacts has reached an unprecedented quasi-omnipresence. Some of these cultural artifacts are elevated to the status of artworks which indicates a special appreciation of these objects. For many persons, the perception of such artworks coincides with aesthetic experiences (AE) that can positively affect health and wellbeing. AEs are composed of complex cognitive and affective mental and physiological states. More profound scientific understanding of the neural dynamics behind AEs would allow the development of passive Brain-Computer-Interfaces (BCI) that offer personalized art presentation to improve AE without the necessity of explicit user feedback. However, previous empirical research in visual neuroaesthetics predominantly investigated functional Magnetic Resonance Imaging and Event-Related-Potentials correlates of AE in unnaturalistic laboratory conditions which might not be the best features for practical neuroaesthetic BCIs. Furthermore, AE has, until recently, largely been framed as the experience of beauty or pleasantness. Yet, these concepts do not encompass all types of AE. Thus, the scope of these concepts is too narrow to allow personalized and optimal art experience across individuals and cultures. This narrative mini-review summarizes the state-of-the-art in oscillatory Electroencephalography (EEG) based visual neuroaesthetics and paints a road map toward the development of ecologically valid neuroaesthetic passive BCI systems that could optimize AEs, as well as their beneficial consequences. We detail reported oscillatory EEG correlates of AEs, as well as machine learning approaches to classify AE. We also highlight current limitations in neuroaesthetics and suggest future directions to improve EEG decoding of AE.
1 Introduction
Modern humans live in environments in which we are almost constantly confronted with artifacts. Many of these artifacts primarily serve instrumental purposes, i.e., they are designed to fulfill specific practical goals. Although, the design of many of these artifacts also take aesthetic considerations into account, a subset of artifacts are especially appreciated for their aesthetic qualities and designated with the label “art” or “artwork”. The field of neuroaesthetics studies the neural correlates of aesthetic experience (AE) (Vessel, 2022). While some of this research looks at aesthetics from the lens of economics and aims to discover sensory objects feature that increase economic interests such as product sales (Costa-Feito et al., 2019), an increasing number of studies report positive correlations between AEs and health and wellbeing (e.g., Fancourt and Finn, 2019; see Skov and Nadal, 2023, for a critique of such reports). With the internet and social media, many humans have now an unprecedented access to artworks. The abundance of available digital art together with advances in machine learning could allow for the personalization of AE with brain-computer-interfaces (BCI), and thus increase a kind of user experience that might positively affect human existence. BCIs are systems that allow direct communication between computers and brain signals (Vidal, 1973). For example, BCIs that decode correlates of AE could be used in combination with recommender systems that curate personalized art exhibitions in virtual museums in order to optimize visitor satisfaction. Although, the personalization of AE based on behavioral data already exists in digital spaces such as social media, such personalization algorithms often require explicit user feedback which can interrupt AE and thereby diminish user experience.
In order to alleviate this current limitation of AE personalization, the development of human-machine-interfaces that implicitly measure individual AE in real time offers a promising lead. One type of human-machine-interface that seems particularly suited to this endeavor are passive non-invasive BCI such as those based on Electroencephalography (EEG). Previous neuroimaging during AEs focused mostly on correlating beauty/pleasantness ratings with functional Magnetic Resonance Imaging (fMRI) signals (Vessel, 2022) and EEG Event-Related-Potentials (ERPs) (Jacobsen and Klein, 2022) in unecological lab conditions. While fMRI cannot be used in ecological conditions, EEG is used in many environments, even real museums (King and Parada, 2021). However, aesthetic preference does not necessarily arise time-locked to stimulus onset and might develop over larger timescales (Carbon, 2023). Thus, in addition to ERPs, EEG oscillatory features can contain information about aesthetic preference (Strijbosch et al., 2022). Therefore, EEG oscillatory features could be promising for aesthetic passive BCIs in ecological conditions outside the lab. However, the neuro-cognitive mechanisms of art experience in general and art preference in particular, remain unclear and more empirical research on neuro-aesthetics is required. This article summarizes the state-of-the-art in aesthetic preference decoding based on oscillatory EEG features and develops a road map toward the development of ecologically valid passive EEG-based BCI systems that could personalize user AE.
2 Aesthetic experience and art preference
AE can be defined as “a perceptual experience that is evaluative, affectively absorbing and engages comprehension (meaning) processes” (Vessel, 2022). This definition corresponds to the Aesthetic Triad Model (Chatterjee and Vartanian, 2014) which, based mostly on experimental aesthetics fMRI research, considers AE emerging from the interaction of three brain systems: sensory-motor, emotion-valuation, and knowledge-meaning. Similarly, Schaeffer (2015) postulates three main components of AE: attention, affect, and reward. To be precise, Schaeffer uses the French word “emotion” instead of “affect,” but following the definitory framework proposed by Schiller et al. (2023), we use the more general term “affect” composed of valence, arousal, and motivation. In this framework, “emotions” are considered a subset of conscious affects. Although, AEs are often related to the perception of artworks, the definitions above remains neutral with regards to the categorical status of the perceived objects, and it seems plausible that non-art objects, e.g., natural landscapes, can evoke similar AEs to art-related AEs. Nonetheless, the scope of this mini-review is constrained to the decoding of AEs during art watching which seems the most practical condition for improving AE with a BCI.
It seems evident that attentional information regulation is needed for any kind of experience. And indeed, works of art often invite attention. However, attentional EEG markers alone might not be ideal or sufficient features for AE decoding. Both negatively and positively valenced stimuli draw high amounts of attention due to the evolutionary significance of searching rewards and avoiding threats (Karim et al., 2017). Furthermore, both internally and externally oriented attentional mechanisms are involved in the processing of visual AE (Ansorge et al., 2022).
AEs cover a vast spectrum of different affective experiences (Menninghaus et al., 2018) which makes it very difficult to train a machine learning model to discriminate between all different classes of AE (Mühl et al., 2014). However, as we are primarily concerned with improving AE of art watching, we can simplify the problem to decoding and ranking aesthetic preference for various art stimuli.
Many neuroaesthetic fMRI studies reported activation of reward and pleasure processing areas in the brain during aesthetic experiences (Chuan-Peng et al., 2019). In the aesthetic literature, “pleasantness” often refers to objective stimulus features related to beauty (e.g., harmony, symmetry) (e.g., Babiloni et al., 2013). We, on the other hand, define “pleasantness” as related to an activation of hedonic brain systems that might be independent of objective stimulus properties. As Berridge and Kringelbach (2015) show, the reward system of the human brain consists of two major independent pathways, one related to pleasure and “liking” generated by opioids and endocannabinoids, and another one related to motivation and “wanting” produced by dopamine. Furthermore, activation of these reward circuits may not lead to the conscious experience of “liking” or “wanting” (Berridge and Winkielman, 2010). These types of experiences are difficult to decode, because training labels come from subjective aesthetic ratings. Therefore, preferring one aesthetic stimulus over another aesthetic stimulus is not reducible to feeling a higher amount of pleasure while perceiving this stimulus compared to the other one. Nor is beautiful art always liked more than non-beautiful art (Muth et al., 2020). The independence of these two reward systems has led scientists in the tradition of Kantian aesthetics (Kant, 1983) to affirm the nature of aesthetic appreciation as inherently disinterested (e.g Sarasso et al., 2020). Indeed, empirical data suggest that aesthetic pleasure does not need to be correlated with extrinsic motivations such as the desire to own or control the appreciated aesthetic object (Chatterjee and Vartanian, 2016). However, from this it does not follow that aesthetic appreciation is essentially disinterested, because even though the aesthetic object might not be perceived in an instrumental fashion to fulfill an external goal, beholders might be intrinsically motivated and therefore interested to interact with an aesthetic object, because they will experience intrinsic rewards through this interaction. It has been shown that motor activity can be suppressed during AEs such as the perception of beauty (Kawabata and Zeki, 2004). When Sarasso et al. (2020) interpret this phenomenon as evidence for aesthetic disinterest, they miss that movement is often a constitutional part of AE and that moments of stillness can lead to further motor interaction with an artwork (Kühnapfel et al., 2023). Because AEs are intrinsically rewarding, they motivate to prolong the AE and to search for more AEs in the future (Reeves, 1989). Still, the exact role of rewards in AE remains controversial. Some scientists claim that aesthetic appreciation can be reduced to the valuation of sensory objects (Skov and Nadal, 2020), whereas others disagree with this reduction (Vessel, 2022). Nonetheless, we hypothesize that EEG correlates related to reward processing should be discriminative for aesthetic preferences.
3 Oscillatory EEG correlates of visual aesthetic preference
As many Machine Learning classification algorithms require hand-crafted features, the following section will describe oscillatory EEG correlates of visual aesthetic preference that could constitute informative features. We conducted a literature review by searching public databases, as well as references in the neuroaesthetic literature. The search query was: “+aesthetic*|art*|paint*+EEG|brain|neur*+oscillat*|wav*|frequen*|rhythm.” We only included studies reporting oscillatory EEG correlates of AE for static visual art stimuli in naturalistic conditions. These findings are discussed as potential correlates of attention, affect and reward during AE (see Table 1 for a summary).
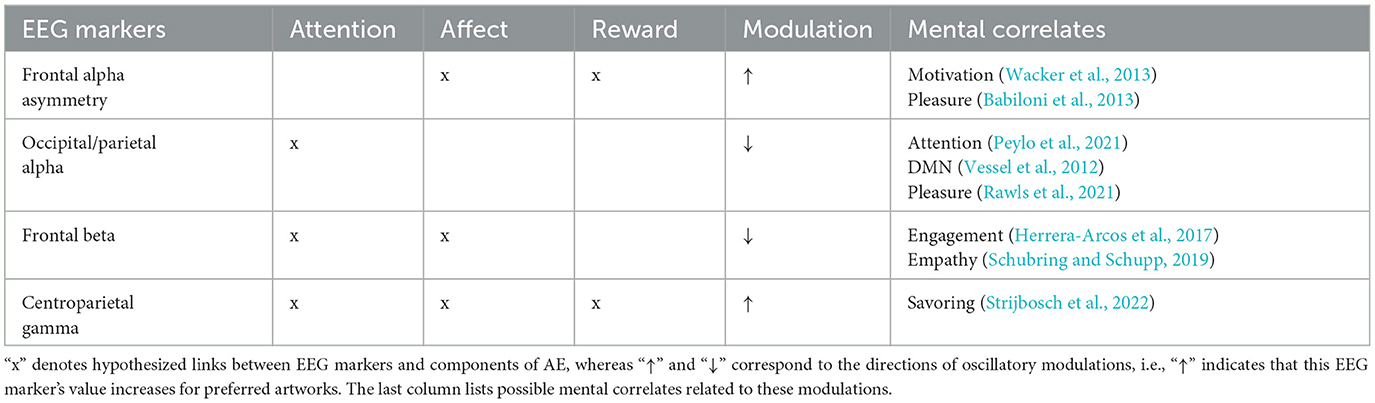
Table 1. This table summarizes EEG rhythm modulations correlated to attentional, affective and reward components of AE.
3.1 Attention
Although correlates of visual attention are widely studied with EEG and harnessed in BCI (Nam et al., 2018), to our knowledge only Rawls et al. (2021) report rhythm modulations commonly associated with visual attention that were informative of art preference. Instructing 44 subjects to give preference ratings to binarized Jackson Pollock paintings and computer generated cantor fractals after 4s viewing time, they found a suppression of the parietal alpha rhythm correlated with art preference. Parietal alpha modulations have been implicated in attentional processing (Peylo et al., 2021) and the Default Mode Network (DMN) which has been linked to aesthetically moving art watching (Vessel et al., 2012, 2013). However, the authors noted that it might be related to stimulus properties such as visual complexity and not necessarily be related to preference. Another brain rhythm associated with the DMN, the theta rhythm, was investigated by Strijbosch et al. (2022) during aesthetically moving art watching, but they found no relation between theta modulations and AE.
3.2 Affect
Many of the brain areas involved in affective processing are located subcortically which makes it difficult to measure their activity with EEG (Mühl et al., 2014). Nonetheless, beta suppression has been reported during empathy and affective processing in general (Schubring and Schupp, 2019). Herrera-Arcos et al. (2017) conducted a mobile EEG study (#subjects = 25) during an Otto Dix exhibition in a real museum with a commercial Muse EEG. Their analyses show a correlation between beta suppression and artwork preference. This beta suppression was interpreted as related to emotional engagement by the authors. However, it is also possible that the measured beta suppression was generated by motor activity, especially under mobile recording conditions (Pope et al., 2022). Still, motor activity could potentially be related to affective processing and informative of aesthetic preference (Kühnapfel et al., 2023).
3.3 Reward
fMRI scans during AEs often report activation of brain areas involved in reward processing (e.g., Kawabata and Zeki, 2004). Unfortunately, similarly to affective processing, many reward related areas in the brain are also subcortical and hard to measure. Still, reward related-signals in the frontal cortex could be measurable by frontal EEG electrode, an hypothesis which is strengthened by a multitude of experimental reports of a relation between frontal alpha asymmetry (FAA) in the EEG and reward processing across different reward modalities (see Sabu et al., 2022 for a review). Although, FAA has been linked to pleasure and liking, some suggest that it is generated by motivational dopamine release (Wacker et al., 2013). Babiloni et al. (2013) collected mobile EEG data (#subjects = 25) during a Dutch Golden Age exhibition in a museum and found a correlation between FAA and beauty/pleasantness ratings. Babiloni et al. (2015) reproduced this correlation during a Titian exhibition (#subjects = 27). Cheung et al. (2019) also reported a link between FAA and beauty ratings (#subjects = 20) for Western art displayed on a screen. Therefore, FAA could be a good marker for an AE that motivates to prolong the AE or to reproduce it in the future. However, one has to keep in mind that FAA is related to motivational processing in general and not limited to a particular valence (Lacey and Gable, 2022). Thus, artworks that evoke anger or other negatively valenced approach-motivation related experiences could also generate FAA.
3.4 Mixed component
Strijbosch et al. (2022) studied the neural dynamics during aesthetically moving experiences with EEG. Showing 35 participants a wide range of diverse artworks for 6 s, they reported a gamma increase 1 s after stimulus presentation until the end of the trial. This was interpreted as a correlate of savoring, a process of up-regulation of positive affect by sustaining attention on an experience. Similar gamma modulations were found with regards to other types of positively affecting AE, such as enjoying the taste of chocolate (Berk et al., 2016; Silver et al., 2018). EEG gamma rhythms are often contaminated by muscle artifacts and are, therefore, commonly filtered out in EEG analysis (Jeunet et al., 2018). And although, Strijbosch et al. (2022) followed a rigorous artifact removal protocol, muscle contamination remains possible.
Now that we have reviewed potential oscillatory EEG markers of aesthetic preference, we will discuss their use as features for machine learning classification of AE.
4 Classification of aesthetic preference based on oscillatory EEG markers
Almost no publications reporting aesthetic preference classification results based on EEG signals exist to our knowledge. Fraiwan et al. (2023) reported an aesthetic enjoyment classification accuracy above 98% using a deep neural network and Multiscale Entropy features on a mobile data set from Cruz-Garza et al. (2017) (#subjects = 28). EEG Entropy measure have been shown to contain meaningful information for emotion decoding (Patel et al., 2021) and decoding of aesthetical preference of music (Carpentier et al., 2019). However, EEG classification almost never yields such high performance in practice and we should remain skeptical (Jeunet et al., 2018). The data could contain informative muscular artifacts, as the authors do not report any artifact removal protocol or the trained model could have been overfitted on the data, as deep learning models often are (Goodfellow et al., 2016). Nonetheless, brain entropy measures could be useful for visual aesthetic preference decoding.
Mazzacane et al. (2023) used a novel classification algorithm based on temporal decision trees (Sciavicco and Stan, 2020) to extract symbolic rules ralating EEG amplitudes of specific frequency bands and electrode locations to aesthetic liking or disliking in an ecological museum context (#subjects = 16). The authors report high classification performance using features from beta and gamma bands that could be related to affective and reward processing. However, we remain skeptical to their claim that their temporal decision tree classification algorithm makes muscle artifact removal unnecessary and hypothesize that the beta and gamma activity used by the classifier could be generated by muscular and movement artifacts since they were not controlled for, cleaned nor removed.
Surprisingly, more established EEG classification algorithms (see Lotte et al., 2018, for a review) have not been applied to AE decoding to our knowledge, and should be explored in the neuroaesthetic domain. Deep learning classifiers (e.g., Lawhern et al., 2016; Schirrmeister et al., 2017) might be worth investigating as well, because our theoretical and empirical knowledge about which EEG features contain discriminant information for AE decoding is lacking. However, large public datasets required to benchmark decoding algorithms of AE are missing (Jayaram and Barachant, 2018). For now, BCI classification algorithms based on Riemannian Geometry seem to work best and often outperform deep learning methods (Congedo et al., 2017; Roy et al., 2022) which is why some state-of-the-art EEG BCI decoding implements Riemmanian Geometry in deep neural network architectures (e.g., Kobler et al., 2022).
5 Discussion
Our literature search yielded six publications that investigated oscillatory EEG markers of AE that reported EEG modulations in alpha, beta and gamma frequency bands. This variability could be explainable by different experimental protocols, e.g., artworks used and aesthetic ratings. Thus, robust EEG features to decode aesthetic preference that generalize to all types of AE remain unclear.
5.1 Pitfalls
We related specific EEG frequency bands with attentional, affective and reward-related components of AE, however neural rhythms can not be one-to-one mapped to mental states (Brouwer et al., 2015). Furthermore, EEG measurements suffer from high variability within and between subjects and even data from the same subject might be very different if recorded at different times (Fairclough and Lotte, 2020). Additionally, it has been shown that context influences AE, and AEs in laboratory conditions are quite different from AE in the wild (Carbon, 2020). As such, we do not know whether correlates of AE discovered during a laboratory experiment will generalize to natural contexts outside the laboratory. To our knowledge, only FAA has been reported in both conditions. However, in experimental conditions, even in real museum settings, subjects often have to look at an affective stimulus, even if they would prefer not to, which influences FAA (Lacey and Gable, 2022).
Due to the need of a preference groundtruth, and without direct access to reward processing information in the brain, aesthetic preference decoding has to rely on explicit rating tasks that assign a subjective value to an artwork. This explicit aesthetic judgment task can introduce confounds, as shown by ERPs that only appear with aesthetic rating tasks (Höfel and Jacobsen, 2017). Similarly, some EEG oscillations might be related to aesthetic judgment and not AE per se. Therefore, these might not appear in natural art watching conditions.
Different artworks might evoke very different AEs and consequently different EEG signals. Additionally, various visual stimulus features are known to affect the EEG signal such as luminance (Eroğlu et al., 2020) or complexity (Rawls et al., 2021). Therefore, it remains unclear, whether empirical results gathered with one type of art stimuli will generalize to other types of art. Last, but not least, natural visual AE is an embodied experience that involves motor processes such as eye movements. Therefore, EEG data of such experiences should be subjected to similar rigorous artifact removal protocols as other mobile EEG imaging scenarios (Gorjan et al., 2022).
5.2 Future work
It was mentioned before that the subcortical location of many reward and affect processing areas involved in aesthetic processing makes EEG decoding difficult. Nonetheless, their activity can be estimated using computational modeling. Singer et al. (2023) used fMRI-informed EEG models of reward processing to decode activity from the ventral striatum using EEG and demonstrated good model performance across aesthetic and non aesthetic domains, as well as across subjects. Another possibility that could improve decoding of aesthetic appreciation would be to use EEG source localization algorithms with realistic headmodels and define reward areas as regions of interest. Although EEG source localization is limited in its accuracy, empirical results have shown that extracting information from source localized regions of interest can improve classification performance (Edelman et al., 2019). One limitation of this approach is that source localization algorithms are computationally expensive. Potentially, investigating functional connectivity of EEG electrodes (Gonzalez-Astudillo et al., 2022), might be an alternative and/or a complement to anatomical source space feature extraction. Brain networks such as the DMN are activated during AE (Vessel, 2022). Kontson et al. (2015) reported increased functional connectivity between frontal and parieto-occipital EEG electrodes during art watching compared to a baseline. However, they did not investigate functional connectivity for different aesthetic preference levels.
Finally, AE is not exhausted by attention, affect, and reward, but also includes semantic and motor processes. While decoding semantic content in EEG signals is very difficult (e.g., Gifford et al., 2022), EEG motor correlates are relatively well studied in BCI (Yuan and He, 2014). Neuroaesthetic research has shown that art watching can activate motor related brain areas which could be related to motor simulation (e.g., Umiltá et al., 2012) and empathy (Gallese, 2017). Unfortunately, the link between embodied cognition and art appreciation has not been shown using neuroimaging. However, correlations between motor priming and art appreciation have been reported (e.g., Ticini et al., 2014). We hypothesize that EEG correlates of motor control such as mu rhythm modulation could, therefore, be informative of AE.
6 Concluding remarks
Neuroaesthetic research on AE decoding is still in its infancy. We reviewed sparse and inconsistent reports of EEG oscillatory correlates of AE. Although, we focused on AE of visual art here, empirical data suggests that other AEs are not fundamentally different (Vessel, 2022). Thus, some of the neural correlates of art preference reviewed here could generalize to other types of preference. Still, our focus on EEG for AE decoding constitutes a limitation. Other physiological signals, e.g., heart rate and skin conductance, are informative of affective states in general (Shu et al., 2018; Rinella et al., 2022) and of AE in particular (Kühnapfel et al., 2023). Indeed, combining EEG with these signals can improve mental state classification (Hogervorst et al., 2014) and should be explored for AE decoding. Overall, there remain a number of challenges to solve before BCI could reliably and rigorously decode AE from EEG, for different artworks and different contexts. We hope this mini-review modestly contributed to identify these challenges and to propose relevant directions for future works.
Author contributions
MW: Conceptualization, Data curation, Investigation, Methodology, Writing — original draft. FL: Conceptualization, Funding acquisition, Methodology, Project administration, Supervision, Writing — review & editing.
Funding
The author(s) declare financial support was received for the research, authorship, and/or publication of this article. This research was funded by CHISTERA project BITSCOPE (grant ANR-21-CHRA-0003-01).
Conflict of interest
The authors declare that the research was conducted in the absence of any commercial or financial relationships that could be construed as a potential conflict of interest.
The author(s) declared that they were an editorial board member of Frontiers, at the time of submission. This had no impact on the peer review process and the final decision.
Publisher's note
All claims expressed in this article are solely those of the authors and do not necessarily represent those of their affiliated organizations, or those of the publisher, the editors and the reviewers. Any product that may be evaluated in this article, or claim that may be made by its manufacturer, is not guaranteed or endorsed by the publisher.
References
Ansorge, U., Pelowski, M., Quigley, C., Peschl, M., and Leder, H. (2022). Art and perception: using empirical aesthetics in research on consciousness. Front. Psychol. 13:895985. doi: 10.3389/fpsyg.2022.895985
Babiloni, F., Cherubino, P., Graziani, I., Trettel, A., Infarinato, F., Picconi, D., et al. (2013). “Neuroelectric brain imaging during a real visit of a fine arts gallery: a neuroaesthetic study of XVII century dutch painters,” in Proceedings of the Annual International Conference of the IEEE/EMBS (Osaka: IEEE), 6179–6182. doi: 10.1109/EMBC.2013.6610964
Babiloni, F., Rossi, D., Cherubino, P., Trettel, A., Picconi, D., Maglione, A., et al. (2015). “The first impression is what matters: a neuroaesthetic study of the cerebral perception and appreciation of paintings by titian,” in Proceedings of the Annual International Conference of the IEEE/EMBS (Milan: IEEE), 7990–7993. doi: 10.1109/EMBC.2015.7320246
Berk, L., Lee, J., Mali, D., Lohman, E., Bains, G., Daher, N., et al. (2016). Chocolate and the brain: Cacao increases power spectral density (μv2) of EEG gamma wave band activity (31–40hz) which is associated with neuronal synchronization, enhanced cognition, memory, recall and physiological benefits. FASEB J. 30. doi: 10.1096/fasebj.30.1_supplement.679.14
Berridge, K., and Kringelbach, M. L. (2015). Pleasure systems in the brain. Neuron 86, 646–664. doi: 10.1016/j.neuron.2015.02.018
Berridge, K., and Winkielman, P. (2010). What is an unconscious emotion? (the case of unconscious “liking”). Cogn. Emot. 17, 181–211. doi: 10.1080/02699930302289
Brouwer, A., Zander, T., van Erp, J., Korteling, J., and Bronkhorst, A. (2015). Using neurophysiological signals that reflect cognitive or affective state: six recommendations to avoid common pitfalls. Front. Neurosci. 9:136. doi: 10.3389/fnins.2015.00136
Carbon, C. C. (2020). Ecological art experience: how we can gain experimental control while preserving ecologically valid settings and contexts. Front. Psychol. 11:800. doi: 10.3389/fpsyg.2020.00800
Carbon, C. C. (2023). About the need for a more adequate way to get an understanding of the experiencing of aesthetic items. Behav. Sci. 13. doi: 10.3390/bs13110907
Carpentier, S., McCulloch, A., Brown, T., Faber, S., Ritter, P., Wang, Z., et al. (2019). Complexity matching: brain signals mirror environment information patterns during music listening and reward. J. Cogn. Neurosci. 32, 1–11. doi: 10.1101/693531
Chatterjee, A., and Vartanian, O. (2014). Neuroaesthetics. Trends Cogn. Sci. 18, 370–375. doi: 10.1016/j.tics.2014.03.003
Chatterjee, A., and Vartanian, O. (2016). Neuroscience of aesthetics. Ann. N.Y. Acad. Sci. 1369, 172–194. doi: 10.1111/nyas.13035
Cheung, M. C., Law, D., Yip, J., and Wong, C. W. Y. (2019). Emotional responses to visual art and commercial stimuli: implications for creativity and aesthetics. Front. Psychol. 10:14. doi: 10.3389/fpsyg.2019.00014
Chuan-Peng, H., Huang, Y., Eickhoff, S. B., Peng, K., and Sui, J. (2019). Seeking the beauty center in the brain: a meta-analysis of fmri studies of beautiful human faces and visual art. Cogn. Affect. Behav. Neurosci. 20, 1200–1215. doi: 10.3758/s13415-020-00827-z
Congedo, M., Barachant, A., and Bhatia, R. (2017). Riemannian geometry for EEG-based brain-computer interfaces; a primer and a review. Brain-Comput. Int. 4, 1–20. doi: 10.1080/2326263X.2017.1297192
Costa-Feito, A., González-Fernández, A.M., Rodríguez-Santos, C., and Cervantes-Blanco, M. (2019). Electroencephalography in consumer behaviour and marketing: a science mapping approach. Humanit. Soc. Sci. Commun. 10:474. doi: 10.1057/s41599-023-01991-6
Cruz-Garza, J., Brantley, J., Nakagome, S., Kontson, K., Robleto, D., Contreras-Vidal, J., et al. (2017). Mobile EEG recordings in an art museum setting. IEEE Dataport.
Edelman, B. J., Meng, J., Suma, D., Zurn, C. A., Nagarajan, E., Baxter, B. S., et al. (2019). Noninvasive neuroimaging enhances continuous neural tracking for robotic device control. Sci. Robot. 4:aaw6844. doi: 10.1126/scirobotics.aaw6844
Eroğlu, K., Kayikçioğlu, T., and Osman, O. (2020). Effect of brightness of visual stimuli on EEG signals. Behav. Brain Res. 382:112486. doi: 10.1016/j.bbr.2020.112486
Fairclough, S., and Lotte, F. (2020). Grand challenges in neurotechnology and system neuroergonomics. Front. Neuroergonom. 1:602504. doi: 10.3389/fnrgo.2020.602504
Fancourt, D., and Finn, S. (2019). What is the evidence on the role of the arts in improving health and well-being? A scoping review. Nord. J. Arts Cult. Health 2, 77–83. doi: 10.18261/issn.2535-7913-2020-01-08
Fraiwan, M., Alafeef, M., and Almomani, F. (2023). Gauging human visual interest using multiscale entropy analysis of EEG signals. J. Ambient. Intell. Humaniz. Comput. 12, 2435–2447. doi: 10.1007/s12652-020-02381-5
Gallese, V. (2017). “The empathic body in experimental aesthetics-embodied simulation and art,” in Empathy, ed. W. Lux (London: Palgrave Macmillan), 181–199. doi: 10.1057/978-1-137-51299-4_7
Gifford, A. T., Dwivedi, K., Roig, G., and Cichy, R. M. (2022). A large and rich EEG dataset for modeling human visual object recognition. Neuroimage 264:119754. doi: 10.1016/j.neuroimage.2022.119754
Gonzalez-Astudillo, J., Cattai, T., Bassignana, G., Corsi, M. C., and Fallani, F. D. V. (2022). Network-based brain-computer interfaces: principles and applications. J. Neural Eng. 18. doi: 10.1088/1741-2552/abc760
Gorjan, D., Gramann, K., De Pauw, K., and Marusic, U. (2022). Removal of movement-induced EEG artifacts: current state of the art and guidelines. J. Neural Eng. 19. doi: 10.1088/1741-2552/ac542c
Herrera-Arcos, G., Tamez-Duque, J., Acosta, E., Kwan-Loo, K., de Alba, M., Tamez-Duque, U., et al. (2017). Modulation of neural activity during guided viewing of visual art. Front. Hum. Neurosci. 11:581. doi: 10.3389/fnhum.2017.00581
Höfel, L., and Jacobsen, T. (2017). Electrophysiological indices of processing symmetry and aesthetics. J. Psychophysiol. 21, 9–21. doi: 10.1027/0269-8803.21.1.9
Hogervorst, M., Brouwer, A., and Erp, J. (2014). Combining and comparing EEG, peripheral physiology and eye-related measures for the assessment of mental workload. Front. Neurosci. 8:322. doi: 10.3389/fnins.2014.00322
Jacobsen, T., and Klein, S. (2022). “Electrophysiology,” in The Oxford Handbook of Empirical Aesthetics, ed. V. Nadal (Oxford: Oxford University Press), 291–307. doi: 10.1093/oxfordhb/9780198824350.013.13
Jayaram, V., and Barachant, A. (2018). MOABB: trustworthy algorithm benchmarking for BCIs. J. Neural Eng. 15, 6.
Jeunet, C., Debener, S., Lotte, F., Mattout, J., Scherer, R., Zich, C., et al. (2018). “Mind the traps! Design guidelines for rigorous BCI experiments,” in Brain-computer Interfaces Handbook: Technological and Theoretical Advances, eds C. S. Nam, A. Nijholt, and F. Lotte (Baton Rouge, FL: CRC Press), 613–634. doi: 10.1201/9781351231954-32
Kant, I. (1983). “Kritik der urteilskraft,” in Kant Werke, ed. W. Weischedel (Darmstadt: Wissenschaftliche Buchgesellschaft).
Karim, A. K. M. R., Proulx, M., de Sousa, A. A., and Likova, L. T. (2017). Do we enjoy what we sense and perceive? A dissociation between aesthetic appreciation and basic perception of environmental objects or events. Cogn. Affect. Behav. Neurosci. 22, 904–951. doi: 10.3758/s13415-022-01004-0
Kawabata, H., and Zeki, S. (2004). Neural correlates of beauty. J. Neurophysiol. 91, 1699–1705. doi: 10.1152/jn.00696.2003
King, J. L., and Parada, F. J. (2021). Using mobile brain/body imaging to advance research in arts, health, and related therapeutics. Eur. J. Neurosci. 54, 8364–8380. doi: 10.1111/ejn.15313
Kobler, R., Hirayama, J. I., Zhao, Q., and Kawanabe, M. (2022). Spd domain-specific batch normalization to crack interpretable unsupervised domain adaptation in EEG. Adv. Neural Inf. Process. Syst. 35, 6219–6235.
Kontson, K., Megjhani, M., Brantley, J., Cruz-Garza, J., Nakagome, S., Robleto, D., et al. (2015). Your brain on art: emergent cortical dynamics during aesthetic experiences. Front. Hum. Neurosci. 9:626. doi: 10.3389/fnhum.2015.00626
Kühnapfel, C., Fingerhut, J., Brinkmann, H., Ganster, V., Tanaka, T., Specker, E., et al. (2023). How do we move in front of art? How does this relate to art experience? Linking movement, eye tracking, emotion, and evaluations in a gallery-like setting. Empir. Stud. Arts 42, 86–146. doi: 10.1177/02762374231160000
Lacey, M., and Gable, P. (2022). Frontal asymmetry as a neural correlate of motivational conflict. Symmetry 14. doi: 10.3390/sym14030507
Lawhern, V., Solon, A., Waytowich, N., Gordon, S., Hung, C., Lance, B., et al. (2016). EEGNET: a compact convolutional network for EEG-based brain-computer interfaces. J. Neural Eng. 15:056013. doi: 10.1088/1741-2552/aace8c
Lotte, F., Bougrain, L., Cichocki, A., Clerc, M., Congedo, M., Rakotomamonjy, A., et al. (2018). A review of classification algorithms for EEG-based brain-computer interfaces: a 10 year update. J. Neural Eng. 15, 31005. doi: 10.1088/1741-2552/aab2f2
Mazzacane, S., Coccagna, M., Manzella, F., Pagliarini, G., Sironi, V., Gatti, A., et al. (2023). Towards an objective theory of subjective liking: a first step in understanding the sense of beauty. PLoS ONE 18:e0287513. doi: 10.1371/journal.pone.0287513
Menninghaus, W., Wagner, V., Wassiliwizky, E., Schindler, I., Hanich, J., Jacobsen, T., et al. (2018). What are aesthetic emotions? Psychol. Rev. 126, 171–195. doi: 10.1037/rev0000135
Mühl, C., Allison, B., Nijholt, A., and Chanel, G. (2014). A survey of affective brain computer interfaces: principles, state-of-the-art, and challenges. Brain Comput. Interfaces 1, 66–84. doi: 10.1080/2326263X.2014.912881
Muth, C., Briesen, J., and Carbon, C. C. (2020). “I like how it looks but it is not beautiful”. Poetics 79. doi: 10.1016/j.poetic.2019.101376
Nam, C. S., Nijholt, A., and Lotte, F. (2018). Brain-computer Interfaces Handbook: Technological and Theoretical Advances. Baton Rouge, FL: CRC Press. doi: 10.1201/9781351231954
Patel, P., Rajkumar, R., and Annavarapu, R. N. (2021). EEG-based human emotion recognition using entropy as a feature extraction measure. Brain Inform. 8:20. doi: 10.1186/s40708-021-00141-5
Peylo, C., Hilla, Y., and Sauseng, P. (2021). Cause or consequence? alpha oscillations in visuospatial attention. Trends Neurosci. 44, 705–713. doi: 10.1016/j.tins.2021.05.004
Pope, K., Lewis, T., Fitzgibbon, S., Janani, A., Grummett, T., Williams, P., et al. (2022). Managing electromyogram contamination in scalp recordings: an approach identifying reliable beta and gamma EEG features of psychoses or other disorders. Brain Behav. 12:e2721. doi: 10.1002/brb3.2721
Rawls, E., White, R., Kane, S., and Stevens, C. E. J. (2021). Parametric cortical representations of complexity and preference for artistic and computer-generated fractal patterns revealed by single-trial EEG power spectral analysis. Neuroimage 236:118092. doi: 10.1016/j.neuroimage.2021.118092
Reeves, J. (1989). The interest-enjoyment distinction in intrinsic motivation. Motiv. Emot. 13, 83–103. doi: 10.1007/BF00992956
Rinella, S., Massimino, S., Fallica, P. G., Giacobbe, A., Donato, N., Coco, M., et al. (2022). Emotion recognition: photoplethysmography and electrocardiography in comparison. Biosensors 12:811. doi: 10.3390/bios12100811
Roy, R. N., Hinss, M. F., Darmet, L., Ladouce, S., Jahanpour, E. S., Somon, B., et al. (2022). Retrospective on the first passive brain-computer interface competition on cross-session workload estimation. Front. Neuroergonom. 3:838342. doi: 10.3389/fnrgo.2022.838342
Sabu, P., Stuldreher, I., Kaneko, D., and Brouwer, A. (2022). A review on the role of affective stimuli in event-related frontal alpha asymmetry. Front. Comput. Sci. 4:869123. doi: 10.3389/fcomp.2022.869123
Sarasso, P., Neppi-Modona, M., Sacco, K., and Ronga, I. (2020). Stopping for knowledge: the sense of beauty in the perception-action cycle. Neurosci. Biobehav. Rev. 118, 723–738. doi: 10.1016/j.neubiorev.2020.09.004
Schiller, D., Yu, A. N. C., Alia-Klein, N., Becker, S., Cromwell, H. C., Dolcos, F., et al. (2023). The human affectome. Neurosci. Biobehav. Rev. 105450. doi: 10.1016/j.neubiorev.2023.105450
Schirrmeister, R., Springenberg, J., Fiederer, L., Glasstetter, M., Eggensperger, K., Tangermann, M., et al. (2017). Deep learning with convolutional neural networks for EEG decoding and visualization: convolutional neural networks in EEG analysis. Hum. Brain Mapp. 38, 5391–5420. doi: 10.1002/hbm.23730
Schubring, D., and Schupp, H. (2019). Affective picture processing: alpha- and lower beta-band desynchronization reflects emotional arousal. Psychophysiology 56:e13386. doi: 10.1111/psyp.13386
Sciavicco, G., and Stan, I. E. (2020). “Knowledge extraction with interval temporal logic decision trees,” in Proc. of 27th International Symposium on Temporal Representation and Reasoning (TIME), Leibniz International Proceedings in Informatics (Dagstuhl).
Shu, L., Xie, J., Yang, M., Li, Z., Li, Z., Liao, D., et al. (2018). A review of emotion recognition using physiological signals. Sensors 18:2074. doi: 10.3390/s18072074
Silver, S., Al-Tikriti, R., and Jin, N. (2018). Dark chocolate (70% Cacao) modulates gamma wave frequencies in vigorously active individuals. Loma Linda Univ. Res. Rep. 12. doi: 10.1096/fasebj.2018.32.1_supplement.878.9
Singer, N., Poker, G., Dunsky-Moran, N., Nemni, S., Reznik Balter, S., Doron, M., et al. (2023). Development and validation of an fmri-informed EEG model of reward-related ventral striatum activation. Neuroimage 276:120183. doi: 10.1016/j.neuroimage.2023.120183
Skov, M., and Nadal, M. (2020). A farewell to art: aesthetics as a topic in psychology and neuroscience. Perspect. Psychol. Sci. 15, 630–642.
Skov, M., and Nadal, M. (2023). Can arts-based Interventions Improve Health? A Conceptual and Methodological Critique of Art Therapy [preprint]. doi: 10.31234/osf.io/sp9y3
Strijbosch, W., Vessel, E. A., Welke, D., Mita, O., Gelissen, J., Bastiaansen, M., et al. (2022). On the neuronal dynamics of aesthetic experience: evidence from electroencephalographic oscillatory dynamics. J. Cogn. Neurosci. 34, 461–479. doi: 10.1162/jocn_a_01812
Ticini, L., Rachman, L., Pelletier, J., and Dubal, S. (2014). Enhancing aesthetic appreciation by priming canvases with actions that match the artist's painting style. Front. Hum. Neurosci. 8:391. doi: 10.3389/fnhum.2014.00391
Umiltá, M., Berchio, C., Sestito, M., and Gallese, V. (2012). Abstract art and cortical motor activation: an EEG study. Front. Hum. Neurosci. 6:311. doi: 10.3389/fnhum.2012.00311
Vessel, E. A. (2022). “Neuroaesthetics,” in Encyclopedia of Behavioral Neuroscience, ed. D. Sala (Amsterdam: Elsevier), 661–670. doi: 10.1016/B978-0-12-809324-5.24104-7
Vessel, E. A., Starr, G. G., and Rubin, N. (2012). The brain on art: intense aesthetic experience activates the default mode network. Front. Neurosci. 7:66. doi: 10.3389/fnhum.2012.00066
Vessel, E. A., Starr, G. G., and Rubin, N. (2013). Art reaches within: aesthetic experience, the self and the default-mode network. Front. Neurosci. 7:258. doi: 10.3389/fnins.2013.00258
Vidal, J. (1973). Toward direct brain-computer communication. Annu. Rev. Biophys. Bioeng. 7, 157–189. doi: 10.1146/annurev.bb.02.060173.001105
Wacker, J., Mueller, E., Pizzagalli, D., Hennig, J., and Stemmler, G. (2013). Dopamine-d2-receptor blockade reverses the association between trait approach motivation and frontal asymmetry in an approach-motivation context. Psychol. Sci. 24, 489–497. doi: 10.1177/0956797612458935
Keywords: Electroencephalography (EEG), brain-computer-interfaces, neuroaesthetics, aesthetic preference, oscillatory activity
Citation: Welter M and Lotte F (2024) Ecological decoding of visual aesthetic preference with oscillatory electroencephalogram features—A mini-review. Front. Neuroergon. 5:1341790. doi: 10.3389/fnrgo.2024.1341790
Received: 20 November 2023; Accepted: 19 January 2024;
Published: 21 February 2024.
Edited by:
David Perpetuini, University of Studies G. d'Annunzio Chieti and Pescara, ItalyCopyright © 2024 Welter and Lotte. This is an open-access article distributed under the terms of the Creative Commons Attribution License (CC BY). The use, distribution or reproduction in other forums is permitted, provided the original author(s) and the copyright owner(s) are credited and that the original publication in this journal is cited, in accordance with accepted academic practice. No use, distribution or reproduction is permitted which does not comply with these terms.
*Correspondence: Marc Welter, bWFyYy53ZWx0ZXJAaW5yaWEuZnI=