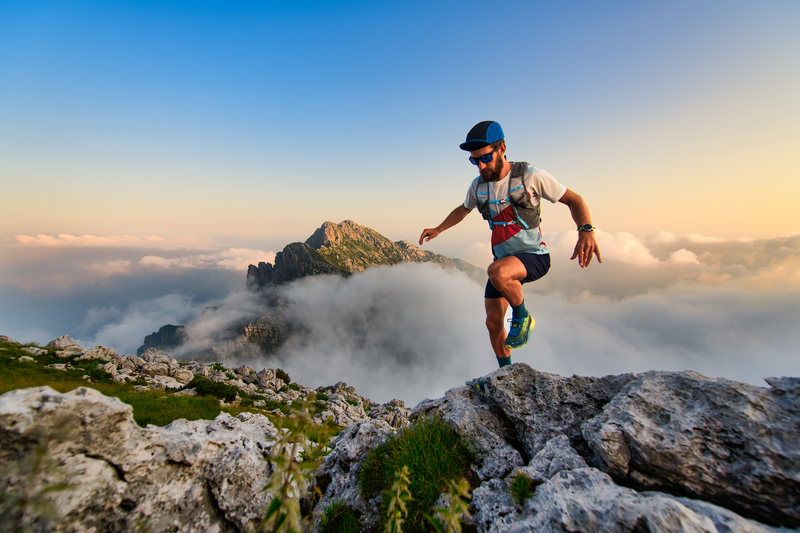
94% of researchers rate our articles as excellent or good
Learn more about the work of our research integrity team to safeguard the quality of each article we publish.
Find out more
MINI REVIEW article
Front. Neuroergonomics , 20 March 2023
Sec. Clinical Neuroergonomics
Volume 4 - 2023 | https://doi.org/10.3389/fnrgo.2023.1142182
This article is part of the Research Topic Insights in Clinical Neuroergonomics View all 4 articles
Introduction: Functional near-infrared spectroscopy (fNIRS) is a non-invasive optical neuroimaging technique used to assess surgeons' brain function. The aim of this narrative review is to outline the effect of expertise, stress, surgical technology, and neurostimulation on surgeons' neural activation patterns, and highlight key progress areas required in surgical neuroergonomics to modulate training and performance.
Methods: A literature search of PubMed and Embase was conducted to identify neuroimaging studies using fNIRS and neurostimulation in surgeons performing simulated tasks.
Results: Novice surgeons exhibit greater haemodynamic responses across the pre-frontal cortex than experts during simple surgical tasks, whilst expert surgical performance is characterized by relative prefrontal attenuation and upregulation of activation foci across other regions such as the supplementary motor area. The association between PFC activation and mental workload follows an inverted-U shaped curve, activation increasing then attenuating past a critical inflection point at which demands outstrip cognitive capacity Neuroimages are sensitive to the impact of laparoscopic and robotic tools on cognitive workload, helping inform the development of training programs which target neural learning curves. FNIRS differs in comparison to current tools to assess proficiency by depicting a cognitive state during surgery, enabling the development of cognitive benchmarks of expertise. Finally, neurostimulation using transcranial direct-current-stimulation may accelerate skill acquisition and enhance technical performance.
Conclusion: FNIRS can inform the development of surgical training programs which modulate stress responses, cognitive learning curves, and motor skill performance. Improved data processing with machine learning offers the possibility of live feedback regarding surgeons' cognitive states during operative procedures.
Neuroergonomics is concerned with the study of brain behavior under naturalist conditions. It encompasses the application of neuroscience, and the biological substrates that underlie brain function, to ergonomics. “Surgical neuroergonomics”, the convergence of two disciplines in the surgical setting, seeks to understand the cognitive processes that underpin operative performance, and inform the design of safer operating systems and more robust training paradigms. Various neuromonitoring techniques have been used to increase the understanding of neural processes that underpin task acquisition in surgery. Examples of such modalities include functional magnetic resonance imaging (fMRI), positron emission topography (PET), electroencephalography (EEG), and functional near-infrared spectrometry (fNIRS).
Of these, the optical neuroimaging modality fNIRS is the most frequently used technique for surgical neuroergonomic applications (Patel et al., 2020; Hannah et al., 2022) as it is safe, radiation free, comparatively inexpensive and enables surgeons to operate freely in naturalistic settings (Modi et al., 2017a; Chen et al., 2020). In fNIRS, near-infrared light is radiated to the brain via light sources and absorbed by oxygenated (HbO2) and deoxygenated (HHb) hemoglobin in the cerebral vasculature (Figure 1). The Modified Beer-Lambert Law estimates the optical parameters (absorption coefficient) from which the brain oxygenation is later derived. Areas of increased cerebral activity correlate with an increase in oxygen requirement, however there are regional (e.g., extracerebral) confounders (Erdogan et al., 2014; Scholkmann et al., 2022) which should be recorded to increase the accuracy of fNIRS signals (Scholkmann et al., 2022). Conventionally, fNIRS uses “long channels”, where light emitters are placed 3 cm away from detectors on the scalp. “Short channels” placed 8–10 mm away from the light source demonstrate strong bias toward extracerebral signals (scalp, skull and meninges); the addition of these channels enables regression of confounding haemodynamic activity (Strangman et al., 2013; Brigadoi and Cooper, 2015; Caldwell et al., 2016; Reddy et al., 2021). Systemic factors (e.g., heart rate, arterial CO2) likewise act as confounders in multiple ways, illustrated by the vasodilatory effect of CO2 which modulates neurovascular coupling, or noise generated from heart rate variations (Lindauer et al., 2010; Kirilina et al., 2012; Caldwell et al., 2016; Scholkmann et al., 2022). Recording systemic responses using instruments such as capnographs or plethysmographs allows for regression of these effects out of fNIRS signals.
Figure 1. (A) Optical neuroimaging cap (B) long and short fNIRS detector channels. Short detector channels measure confounding extracerebral signal whilst long channels measure extracerebral and cerebral signals.
Whilst neural patterns of activation in surgeons related to stress and expertise have previously been reviewed systematically (Arora et al., 2010; Modi et al., 2017b; Hannah et al., 2022), studies conducted since (Crewther et al., 2016; Singh et al., 2018; Modi et al., 2020) merit inclusion for an up-to-date understanding of the progress made in the field of surgical neuroergonomics. In particular, prior reviews narrowed their scope, omitting recent developments in neuroimages of stress and performance enhancement such as robotic assistance or neuroaugmentation (e.g., neurostimulation). To address these gaps, we conducted a narrative review of literature across key areas including surgical skill assessment and proficiency, workload and stress and performance enhancement either through external or robotic assistance or neurostimulation. Across these domains we specifically aimed to determine whether neuroimages can reliably classify surgical skill; whether there are neural signatures of high mental workload; and aimed to characterize the impact of performance enhancing interventions on neural responses in surgeons. Finally, we aimed to highlight key progress areas required in neuroergonomics to implement live cognitive feedback in the theater environment, including trends in data collection and processing. Findings are summarized in Table 1.
Table 1. Summary of current understanding, research gaps and future applications of neuromonitoring and neurostimulation to surgical training.
The versatility and accessibility of fNIRS has enabled the study of cognitive activation patterns with surgical tasks and training, exploring the effects of task complexity and expertise. Areas implicated in motor tasks include the prefrontal cortex (PFC), supplementary motor area (SMA), primary motor cortex (M1), and cerebellum (Modi et al., 2017b; Hannah et al., 2022). The PFC can be further subdivided into the dorsolateral (DLPFC), ventrolateral (VLPFC) and medial-frontopolar subregions. fNIRS array design and channel sensitivity distribution can optimize spatial resolution of the underlying macroanatomy, to better understand specific functional localization (Walia et al., 2021). In simple bimanual tasks such as knot-tying, novices exhibit greater DLPFC activation than experts, this effect diminishes with practice and improved performance (Shetty et al., 2016; Modi et al., 2017b; Izzetoglu et al., 2021; Hannah et al., 2022). Izzetoglu et al. (2021) note deactivation specifically in the left DLPFC. The DLPFC is understood to subserve executive functions: working memory and attention, task-planning and cognitive flexibility (Carlén, 2017). For more complex tasks, such as laparoscopic or robotic suturing, the training period required to first observe PFC excitation and indeed subsequent reduction in PFC activation is substantially longer (Walia et al., 2022). Ohuchida et al. (2009) observed minimal PFC activation in those naïve to laparoscopic suturing with subsequent practice-related PFC attenuation occurring over a protracted time interval. Shetty et al. (2016) describe persistent PFC activation in trainees despite a week of practice and technical performance improvement; similarly, Walia et al. (2022) describe right DLPFC activation in an expert group. Only after years of practice and regular task execution was PFC attenuation observed in expert consultants (Shetty et al., 2016).
Expertise is also characterized by re-organization and redistribution of activation across other motor regions of the cortex; bi-manually skilled experts recruit regions involved in action observation (ventral premotor cortex), motor planning (posterior parietal cortex) and action execution (M1) in order to improve task efficiency (Yang, 2015). Increased supplementary motor area (SMA) and primary motor cortex (M1) activation having been demonstrated to be positively correlated with expertise (Nemani et al., 2018; Hannah et al., 2022). During surgical task planning, motor and parietal regions demonstrate greater activation in experienced surgeons compared to novice students, the latter who exhibit greater bilateral DL, VL and medial PFC activation when making decisions compared to experts, who gain in economy of movement and precision (Bann et al., 2003). Ventromedial activation is also prominent in novices and has been shown to be absent in experts (Leff et al., 2017). The cerebellum's function in motor coordination is reflected in optical neuroimaging surgical data: in laparoscopic tasks, experts demonstrate increased cerebellar activation, whilst novices display attenuated cerebellar activation (Duty et al., 2012).
Correlation between haemodynamic responses across different brain regions demonstrates disparate ‘functional connectivity' between novice and expert surgeons (Nemani et al., 2021; Kamat et al., 2022). For example, Andreu-Perez et al. (2016) demonstrated that during complex bimanual surgical co-ordination tasks, functional connectivity data predicted operator skill level with good accuracy, experts displaying greater motor connectivity whilst novices demonstrated stronger connectivity in prefrontal and premotor regions. In assessing connectivity, the application of fuzzy cognitive maps, capable of describing non-linear relationships and changing connectivity by delivering a graphical representation of a given system, is a complementary technique based on Kosko's causal definition to add to other current methods based on Granger's causal definition, including dynamic causal modeling (Kiani et al., 2022).
Understanding cognitive patterns associated with expertise allows trainers to define neural proficiency, for example via a defined threshold of changes that accompany skill proficiency, which could be spatial distribution of activations, or magnitude of activation in certain regions of interest. Trainees engage in a substantial amount of simulated and virtual learning due to reduced training opportunities and a concern for patient safety, hence a need to ensure simulated and virtual training bestow the same level of skill than physical training (Seymour et al., 2002; Aggarwal et al., 2007; Hogle et al., 2009). Currently, surgical proficiency is assessed against benchmarks such as time taken to perform a skill, numbers of errors made, or subjective rater assessment (Seymour et al., 2002; Aggarwal et al., 2007; Munz et al., 2007). Time-based metrics have been shown to poorly classify trainee expertise (Nemani et al., 2019), whereas fNIRS has been shown to at least match, if not surpass, current metrics in being able to reliably distinguish levels of expertise between trainees, in turn confirming the value of simulated and virtual training (Nemani et al., 2018, 2019; Gao et al., 2021b; Hannah et al., 2022). As highlighted by Nemani et al. (2019) current measures of performance of laparoscopic tasks (such as time to complete task) more frequently misclassify level of motor skill compared to fNIRS.
The singularity of fNIRS may however lie its potential to set a benchmark of neural proficiency for complex, time-consuming tasks, which cannot be assessed with simple time-based measures and are difficult to evaluate beyond patient outcomes and complication rates. To enable this application of optical neuroimaging, it must develop the capacity to integrate and process large amounts of data in real time during prolonged periods, mapping evolving cognitive function throughout an operation to create a benchmark of expert patterns of activation.
Optical neuroimaging can provide an objective measure of cognitive workload. Cognitive workload theory describes intrinsic (inherent to the task), extraneous (the context in which the task is situated), and germane (the processing ability of the subject) cognitive load (Sweller, 2011). Stress adds to extraneous workload and has independently been found to impair surgical performance, affecting both technical and non-technical skills such as communication skills and decision-making (Arora et al., 2010; Crewther et al., 2016; Modi et al., 2018; Singh et al., 2018). Current understanding suggests an inverted-U shape association between PFC activation and mental workload, with activation increasing in-line with workload demands, before attenuating once it passes a critical inflection point at which demands outstrip cognitive capacity (Ossewaarde et al., 2011; Modi et al., 2018; Singh et al., 2018). Modi et al. (2018) demonstrated performance degradation during timed conditions, or temporal stress, associated with relative PFC attenuation. In a subsequent study, they integrated a cognitive stress component (e.g., decision-making) and demonstrated a cumulative effect with temporal stress, PFC deactivation being greatest in temporally and cognitively stressed scenarios (Modi et al., 2020). Furthermore, between-subject variation in behavioral responses to stress may have a basis in the quantitative difference in PFC deactivation: trainees who maintain technical performance under stressful conditions demonstrate increased haemodynamic responses (change in HbO2) in the bilateral ventrolateral PFC and left dorsomedial PFC as compared to trainees whose performance degrades (Modi et al., 2019).
The effect of expertise on stress responses detected by fNIRS is unclear. An improvement in performance under stress conditions has been correlated with increased expertise in certain studies (Modi et al., 2018), whereas others found no association between performance deterioration under stress conditions and expertise (Modi et al., 2019). Research is required to further clarify the relationship between expertise, stress and cortical neuroimage data, which ultimately may indicate a need for a measure of stress resilience independent from expertise. There may be a role for fNIRS not only as a measure for technical proficiency, but also a measure of the neural stress response, which can be targeted by way of individualized surgical training.
The value of measuring neural stress responses may be debated: if neural stress patterns are confined to the cerebral response and do not translate into a deterioration in practice, then what relevance does detecting changes in neural responses have clinically? Conversely, if we are able to correlate increasing stress with decline in performance, then measure the performance decline instead of the neural response. Importantly, neural responses may provide a more accurate measure of stress than physiological measures such as heart rate variability or subjective measures of workload (Modi et al., 2019). Optical neuroimaging may also have a role in assessing the effectiveness of stress reducing interventions such as noise reduction (Engelmann et al., 2014). In addition, establishing a clear mechanism between the onset of stress and neural responses leading to deterioration in performance potentially facilitates interception of stress before progression to performance deterioration, targeting the process before it leads to deleterious effects or clinical harm.
Modern operating tools such as robotic or laparoscopic systems promise to ease the operative burden for trainees and experts. fNIRS has a role in evaluating these systems, by measuring the effect they have on surgeons' brain activation. The superiority of robotic-assisted vs. laparoscopic training is argued for in the literature, citing improved ergonomics, intuitiveness, instrument manipulation and learning curve attenuation (Chandra et al., 2010; Stefanidis et al., 2010; Singh et al., 2018). Indeed, when compared to laparoscopic surgery, surgeons perform better on robotic platforms, despite being less experienced in using them, and exhibit greater PFC activation (Singh et al., 2018).
The advent of robotic systems is also associated with integrated technological aids such as gaze-enhanced or gaze-assisted learning (GEL), a technique proposed to improve motor skills during robotic surgery by constraining the movements of the robot to limit its actions to areas being “gazed” at by the surgeon (Mylonas et al., 2012). This is based on the postulate that as expertise develops, the surgeon's eye movements focus on the target area. An eye tracking device establishes the target area and incorporates this into movement restrictions placed on the robot, which means the surgeon encounters mild resistance if deviating away from the target area. The resistance can easily be overridden by the surgeon who maintains full control. Compared to trainees using robotic systems with no learning aids, GEL leads to greater PFC activation in novice trainees (Mylonas et al., 2012), followed by rapid attenuation of PFC after few practice sessions (James et al., 2013).
At equal expertise level, greater activation of PFC is understood to correlate with increased focus and attention on the task at hand, the cognitive learning curve for motor skills demonstrating first an increase in activation in PFC, M1, SMA, and premotor area (PMA) (Ungerleider et al., 2002; Luft and Buitrago, 2005), followed by a decrease in activation in PFC, SMA and PMA and reorganization of M1 once the task is mastered allowing trainees to lower the intensity of attention directed toward skill performance. What remains to be uncovered is whether short term increase in PFC activation does indeed accelerate the learning curve, or whether it represents the need for increased attention throughout the learning process. Further data collection, including in longitudinal studies of trainees, is necessary to understand the differences between laparoscopic and robotic systems on cognitive performance and the benefits of technological aids. These have the potential to “outsource” processing of data to the machine, and reduce cognitive load, provided it does not impair the neural process of learning.
Direct visualization of cognitive processes which occur during training opens the possibility of targeting these processes with direct cognitive stimulation. Neurostimulation may optimize cognitive responses to surgical training, improving the quality and speed of skill acquisition (Dissanayaka et al., 2017; Biabani et al., 2018; Ciechanski et al., 2018; Patel et al., 2019, 2021; Hung et al., 2021). Transcranial direct-current stimulation (tDCS) is a safe, non-invasive neurostimulation technique (Paulus, 2011) which sustains low-amplitude current (typically < 2 mA) through the brain and modulates neuronal excitability and plasticity. Its intensity is too low to generate neuronal excitability de-novo (Paulus, 2011; Morya et al., 2019). Improvement in motor task performance is conferred safely through tDCS (Bikson et al., 2016; Patel et al., 2019, 2021; Gao et al., 2021a; Hung et al., 2021) along with the prevention of stress induced working memory impairment, which suggests new applications for neuroenhancement (Bogdanov and Schwabe, 2016). Further clarification is still needed regarding the types of skill neurostimulation interventions may enhance as there may be some task-dependent effect on the strength of improvement of learning; in other words, certain tasks may be more improved than others through the use of tDCS. Similarly, the long-term effects on motor retention may also be task specific (Buch et al., 2017).
Technical considerations such as the configuration of the tDCS setup, including the intensity of stimulation delivered and the areas of the cortex to which the stimulation is applied to, should be an area of focus for future study (Batsikadze et al., 2013; Morya et al., 2019). Miniaturization of the neurostimulation technology will facilitate integration into training settings or the live theater. This may be realized through the advent of high definition tDCS which shrinks the large electrode pads conventionally applied to the cortex to targeted electrodes applied to specific regions of the brain (Kuo et al., 2013). There is so far limited data regarding the superiority of HD-tDCS compared to conventional tDCS, outlining another area for further study.
These knowledge gaps notwithstanding, there are broader questions which must be answered prior to the advent of neurostimulation in the training or live theater environment. Current data indicates that tDCS is safe, however there no studies investigating the long-term effect of stimulation on surgeons' motor skills. In addition, the added benefit of using neurostimulation must be based on enough evidence to warrant not only the adverse effects, but also the technical challenges of deploying devices such as fNIRS or tDCS in real operating theater environments. Currently, demonstrated effect sizes regarding the advantage of tDCS on surgical skill may not be large enough to justify this, however more data is required to reach a reliable conclusion (Ciechanski et al., 2019). Other factors must also be taken into consideration, such as the opinions of trainees and patients. Whilst data suggests they are supportive of neuroenhancement (Patel et al., 2021), trainees may be reluctant to devote time, money, and effort to new technologies before there is a strong evidence base to prove that these interventions improve outcomes.
Currently, the use of optical neuroimaging in the surgical community is aligned with the historical use of neuroimaging broadly, which is to describe neural response patterns. This has enabled the identification of differential PFC, SMA, M1, and PMA activation, and neural connectivity, in novices and experts in various contexts, including stress conditions. This knowledge can serve to define cognitive benchmarks of expertise, ensuring high standards of objective performance assessment in surgical training (Nemani et al., 2019).
Whilst fNIRS is undoubtably a powerful and accurate observational tool, its added value in surgical training and assessment lies in its potential to not only describe but modulate stress responses, cognitive learning curves, and motor skill performance. Identifying the critical inflection point of the hypothesized inverted “U-curve”, where excess workload causes PFC attenuation, predicting performance deterioration, would allow timely intervention in the moment of motor skill performance, prompting a change in behavior or environment. Over a more prolonged timeframe, frequent visualization of trainee cognitive learning curves allows for direct targeting of specific skills, individualizing the type and quantity of practice necessary to align with the cognitive benchmarks of proficiency previously established. Behavior can be modulated via conscious feedback, the surgeon being made aware of their cognitive state and prompted to change their mindset or movements; it can also be modulated via aforementioned neurostimulatory modalities.
The cornerstone of bringing optical neuroimaging into the operating theater is, however, the successful development of more accurate, real-time, data processing. Two main components of data processing are regression of physiological parameters and motion artifact removal, both which may act as confounders for true cerebral haemodynamic activity. Recent experience with short channel regression and increasingly with systemic physiology measures have enabled the development of models predicting the impact of extra-cerebral factors on haemodynamic activity. Machine learning algorithms utilize such models to improve data quality, bypassing the need for manual correction. In addition to improving the efficiency of processing, combined regression of systemic physiology measurements and short channel fNIRS also provides superior differentiation of the confounders (Scholkmann et al., 2022). Combined systemic-physiology-augmented-fNIRS (SPA-fNIRS) data analysis methods include generalized linear model (GLM) regression and time-embedded-canonical-correlation analysis (Ortega-Martinez et al., 2022), or wavelet transformation (von Lühmann et al., 2020). Deep learning, a type of machine learning, may provide fast, accurate removal of motion artifact within fNIRS data, with noise features being learnt by software removing the need for assumptions regarding the noise characteristics to be described by the research team (Gao et al., 2022). Whilst machine learning is helpful adjunct, it has yet to prove superiority in improving data quality or accurate detection of artifacts as compared to hand-resolved models.
The limitations of diversifying fNIRS research methodology must be recognized, as the variety of hardware and acquisition parameters, lack of standardization of optode array placement and registration, nomenclature of neuroanatomy and data-processing/regression of confounders make literature interpretation and comparison challenging (Hocke et al., 2018; Nemani et al., 2018; Yücel et al., 2021). Signal quality is subject to distortion by environmental and device noise, poor optode coupling, subject motion artifact, and optical interference by coarse/pigmented hair. Yücel outlines consensus guidelines for methodological reporting in an effort to circumvent such challenges, improving interpretation, reliability and reproducibility of studies (Yücel et al., 2021).
FNIRS is a non-invasive optical neuroimaging technique which has been widely used to assess surgeons' brain function. It has described the cognitive activation patterns of novice surgeons, who exhibit greater haemodynamic responses across the pre-frontal cortex than experts when performing simple surgical tasks. Expert surgical performance is characterized by relative prefrontal attenuation and upregulation of activation foci across other motor regions such as the supplementary motor area. In addition to localized changes in activation, greater connectivity between motor areas is noted in experienced surgeons, whilst novices demonstrate stronger connectivity in prefrontal and premotor regions. The neural effect of stress has been hypothesized to follow an inverted “U-shape”, with critical workload leading to a decrease in pre-frontal activation and deterioration in performance; trainees who maintain technical performance under stress demonstrate less PFC attenuation. Neural correlates of expertise and optimal performance suggest optical neuroimaging is a suitable tool to assess proficiency since neuroimages perform comparably, if not better, than current measures of aptitude, and could depict a cognitive state during surgery leading to the development of cognitive benchmarks of expertise. Beyond the observational abilities of optical neuroimaging, it has the potential to observe identify performance deterioration and trigger a reaction, individualizing training programs to optimize the learning curve, and by direct neurostimulation to accelerate skill acquisition and enhance performance. These future applications of optical neuroimaging and neurostimulation in the live operative environment rely upon the development of improved data processing.
MG, VC, DL, and RP contributed to conception and design of the work and drafted the work. DL, RP, FO-E, and HM revised it critically for important intellectual content. MG, VC, RP, HM, AD, FO-E, and DL provide approval for publication of the content and agree to be accountable for all aspects of the work in ensuring that questions related to the accuracy or integrity of any part of the work are appropriately investigated and resolved. All authors contributed to the article and approved the submitted version.
MG was supported by HEE Specialized Foundation post and acknowledges infrastructure support for this research from the National Institute for Health Research (NIHR) Imperial Biomedical Research Center (BRC).
The authors declare that the research was conducted in the absence of any commercial or financial relationships that could be construed as a potential conflict of interest.
All claims expressed in this article are solely those of the authors and do not necessarily represent those of their affiliated organizations, or those of the publisher, the editors and the reviewers. Any product that may be evaluated in this article, or claim that may be made by its manufacturer, is not guaranteed or endorsed by the publisher.
CO2, Carbon dioxide; EEG, Electroencephalogram; fMRI, Functional magnetic resonance imaging; fNIRS, Functional near infrared spectroscopy; GEL, Gaze-enhanced learning; GLM, Generalized Linear Model; HbO2, Oxygenated hemoglobin; HD-tDCS, High definition transcranial direct-current stimulation; HHb, Deoxygenated hemoglobin; M1, Motor area; PET, Positron emission tomography; PFC, Prefrontal cortex; PMA, Premotor area; SMA, Supplementary motor area; SPA-fNIRS, Systemic-physiology-augmented functional near-infrared spectroscopy; tDCS, Transcranial direct-current stimulation.
Aggarwal, R., Ward, J., Balasundaram, I., Sains, P., Athanasiou, T., and Darzi, A. (2007). Proving the effectiveness of virtual reality simulation for training in laparoscopic surgery. Ann. Surg. 246, 771–779. doi: 10.1097/SLA.0b013e3180f61b09
Andreu-Perez, J., Leff, D. R., Shetty, K., Darzi, A., and Yang, G. (2016). Disparity in frontal lobe connectivity on a complex bimanual motor task aids in classification of operator skill level. Brain Connect. 6, 375–388. doi: 10.1089/brain.2015.0350
Arora, S., Sevdalis, N., Nestel, D., Woloshynowych, M., Darzi, A., and Kneebone, R. (2010). The impact of stress on surgical performance: a systematic review of the literature. Surgery 147, 318–316. doi: 10.1016/j.surg.2009.10.007
Bann, S. D., Khan, M. S., and Darzi, A. W. (2003). Measurement of surgical dexterity using motion analysis of simple bench tasks. World J. Surg. 27, 390–394. doi: 10.1007/s00268-002-6769-7
Batsikadze, G., Moliadze, V., Paulus, W., Kuo, M. F., and Nitsche, M. A. (2013). Partially non-linear stimulation intensity-dependent effects of direct current stimulation on motor cortex excitability in humans. J. Physiol. 591, 1987–2000. doi: 10.1113/jphysiol.2012.249730
Biabani, M., Aminitehrani, M., Zoghi, M., Farrell, M., Egan, G., and Jaberzadeh, S. (2018). The effects of transcranial direct current stimulation on short-interval intracortical inhibition and intracortical facilitation: a systematic review and meta-analysis. Rev. Neurosci. 29, 99–114. doi: 10.1515/revneuro-2017-0023
Bikson, M., Grossman, P., Thomas, C., Zannou, A. L., Jiang, J., Adnan, T., et al. (2016). Safety of transcranial direct current stimulation: evidence based update 2016. Brain Stimul. 9, 641–661. doi: 10.1016/j.brs.2016.06.004
Bogdanov, M., and Schwabe, L. (2016). Transcranial stimulation of the dorsolateral prefrontal cortex prevents stress-induced working memory deficits. J. Neurosci. 36, 1429. doi: 10.1523/JNEUROSCI.3687-15.2016
Brigadoi, S., and Cooper, R. J. (2015). How short is short? Optimum source–detector distance for short-separation channels in functional near-infrared spectroscopy. Neurophotonics 2, 025005. doi: 10.1117/1.NPh.2.2.025005
Buch, E. R., Santarnecchi, E., Antal, A., Born, J., Celnik, P. A., Classen, J., et al. (2017). Effects of tDCS on motor learning and memory formation: a consensus and critical position paper. Clin. Neurophysiol. 128, 589–603. doi: 10.1016/j.clinph.2017.01.004
Caldwell, M., Scholkmann, F., Wolf, U., Wolf, M., Elwell, C., and Tachtsidis, I. (2016). Modelling confounding effects from extracerebral contamination and systemic factors on functional near-infrared spectroscopy. Neuroimage 143, 91–105. doi: 10.1016/j.neuroimage.2016.08.058
Carlén, M. (2017). What constitutes the prefrontal cortex?. Science. 358, 478–482. doi: 10.1126/science.aan8868
Chandra, V., Nehra, D., Parent, R., Woo, R., Reyes, R., Hernandez-Boussard, T., et al. (2010). A comparison of laparoscopic and robotic assisted suturing performance by experts and novices. Surgery 147, 830–839. doi: 10.1016/j.surg.2009.11.002
Chen, W., Wagner, J., Heugel, N., Sugar, J., Lee, Y., Conant, L., et al. (2020). Functional near-infrared spectroscopy and its clinical application in the field of neuroscience: advances and future directions. Front. Neurosci. 14, 724. doi: 10.3389/fnins.2020.00724
Ciechanski, P., Cheng, A., Damji, O., Lopushinsky, S., Hecker, K., Jadavji, Z., et al. (2018). Effects of transcranial direct-current stimulation on laparoscopic surgical skill acquisition. BJS Open 2, 70–78. doi: 10.1002/bjs5.43
Ciechanski, P., Kirton, A., Wilson, B., Williams, C. C., Anderson, S. J., Cheng, A., et al. (2019). Electroencephalography correlates of transcranial direct-current stimulation enhanced surgical skill learning: a replication and extension study. Brain Res. 1725, 146445. doi: 10.1016/j.brainres.2019.146445
Crewther, B. T., Shetty, K., Jarchi, D., Selvadurai, S., Cook, C. J., Leff, D. R., et al. (2016). Skill acquisition and stress adaptations following laparoscopic surgery training and detraining in novice surgeons. Surg. Endosc. 30, 2961–2968. doi: 10.1007/s00464-015-4584-0
Dissanayaka, T., Zoghi, M., Farrell, M., Egan, G. F., and Jaberzadeh, S. (2017). Does transcranial electrical stimulation enhance corticospinal excitability of the motor cortex in healthy individuals? A systematic review and meta-analysis. Eur. J. Neurosci. 46, 1968–1990. doi: 10.1111/ejn.13640
Duty, B., Andonian, S., Ma, Y., Peng, S., Shapiro, E., Dhawan, V., et al. (2012). Correlation of laparoscopic experience with differential functional brain activation: a positron emission tomography study with oxygen 15–labeled water. Arch. Surg. 147, 627–632. doi: 10.1001/archsurg.2012.807
Engelmann, C. R., Neis, J. P., Kirschbaum, C., Grote, G., and Ure, B. M. (2014). A noise-reduction program in a pediatric operation theatre is associated with surgeon's benefits and a reduced rate of complications: a prospective controlled clinical trial. Ann. Surg. 259, 1025–1033. doi: 10.1097/SLA.0000000000000253
Erdogan, S. B., Yücel, M. A., and Akin, A. (2014). Analysis of task-evoked systemic interference in fNIRS measurements: insights from fMRI. Neuroimage 87, 490–504. doi: 10.1016/j.neuroimage.2013.10.024
Gao, Y., Cavuoto, L., Dutta, A., Kruger, U., Yan, P., Nemani, A., et al. (2021a). Decreasing the surgical errors by neurostimulation of primary motor cortex and the associated brain activation via neuroimaging. Front. Neurosci. 15, 651192. doi: 10.3389/fnins.2021.651192
Gao, Y., Chao, H., Cavuoto, L., Yan, P., Kruger, U., Norfleet, J. E., et al. (2022). Deep learning-based motion artifact removal in functional near-infrared spectroscopy. Neurophotonics 9, 041406. doi: 10.1117/1.NPh.9.4.041406
Gao, Y., Yan, P., Kruger, U., Cavuoto, L., Schwaitzberg, S., De, S., et al. (2021b). Functional brain imaging reliably predicts bimanual motor skill performance in a standardized surgical task. IEEE Trans. Biomed. Eng. 68, 2058–2066. doi: 10.1109/TBME.2020.3014299
Hannah, T. C., Turner, D., Kellner, R., Bederson, J., Putrino, D., and Kellner, C. P. (2022). Neuromonitoring correlates of expertise level in surgical performers: a systematic review. Front. Hum. Neurosci. 16, 705238. doi: 10.3389/fnhum.2022.705238
Hocke, L. M., Oni, I. K., Duszynski, C. C., Corrigan, A. V., Frederick, B. d., et al. (2018). Automated processing of fNIRS data-a visual guide to the pitfalls and consequences. Algorithms 11, 67. doi: 10.3390/a11050067
Hogle, N. J., Chang, L., Strong, V. E. M., Welcome, A. O. U., Sinaan, M., Bailey, R., et al. (2009). Validation of laparoscopic surgical skills training outside the operating room: a long road. Surg. Endosc. 23, 1476–1482. doi: 10.1007/s00464-009-0379-5
Hung, C., Zeng, B., Zeng, B., Sun, C., Cheng, Y., Su, K., et al. (2021). The efficacy of transcranial direct current stimulation in enhancing surgical skill acquisition: a preliminary meta-analysis of randomized controlled trials. Brain Sci. 11, 707. doi: 10.3390/brainsci11060707
Izzetoglu, K., Aksoy, M. E., Agrali, A., Kitapcioglu, D., Gungor, M., and Simsek, A. (2021). Studying brain activation during skill acquisition via robot-assisted surgery training. Brain Sci. 11, 937. doi: 10.3390/brainsci11070937
James, D. R. C., Leff, D. R., Orihuela-Espina, F., Kwok, K., Mylonas, G. P., Athanasiou, T., et al. (2013). Enhanced frontoparietal network architectures following “gaze-contingent” versus “free-hand” motor learning. Neuroimage 64, 267–276. doi: 10.1016/j.neuroimage.2012.08.056
Kamat, A., Norfleet, J., Intes, X., Dutta, A., and De, S. (2022). Perception action cycle-related brain networks differentiate experts and novices: a combined EEG, fNIRS study during a complex surgical motor task. Proc.SPIE 11945, 1194508. doi: 10.1117/12.2610063
Kiani, M., Andreu-Perez, J., Hagras, H., Papageorgiou, E. I., Prasad, M., and Lin, C.-. (2022). Effective brain connectivity for fNIRS with fuzzy cognitive maps in neuroergonomics. IEEE Trans. Cogn. Dev. Syst. 14, 50–63. doi: 10.1109/TCDS.2019.2958423
Kirilina, E., Jelzow, A., Heine, A., Niessing, M., Wabnitz, H., Brühl, R., et al. (2012). The physiological origin of task-evoked systemic artefacts in functional near infrared spectroscopy. Neuroimage 61, 70–81. doi: 10.1016/j.neuroimage.2012.02.074
Kuo, H. I., Bikson, M., Datta, A., Minhas, P., Paulus, W., Kuo, M. F., et al. (2013). Comparing cortical plasticity induced by conventional and high-definition 4 × 1 ring tDCS: a neurophysiological study. Brain Stimul. 6, 644–648. doi: 10.1016/j.brs.2012.09.010
Leff, D. R., Yongue, G., Vlaev, I., Orihuela-Espina, F., James, D., Taylor, M. J., et al. (2017). “Contemplating the next maneuver”: functional neuroimaging reveals intraoperative decision-making strategies. Ann. Surg. 265, 320–330. doi: 10.1097/SLA.0000000000001651
Lindauer, U., Dirnagl, U., Füchtemeier, M., Böttiger, C., Offenhauser, N., Leithner, C., et al. (2010). Pathophysiological interference with neurovascular coupling - when imaging based on hemoglobin might go blind. Front. Neuroenerg. 2, 25. doi: 10.3389/fnene.2010.00025
Luft, A. R., and Buitrago, M. M. (2005). Stages of motor skill learning. Mol. Neurobiol. 32, 205–216. doi: 10.1385/MN:32:3:205
Modi, H. N., Singh, H., Darzi, A., and Leff, D. R. (2020). Multitasking and time pressure in the operating room: impact on surgeons' brain function. Ann. Surg. 272, 648–657. doi: 10.1097/SLA.0000000000004208
Modi, H. N., Singh, H., Fiorentino, F., Orihuela-Espina, F., Athanasiou, T., Yang, G., et al. (2019). Association of residents' neural signatures with stress resilience during surgery. JAMA Surg. 154, e192552. doi: 10.1001/jamasurg.2019.2552
Modi, H. N., Singh, H., Orihuela-Espina, F., Athanasiou, T., Fiorentino, F., Yang, G., et al. (2018). Temporal stress in the operating room: brain engagement promotes “Coping” and disengagement prompts “Choking”. Ann. Surg. 267, 683–691. doi: 10.1097/SLA.0000000000002289
Modi, H. N., Singh, H., Yang, G., Darzi, A., and Leff, D. R. (2017a). A decade of imaging surgeons' brain function (part I): terminology, techniques, and clinical translation. Surgery 162, 1121–1130. doi: 10.1016/j.surg.2017.05.021
Modi, H. N., Singh, H., Yang, G., Darzi, A., and Leff, D. R. (2017b). A decade of imaging surgeons' brain function (part II): a systematic review of applications for technical and nontechnical skills assessment. Surgery 162, 1130–1139. doi: 10.1016/j.surg.2017.09.002
Morya, E., Monte-Silva, K., Bikson, M., Esmaeilpour, Z., Biazoli, C. E. Jr, Fonseca, A., et al. (2019). Beyond the target area: an integrative view of tDCS-induced motor cortex modulation in patients and athletes. J. Neuroeng Rehabil. 16, 141–141. doi: 10.1186/s12984-019-0581-1
Munz, Y., Almoudaris, A. M., Moorthy, K., Dosis, A., Liddle, A. D., and Darzi, A. W. (2007). Curriculum-based solo virtual reality training for laparoscopic intracorporeal knot tying: objective assessment of the transfer of skill from virtual reality to reality. Am. J. Surg. 193, 774–783. doi: 10.1016/j.amjsurg.2007.01.022
Mylonas, G. P., Kwok, K., James, D. R. C., Leff, D., Orihuela-Espina, F., Darzi, A., et al. (2012). Gaze-Contingent Motor Channelling, haptic constraints and associated cognitive demand for robotic MIS. Med. Image Anal. 16, 612–631. doi: 10.1016/j.media.2010.07.007
Nemani, A., Kamat, A., Gao, Y., Yucel, M. A., Gee, D., Cooper, C., et al. (2021). Functional brain connectivity related to surgical skill dexterity in physical and virtual simulation environments. Neurophotonics 8, 015008. doi: 10.1117/1.NPh.8.1.015008
Nemani, A., Kruger, U., Cooper, C. A., Schwaitzberg, S. D., Intes, X., and De, S. (2019). Objective assessment of surgical skill transfer using non-invasive brain imaging. Surg. Endosc. 33, 2485–2494. doi: 10.1007/s00464-018-6535-z
Nemani, A., Yücel, M. A., Kruger, U., Gee, D. W., Cooper, C., Schwaitzberg, S. D., et al. (2018). Assessing bimanual motor skills with optical neuroimaging. Sci. Adv. 4, eaat3807. doi: 10.1126/sciadv.aat3807
Ohuchida, K., Kenmotsu, H., Yamamoto, A., Sawada, K., Hayami, T., Morooka, K., et al. (2009). The frontal cortex is activated during learning of endoscopic procedures. Surg. Endosc. 23, 2296. doi: 10.1007/s00464-008-0316-z
Ortega-Martinez, A., von Lühmann, A., Farzam, P., Rogers, D., Mugler, E., Boas, D. A., et al. (2022). Multivariate Kalman filter regression of confounding physiological signals for real-time classification of fNIRS data. Neurophotonics 9, 025003. doi: 10.1117/1.NPh.9.2.025003
Ossewaarde, L., Qin, S., Van Marle, H. J. F., van Wingen, G. A., Fernández, G., and Hermans, E. J. (2011). Stress-induced reduction in reward-related prefrontal cortex function. Neuroimage 55, 345–352. doi: 10.1016/j.neuroimage.2010.11.068
Patel, R., Ashcroft, J., Patel, A., Ashrafian, H., Woods, A. J., Singh, H., et al. (2019). The impact of transcranial direct current stimulation on upper-limb motor performance in healthy adults: a systematic review and meta-analysis. Front. Neurosci. 13, 1213. doi: 10.3389/fnins.2019.01213
Patel, R., Dawidziuk, A., Darzi, A., Singh, H., and Leff, D. R. (2020). Systematic review of combined functional near-infrared spectroscopy and transcranial direct-current stimulation studies. Neurophotonics 7, 020901. doi: 10.1117/1.NPh.7.2.020901
Patel, R., Rai, A., Thornton-Wood, F., Wilkinson, A., Darzi, A., Singh, H., et al. (2021). Neuroenhancement of future surgeons – Opinions from students, surgeons and patients. Brain Stimul. 14, 616–618. doi: 10.1016/j.brs.2021.03.012
Paulus, W. (2011). Transcranial electrical stimulation (tES – tDCS; tRNS, tACS) methods. Neuropyschol. Rehabil. 21, 602–617. doi: 10.1080/09602011.2011.557292
Reddy, P., Izzetoglu, M., Shewokis, P. A., Sangobowale, M., Diaz-Arrastia, R., and Izzetoglu, K. (2021). Evaluation of fNIRS signal components elicited by cognitive and hypercapnic stimuli. Sci. Rep. 11, 23457–23457. doi: 10.1038/s41598-021-02076-7
Scholkmann, F., Tachtsidis, I., Wolf, M., and Wolf, U. (2022). Systemic physiology augmented functional near-infrared spectroscopy: a powerful approach to study the embodied human brain. Neurophotonics 9, 1–24. doi: 10.1117/1.NPh.9.3.030801
Seymour, N. E., Gallagher, A. G., Roman, S. A., O'Brien, M. K., Bansal, V. K., Andersen, D. K., et al. (2002). Virtual reality training improves operating room performance: results of a randomized, double-blinded study. Ann. Surg. 236, 458–454. doi: 10.1097/00000658-200210000-00008
Shetty, K., Leff, D. R., Orihuela-Espina, F., Yang, G., and Darzi, A. (2016). Persistent prefrontal engagement despite improvements in laparoscopic technical skill. JAMA Surg. 151, 682–684. doi: 10.1001/jamasurg.2016.0050
Singh, H., Modi, H. N., Ranjan, S., Dilley, J. W. R., Airantzis, D., Yang, G. Z., et al. (2018). Robotic surgery improves technical performance and enhances prefrontal activation during high temporal demand. Ann. Biomed. Eng. 46, 1621–1636. doi: 10.1007/s10439-018-2049-z
Stefanidis, D., Wang, F., Korndorffer, J. R. Jr, Dunne, J. B., and Scott, D. J. (2010). Robotic assistance improves intracorporeal suturing performance and safety in the operating room while decreasing operator workload. Surg. Endosc. 24, 377–382. doi: 10.1007/s00464-009-0578-0
Strangman, G. E., Li, Z., and Zhang, Q. (2013). Depth sensitivity and source-detector separations for near infrared spectroscopy based on the Colin27 brain template. PLoS ONE 8, e66319. doi: 10.1371/journal.pone.0066319
Sweller, J. (2011). Cognitive load theory. In: Anonymous. San Diego, CA: Elsevier Academic Press. p. 37–76.
Ungerleider, L. G., Doyon, J., and Karni, A. (2002). Imaging brain plasticity during motor skill learning. Neurobiol. Learn. Mem. 78, 553–564. doi: 10.1006/nlme.2002.4091
von Lühmann, A., Li, X., Müller, K., Boas, D. A., and Yücel, M. A. (2020). Improved physiological noise regression in fNIRS: a multimodal extension of the general linear model using temporally embedded canonical correlation analysis. Neuroimage 208, 116472. doi: 10.1016/j.neuroimage.2019.116472
Walia, P., Fu, Y., Schwaitzberg, S. D., Intes, X., De, S., Cavuoto, L., et al. (2021). Neuroimaging guided tES to facilitate complex laparoscopic surgical tasks - insights from functional near-infrared spectroscopy. Annu. Int. Conf. IEEE Eng. Med. Biol. Soc. 2021, 7437–7440. doi: 10.1109/EMBC46164.2021.9631005
Walia, P., Fu, Y., Schwaitzberg, S. D., Intes, X., De, S., Dutta, A., et al. (2022). Portable neuroimaging differentiates novices from those with experience for the Fundamentals of Laparoscopic Surgery (FLS) suturing with intracorporeal knot tying task. Surg. Endosc. doi: 10.1007/s00464-022-09727-4. [Epub ahead of print].
Yang, J. (2015). The influence of motor expertise on the brain activity of motor task performance: a meta-analysis of functional magnetic resonance imaging studies. Cogn. Affect. Behav. Neurosci. 15, 381–394. doi: 10.3758/s13415-014-0329-0
Keywords: neuroergonomic, functional near-infrared spectroscopy, surgical training, neurostimulation, neuroimaging, neuromonitoring
Citation: Goble M, Caddick V, Patel R, Modi H, Darzi A, Orihuela-Espina F and Leff DR (2023) Optical neuroimaging and neurostimulation in surgical training and assessment: A state-of-the-art review. Front. Neuroergon. 4:1142182. doi: 10.3389/fnrgo.2023.1142182
Received: 11 January 2023; Accepted: 03 March 2023;
Published: 20 March 2023.
Edited by:
Suvranu De, Florida State University, United StatesReviewed by:
Anirban Dutta, University of Lincoln, United KingdomCopyright © 2023 Goble, Caddick, Patel, Modi, Darzi, Orihuela-Espina and Leff. This is an open-access article distributed under the terms of the Creative Commons Attribution License (CC BY). The use, distribution or reproduction in other forums is permitted, provided the original author(s) and the copyright owner(s) are credited and that the original publication in this journal is cited, in accordance with accepted academic practice. No use, distribution or reproduction is permitted which does not comply with these terms.
*Correspondence: Mary Goble, bWFyeS5nb2JsZUBpbXBlcmlhbC5hYy51aw==
Disclaimer: All claims expressed in this article are solely those of the authors and do not necessarily represent those of their affiliated organizations, or those of the publisher, the editors and the reviewers. Any product that may be evaluated in this article or claim that may be made by its manufacturer is not guaranteed or endorsed by the publisher.
Research integrity at Frontiers
Learn more about the work of our research integrity team to safeguard the quality of each article we publish.